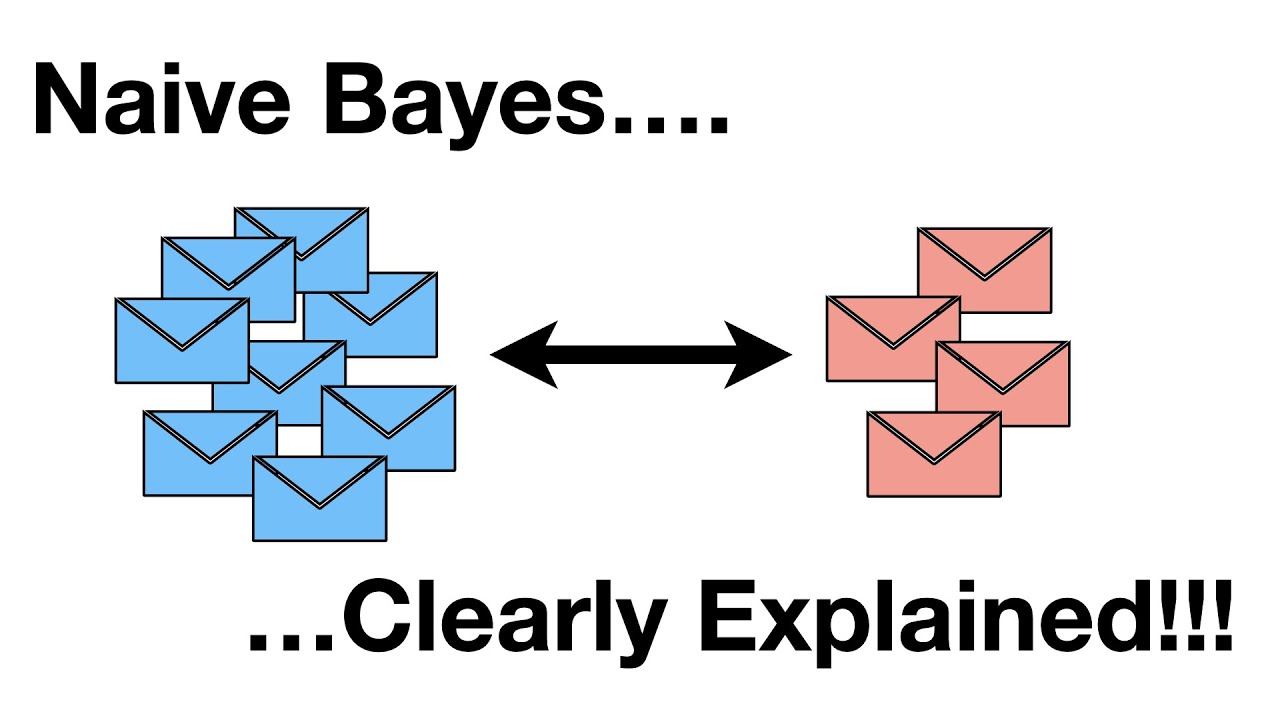
나이브 베이즈 분류 : 특성들 사이의 독립을 가정하는 베이즈 정리를 적용한 확률 분류기의 일종
백오브워즈 가정 : 저자의 의도가 단어 사용 여부나 그 빈도에서 드러난다고 보는 가정.
-
TF-IDF(Term Frequency-Inverse Documnet Frequency) : 어떤 단어의 주제 예측 능력이 강할 수록 가중치가 커지고, 그 반대의 경우 작아짐.
주제예측능력을 측정할 때 term자체는 자주 등장하지만 document전체제 자주 등장하지 않을 때 주제예측능력이 증가한다.
TF−IDF(w)=TF(w)×logDF(w)N
-
Deep Averaging Network( lyyer et al. 2015) : 문장에 속한 단어의 임베딩을 평균을 취해 문장의 임베딩을 만듬2
베이즈 이론 :
P(A∣B)=P(B)P(B∣A)P(A)
P(A|B) : posterior, P(B|A) : likelihood, P(A) : prior, p(B) : merginal
C 클래스에서 각가의 d 개의 document에 에 대하여 document가 c에 속할 확률은 P(c|d)로 나타낼 수 있다. 이에 대한 식은
P(c∣d)=P(d)P(d∣c)P(c)
분모를 dropping시킨 후 chain rule 까지 적용
P(d∣c)P(c)=P(w1,w2,...,wn∣c)P(c)=P(wn∣wn−1,...,w1)×P(wn−1∣wn−2,...,w1)P(c)×...×P(w1∣c)P(c)
P(d∣c)P(c)=P(c)wi∈W∏P(wi∣c)
예시) 스팸메일인지 아닌지 분류하는 문장 분류
1.(Training) | me free lottery | Spam |
---|
2.(Training) | free get free you | Spam |
3.(Training) | you free scholarship | Inbox |
4.(Training) | free to contact me | Inbox |
5.(Training) | you won award | Inbox |
6.(Training) | you ticket loterry | Spam |
7(test) | you free lottery | ? |
P(cspam∣d)=P(cspam)P(wyou∣cspam)P(wfree∣cspam)P(wlottery∣cspam)=21×102×103×102=10006
1.(Training) | me free lottery | Spam |
---|
2.(Training) | free get free you | Spam |
3.(Training) | you free scholarship | Inbox |
4.(Training) | free to contact me | Inbox |
5.(Training) | you won award | Inbox |
6.(Training) | you ticket loterry | Spam |
7(test) | you free lottery | ? |
P(cInbox∣d)=P(cInbox)P(wyou∣cInbox)P(wfree∣cInbox)P(wlottery∣cInbox)=21×102×102×100=0
P(cspam∣d)>P(cInbox∣d)
그러므로 스펨메일에 속한다고 분류한다.