1. Basics of Recurrent Neural Networks(RNNs)
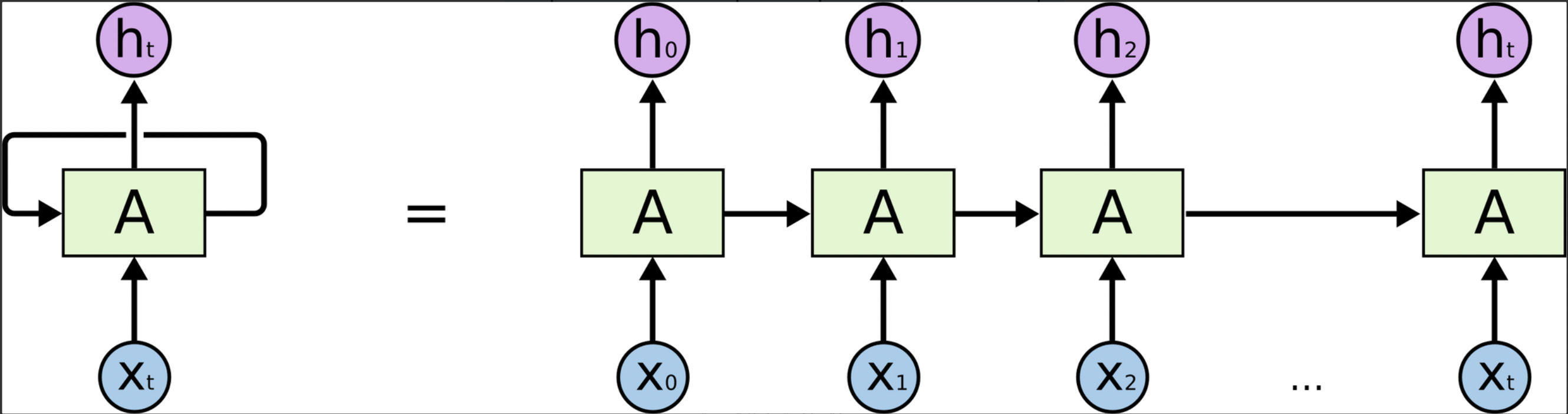
https://colah.github.io/posts/2015-08-Understanding-LSTMs/
Sequence 데이터에 대해서,
각 time step 입력 벡터 xt, 이전 time step의 hidden state 벡터 ht를
input으로 하여 현재 time step의 ht를 출력하는 구조.
- 서로 다른 각각의 time step에서 입력 데이터를 처리할 때, 동일한 parameter을 가진 A를 반복적으로 사용
- ht는 다음 time step에서 입력으로 사용하는 동시에, 현재 time step의 output을 위해 사용됨
Recurrent Neural Network
ht=fW(ht−1,xt)
- ht−1: old hidden state vector → 입력
- xt: input vector at some time step → 입력
- ht: new hidden state vector → 출력
- fW: RNN function with parameter W → Linear transformation matrix
- yt: output vector at time step t → 최종 output(task별로 특정 time step에서 요구)
⇒ fW의 parameter W는 모든 time step에서 동일한 값을 활용
ht=fW(ht−1,xt)→{ht=tanh(Whhht−1+Wxhxt)yt=Whyht
2. Types of RNNs
- One-to-one: Standard Neural Network
- One-to-many: Image Captioning(하나의 이미지에 대한 글을 출력)
- Many-to-one: Sentiment Classification(글에 대한 긍/부정 분류)
- Sequence-to-sequence
- 입력을 다 읽은 후, 예측 수행: Machine Translation
- 입력과 동시에 예측 수행: Video classification on frame level
3. Character-level Language Model
단어 순서 기반 다음 단어 맞추기(Many-to-many)
ex. “hello”
⇒ Vocabulary: [h, e, l, o]
1) h 입력 → e 예측
2) e 입력 → l 예측
3) l 입력 → l 예측 …
- ht=tanh(Whhht−1+Wxhxt+b)
- Logit=Whyht+b
Backpropagation through time(BPTT)
Character-level Language Model 학습 방법의 일종
: 보통 sequence 길이가 너무 길면, 한번에 GPU로 학습하기 어렵기 때문에 truncation을 통해 진행
(한번에 학습하는 sequence 길이를 제한)
Searching for Interpretable Cells
RNN이 필요한 지식을 어떻게 배우는지에 대한 정보 분석(ht). 일종의 역추적.
: 우리가 분석하는 정보가 어느 차원에 저장되는지 역추적
→ ht내 특정 차원을 고정하고 결과가 어떻게 변하는지 분석
Vanishing/Exploding Gradient Problem in RNN
Vanila RNN에서는 Whh를 반복적으로 사용하기 때문에 gradient가 발산 또는 수렴하는 현상 발생.
4. Long Short-Term Memory(LSTM),
Gated Recurrent Unit(GRU)
Long Short-Term Memory(LSTM)
RNN의 Gradient 문제를 해결하여 time step이 먼 경우에도 정보를 보존하도록 구성.
RNN: ht=fW(xt,ht−1) → LSTM: {Ct,ht}=LSTM(xt,Ct−1,ht−1)
Ct: cell state vector = 우리가 보존하려는 정보
ht는 Ct를 한번 더 일종의 가공을 통해 해당 time step에서 노출할 필요가 있는 정보를 남긴 벡터이며 이를 이용해서 해당 time step의 output layer의 input 또는 다음 time step의 input으로 사용됨.
- Input gate(i): Whether to write to cell
- Forget gate(f): Whether to erase cell
- Output gate(o): How much to reveal cell
- Gate gate(g): How much to write to cell
→ i, f, o는 sigmoid를 통과하여 0과 1사이의 값을 얻고 나중에 다른 벡터와 elementwise multiplication 수행
→ g는 tanh를 통과하여 -1과 1사이의 값을 얻으며 hidden state vector 내 유의미한 정보를 저장
⇒ i, f, o, g는 cell state를 적절하게 변환하기 위해 사용됨
Gated Recurrent Unit(GRU)
LSTM을 경량화하여 적은 메모리를 사용하고 계산을 빠르게 하도록 함.
: C와 h를 일원화 하여 효율을 높임
Summary
- RNN의 flexibility
- Vanilla RNNs은 단순한 구조로 인해 잘 작동하지 않음
- RNN 내 Backward flow of gradients은 explode 또는 vanish 문제 존재
- LSTM, GRU → 덧셈 연산을 통해 gradient flow 문제를 개선