Conditinal Generative Adversarial Nets
Abstract
- y data를 추가하여 만든 conditional GAN
- CGAN을 이용하면 class label(ex.MNIST 숫자 0-9) 에 맞는 이미지 생성 가능
experiments
MNIST image 생성 ... (4.1)
image tagging ... (4.2)
1. Introduction
- GAN : 생성되는 데이터의 종류를 control 할 수 없음
- CGAN : class label에 기반한 추가 정보를 통해 데이터 생성 과정을 제어할 수 있음
2.1 Multi-modal Learning For Image Labelling
- 많은 카테고리를 다뤄야 하는 모델에 관한 문제
- input-output mapping(one to many mapping) : conditional GAN이 하나의 solution이 될 수 있음
ex) 하나의 이미지에 대해서 여러개의 tagging이 가능한 경우
3. Conditinal Adversarial Nets
3.1 Generative Adversarial Nets
- G : 데이터 생성 모델
- D : 데이터 판별 모델
minGmaxDV(D,G)=Ex pdata(x)[logD(x)]+Ez p(z)[log(1−D(G(z)))]
3.2 Conditional Adversarial Nets
y (extra information ex.class label/data from other modalities)을 G , D 의 input layer에 추가로 넣음으로써 conditional model로 확장 가능
minGmaxDV(D,G)=Ex pdata(x)[logD(x∣y)]+Ez p(z)[log(1−D(G(z∣y)))]
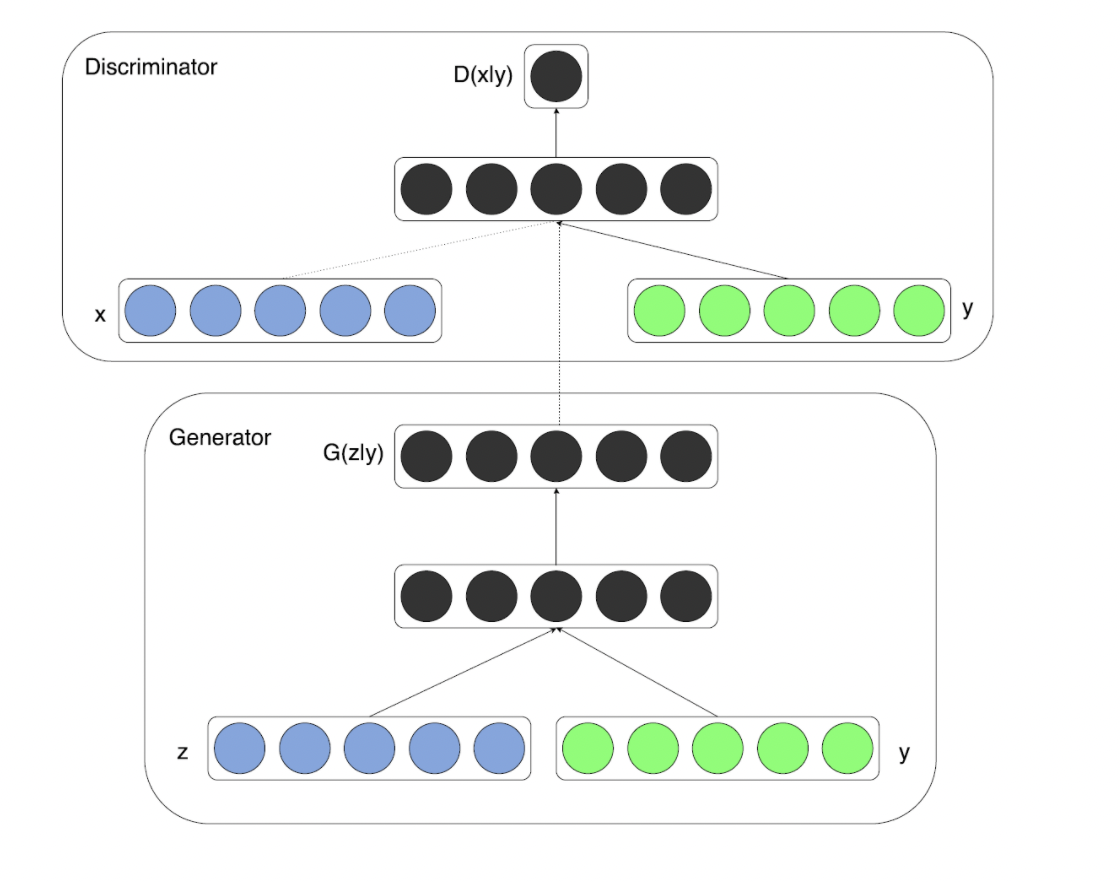
4.Experimental Results
4.1 Unimodal
MNIST data set
Generator
- multilayer perceptron 구조
- input : 100 + 10 dim
z ~ uniform distribution , 100 dim
class label에 따른 이미지를 생성해야하므로 label정보를 포함 (one-hot incoding : 10 dim)
- Activator : Relu
- output : 784 (MNIST : 28*28)
Discriminator
- multilayer perceptron 구조
- input : 784 + 10 dim(label)
- Activator : Relu
- output : 1 dim (using sigmoid for 1-dim probability)
initial learning rate
0.1 (exponentianlly decreased down to 0.000001)
dropout rate
0.5
stopping rule
best setimate of log-likelihood on the validation set
y에 따라 이미지가 잘 생성된 것을 확인할 수 있음
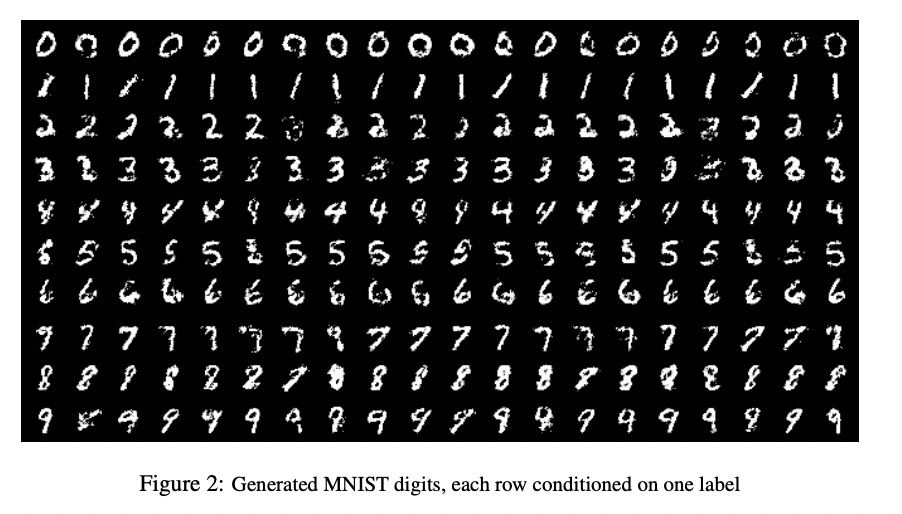
다만, log-likelihood의 값을 보면 CGAN이 GAN의 성능까지는 아직 따라가지 못 하고 있는 것이 확인 됨.
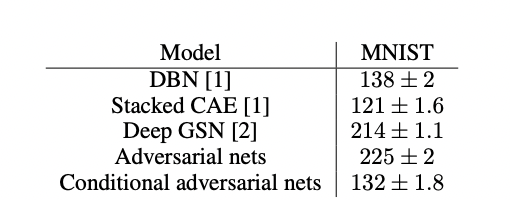
4.2 multimiodal
MIR Flickr data set
generator
input : noise + image feature
ouput : word vectors ( 해당 이미지를 설명한다고 판단되는)
여러 이미지에 대해 사람이 직접 넣은 tag와 CGAN이 생성해 낸 태그
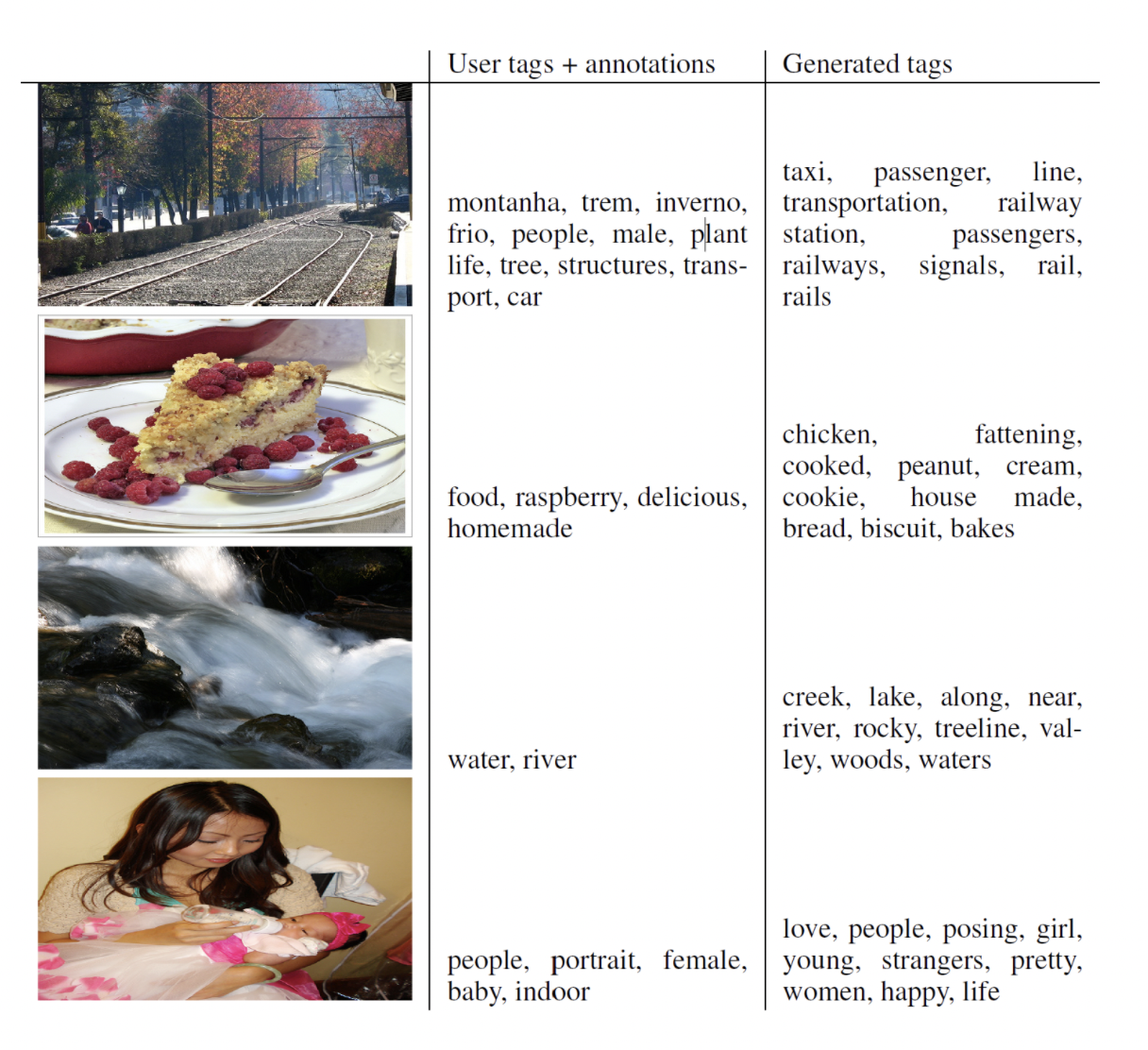
5. Future Work
해당 논문은 서론의 내용을 소개했지만, 조건부 생성모델의 잠재력 및 응용 가능성을 내포함.