DREAMLLM: SYNERGISTIC MULTIMODAL COMPREHENSION AND CREATION (ICLR 2024 Spotlight)
https://arxiv.org/abs/2309.11499
Abstract
- DREAMLLM은 처음으로 다목적 MLLM(Multimodal Large Language Models)을 제안
다중모달의 이해(comprehension)와 생성(creation) 사이의 시너지를 활용함
- 첫번째로, raw multimodal space에서 직접적으로 샘플링함으로써 language와 image의 posterior을 모델링
- 두번째로, DREAMLLM은 모든 conditional, marginal, 그리고 joint multimodal distribution을 효과적으로 학습하기 위해서, 텍스트와 이미지 콘텐츠를 함께 모델링하고, 비구조적 레이아웃을 포함시킴
위 두가지 fundamental principle을 통해
- 모든 conditional, marginal and joint multimodal distribution을 효과적으로 학습
지금까지 MLLMs들은
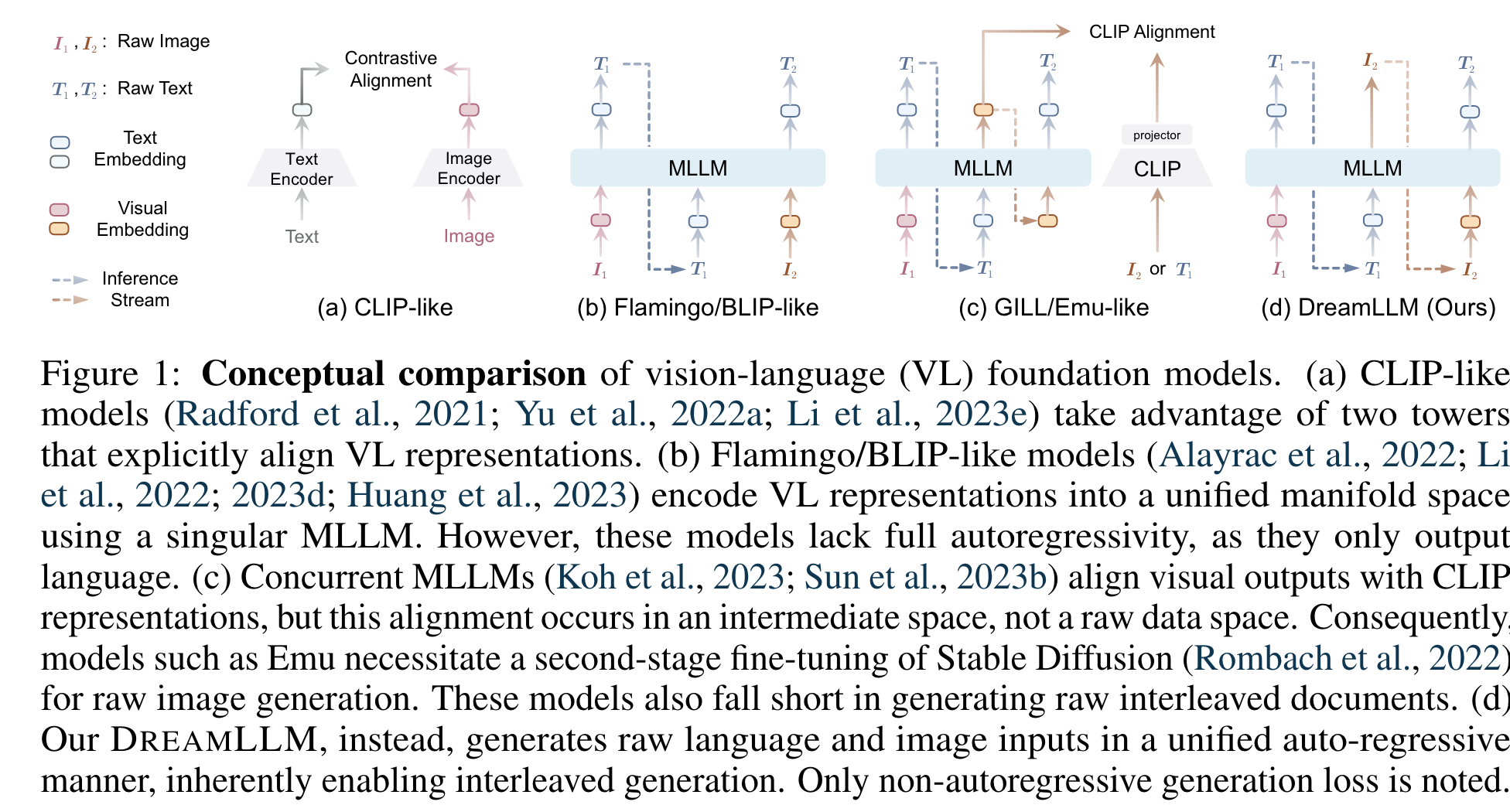
- CLIP encoder와 explicitly align하도록 embedding을 생성
- 그러나 위 방법은 고유 modality gap 으로 인해 이미지 생성에서 제한이 존재함.
- 이러한 modality gap으로 인해, CLIP은 modality-shared 정보에만 초점을 맞춰져 있었고, multimodal comprehension을 향상시킬 수 있는 modality-specific knowledge 는 간과됨.
- universal generative model (simultaneously learns language and image posterios)이 필요함을 강조.
Contribution
- CLIP 임베딩 과는 다르게 DREAMLLM은 모든 모달리티의 raw data를 입력 뿐만 아니라 출력으로도 완전한 end-to-end 방식으로 처리. (입력과 출력이 동일).
- 이 때 MLLM의 이해능력을 저해하지 않고 image posterior를 학습
- 이를 해결하기 위해 dream query 를 도입
- a set of learnable embeddings that encapsulate the semantics encoded by MLLMs.
- This approach avoids altering the output space of MLLMs.
- Raw image는 위 dream query로 condition된 SD image decoder에 의해 생성됨.
- 교차 생성 사전학습 (I-GPT)
- 교차되는 이미지-텍스트 멀티모달 입력을 encoding 그리고 decoding 함으로써, 인터넷으로부터 교차된 멀티모달 corpora를 생성하도록 훈련됨.
- 기존 multimodal input을 encoding하는 방법이랑 다르게, multimodal output을 decoding.
unique \<dream> token을 사용해서 교차된 레이아웃 학습을 해결.
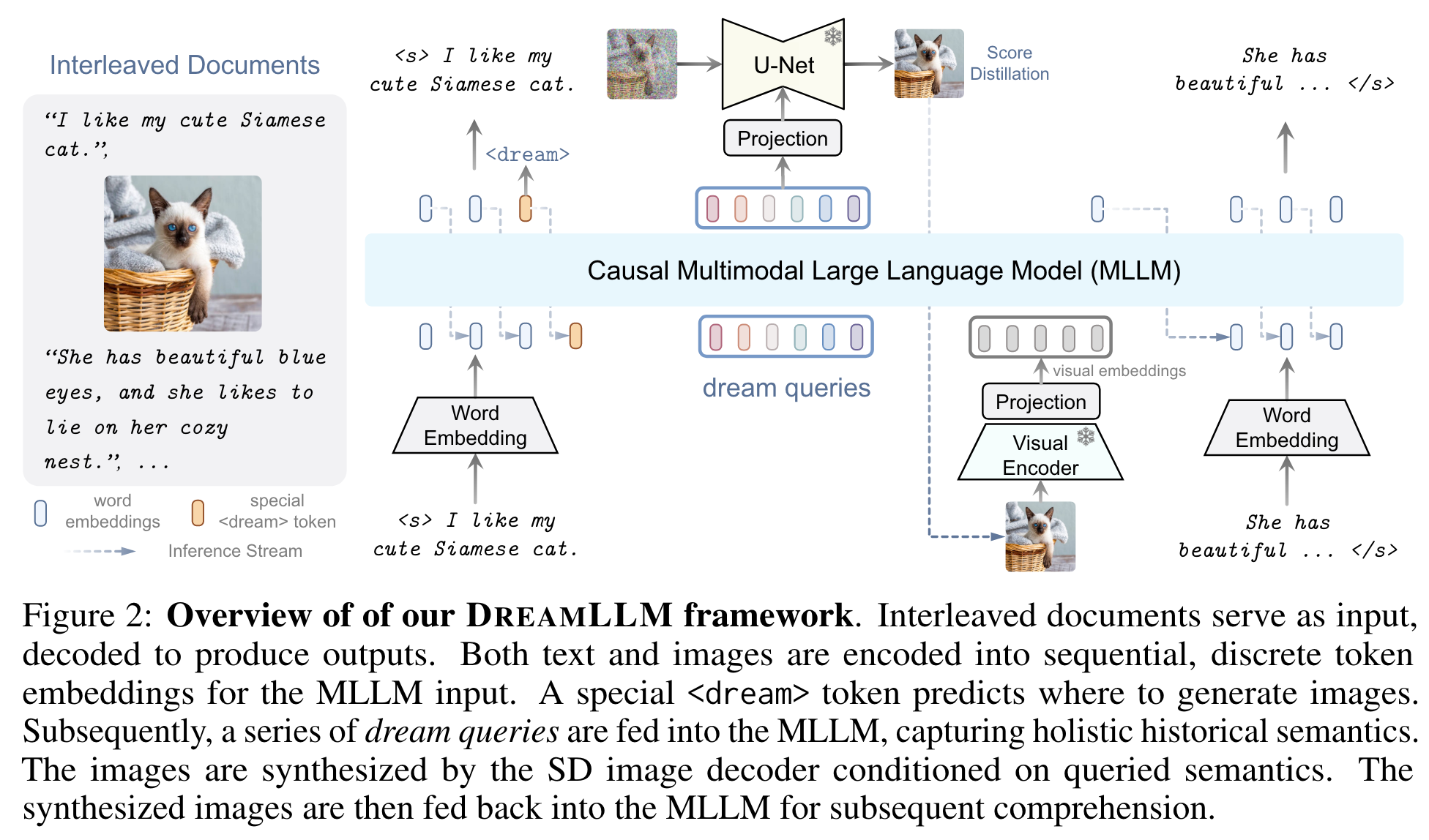