import math
import numpy as np
import pandas as pd
import random
import re
import torch
import urllib.request
from torch.utils.data import DataLoader, Dataset
from transformers import PreTrainedTokenizerFast
import urllib.request
Chatbot_Data = pd.read_csv("./data/BorrowChatData.csv")
# Test 용으로 300개 데이터만 처리한다.
Chatbot_Data = Chatbot_Data[:300]
Chatbot_Data.head()
Q_TKN = "<usr>"
A_TKN = "<sys>"
BOS = "</s>"
EOS = "</s>"
SENT = '<unused1>'
PAD = "<pad>"
MASK = "<unused0>"
# 허깅페이스 transformers 에 등록된 사전 학습된 koGTP2 토크나이저를 가져온다.
koGPT2_TOKENIZER = PreTrainedTokenizerFast.from_pretrained("skt/kogpt2-base-v2", bos_token=BOS, eos_token=EOS, unk_token="<unk>", pad_token=PAD, mask_token=MASK,)
class ChatbotDataset(Dataset):
def __init__(self, chats, max_len=40): # 데이터셋의 전처리를 해주는 부분
self._data = chats
self.max_len = max_len
self.q_token = Q_TKN
self.a_token = A_TKN
self.sent_token = SENT
self.eos = EOS
self.mask = MASK
self.tokenizer = koGPT2_TOKENIZER
def __len__(self): # chatbotdata 의 길이를 리턴
return len(self._data)
def __getitem__(self, idx): # 챗봇 데이터를 차례차례 DataLoader로 넘겨주는 메서드
turn = self._data.iloc[idx]
q = turn["Q"] # 질문을 가져온다.
q = re.sub(r"([?.!,])", r" ", q) # 구둣점들을 제거한다.
a = turn["A"] # 답변을 가져온다.
a = re.sub(r"([?.!,])", r" ", a) # 구둣점들을 제거한다.
q_toked = self.tokenizer.tokenize(self.q_token + q + self.sent_token)
q_len = len(q_toked)
a_toked = self.tokenizer.tokenize(self.a_token + a + self.eos)
a_len = len(a_toked)
#질문의 길이가 최대길이보다 크면
if q_len > self.max_len:
a_len = self.max_len - q_len #답변의 길이를 최대길이 - 질문길이
if a_len <= 0: #질문의 길이가 너무 길어 질문만으로 최대 길이를 초과 한다면
q_toked = q_toked[-(int(self.max_len / 2)) :] #질문길이를 최대길이의 반으로
q_len = len(q_toked)
a_len = self.max_len - q_len #답변의 길이를 최대길이 - 질문길이
a_toked = a_toked[:a_len]
a_len = len(a_toked)
#질문의 길이 + 답변의 길이가 최대길이보다 크면
if q_len + a_len > self.max_len:
a_len = self.max_len - q_len #답변의 길이를 최대길이 - 질문길이
if a_len <= 0: #질문의 길이가 너무 길어 질문만으로 최대 길이를 초과 한다면
q_toked = q_toked[-(int(self.max_len / 2)) :] #질문길이를 최대길이의 반으로
q_len = len(q_toked)
a_len = self.max_len - q_len #답변의 길이를 최대길이 - 질문길이
a_toked = a_toked[:a_len]
a_len = len(a_toked)
# 답변 labels = [mask, mask, ...., mask, ..., <bos>,..답변.. <eos>, <pad>....]
labels = [self.mask,] * q_len + a_toked[1:]
# mask = 질문길이 0 + 답변길이 1 + 나머지 0
mask = [0] * q_len + [1] * a_len + [0] * (self.max_len - q_len - a_len)
# 답변 labels을 index 로 만든다.
labels_ids = self.tokenizer.convert_tokens_to_ids(labels)
# 최대길이만큼 PADDING
while len(labels_ids) < self.max_len:
labels_ids += [self.tokenizer.pad_token_id]
# 질문 + 답변을 index 로 만든다.
token_ids = self.tokenizer.convert_tokens_to_ids(q_toked + a_toked)
# 최대길이만큼 PADDING
while len(token_ids) < self.max_len:
token_ids += [self.tokenizer.pad_token_id]
#질문+답변, 마스크, 답변
return (token_ids, np.array(mask), labels_ids)
def collate_batch(batch):
data = [item[0] for item in batch]
mask = [item[1] for item in batch]
label = [item[2] for item in batch]
return torch.LongTensor(data), torch.LongTensor(mask), torch.LongTensor(label)
train_set = ChatbotDataset(Chatbot_Data, max_len=40)
#윈도우 환경에서 num_workers 는 무조건 0으로 지정, 리눅스에서는 2
train_dataloader = DataLoader(train_set, batch_size=32, num_workers=0, shuffle=True, collate_fn=collate_batch,)
# 결과 확인
print("start")
for batch_idx, samples in enumerate(train_dataloader):
token_ids, mask, label = samples
print("token_ids ====> ", token_ids)
print("mask =====> ", mask)
print("label =====> ", label)
print("end")
import torch
from torch.utils.data import DataLoader
from transformers import PreTrainedTokenizerFast, GPT2LMHeadModel
from ChatbotDataset import ChatbotDataset, Chatbot_Data, collate_batch
Q_TKN = "<usr>"
A_TKN = "<sys>"
BOS = '</s>'
EOS = '</s>'
MASK = '<unused0>'
SENT = '<unused1>'
PAD = '<pad>'
koGPT2_TOKENIZER = PreTrainedTokenizerFast.from_pretrained("skt/kogpt2-base-v2",
bos_token=BOS, eos_token=EOS, unk_token='<unk>',
pad_token=PAD, mask_token=MASK)
model = GPT2LMHeadModel.from_pretrained('skt/kogpt2-base-v2')
device = torch.device("cuda" if torch.cuda.is_available() else "cpu")
train_set = ChatbotDataset(Chatbot_Data, max_len=40)
#윈도우 환경에서 num_workers 는 무조건 0으로 지정, 리눅스에서는 2
train_dataloader = DataLoader(train_set, batch_size=32, num_workers=0, shuffle=True, collate_fn=collate_batch,)
model.to(device)
model.train()
learning_rate = 3e-5
criterion = torch.nn.CrossEntropyLoss(reduction="none")
optimizer = torch.optim.Adam(model.parameters(), lr=learning_rate)
epoch = 10
Sneg = -1e18
print ("start")
for epoch in range(epoch):
for batch_idx, samples in enumerate(train_dataloader):
optimizer.zero_grad()
token_ids, mask, label = samples
token_ids = token_ids.to('cuda')
mask = mask.to('cuda')
label = label.to('cuda')
out = model(token_ids)
out = out.logits #Returns a new tensor with the logit of the elements of input
mask_3d = mask.unsqueeze(dim=2).repeat_interleave(repeats=out.shape[2], dim=2)
mask_out = torch.where(mask_3d == 1, out, Sneg * torch.ones_like(out))
loss = criterion(mask_out.transpose(2, 1), label)
# 평균 loss 만들기 avg_loss[0] / avg_loss[1] <- loss 정규화
avg_loss = loss.sum() / mask.sum()
avg_loss.backward()
# 학습 끝
optimizer.step()
print ("end")
with torch.no_grad():
while 1:
q = input("user > ").strip()
if q == "quit":
break
a = ""
while 1:
input_ids = torch.LongTensor(koGPT2_TOKENIZER.encode(Q_TKN + q + SENT + A_TKN + a)).unsqueeze(dim=0)
model = model.to('cpu')
pred = model(input_ids)
pred = pred.logits
gen = koGPT2_TOKENIZER.convert_ids_to_tokens(torch.argmax(pred, dim=-1).squeeze().numpy().tolist())[-1]
if gen == EOS:
break
a += gen.replace("▁", " ")
print("Chatbot > {}".format(a.strip()))
가장 많이 사용되는 송영숙님의 깃허브에 있는 챗봇 데이터셋을 참고해서 프로젝트 시나리오를 추가
메타데이터는 사용자 입력, 원하는 대답, 내용 분류된다
서비스 시나리오를 구상해서 약 100개의 대화 유형을 데이터셋으로 만들어 학습
결과
고정된 답변만 가능하고 그마저도 틀리는 경우가 발생
서비스 이외의 대답은 불가
대안 :
송영숙님의 데이터셋을 추가해서 대화 가능한 범위 확대
원인 :
기존 데이터셋에 내용 분류에 해당하는 값들을 넣어주지 않아서 생긴 문제
대안 :
내용 분류에 해당하는 값으로 서비스 관련(3)을 추가했다.
ChatGPT3 출시로 기존에 있던 KoGPT2를 파인튜닝하는 방식보다 더 쉽게 좋은 성능을 낼 수 있어서 ChatGPT3를 사용한 서비스용 챗봇으로 변경
터미널에서 아래 명령어 입력으로 설치
pip install openai
import pandas as pd
import openai
openai.organization = "Organization 키를 입력"
openai.api_key = "발급받은 API 키를 입력."
코드 설명
def get_openai_response(prompt, print_output=False):
completions = openai.Completion.create(
engine='text-davinci-003', # Determines the quality, speed, and cost.
temperature=0.5, # Level of creativity in the response
prompt=prompt, # What the user typed in
max_tokens=3072, # Maximum tokens in the prompt AND response
n=1, # The number of completions to generate
stop=None, # An optional setting to control response generation
)
# Displaying the output can be helpful if things go wrong
if print_output:
print(completions)
# Return the first choice's text
return completions.choices[0].text
|코드|기능|
|---|---|
|get_openai_response|ChatGPT API의 응답을 반환하는 함수|
|prompt를 request로 요청| completions에 JSON 형태의 response가 API를 통해 반환|
|return completions.choices[0].text|completions에서 사용자가 원하는 GPT 언어모델의 응답을 추출해서 반환|
> **코드 설명**
def split_script_1000(script):
split_script = script.split('. ')
result = []
counter = 0
tmp = ''
for i in split_script:
tmp += i
counter += len(i)
if counter > 1000:
result.append(tmp)
tmp = ''
counter = 0
return result
|코드|기능|
|---|---|
|split_script_1000 함수|입력된 str 형태의 스크립트를 ". " 기준으로 분할해서 result에는 ". "로 분할된 스크립트의 리스트가 반환|
> 그냥 "."이 아닌 ". "로 분할하는 이유는 실수(1.1, 2.2, 3.3... etc)는 포함시키지 않기 위해
---
### 3.2.3 Main
df = pd.read_csv('script.csv')
prompt = 'Rewrite this script professionally and add subheadings No Titles No Introduction No Conclusion.'
result_list = []
for ind, row in df.iterrows():
eng_result = ''
title = row['TITLE']
script = row['SCRIPT'].strip('\n')
split_script = split_script_1000(script)
for ss in split_script:
request = prompt + ss
response = get_openai_response(request)
eng_result += response
result_list.append({'title': title, 'eng': eng_result})
df_result = pd.DataFrame(result_list)
df_result.to_csv('rewrited_script.csv', mode='a', index=False, encoding='utf-8-sig')
- prompt는 "각각의 문단에 소제목을 달고 제목, 개요 및 결론을 내지 말고 이 글을 다시 써주세요."라는 요청에 1000자 단위로 분할된 스크립트를 추가해서 API를 호출한다.
- script.csv의 형태는 다음과 같다.
- 엑셀로 열어보면 TITLE 칼럼과 SCRIPT칼럼을 가진 일반적인 데이터 프레임이다.
- TITLE에는 문서의 이름 SCRIPT에는 문서 내용을 써넣으면 된다.
결과는 rewrited_script.csv에 저장된다.
> prompt를 수정하여 API 결과에 따른 다양한 서비스를 만들 수 있다.
---
> text-davinci-003는 1000토큰(1k)당 원화 26원
이 모델 사용할 때 많이 나온 이유는 프롬프트에 제시한 자료 자체를 토큰으로 인식하는데
대화내용이 축적되지 않아서 비용이 과도하게 발생해서 이 문제를 해결하기 위해 찾아보다가 gpt-3.5-turbo가 10%의 비용으로 더 쉽게 대화내용을 축적할 수 있기 때문에 엔진을 교체했다
---
# ?. Errors
## ?.1 RuntimeError: Expected all tensors to be on the same device, but found at least two devices
> 원인
model과 나머지 텐서들이 연산되는 과정에서 서로 다른 디바이스를 사용해서 발생한 에러
> 해결
CPU와 GPU는 메모리 공간이 분리된 다른 기기다. 텐서끼리 연산할 때 같은 기기가 아니라서 발생
- 먼저 각각의 텐서에서 사용중인 디바이스를 확인하고
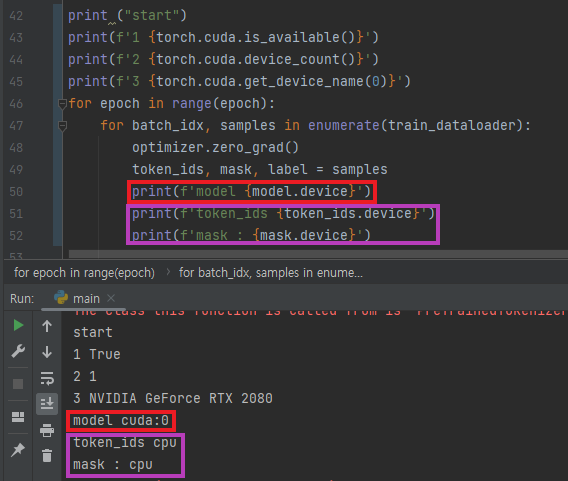
- 사용할 디바이스를 일치시켜주면 해결된다
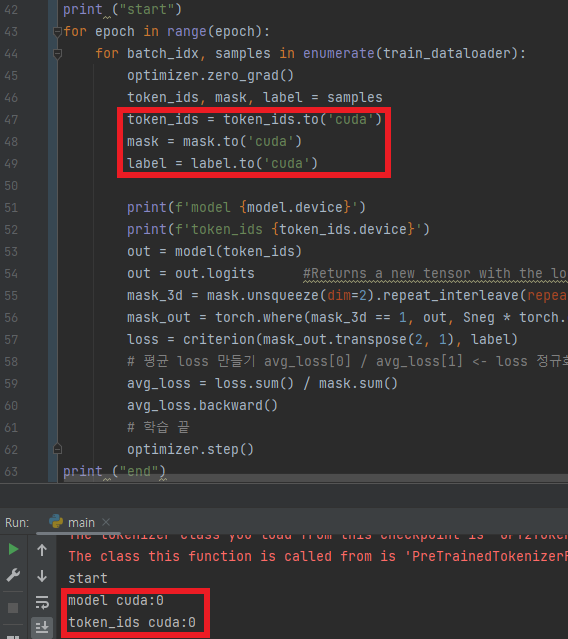
### ?.1.1 디바이스 문제 해결방법
> #### 디바이스 확인
##### - GPU 사용가능 여부
```print(f'1 {torch.cuda.is_available()}')```
##### - 디바이스 수
```print(f'2 {torch.cuda.device_count()}')```
##### - 사용중인 디바이스 이름 확인
```print(f'3 {torch.cuda.get_device_name(0)}')```
##### - cpu와 gpu 중 해당 텐서의 위치
```텐서.device```
##### - cpu에서 gpu로 전환
```텐서.to('cuda')```
>#### 데이터가 텐서로 변환되지 않은 경우
##### - 텐서변환 확인
y = torch.as_tensor(x, dtype=torch.half, device='cpu')
device = torch.device("cuda")
x = torch.tensor([[1, 2, 3], [4, 5, 6]], dtype=torch.int32, device=device)
>#### 연산하는 텐서 간의 데이터 타입이 다른 경우
##### - 타입 확인
print(x.dtype)
## ?.2 TypeError: can't convert cuda:0 device type tensor to numpy. Use Tensor.cpu() to copy the tensor to host memory first.
> 원인
GPU에 할당된 텐서를 넘파이 배열로 변환하려 해서 발생
> 해결
이전 에러 해결을 위해 CUDA로 사용중이던 텐서의 디바이스를 넘파이 배열 사용 전에 cpu로 바꾼다.
## ?.3 ValueError: Connection error, and we cannot find the requested files in the cached path. Please try again or make sure your Internet connection is on.
> 해결
재실행하면 된다
---
출처
- [PyTorch 딥러닝 챗봇](https://wikidocs.net/book/7439)
- [ChatGPT API 사용법](https://eppiroi.tistory.com/61)