제목: A decade of structural variants: description, history and methods to detect structural variation (2015) : 리뷰 논문
링크: https://academic.oup.com/bfg/article/14/5/305/184088
0. Abstract
- Structural Variation (SV)은 우연의 현상이라고 여겨져 왔었으나, 이제는 interindividual genetic variation의 가장 큰 원인으로 여겨진다.
- Phenotypic variation과 disease에도 영향을 크게 끼치는 것으로 알려짐
- 이번 논문에서는 SV에 대한 간단한 소개, 역사, 그리고 SV를 detect하는 여러 방법들에 대한 소개
1. Brief Introduction
- SV is generally defined as a region of DNA that shows a change in copy number (deletions, insertions, duplications), orientation (inversions), or chromosomal location (translocations) between individuals
- SV can be balanced, with no loss or gain of genetic material, such as inversions of genetic fragments or translocations of a stretch of DNA within or between chromosomes.
- SV can be unbalanced, where a part of the genome is lost or duplicated, which is termed copy number variation (CNV). (ex: Chromosomal aneuploidies would be an extreme case of unbalanced SV)
2. History of SV
- Genomic SV의 존재 자체는 예전부터 널리 알려져 있었다. (karyotyping과 같이 cytogenetic level에서 확인을 하면서)
- Drosophila 에서 일어난 gene duplication은 무려 1936년에 이미 확인 되었었다.
- 하지만, 현미경으로도 (육안으로도) 확인이 가능한 variation은 굉장히 드물었고 왠만한 genetic variation은 주로 SNPs, indels, repetitive elements로 이루어졌을거라 생각해서 별로 중요하게 여기지는 않았다.
- 10년 전 쯤 (논문 기준으로 2005년), BAC array-comparative genomic hybridization (array-CGH)와 ROMA 기술의 발전으로 인해 첫 genome SV 분석이 가능해졌다.
- 이를 바탕으로 한 2개의 스터디 (Sebat, et al, Iafrate, et al)에서 intermediate size SV (karyotype에서 확인 불가능)이 상당한 양의 genetic variation을 가지고 있음에도 불구하고 아직 밝혀진 사실이 거의 없다라고 보여줌.
- 또한, 증상적으로 (phenotypically) 건강한 사람들에게서도 common한 large CNV들도 많고, 이런 변이들도 gene dosage, 즉 유전병에도 영향을 줄 수 있다는 것을 밝히게 되면서, genetic analysis의 새로운 챕터를 열게 되었음.
- Rare SV와 연관되어 있는 질병은 꽤 많다: IRGM gene-Crohn’s disease / LCE gene-psoriasis / SNCA gene - Parkinson
- 그렇다고, 모든 SV가 damaging한 phenotype과 관련이 있는 것은 아니고, 아주 일부만 질병과 연관되어 있다. 그런 면에서 SV가 아직 밝혀지지 않은 질병의 원인을 100% 찾는데는 힘들 것. (SV-disease association에 대한 추가 논문)
- 최근 몇년 동안 SV와 breakpoint에 대한 지식은 NGS 기술과 알고리즘의 발전으로 인해 더 풍부해졌다.
- SV 정보를 축적하기 위한 여러 프로젝트들이 진행이 되고 있다:
- Database of Genomic Variants (DGV)
- InvFESt project - predictions on human polymorphic inversions
3. Types of SV
- 이 논문에서 다룰 SV의 주요 종류들은 다음과 같다:
- Translocation: DNA segment가 intra-inter chromosomally 이동. Genetic material의 gain/loss 없음
- Inversion: DNA segment의 orientation이 reverse된 경우 (centromere가 껴있으면 pericentric, else: paracentric)
- Insertion: 새로운 서열의 생성
- CNV: DNA segment가 variable number 만큼 복사 되었을 때
- Deletion: loss of genetic material
- Duplication: tandem/interspersed duplication
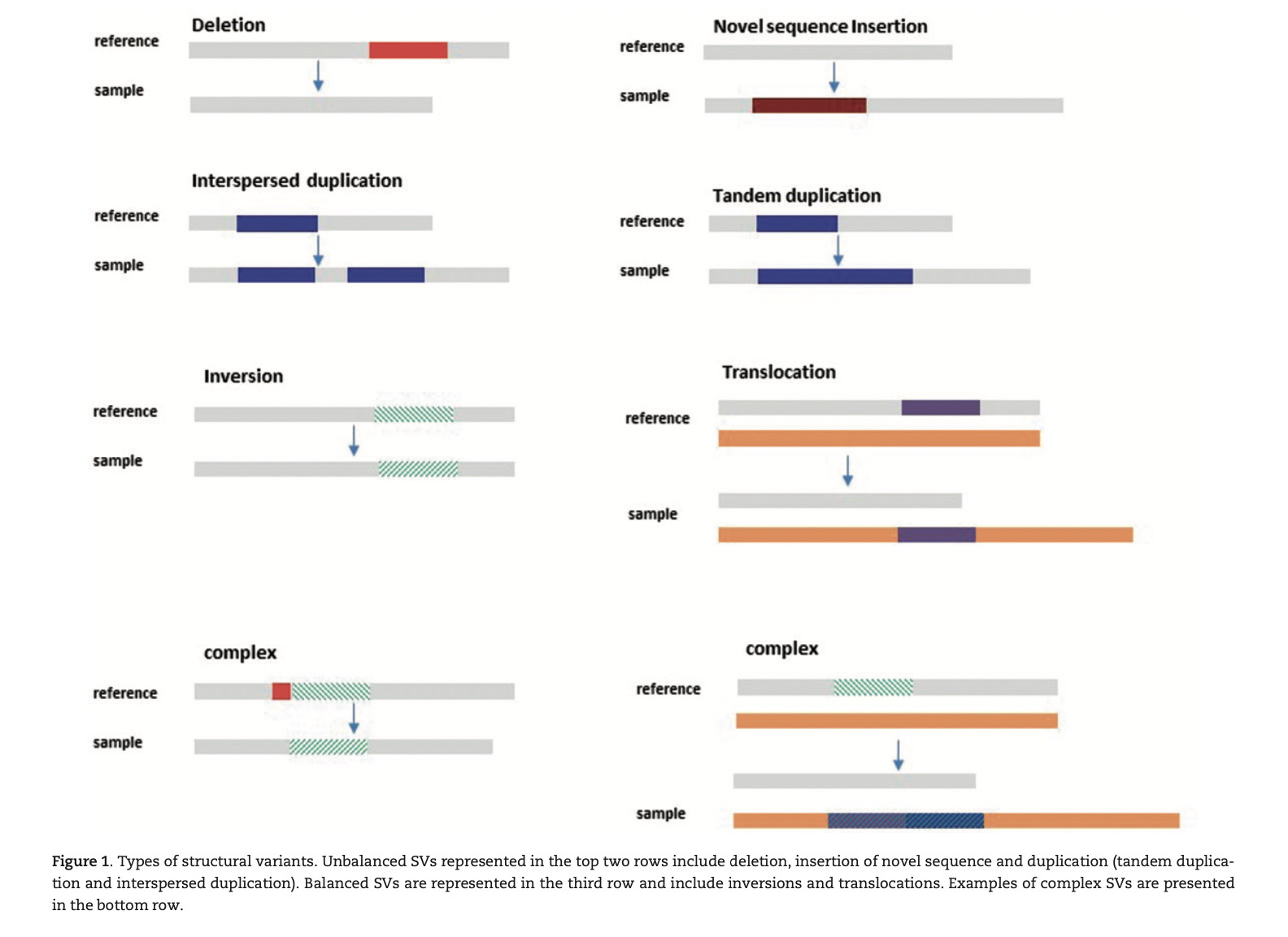
4. Generation of structural variants
- SV가 일어날 수 있는 변이 메커니즘들이 있다 (meiotically and mitotically)
- Recombination error (e.g. non-allelic homologous recombination; NAHR); DNA break repair (e.g. non-homologous end joining; NHEJ, microhomology-mediated break-induced replication; MMBIR); Errors in replication (fork stalling and template switching; FoSTeS) 등등..
- (자세한건 분자세포생물학 책 정독 ㄱㄱ)
5. Detection of SV
- SV 종류들 중에서 가장 지식이 많은 분야는 Copy Number와 관련되어 있는 변이들이다. (이유: 지금의 기술로 잘 찾아짐)
- 2004년에 array-CGH로 인해 SV 분석의 분야를 활성화 시켰다. (array-CGH는 CNV 탐색에 좋은 기법이다)
- Array-CGH: Analysis of intensity ratios of the hybridization of two differentially dyed DNAs against the same target oligonucleotides.
단점: Detects imbalances between two individual genomes, and thus cannot provide an absolute copy number. Also blind to balanced SV events.
- 몇 년 뒤에, SNP-array 데이터를 이용한 CNV 추출하는 알고리즘들이 생겼다
이 알고리즘들은 (PennCNV, QuantiSNP, Birdsuite etc) SNP intensity 정보를 이용하여 시도를 했으나, array-CGH 보다 CNV call을 못함
- 장점: array-CGH에 비해 balanced SV의 존재를 유추할 수 있음
- 더 최근에는 이제 NGS의 발전으로 인해 genomic SV의 발견이 좀 더 섬세해질 수 있었다.
- NGS 초기에는 single read로 진행이 되었지만, 기술의 발전으로 paired-end reads가 더 흔해졌다.
- Paired-end reads는 reference sequence에 더 align이 잘되고, SV 탐지에 추가적인 정보를 제공해준다
- 이로 인해 small CNV 탐지도 가능해졌고, breakpoints들의 다양한 characterization이 가능해졌다.
- 하지만, 최고의 성능을 위한 최적의 알고리즘을 아직도 찾는 과정이다.
- 주로 이 4개의 전략에 기반을 둔다:
- Read Depth (RD): Read Depth analysis는 주로 CNV를 발견한다. RD는 주어진 인터벌에서의 read density을 바탕으로 검색. 비록 RD 분석이 유일하게 copy-number를 정확하게 예측할 수 있는 sequencing-based 방법이지만, breakpoint 해상도가 낮고, sequence coverage에 많이 의존한다. 또한, PCR-induced coverage bias와 balanced SV와 같은 inversion, translocation을 찾지 못한다. (예시 알고리즘: CNVnator)
- Paired read (PR): involves the identification of clusters of aberrantly mapped read pairs, suggesting the presence of SV breakpoints between the reads. 이 mapped paired-reads의 정렬이 다를 경우 다양한 시나리오에 대해서 다양한 종류의 SV를 유추해낼 수 있다. (아래의 Figure의 B.) 하지만, 단점들은: breakpoint의 resolution은 library의 insert size mean, std, coverage에 영향을 받을 것이고, library의 insert size에 따라 insertion을 못 잡을 수도 있다. (예시 툴: Breakdancer)
- Split Reads (SR) + Clip Reads (CR): Aimed at direct identification of sequence reads that span the breakpoints of SV. Provides single nucleotide-level resolution. SR 방법은 NGD 데이터에서는 short read를 align하는데의 어려움으로 인한 한계가 있다. Gap이 클수록 align을 하기가 더 어려움. (예시 툴: Socrates)
- De novo sequence assembly (AS): enables the fine-scale discovery of SVs, including novel (non-reference) sequence insertions, as they do not rely on a reference sequence. Computational cost가 높고 시간 소모와 assembly 에러가 자주 일어날 수 있지만, targeted sequencing assembly를 하는데 굉장히 가치가 높다. (예시 툴: TIGRA)
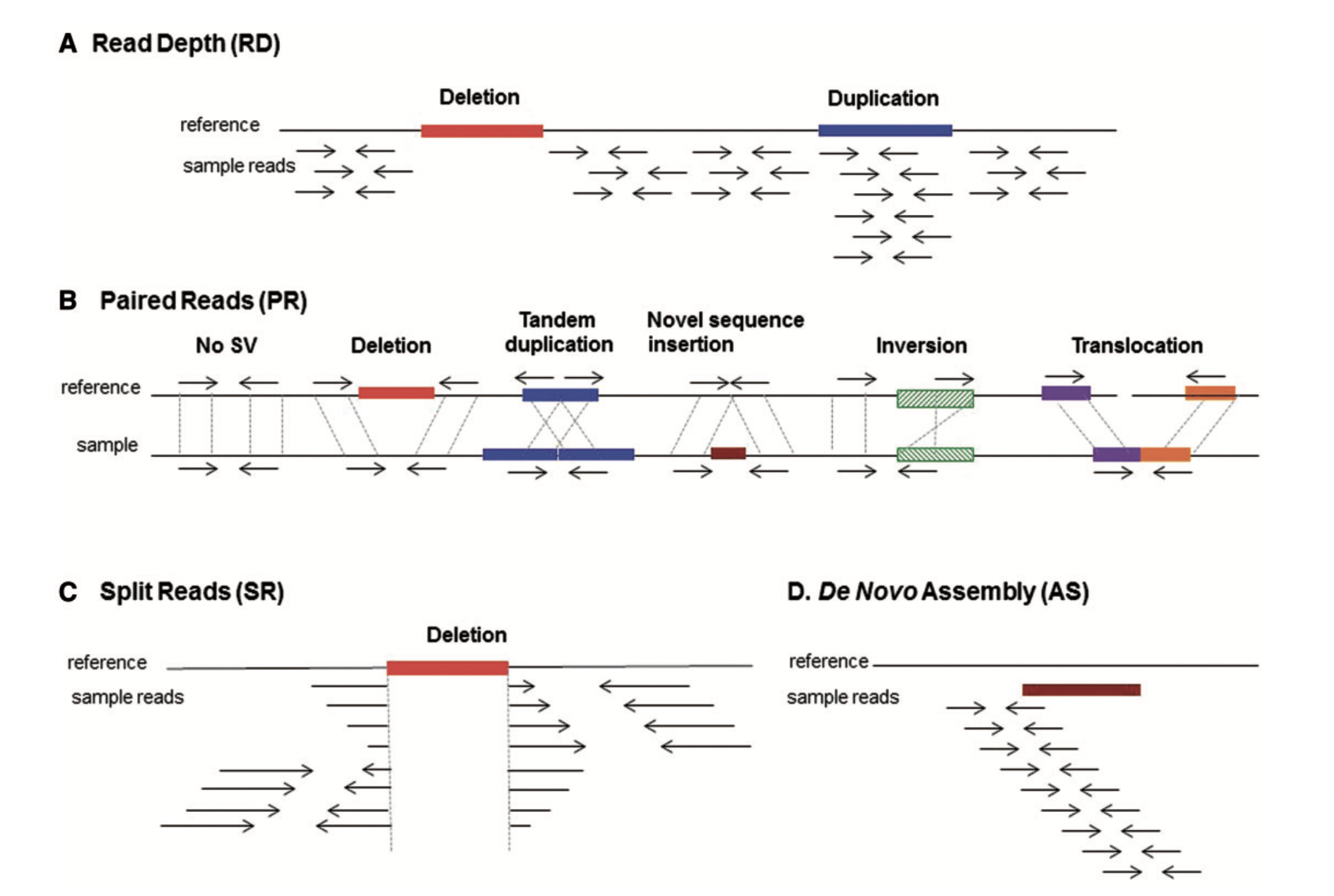
- 위에서 언급한 다양한 방법들은 고유한 장단점이 있지만, 그 어느 방법은 SV의 full spectrum을 잡지 못한다.
- 그래서 조합으로 사용하는 경우가 많아짐:
- RD와 PR을 합쳐 CNV를 더 잘 잡는 툴들: inGAP-sv, CNVer, VariationHunter etc.
- PR + SR : Pindel, PRISM, Delly
- 그러나, 조합으로 만든 3개의 알고리즘으로 잡은 SV들은 크게 겹치지가 않고, 1000 Genome Project에서 검토를 한 결과, 각 방법론은 sensitivity와 specificity가 매우 다양하게 측정되었음. 그나마 GenomeSTRIP이라는 툴이 나았다 (low false discovery rate 때문에). False discovery rate를 더 줄이기 위해 forestSV라는걸 만들었고 (RD + PR 조합).
6. Conclusion & Key points
- Structure variation is a common form of inter-individual genetic variation
- Balanced SV poses bigger challenges and has been less studied than unbalanced forms
- Structural variants are mostly discovered through array-CGH, intensity analysis from SNP arrays and read-pair and RD analysis of high-throughput sequencing data
- SV can be generated by errors in recombination, replication or by erroneous break repair mechanisms, arising both mitotically and meiotically.
- SV의 간단한 소개, 왜 일어나는지, 그리고 이를 탐지하기 위한 역사를 알기에 간단하게 정리된 리뷰 논문이였다