📖 Convolution
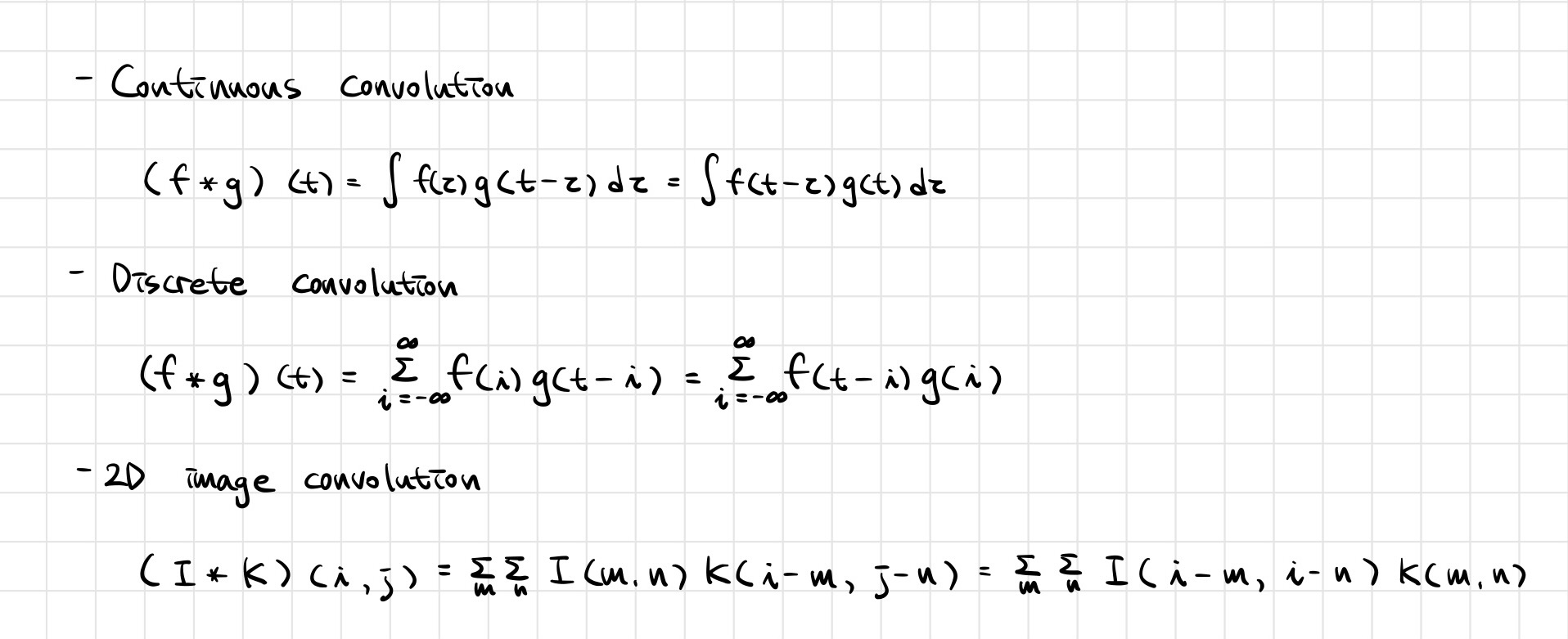
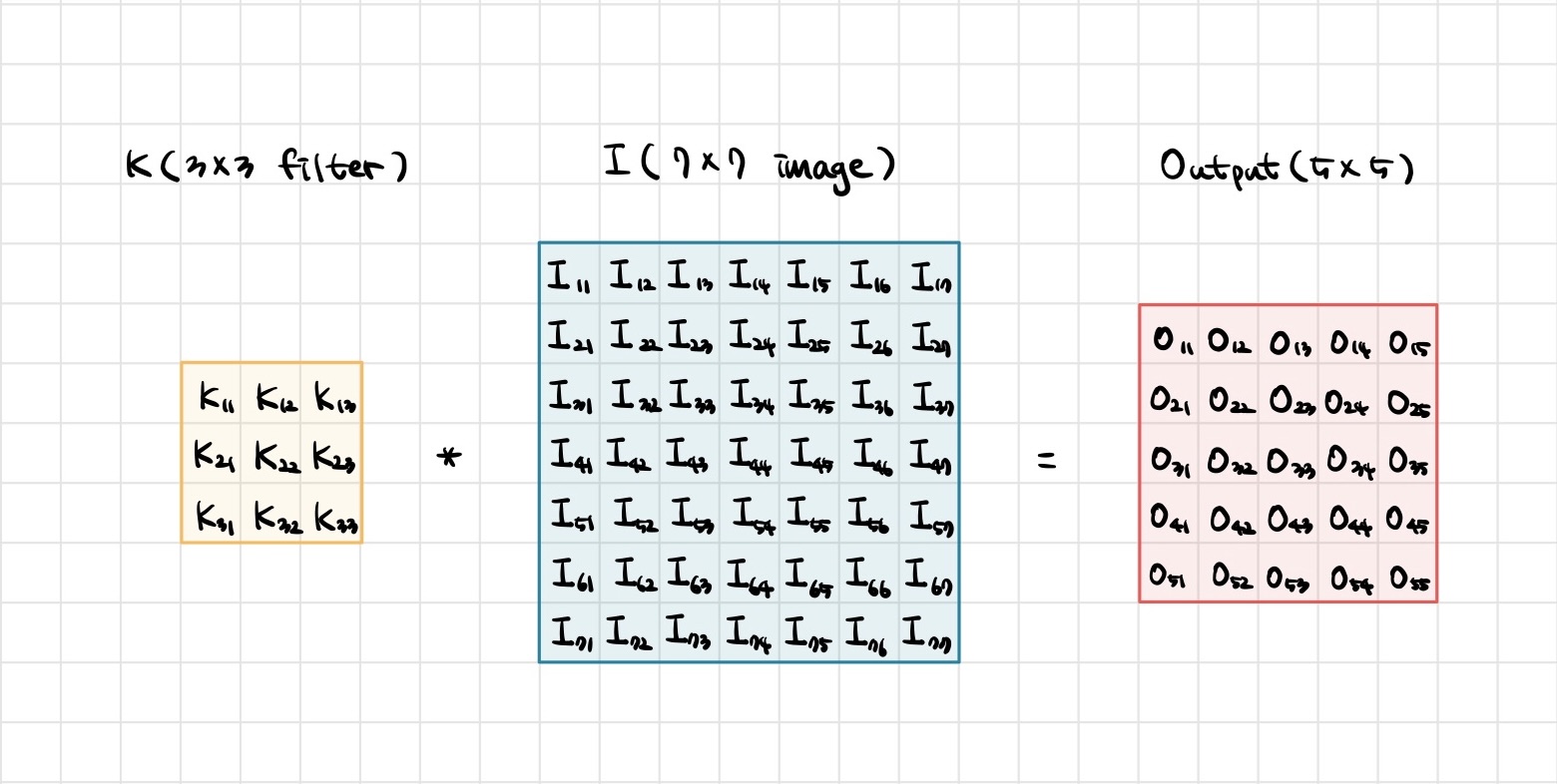
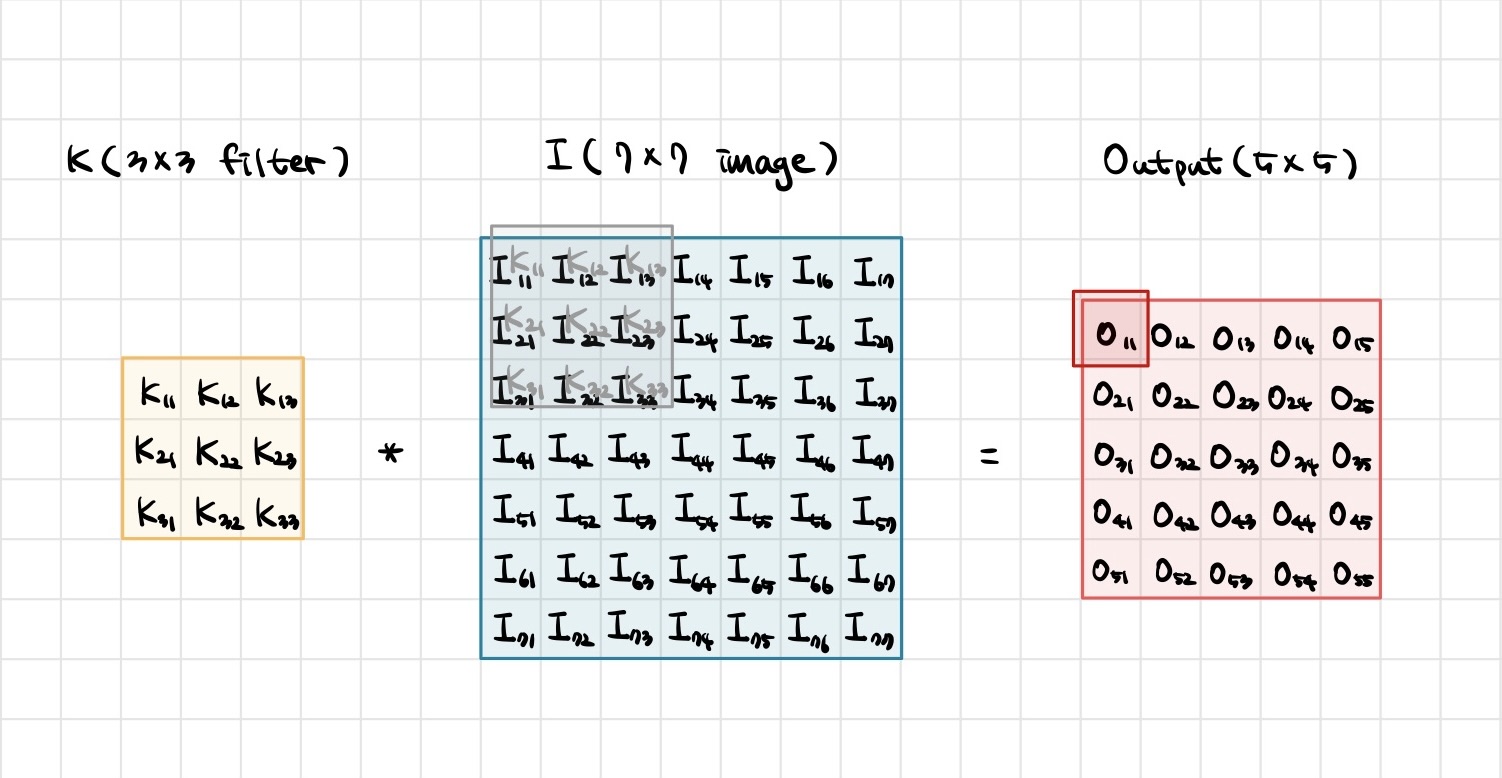
O11=I11K11+I12K12+I13K13+I21K21+I22K22+I23K23+I31K31+I32K32+I33K33+bias
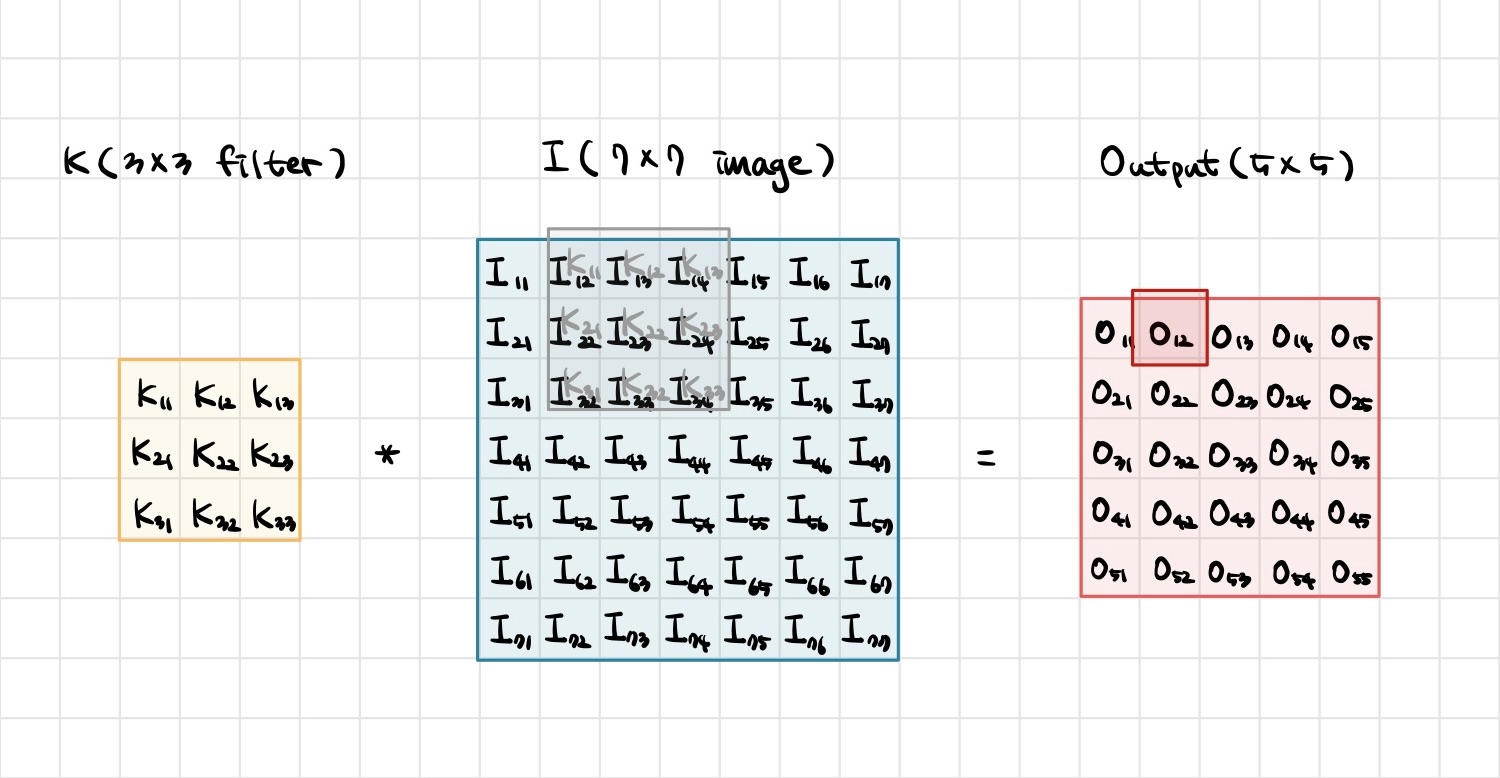
O12=I12K11+I13K12+I14K13+I22K21+I23K22+I24K23+I32K31+I33K32+I34K33+bias
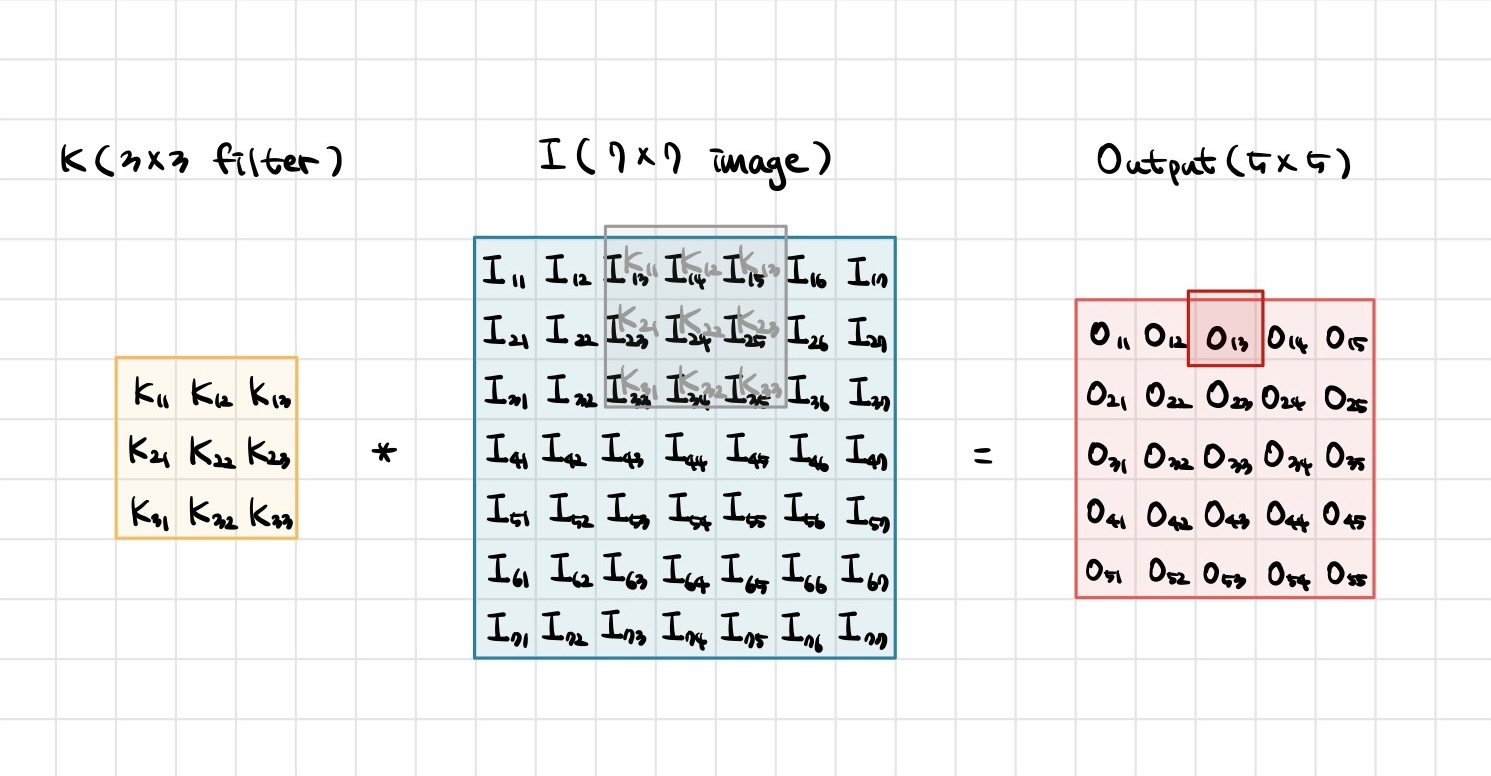
O13=I13K11+I14K12+I15K13+I23K21+I24K22+I25K23+I33K31+I34K32+I35K33+bias
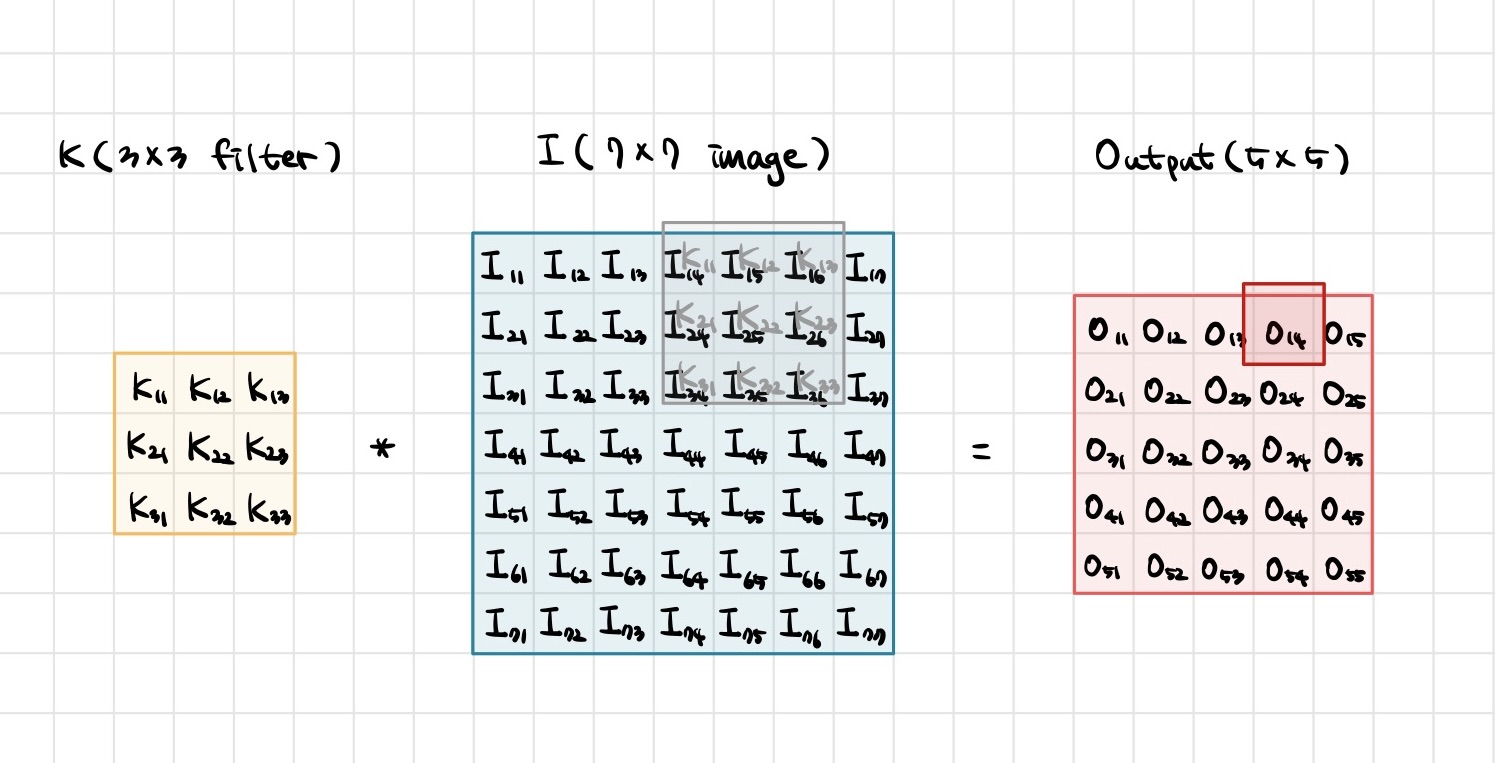
O14=I14K11+I15K12+I16K13+I24K21+I25K22+I26K23+I34K31+I35K32+I36K33+bias
- 2D convolution in action
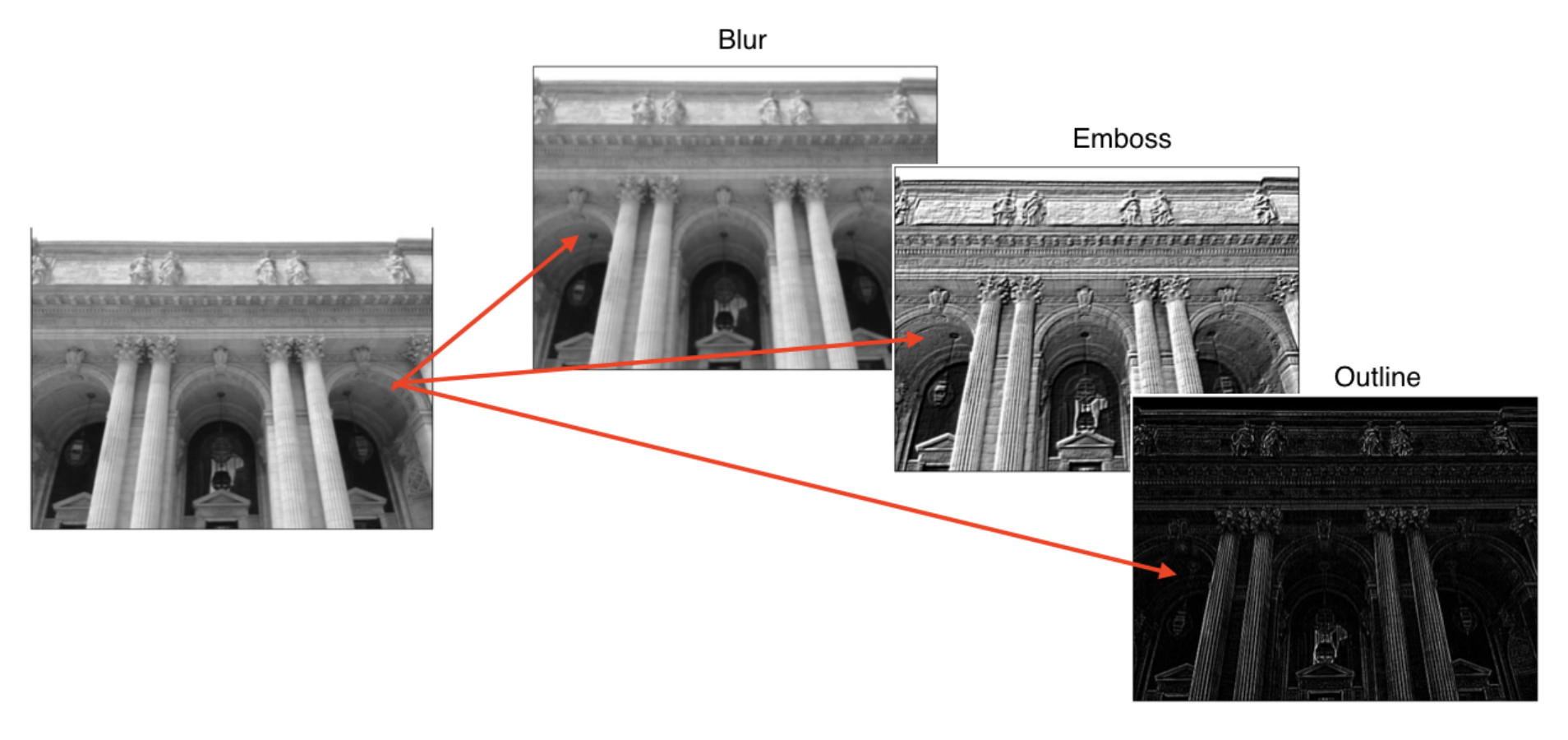
📖 RGB Image Convolution
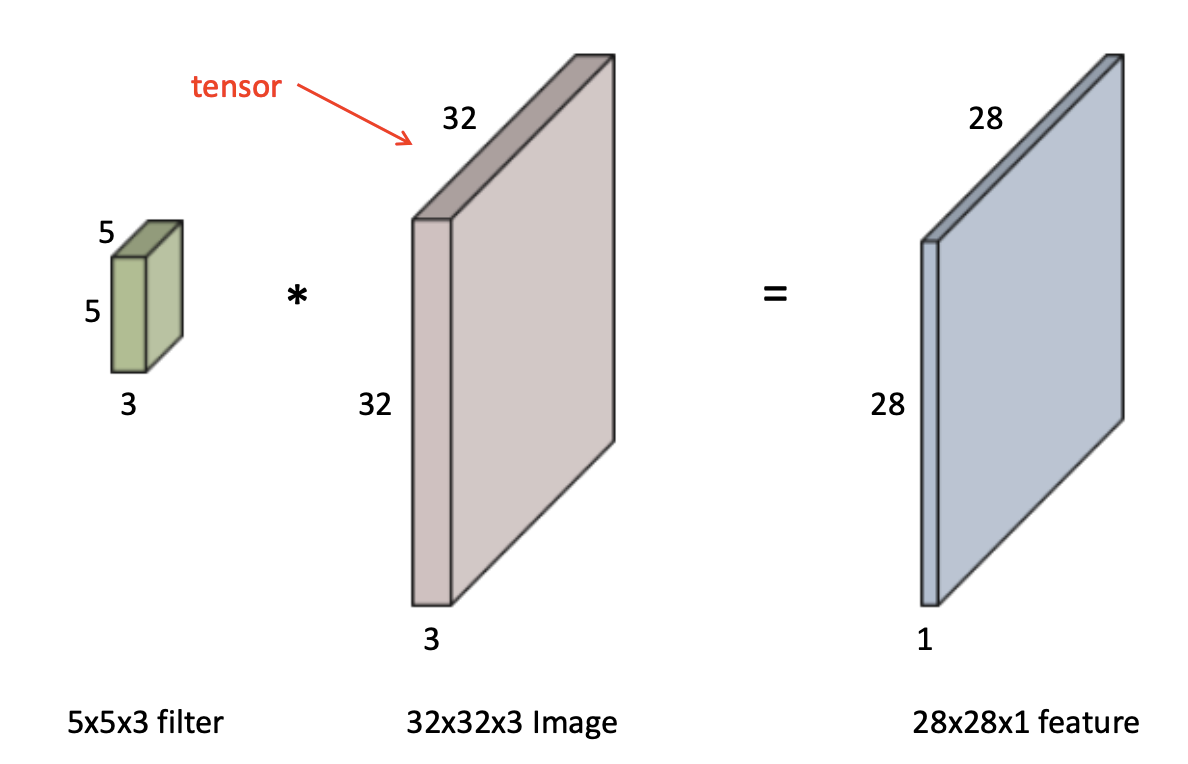
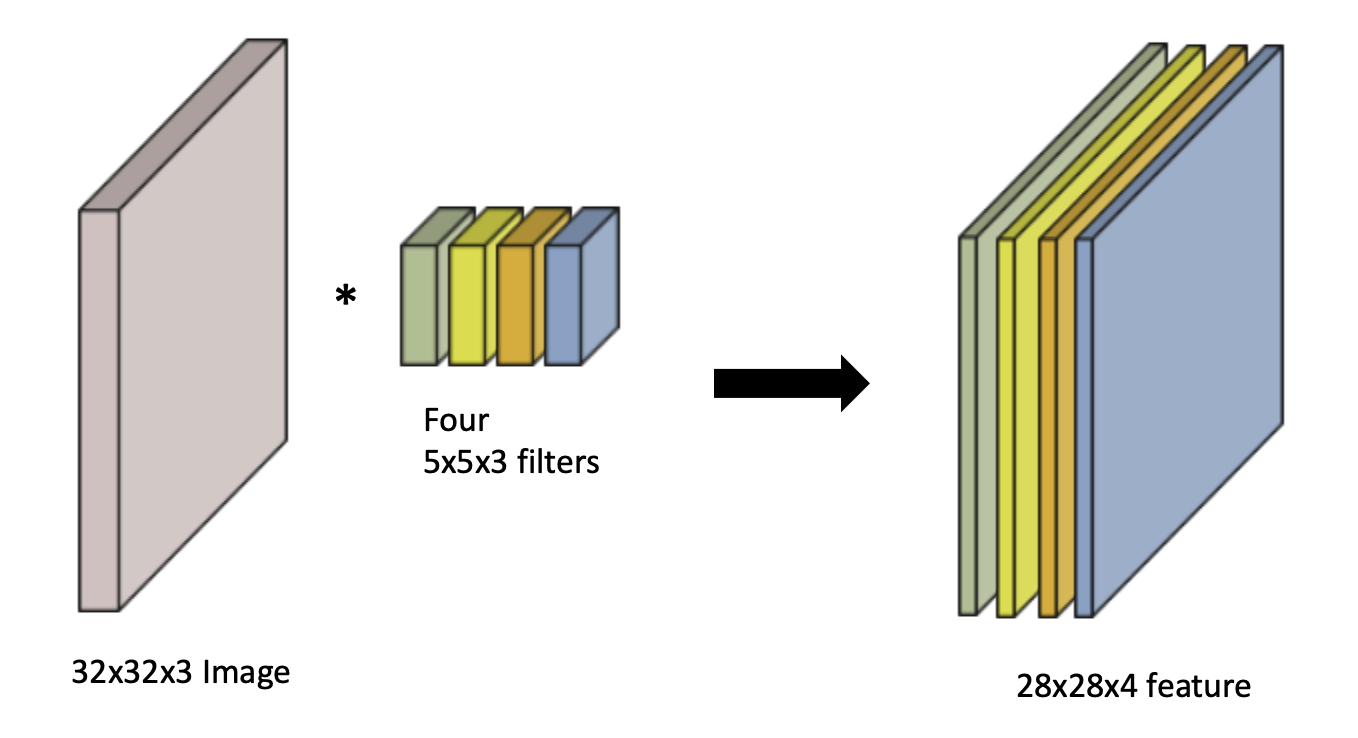
📖 Stack of Convolutions
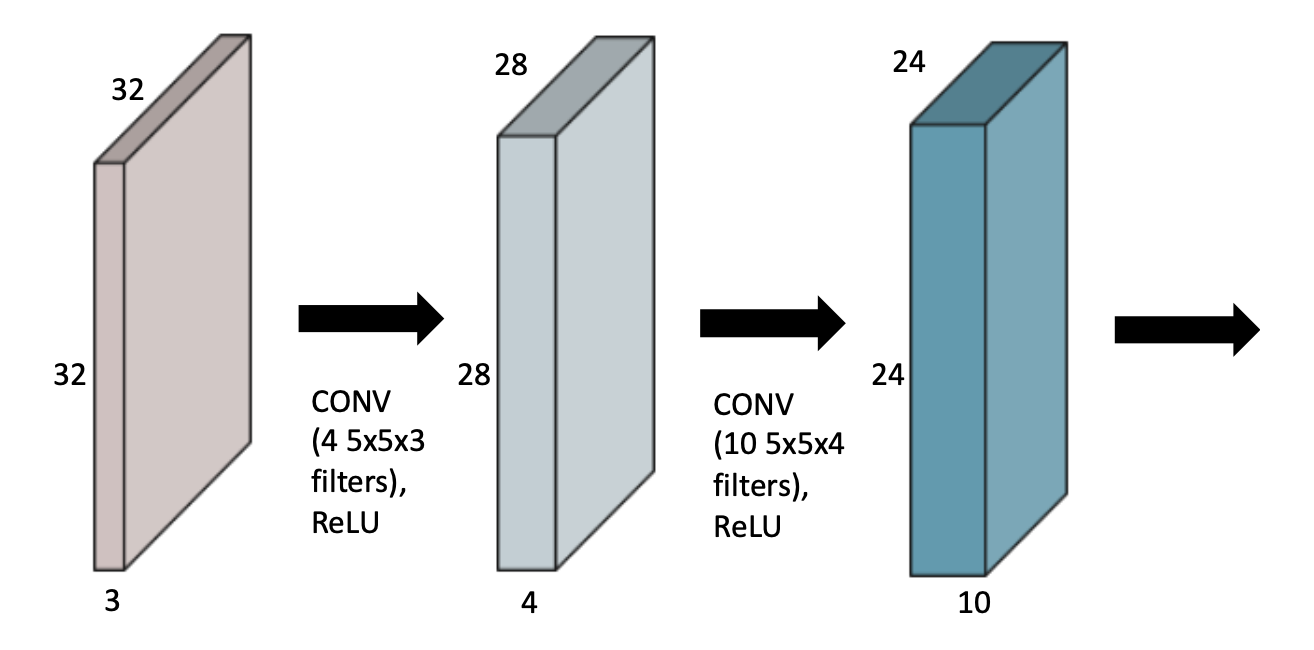
📖 Convolutional Neural Networks
- CNN consists of convolution layer, pooling layer, and fully connected layer.
- Convolution and pooling layers: feature extraction
- Fully connected layer: decision making (e.g., classification)
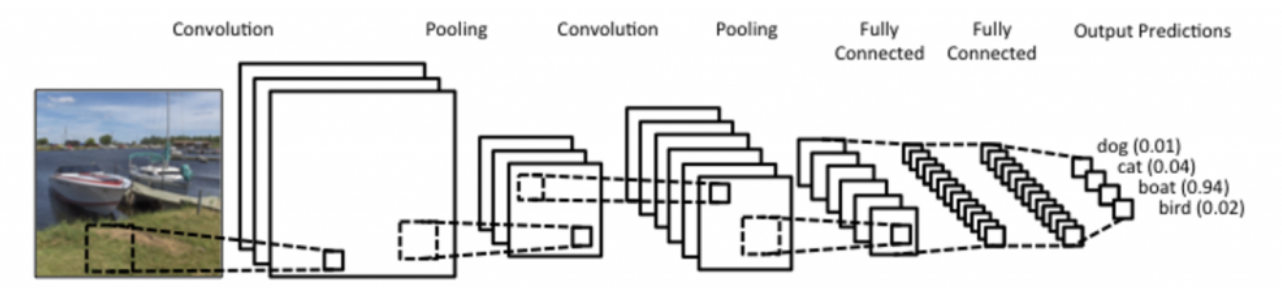
📖 Convolution Arithmetic (of GoogLeNet)
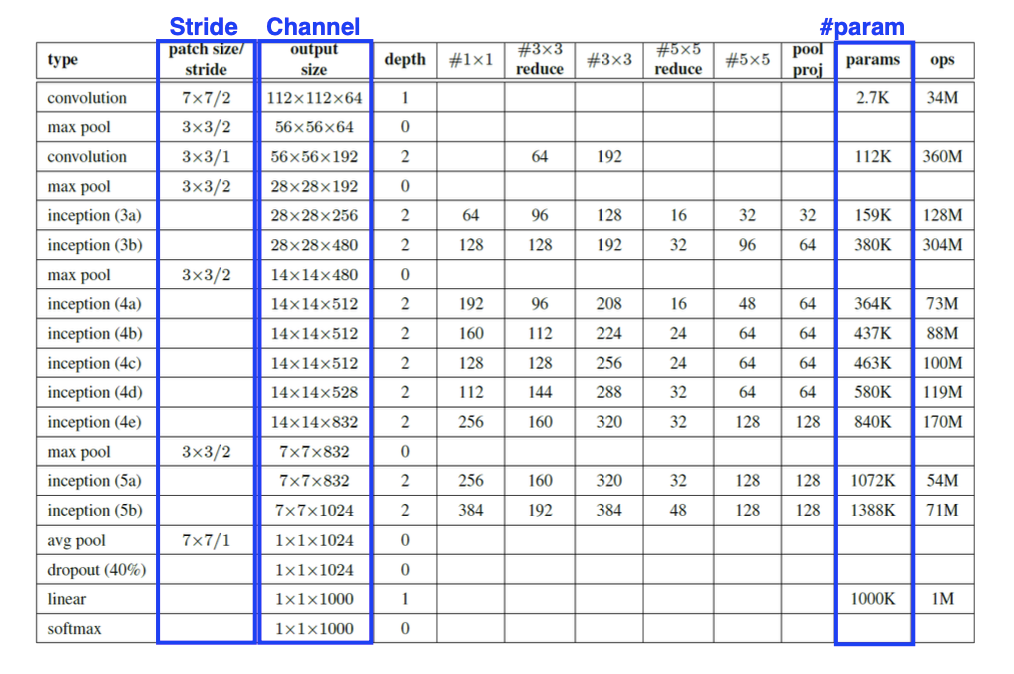
📖 Stride
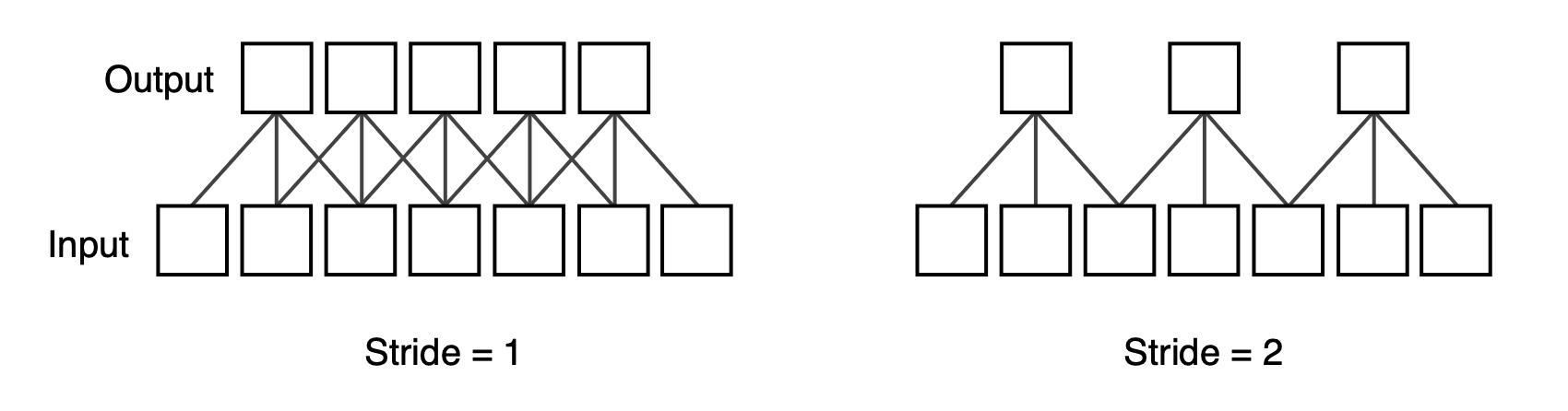
📖 Padding
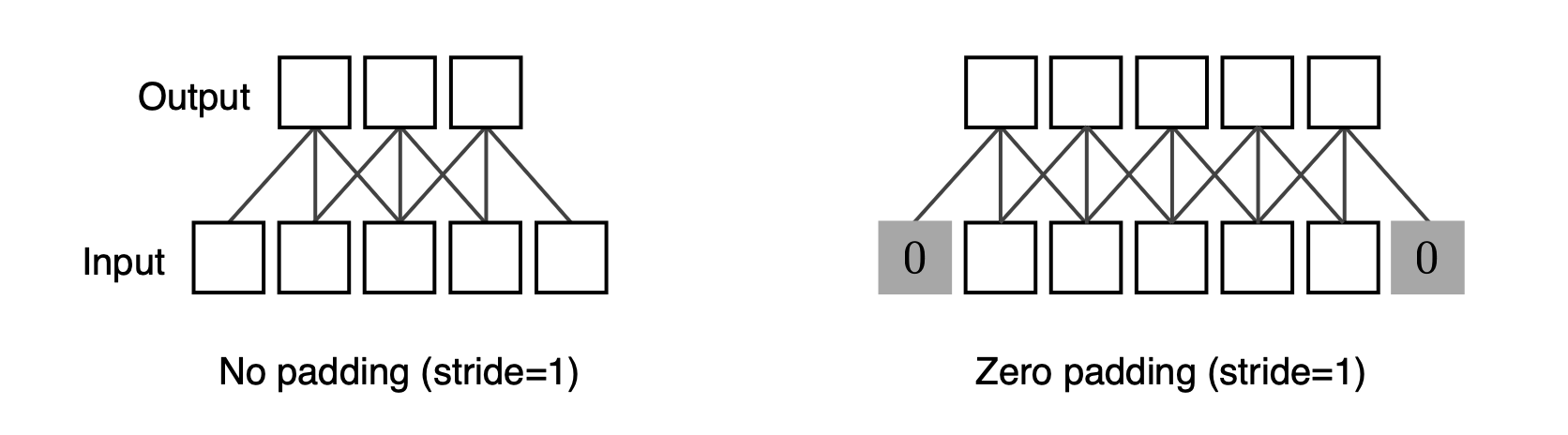
📖 Stride? Padding?
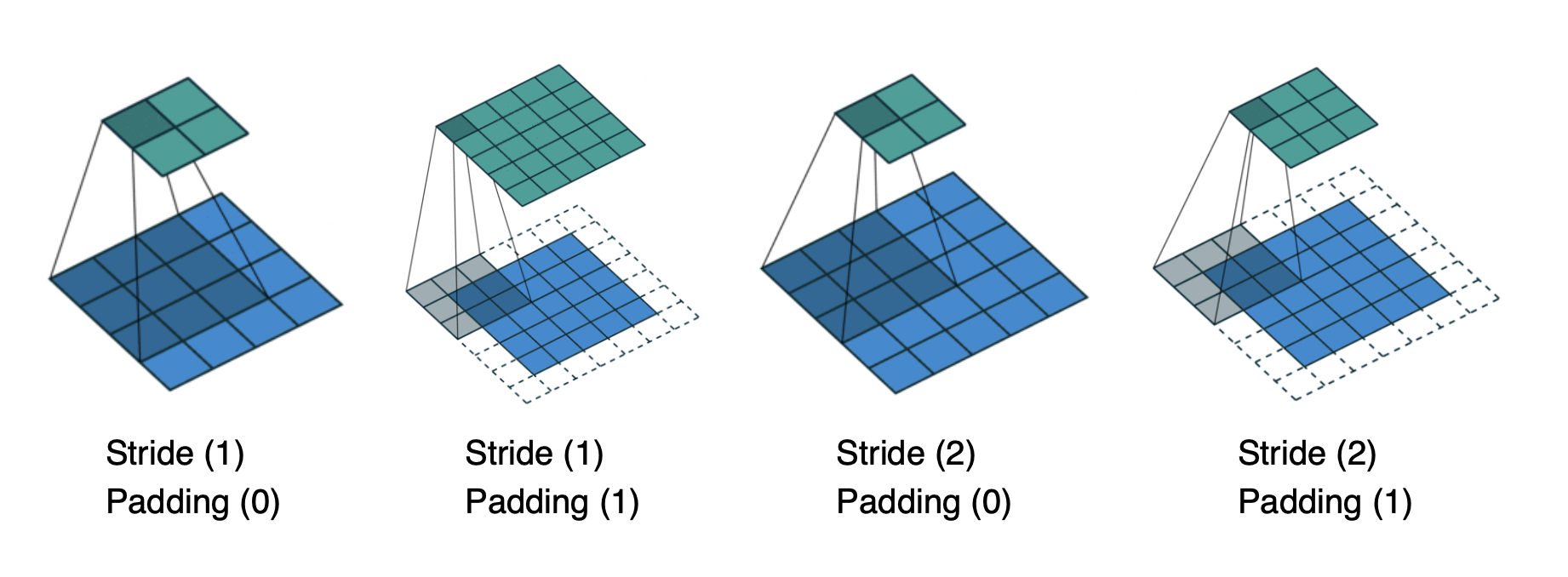
📖 Convolution Arithmetic
-
Padding (1), Stride (1), 3 × 3 Kernel
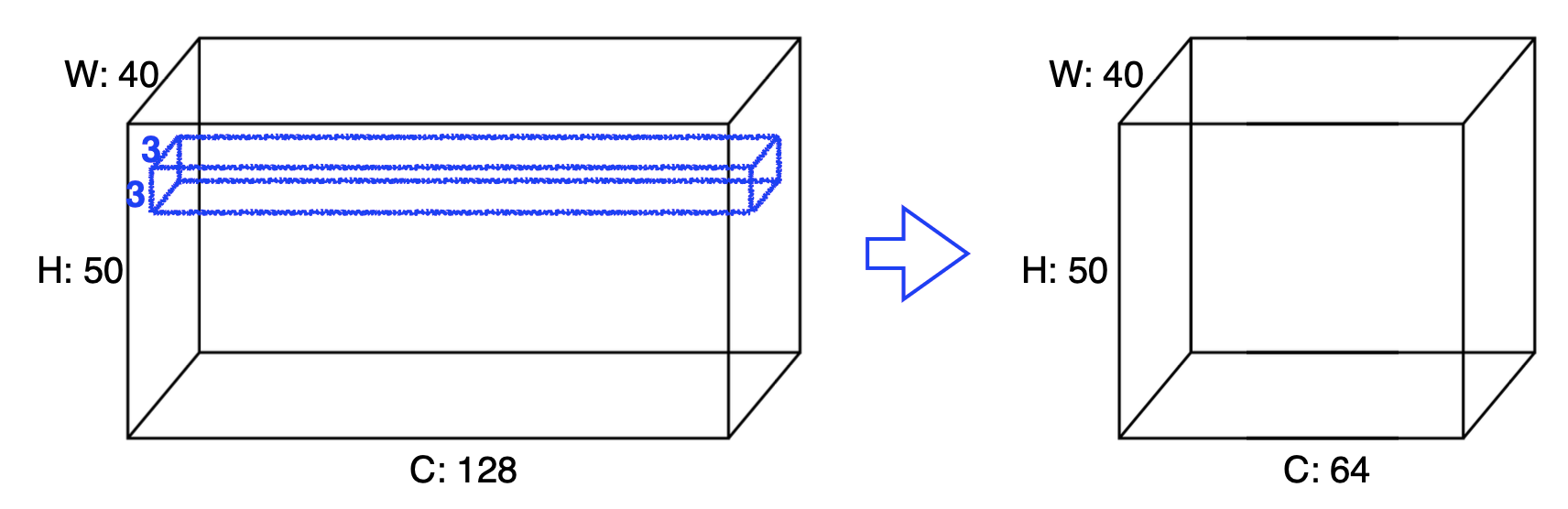
-
What is the number of parameters of this model?
- The answer is 3×3×128×64=73,728
📖 Exercise
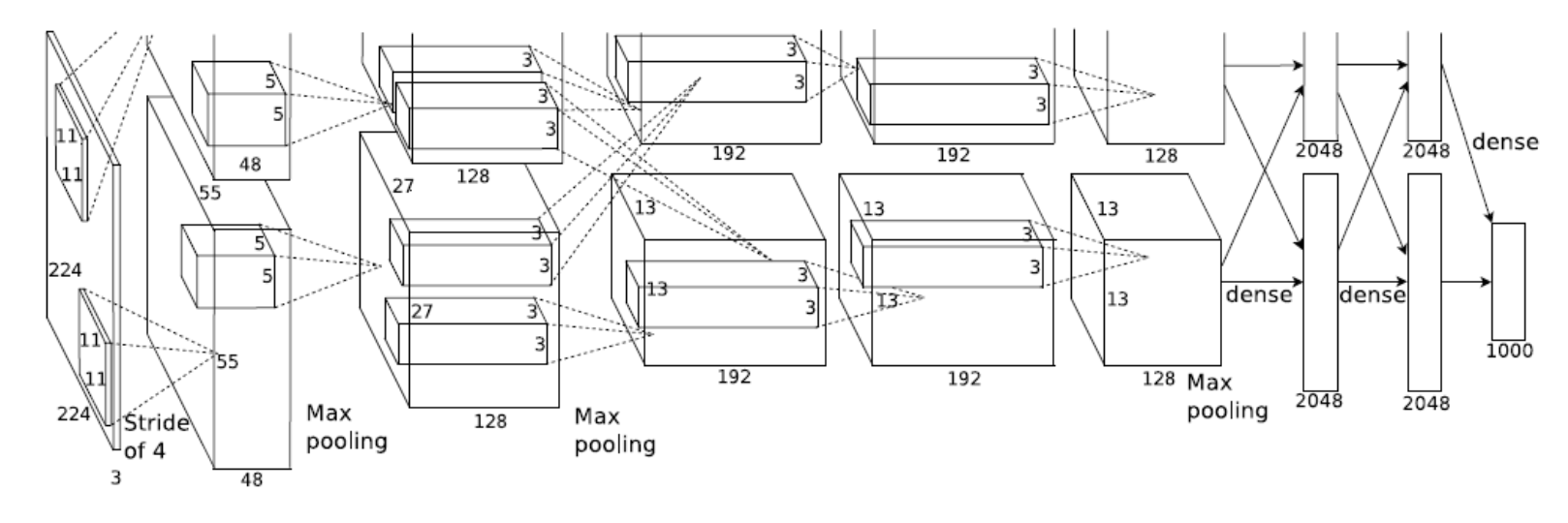
- What is the number of parameters of this model?
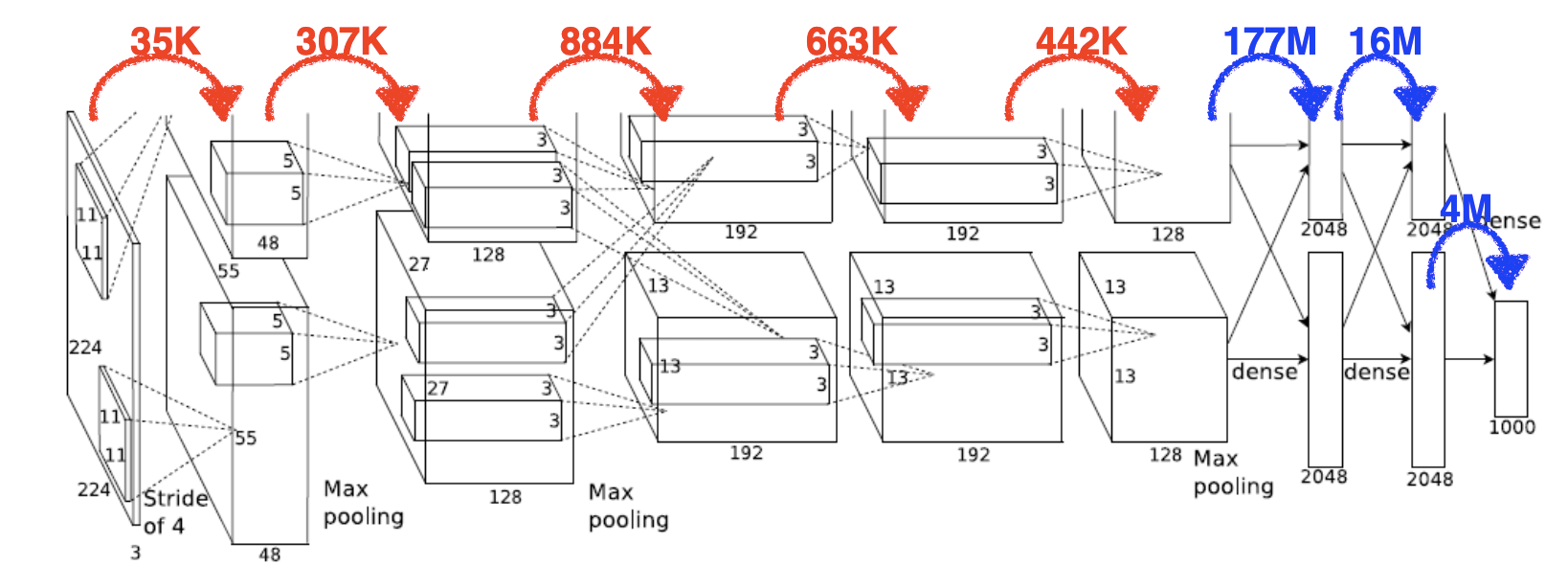
- 1) 11×11×3×48×2≈35k
- 2) 5×5×48×128×2≈307k
- 3) 3×3×128×2×192×2≈884k
- 4) 3×3×192×192×2≈663k
- 5) 3×3×192×128×2≈442k
- 6) 13×13×128×2×2048×2≈177M
- 7) 2048×2×2048×2≈16M
- 8) 2048×2×1000≈4M
📖 1x1 Convolution
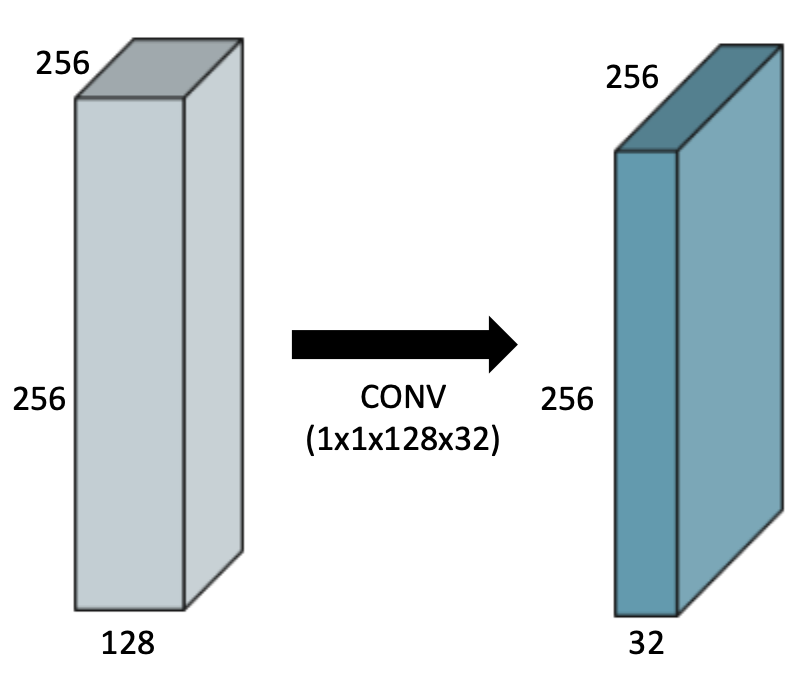
- Why?
- Dimension reduction
- To reduce the number of parameters while increasing the depth
- e.g., bottleneck architecture
<이 게시물은 최성준 교수님의 'Convolutional Neural Networks' 강의 자료를 참고하여 작성되었습니다.>
본 포스트의 학습 내용은 [부스트캠프 AI Tech 5기] Pre-Course 강의 내용을 바탕으로 작성되었습니다.
부스트캠프 AI Tech 5기 Pre-Course는 일정 기간 동안에만 운영되는 강의이며,
AI 관련 강의를 학습하고자 하시는 분들은 부스트코스 AI 강좌에서 기간 제한 없이 학습하실 수 있습니다.
(https://www.boostcourse.org/)