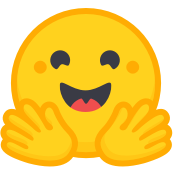
개요
- Task를 정의하고 그에 맞게 dataset을 가공시킵니다
Processors
task를 정의하고 dataset을 가공
**Tokenizer**
텍스트 데이터를 전처리
- 적당한 model을 선택하고 이를 만듭니다.
Model
다양한 모델을 정의
- model에 데이터들을 태워서 학습을 시킴
**Optimizer**
optimizer와 학습 schedule(warm up 등)을 관리
Trainer
학습 과정을 전반 관리
- 3을 통해 나온 weight와 설정(config)들을 저장합니다.
Config
weight와 tokenizer, model을 쉽게 불러올 수 있도록 각종 설정을 저장
- 저장한 model의 checkpoint는 배포하거나, evaluation을 할 때 사용하고는 하죠.
Model
from transformers import TFBertForPreTraining
model = TFBertForPreTraining.from_pretrained('bert-base-cased')
from transformers import TFAutoModel
model = TFAutoModel.from_pretrained("bert-base-cased")
- 기본적으로 모델들은
PretrainedModel
클래스를 상속받고 있음
PretrainedModel
클래스는 학습된 모델을 불러오고, 다운로드하고, 저장하는 등 모델 전반에 걸쳐 적용되는 메소드를 가지고 있음
- 상속 구조를 가지고 있기 때문에, 실제로 사용할 모델이
BERT
이건 GPT
이건 상관없이 모델을 불러오고 다운로드/저장하는 등의 작업에 활용하는 메소드는 부모 클래스의 것을 도일하게 활용
AutoModel
- 모든 클래스에 걸쳐 Auto 클래스가 존재
- 모델에 관한 정보를 처음부터 명시하지 않아도 되어 조금 유용하게 사용 가능
- 모델의 상세정보를 확인할 필요 없이 Model ID (ex:bert-base-cased) 만으로도 손쉽게 모델 구성이 가능
- 정확한 용도에 맞게 사용하려면 모델별 상세 안내 페이지를 참고해서 최적의 모델 선택
Tokenzier
from transformers import BertTokenizer
tokenizer = BertTokenizer.from_pretrained('bert-base-cased')
from transformers import AutoTokenizer
tokenizer = AutoTokenizer.from_pretrained('bert-base-cased')
encoded = tokenizer("This is Test for aiffel")
print(encoded)
-------------------------------------------------------
>>> {'input_ids': [101, 1188, 1110, 5960, 1111, 170, 11093, 1883, 102],
'token_type_ids': [0, 0, 0, 0, 0, 0, 0, 0, 0], 'attention_mask': [1, 1,
1, 1, 1, 1, 1, 1, 1]}
- transformers는 바로 불러와 사용할 수 있도록 다양한 tokenizer를 각 모델에 맞추어 구비
- 내가 선택한 모델이 어떠한 tokenizer를 사용하는지 정도 미리 체크
- Model과 Toeknizer는 Pretrained model 기반 NLP framework를 사용할 때 가장 중요한 두 가지 클래스
- 파라미터가 동일한 Model이라 하더라도 Tokenizer가 다르거나 Tokenizer 내의 Directory가 달라지면 사실상 완전히 다른 모델이 됨
- Tokenizer는 어떤 언어를 다루느냐 하는 코퍼스 데이터셋에 따라서도 달라짐
- 예제
bert-base-uncased
: 108MB param, all lowercase
bert-large-cased
: 340MB param, both upper and lower
bert-base-uncassed
: 108MB param, multi language, both upper and lower
- tokenizer 또한 직접 명시하여 내가 사용할 것을 지정해 주거나, AutoTokenizer를 사용하여 이미 구비된 model에 알맞는 tokenizer를 자동으로 불러올 수도 있음
- model을 사용할 때 명시했던 것과 동일한 ID로 tokenizer를 생성해야함
- tokenize할 때에
padding
, truncation
등 다양한 옵션을 설정할 수 있으며, 모델이 어떤 프레임워크를 사용하는가(Tensorflow 또는 PyTorch)에 따라 input 타입을 변경 시켜주는 return_tensors
인자도 있음
Processor
class DataProcessor:
"""sequence classification을 위해 data를 처리하는 기본 processor"""
def get_example_from_tensor_dict(self, tensor_dict):
"""
tensor dict에서 example을 가져오는 메소드
"""
raise NotImplementedError()
def get_train_examples(self, data_dir):
"""train data에서 InputExample 클래스를 가지고 있는 것들을 모으는 메소드"""
raise NotImplementedError()
def get_dev_examples(self, data_dir):
"""dev data(validation data)에서 InputExample 클래스를 가지고 있는 것들을 모으는 메소드"""
raise NotImplementedError()
def get_test_examples(self, data_dir):
"""test data에서 InputExample 클래스를 가지고 있는 것들을 모으는 메소드"""
raise NotImplementedError()
def get_labels(self):
"""data set에 사용되는 라벨들을 리턴하는 메소드"""
raise NotImplementedError()
def tfds_map(self, example):
"""
tfds(tensorflow-datasets)에서 불러온 데이터를 DataProcessor에 알맞게 가공해주는 메소드
"""
if len(self.get_labels()) > 1:
example.label = self.get_labels()[int(example.label)]
return example
@classmethod
def _read_tsv(cls, input_file, quotechar=None):
"""tab으로 구분된 .tsv파일을 읽어들이는 클래스 메소드"""
with open(input_file, "r", encoding="utf-8-sig") as f:
return list(csv.reader(f, delimiter="\t", quotechar=quotechar))
- 각 Task의 특정 전처리 과정을 포함해 Model에 넣을 수 있는 적절한 입력 형태로 변경
- raw data를 가공하여 model에 태울 수 있는 형태로 만들어주는 작업을 하는 추상 클래스
- SQuAD, GLUE 등 가장 대표적인 NLP의 문제들에 쉽게 접근할 수 있도록 processor를 만들어 둠
- 내가 직접 수집한 데이터를 가공하고 싶다면, 내 데이터에 알맞은 processor를 직접 정의
- Task 별 복잡한 데이터 전처리 작업을 수행하는 processor를 직접 만들때는,
DataProcessor
를 상속받아 만들어줌. 내가 가지고 있는 데이터에 따라 추가해야 하는 부분이 생길 수도 있음.
Config
from transformers import BertConfig
config = BertConfig.from_pretrained("bert-base-cased")
print(config.__class__)
print(config)
from transformers import AutoConfig
config = AutoConfig.from_pretrained("bert-base-cased")
print(config.__class__)
print(config)
model = TFBertForPreTraining.from_pretrained('bert-base-cased')
config = model.config
print(config.__class__)
print(config)
- 모델을 학습시키기 위한 요소들을 명시한
json
파일로 되어 있음
- batch size, learning rate, weigth_decay 등 train에 필요한 요소들부터 tokenizer에 특수 토큰 (special token eg
[mask]
) 들을 미리 설정하는 등 설정에 관한 전반적인 것들 명시
- PretrainedModel을 save_pretrained 메소드를 이용하면 모델의 체크포인트와 함께 저장되도록 되어있음.
- hugging face의 pretrained model을 그대로 사용하게 되면 자동으로 config파일이 로드되어 명시할 필요가 없지만, 설정을 변경하고 싶거나 나만의 모델을 학습시킬 때에는 config파일을 직접 불러와야 함.
- config 또한 model, tokenizer처럼 Model ID만 있으면, Config 클래스를 명확히 지정하거나 혹은
AutoConfig
를 이용하는 방식으로 불러올 수 있음
Trainer
from dataclasses import dataclass, field
from enum import Enum
from typing import Dict, Optional
import tensorflow as tf
import tensorflow_datasets as tfds
from transformers import (
TFAutoModelForSequenceClassification,
TFTrainer,
TFTrainingArguments,
AutoConfig,
AutoTokenizer,
glue_convert_examples_to_features,
)
training_args = TFTrainingArguments(
output_dir='./results',
num_train_epochs=1,
per_device_train_batch_size=16,
per_device_eval_batch_size=64,
warmup_steps=500,
weight_decay=0.01,
logging_dir='./logs',
do_train=True,
do_eval=True,
eval_steps=1000
)
model_name_or_path = 'bert-base-uncased'
with training_args.strategy.scope():
model = TFAutoModelForSequenceClassification.from_pretrained(
model_name_or_path,
from_pt=bool(".bin" in model_name_or_path),
)
tokenizer = AutoTokenizer.from_pretrained(
model_name_or_path,
)
ds, info = tfds.load('glue/mrpc', with_info=True)
train_dataset = glue_convert_examples_to_features(ds['train'], tokenizer, 128, 'mrpc')
train_dataset = train_dataset.apply(tf.data.experimental.assert_cardinality(info.splits['train'].num_examples))
trainer = TFTrainer(
model=model,
args=training_args,
train_dataset=train_dataset,
)
trainer.train()
test_dataset = glue_convert_examples_to_features(ds['test'], tokenizer, 128, 'mrpc')
test_dataset = test_dataset.apply(tf.data.experimental.assert_cardinality(info.splits['test'].num_examples))
trainer.evaluate(test_dataset)
- training, fine-tuning, evaluation 모두 trainer class를 이용하여 할 수 있음
- tensorflow의
tf.keras.model
API를 이용도 가능
TFTrainer
를 이용할 경우에는 TrainingArguments
를 통해 Huggingface 프레임워크에서 제공하는 기능들을 통합적으로 커스터마이징하여 모델을 학습시킬 수 있다는 장점
TFTrainer
를 사용할 경우에는 학습에 필요한 arguments을 TFTrainingArguments
을 통해서 정의해 주어야 함
- 이후 데이터셋을 생성하여, model, training_args과 함께
TFTrainer
에 전달하는 것으로 학습을 위한 준비가 마무리. 이후 trainer.train()
을 호출하면 실제 학습이 진행
GLUE datasets과 Hugginface
GLUE datasets
- SQuAD처럼 기존에 유명한 데이터셋 한 가지만 가지고 성능을 논하는 것이 아니라 다양한 task를 해당 모델 하나만을 이용해 모두 수행해 보면서 종합적인 성능을 논함
- NLP 모델의 성능을 측정하기 위한 데이터셋으로 최근 활용되는 대표적인 것