딥러닝 학습은 데이터를 수많은 layer을 통과시키며 진행된다.
이 과정에서 동일한 layer가 여러 번 사용되며, 반복의 연속이다.
학습 과정에서 하나의 layer를 블록으로 상상해보면, 각 블록을 쌓고 쌓아서 하나의 완성체를 만드는 것이다.
PyTorch를 이용하면 이러한 반복을 손쉽게 다룰 수 있다.
class MyLinear(nn.Module):
def __init__(self, in_features, out_features, bias=True):
super().__init__()
# 예를 들어, input이 (5,7)이고 output이 (5,12)인 경우 weight는 (7,12)인데,
# 이 때 in_features가 7, out_features가 12이다.
self.in_features = in_features
self.out_features = out_features
# weights를 지정해주는 부분, 실제로 직접 입력할 일은 없다.
self.weights = nn.Parameter(torch.randn(in_featrues, out_features))
# Parameter가 아닌 Tensor로 지정해주어도 방식은 동일하긴 하다.
# 그러나 autograd 지정이 어렵다.
self.bias = nn.Parameter(torch.randn(out_features))
def forward(self, x:Tensor): # y_hat 부분 (xw+b)
return x @ self.weights + self.bias
# @ 연산자는
x = torch.randn(5, 7) # 데이터 5개, feature는 7개.
layer = MyLinear(7, 12)
layer(x).shape # => torch.Size([5, 12])
Backward는 기본적인 4단계를 거쳐서 진행된다.
1. 초기화
2. loss 구하기
3. backward
4. 업데이트
for epoch in range(epochs):
... ...
optimizer.zero_grad() # gradient가 업데이트 될 때, 이전 값이 지금에 영향을 주지 않도록 초기화.
outputs = model(inputs) # y_hat에 해당.
loss = criterion(outputs, labels)
loss.backward() # parameter(weight)에 대해 편미분해서 얻은 gradient
optimizer.step() # 한번에 weight를 업데이트 진행(epoch이 돌아갈 때 마다)
class LR(nn.Module):
def __init__(self, dim, lr=torch.scalar_tensor(0.01)):
super(LR, self).__init__():
# parameter 초기화
self.w = torch.zeros(dim, 1, dtype=torch.float_.to(device)
self.b = torch.scalar_tensor(0).to(device)
self.grads={"dw":torch.zeros(dim, 1, dtype=torch.float).to(device),
"db": torch.scalar_tensor(0).to(device)}
self.lr = lr.to(device)
def forward(self, x): # y_hat 구하기 by Sigmoid
z = torch.mm(self.w.T, x)
a = self.sigmoid(z)
return a
def sigmoid(self, z):
return 1/(1+torch.exp(-z))
def backward(self, x, y_hat, y): # __init__에서 선언한 grads 사용.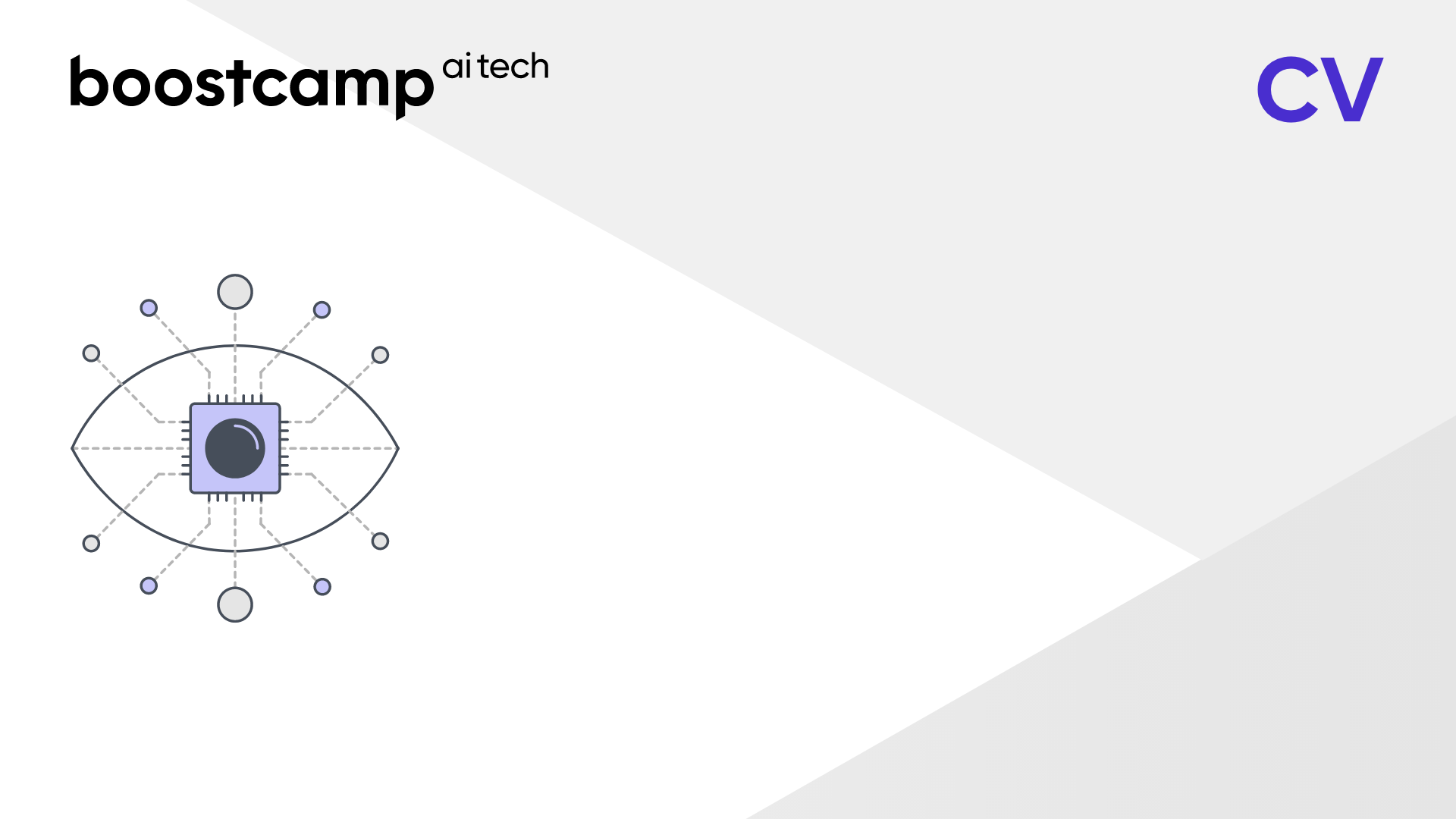
self.grads["dw"] = (1/x.shape[1]) * torch.mm(x, (y_hat-y).T)
self.grads["db"] = (1/x.shape[1]) * torch.sum(y_hat - y)
def optimize(self): # 업데이트 스텝
self.w = self.w - self.lr * self.grads["dw"]
self.b = self.b - self.lr * self.grads["db"]