Vision GNN: An Image is Worth Graph of Nodes
Vision GNN: An Image is Worth Graph of Nodes
Efficient-AI-Backbones/vig_pytorch at master · huawei-noah/Efficient-AI-Backbones
Abstract
널리 사용되는 CNN과 Transformer는 이미지를 grid 또는 sequence 구조로 다루는데, 이는 불규칙하고 복잡한 대상을 잘 파악하지 못한다.
이번 연구에서는 이미지를 graph 구조로 보고, 새로운 Vision GNN (ViG) 구조를 제안한다.
- 이미지를 node처럼 다뤄지는 여러개의 patch로 나눈다.
- 가장 가까운 이웃들을 연결함으로서 graph를 만든다.
Image의 graph 표현에 기반해서, ViG 모델이 모든 node 사이에서 정보를 변환하고 교환하도록 한다.
GNN을 일반적인 visual task에 사용하는 선구적인 연구가 영감을 주길 바란다.
1. Introduction
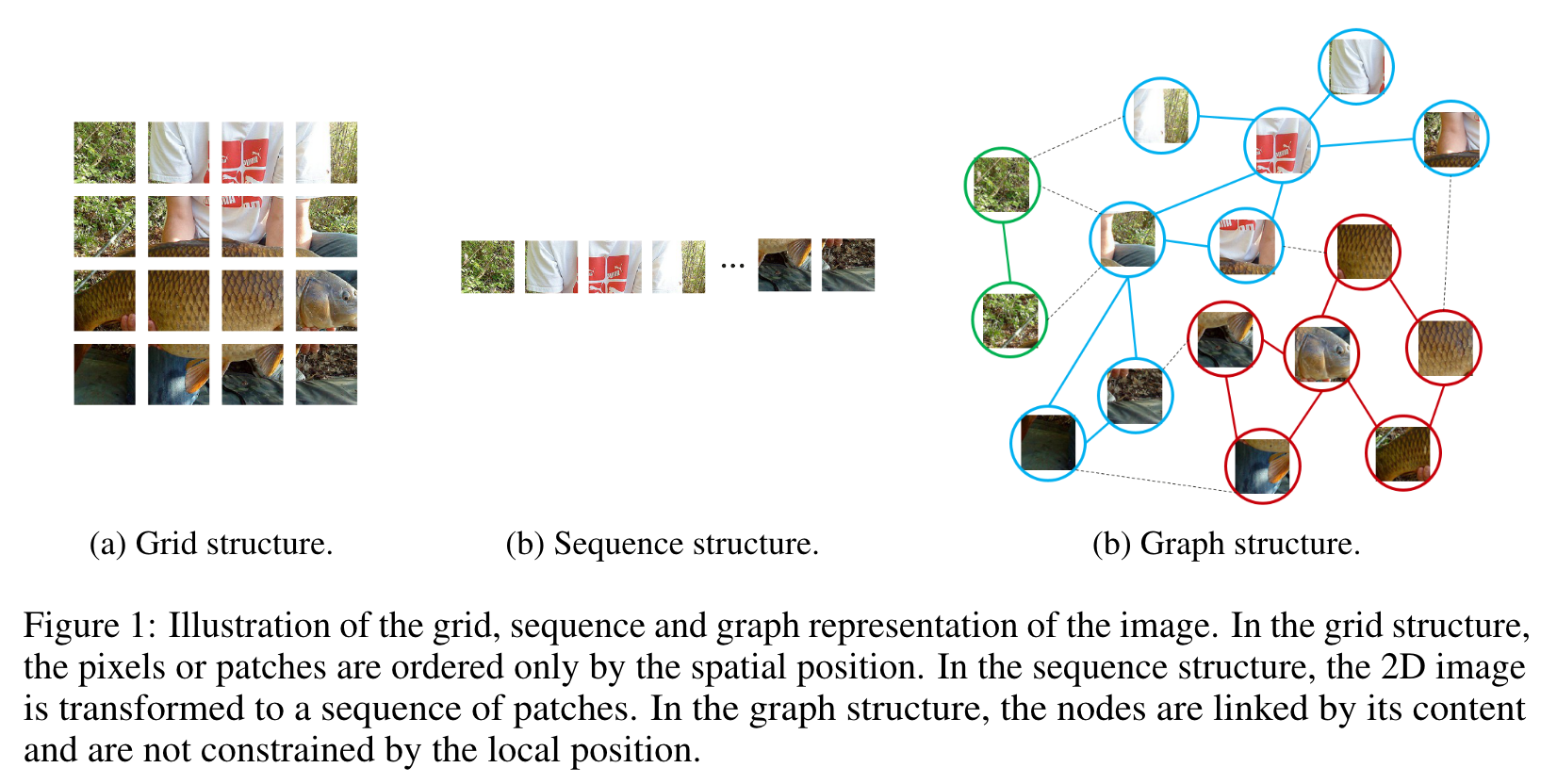
현대 Computer Vision에서 CNNs, Transformer가 가장 많이 사용된다.
이미지는 일반적으로 유클리드 공간에서 표현된다. ViG는 일반적인 grid 또는 sequence 표현 대신, 이미지를 더 유연한 방식으로 다룬다.
Computer Vision 의 기본적인 task는 물체 인식이다. 대부분의 물체는 다양하고 불규칙한 모양이기에 기존의 방식은 낭비가 크고 유연하지 못하다.
물체는 부분의 집합으로 생각될 수 있다. 예를 들자면, 인간이 머리, 상체, 하체로 나눠지고, 이들의 관계가 자연스럽게 graph 구조로 표현될 수 있고, 그래프를 분석함으로 우리는 인간을 인식한다.
이에 더해, graph는 grid, sequence의 일반화된 구조이다. 그렇기에 이미지를 graph로 보는 것은 더 유연하고 효율적인 인식이다.
Image의 Graph representation을 바탕으로 ViG를 만든다.
각각의 pixel을 node로 만드는 건 너무 많은 노드를 만들게 되니까, 이미지를 n개의 patch로 나누고 이를 node로 인식하고, ViG를 이들 node 사이의 정보를 변환하고 교환하는데 사용한다.
ViG의 기본적인 cell은 다음과 같다.
- Grapher
- FFN module
Grapher module은 graph information processing을 위해 graph convolution에 기반해 만들어진다.
전형적인 GNN의 over-smoothing 현상을 피하기 위해, FFN module은 node feature transformation을 사용하고 node diversity를 증진시킨다.
Grapher and FFN module을 사용해, ViG model을 isotropic, pyramid 방식으로 둘 다 만든다.
실험에서, Image classification / Object detection에서 ViG models의 성능을 보인다.
우리가 아는 바로는, 이 연구가 처음으로 성공적으로 graph neural network를 large-scale visual tasks에 적용한 경우이다.
더 많은 영감을 불어 넣어 주길 바란다.
- CNNs이 처음 성공적인 결과를 냈다.
- ViT가 visual tasks를 발전 시킴.
- MLP 또한 새롭게 연구되었다.
Graph Neural Network
- GCNs은 graph data에 광범위하게 사용됨.
- GCN을 computer vision에 적용 시키는 건 point clouds classification, scene graph generation, action recogntion 등.
- Computer vision에 일반적인 사용을 위해, image data에 직접적으로 사용될 GCN-based backbone이 필요하다.
3. Approach
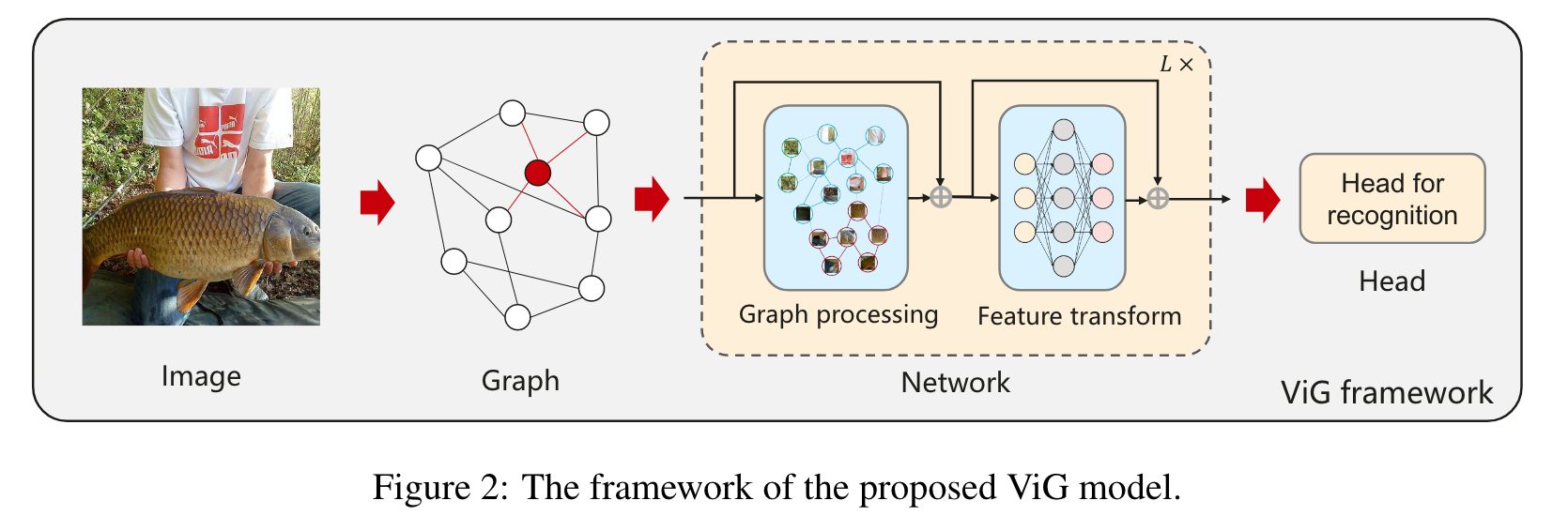
3.1. ViG Block
Graph Structure of Image
H×W×3 인 image를 N patches로 나눈다.
각각의 patch를 feature vecor xi∈RD로 변형해, X=[x1,x2,...,xN] 을 얻는다.
-
D는 feature dimension
-
i=1,2,...,N
이 Features들은 a set of unordered nodes 로 볼 수 있다. : V={v1,v2,...,vN}
각각의 node vi 에 대해,
- K nearest neighbors N(vi)를 찾는다.
- 모든 vj∈N(vi) 에 대해 vj 에서 vi 로 향하는 edge eji 를 추가한다.
그러면 graph G=(V,E) 를 얻게 된다.
- 앞으로 graph construction process를 G=G(X) 로 표현하도록 하겠다.
Image를 graph data로 인식함으로써, representaion을 잡아 내기 위해 GNN을 어떻게 사용할 지 알아 보자.
Image를 이러한 graph로 표현하는 것에는 다음과 같은 이점이 있다.
- graph는 grid와 sequence의 일반화된 자료 구조이고, 이들은 graph의 특별한 경우라 생각할 수 있다.
- graph는 grid 또는 sequence보다 정사각형이 아닌 복잡한 대상을 모델링 하는데 유연하다.
- 물체는 부분의 조합으로 생각될 수 있다. (예 : 사람이 대략적으로 머리, 상체, 팔 다리로 구분되듯이), 그리고 그래프 구조는 이러한 부분 사이의 연결을 표현 할 수 있다.
- GNN에서 발전된 연구들이 visual task를 수행하는데 전환될 수 있다.
Graph-level processing
일반적으로 feature X∈RN×D 에서 시작한다.
- N은 Patch의 수, D 는 Feature dimension
feature들에 기반하여 graph G=G(X)를 만든다.
Graph convolutional layer는 이웃 nodes들 사이의 features를 결합함으로써 정보를 교환할 수 있다.
특히, graph convolution은 다음과 같이 작동한다.
G′=F(G,W)=Update(Aggregate(G,Wagg),Wupdate),
- Wagg 와 Wupdate 는 각각 aggregation 과 update 연산에서 학습 가능한 weights
- aggregation 연산은 이웃 nodes들의 features를 결합해 node의 representation을 계산한다.
- update 연산은 aggregated 된 feature를 더 merge한다.
xi′=h(xi,g(xi,N(xi),Wagg),Wupdate),
- N(xil) 은 xil 의 이웃 nodes의 집합
단순함과 효율성을 위해 max-relative graph convolution을 적용한다. [DeepGCNs]
g(⋅)=x′′=[xi,max({xj−xj∣j∈N(xi)})],h(⋅)=xi′=xi′′Wupdate
graph convolution의 multi-head update operation을 추가적으로 도입한다.
aggregated feature xi′′ 는
- h heads i.e. head1,head2,...,headh 로 나눈다.
- heads은 다른 weights로 각각 update 된다.
모든 heads들은 병렬적으로 update 될 수 있고 마지막 값으로 concatenated 된다.
xi′=[head1Wupdate1,head2Wupdate2,...,headhWupdateh,].
Multi-head update 연산은 model이 다수의 representation subspaces에서 정보를 update하게 하고, feature diversity 측면에서 효과가 있다.
ViG block
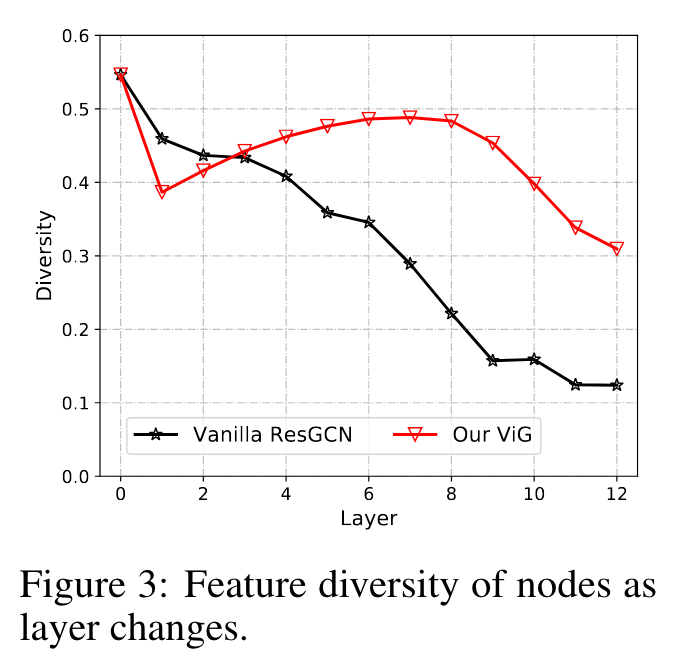
이전의 GCNs은 일반적으로 반복적으로 여러 graph convolution layers에서 graph data의 통합적인 특징을 추출하는데 사용된다.
GCNs의 over-smoothing 현상은 노드 특징을 잘 잡아내지 못하고 성능의 감소를 일으킨다.
이러한 문제를 막기 위해 ViG에 추가적인 feature transformation과 비선형 activation을 도입한다.
graph convolution 전후에 linear layer를 적용한다.
Grapher module은 다음처럼 표현될 수 있다.
Y=σ(GraphConv(XWin))Wout+X,
- X∈RN×D
- Y∈RN×D
- Win 과 Wout 은 fully-connected layers의 weight
- σ 는 activation function (e.g. ReLU and GELU)
- bias term은 제거됨
Feature transformation capacity를 늘리고, over-smoothing 현상을 완화하기 위해, FFN을 각 node에서 사용한다.
FFN module은 2개의 Fully-conntect layers를 포함한 단순한 multi-layer perceptron FFN module이다.
Z=σ(YW1)W2+Y,
- Z∈RN×D
- W1 과 W2 는 fully-connected layers의 weight
- bias term은 제거됨
Grapher와 FFN modules 둘 다 batch normalization이 매 fully-connected layer 또는 graph convolutional layer에서 적용되었는데, 이는 위 식에서는 제외 되었다.
Grapher module과 FFN module의 stack은 ViG block을 구성하고, 이는 network를 만드는게 기초적인 단위가 된다.
ResGCN과 비교 했을 때 ViG는 feature diversity를 유지하고 구별되는 representation을 잘 학습한다.
3.2. Network Architecture
computer vision 분야에서, 일반적으로 사용되는 transformer는 일반적으로 isotropic architecture (e.g., ViT)를 가지고, 반면 CNN은 pyramid architecture (i.e., ResNet)을 가진다.
광범위한 비교를 위해 ViG를 두 가지 구조로 설계해서 각각 비교해 보겠다.
Isotropic arhitecture
Isotropic 구조란 main body가 Network 전체에서 동일한 크기와 모양의 feature를 가지는 구조이다. (e.g. ViT, ResMLP)
세 가지 version의 ViG를 만들었다. i.e. ViG-Ti, S, and B
- node의 수는 N=196 으로 설정.
- receptive field를 단계적으로 넓히기 위해, neighbor nodes K 가 9에서 18까지 선형적으로 증가.
- heads의 수는 h=4 로 기본 설정
Pyramid architecture
Pyramid 구조는 ResNet, PVT 처럼 Layer가 깊어질 수록 점진적으로 작은 spatial size의 feture를 추출하는 multi-scale property를 가진다.
경험적인 증거는 pyramid 구조가 visual task에 유용하다는 증거가 된다.
4가지 다른 형태의 pyramid ViG model을 만들었고 세부 사항은 다음과 같다.
- 많은 nodes를 다루기 위해 처음 두 단계에서 spatial reduction을 사용했다. [PVT]
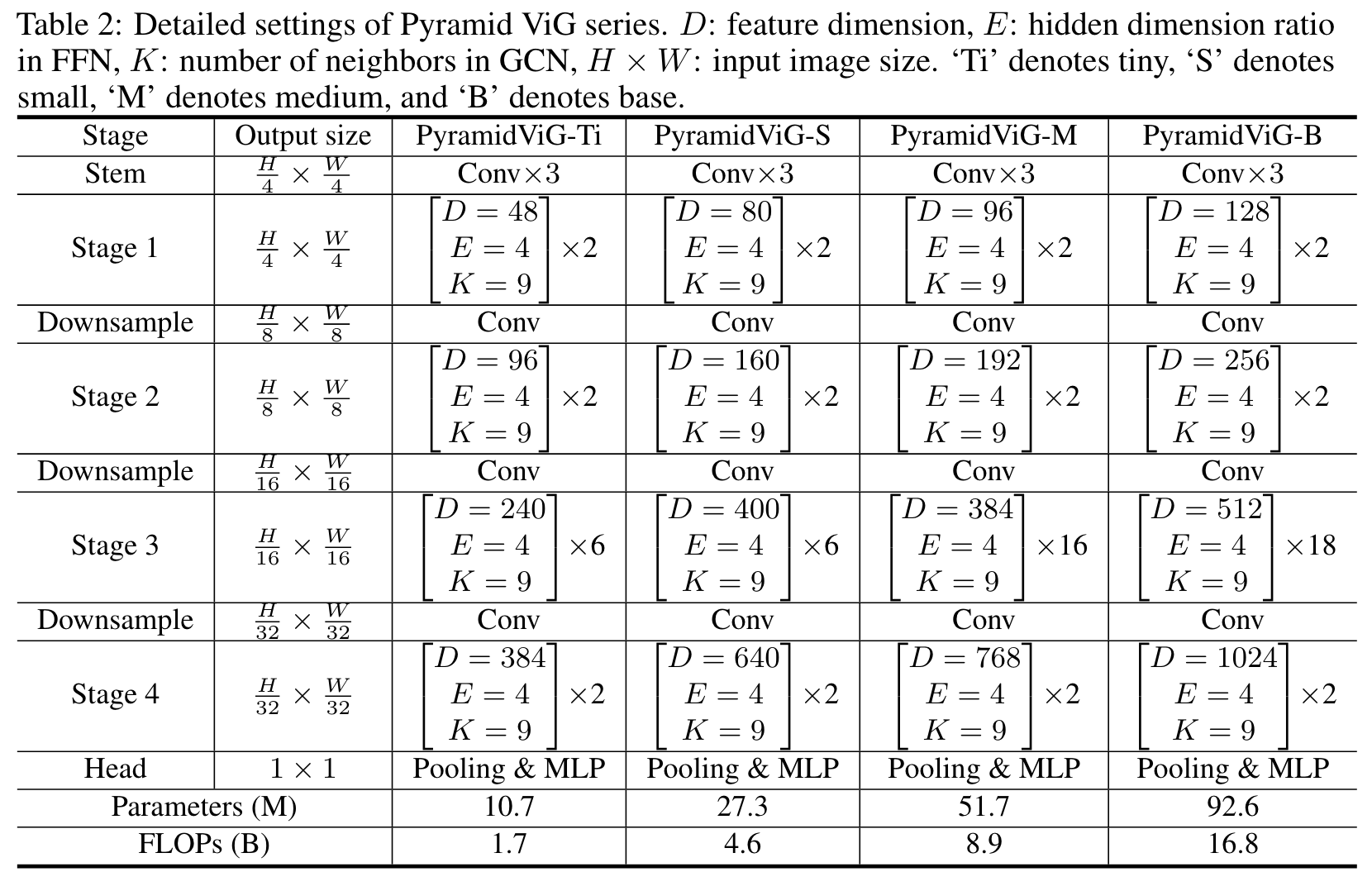
Positional encoding
node의 position information을 표현하기 위해, 각각의 node feature에 positional encoding vector를 더한다.
xi←xi+ei
- ei∈RD
isotropic and pyramid architecture에 둘 다 사용된다.
Pyramid ViG에 대해서는, Swin Transformer와 같이 더 발전된 디자인에서 사용되는 relative positional encoding을 사용했다.
- node i 와 j 에 대해, 이들 사이의 relative positional distance는 eiTej
- graph를 만들기 위해 feature distance에 추가된다.
4. Experiments
4.1.1. Dataset
- Image classification - ImageNet ILSVRC 2012
- Object detection - COCO 2017
4.1.2. Experimental Setting
- 공통
- diated aggregation 사용 [DeepGCNs]
- GELU
- PyTorch, MindSpore으로 구현
- 8개의 NVIDIA V100 GPU
- ImageNet classification
- 공평한 비교를 위해 DeiT에서 제안한 training strategy 사용
- Data augmentation - RandAugment, Mixup, Cutmix, random erasing, repeated augment
- COCO detection
- RetinaNet, Mask R-CNN이 Frame works
- Pyramid ViG를 backbone으로 사용
4.2. Main Results on ImageNet
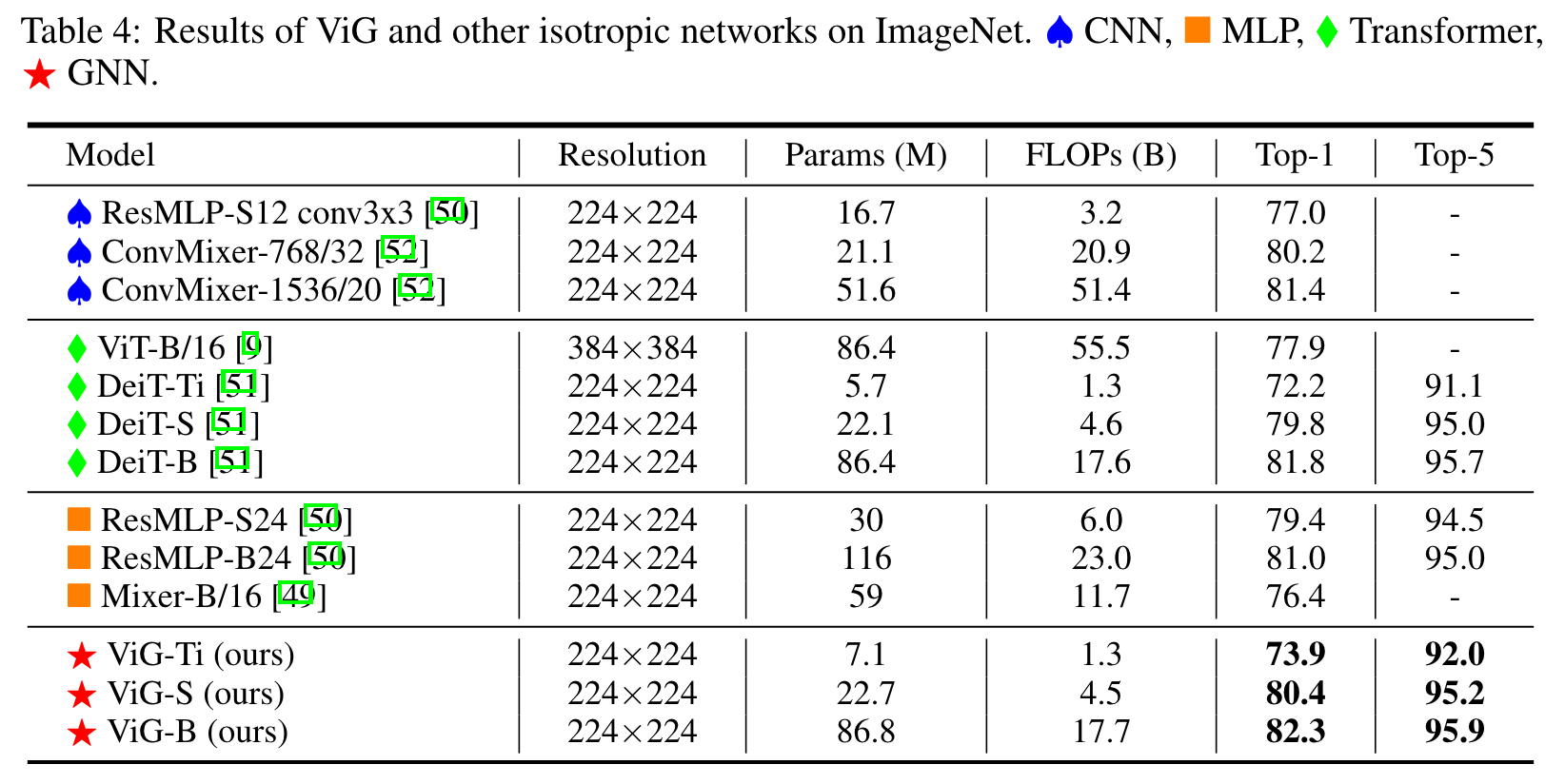
- ViG-Ti가 DeiT-Ti model보다 1.7% 높은 73.9% top-1 accuracy를 비슷한 computational cost로 달성했다.
- Pyramid ViG
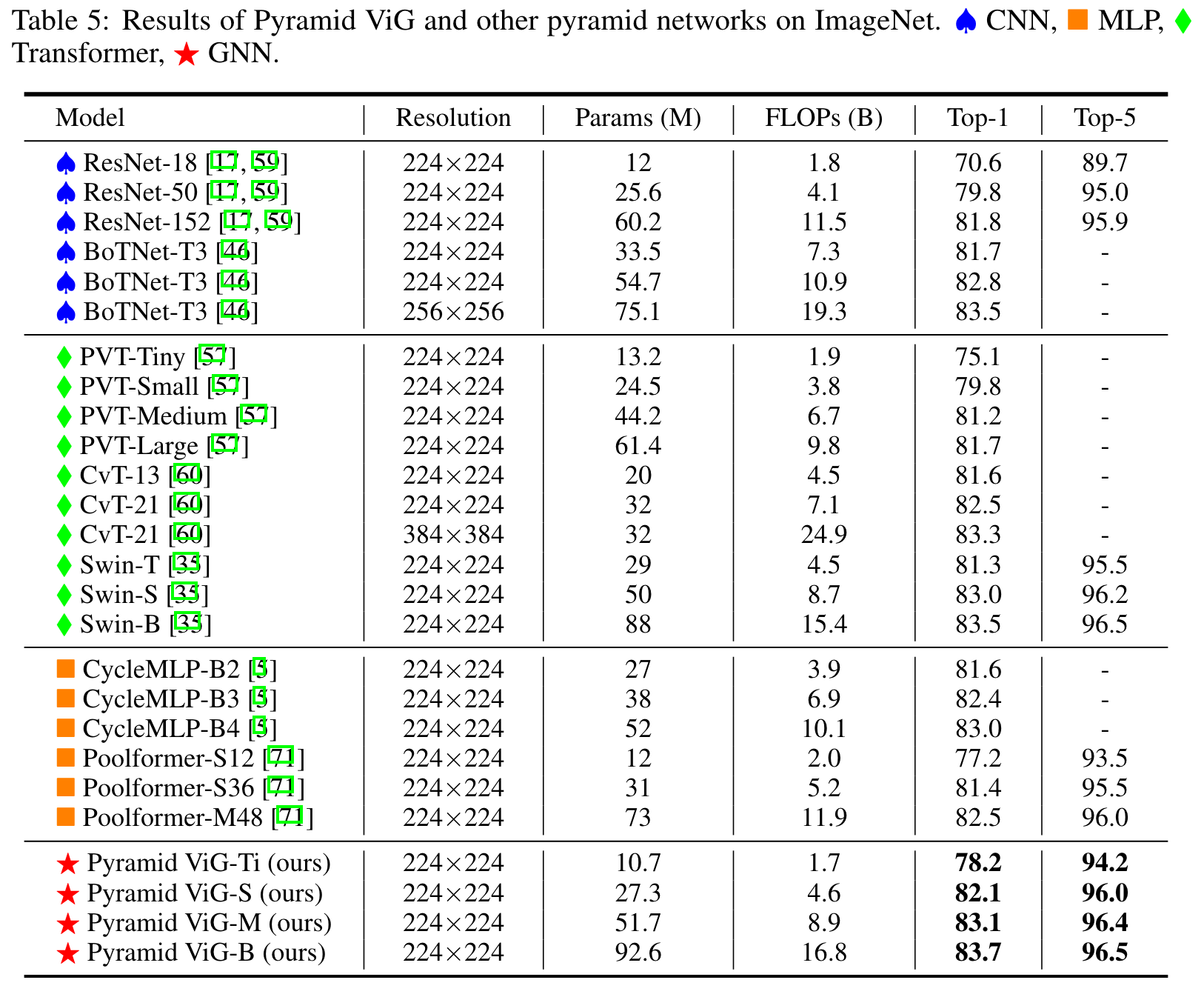
- CNN, MLP, transformer를 사용한 SOTA 와 비슷하거나 더 좋은 성능을 보였다.
- GNN이 visual task에서 basic component가 될 potential을 지님.
4.3. Ablation Study
- Type of graph convolution.
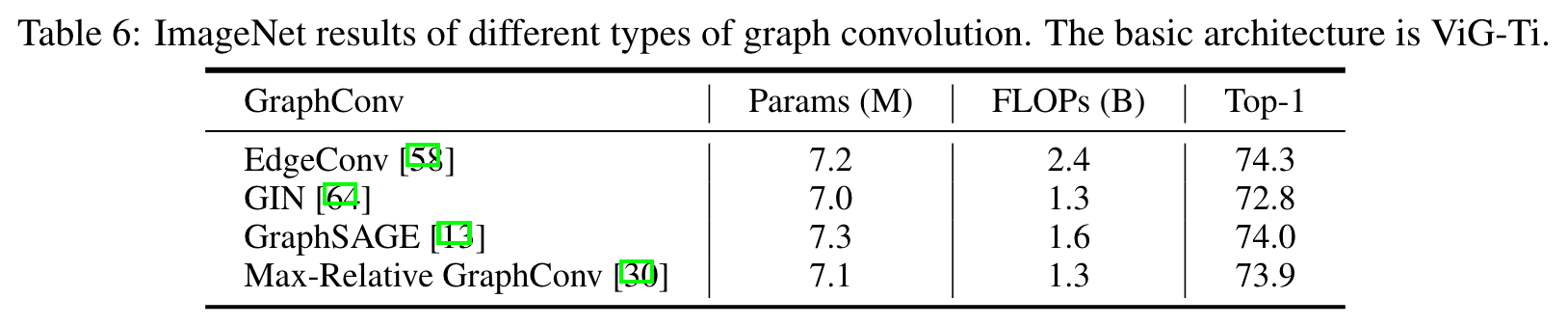
- 다양한 Graph Convolution을 실험 해 봤을 때에도 DeiT-Ti 보다 성능이 좋았다.
- Max-Relative가 FLOPs 와 accuracy를 고려했을 때 가장 유리해 default로 사용했다.
- The effects of modules in ViG.
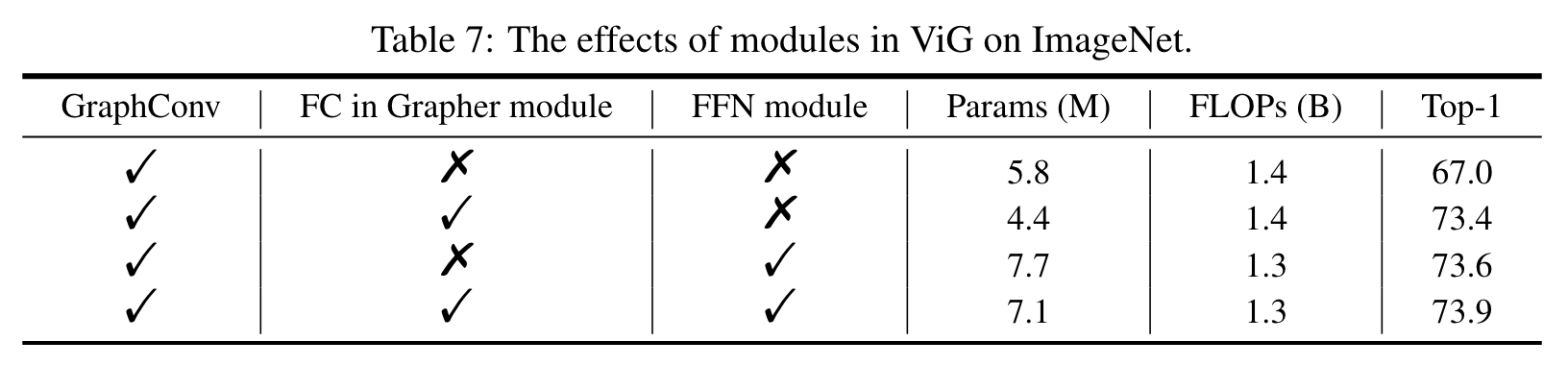
- GNN을 visual task에 적용하기 위해, Grapher module에 FC layers를 도입하고, FFN block을 feature transformation에 사용.
- 공평한 비교를 위해 FLOPs를 비슷하게 만들기 위해 feature demension을 조절
- graph convolution을 image classification에 바로 사용하면 성능이 좋지 않다. FC, FFN을 추가함으로 성능 향상.
- The number of neighbors.

- K 는 hyperparameter.
- 너무 적은 이웃을 참고하면 information 교환이 더디고, 너무 많이 참고하면 over-smoothing
- K 를 3에서 20까지 테스트. 9에서 15가 가장 좋았다.
- The number of heads.

- Multi-head update는 Grapher module이 다른 subspaces의 node features를 처리하게 한다.
- h=4 가 FLOPs, accuracy를 고려했을 때 최선의 선택.
4.4. Object Detection
- 공평한 비교를 위해 ImageNet pretrained Pyramid ViG-S를 RetianNet과 Mask R-CNN의 backbone으로 사용.
- ViG-S가 다른 종류의 representative backbones보다 더 좋은 성능을 보였다.
4.5. Visualization
- 두 개의 node만 표현
- 낮은 layer에서는 색, 질감 같은 low-level, local features에 집중
- 깊은 layer에서는 더 semantic하고 같은 category에 해당한다.
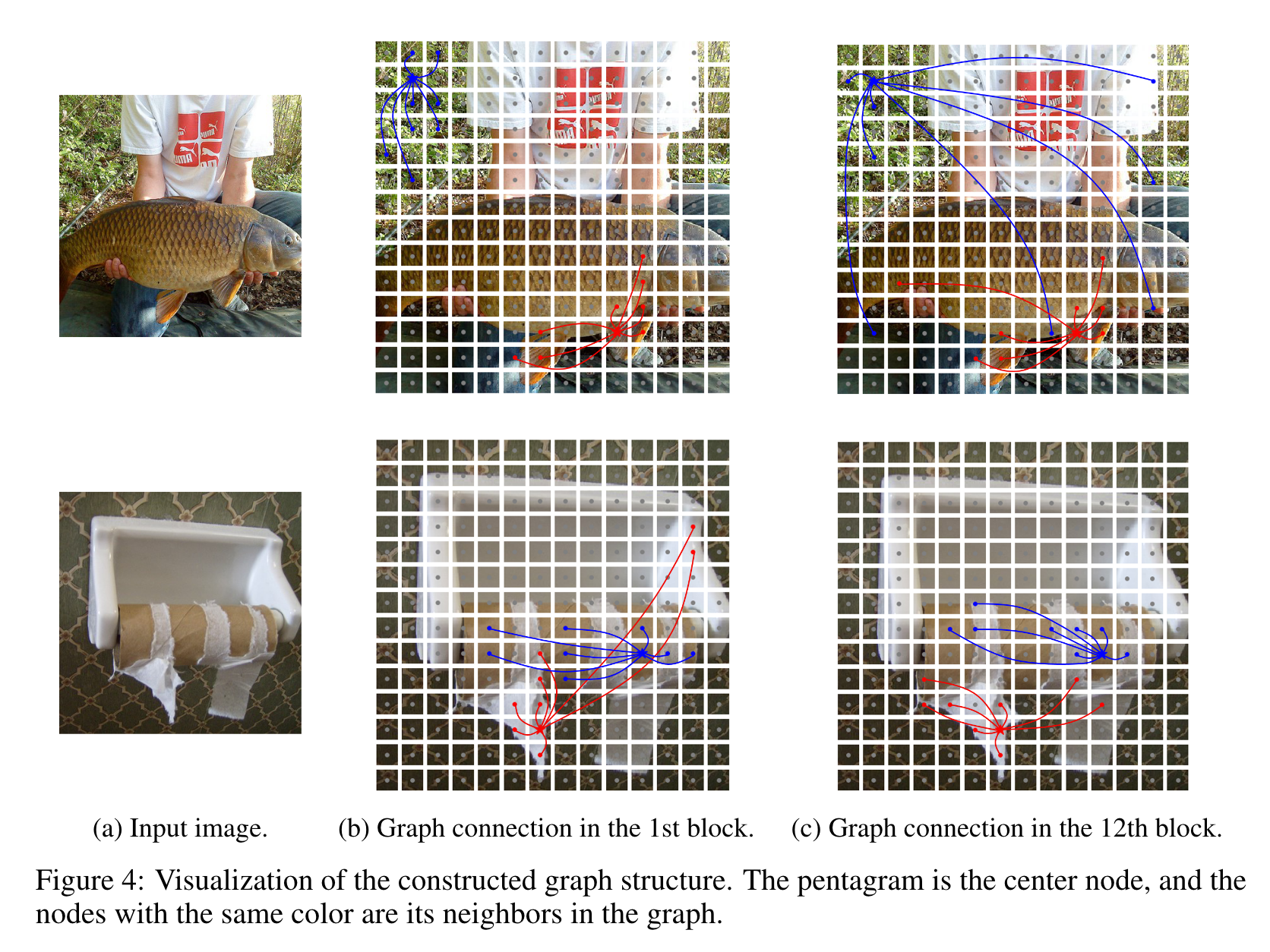
5. Conclusion
Image를 graph로 표현하고 graph neural network를 visual task에 이용하는 방식을 탐구했다.
Image를 patches로 나누고 그들을 node로 본다.
이러한 node를 기반으로 graph를 만드는 것은 불규칙하고 복잡합 대상을 표현하는데 더 적합하다.
Image graph 구조에 GCNs을 사용하는 것은 over-smoothing을 야기하고 성능이 좋지 않다.
Information diversity를 위해 각각의 node 안에 추가적인 feature transformation를 실시한다.
Image의 graph 표현과 improved된 graph block에 기초해, vision GNN (ViG) network를 isotropic, pyramid 구조로 설계했다.
Image recognition과 Object detection의 실험해서 ViG의 우수함을 보였다.
이러한 ViG에 대한 선구적인 연구가 일반적인 visual task에 기초적인 구조가 되길 바란다.
Self Discussion
- 핵심 기술
- Image → graph. node : Patch, edge : K-nearest
- Max-relative GraphConv 사용
- Oversmoothing을 억제하기 위한 Feature transform (Linear Weight, Non-linear activation, Residual connection)
- Relative Positional encoding
- Graph edge를 만드는 과정이 K-nearest? 좀 더 나은 방법이 있지 않았을까…
- Oversmoothing을 억제하는 여러 테크닉들이 인상 깊다.
- ‘Patches Are All You Need?”의 ConvMixer처럼… ‘Graph’가 강력한게 아니라 ‘Patch’가 강력한 representation 아닌가?
- 저자의 주장처럼 Image의 Patch representation을 잘 다루는 방식이 graph라면 의미 있을지도?
References
An Image is Worth 16x16 Words: Transformers for Image Recognition at Scale
MLP-Mixer: An all-MLP Architecture for Vision
Attention is Not All You Need: Pure Attention Loses Rank Doubly Exponentially with Depth
DeepGCNs: Can GCNs Go as Deep as CNNs?
Pyramid Vision Transformer: A Versatile Backbone for Dense Prediction without Convolutions
Swin Transformer: Hierarchical Vision Transformer using Shifted Windows
Training data-efficient image transformers & distillation through attention
Patches Are All You Need?