Exploring Simple Siamese Representation Learning
Exploring Simple Siamese Representation Learning
Xinlei Chen, Kaiming He / Facebook AI Research (FAIR)
Introduction
- 최근 비전 영역 un/self-supervised representation learning이 꽤 발전함
- 여러 아이디어가 있지만, 일반적으로 Siamese network의 형태를 포함한 방식이 많음
- Siamese network을 이용할 때의 문제점은 모든 output이 constant로 "collapsing"한다는 것
- Collapsing을 막기 위해 사용된 기법으로 제시된 것이 최근 주목받은 Contrastive learning(SimCLR), online clustering(SwAV), momentum encoder(BYOL) 등
- 이 논문에서는 large-batch training이 필요없는, 일반적인 batch size(<4096)를 사용해 학습시킬 수 있는 "SimSiam" 방식을 제안
- 실험적으로 stop-gradient operation이 collapsing solution을 효과적으로 방지할 수 있다는 것을 보임
- Siamese network가 inductive bias를 자연적으로 제공
Contrastive learning
- 핵심 아이디어는 positive sample pair 사이 거리를 좁히고, negative sample pair 사이 거리를 넓히는 것
- Contrastive learning은 일반적으로 많은 수의 negative sample들을 이용해 학습
- 잘 동작하기 위해서는 large batch size가 필요
Clustering
- Contrastive learning과는 다르게 negative exemplar를 따로 정의하지 않지만, cluster center를 negative prototype으로 이용
BYOL
- BYOL은 특정 view로부터 또다른 view를 직접 예측
- 한쪽 branch가 momentum encoder로 이루어진 Siamese network로 간주할 수 있음
- stop-gradient operation이 핵심적인 역할
- moving-average가 accuracy를 개선하긴 하지만, collapsing을 방지하는 데에 직접적인 영향은 없음
Method
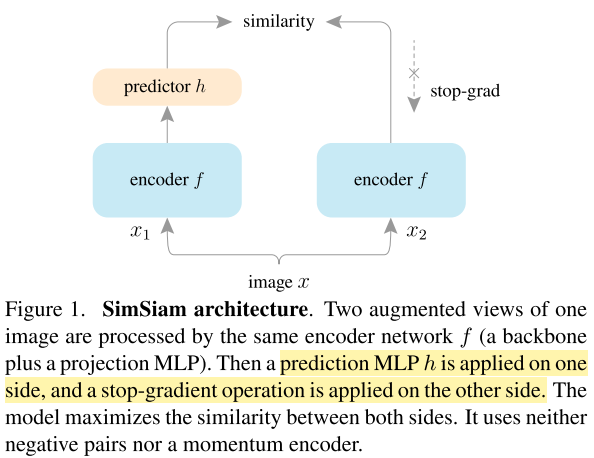
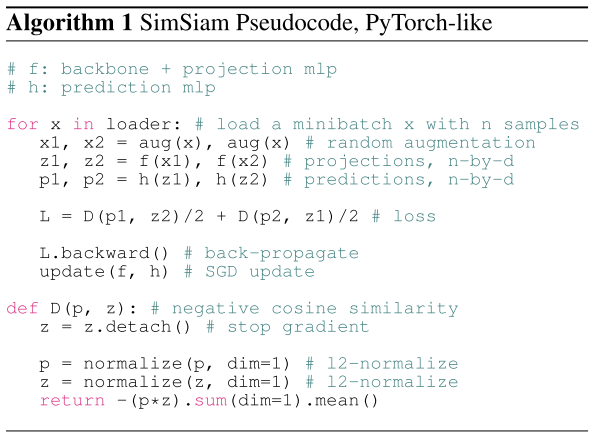
- Negative cosine similarity를 최소화하도록 학습
- Symmetrized loss 사용
L=1/2D(p1,stopgrad(z2))+1/2D(p2,stopgrad(z1))
- Base lr=0.05, default batch size=512
- 서로 다른 batch size에 대해 다른 lr 사용 (lr×BatchSize/256)
- Label을 제외한 ImageNet-1k training set을 사용해 unsupervised pre-training
Empirical Study
Stop-gradient
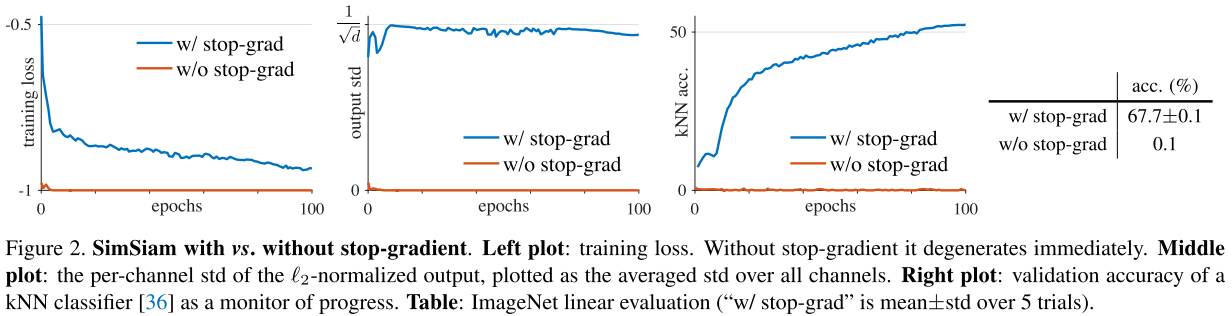
- Stop-gradient가 없는 경우, optimizer가 좋지 않은 solution을 빠르게 찾게 됨
- 좋지 않은 solution: 모든 output이 constant vector로 고정되고, loss가 최소값인 -1로 고정, 모든 샘플에 대해 output std가 0
- Stop-gradient를 적용하면 output std value가 1/d 근처값으로 나오고, 이는 collapsing이 일어나지 않고 output이 unit hypersphere에 분포되었다는 것을 의미함
- Linear classification을 시도했을 때, stop-gradient를 적용한 SimSiam 방식은 67.7% accuracy를 보임
- Stop-gradient가 없는 경우 accuracy는 0.1%로 전혀 학습되지 않은 모습을 보임
- 이는 architecture design만으로는 collapsing을 방지할 수 없다는 것을 의미
Predictor
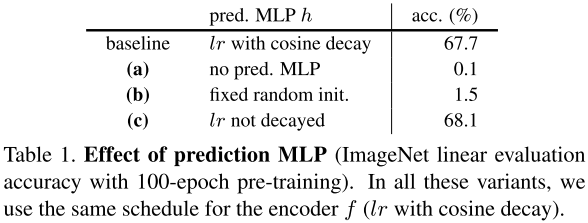
- Predictor의 경우 lr decay 없이 constant lr을 사용할 때 결과가 더 좋음
- Predictor는 latest representation에 맞춰 학습되어야 하기 때문에 representation이 충분히 학습되기 전에 lr을 줄이는 것이 의미가 없을 수 있다고 해석 가능
Others
- Batch size가 256~2048 인 경우 비슷하게 성능이 좋았음
- Batch normalization의 경우 적절하게 사용된다면 성능에 도움이 됨
- Negative cosine similarity 말고도 cross-entropy loss를 사용해도 괜찮게 동작함
- Loss의 symmetrization은 accuracy 향상에 도움은 되지만, collapsing을 방지하는 데에는 도움이 되지 않음
Hypothesis
Comparisons
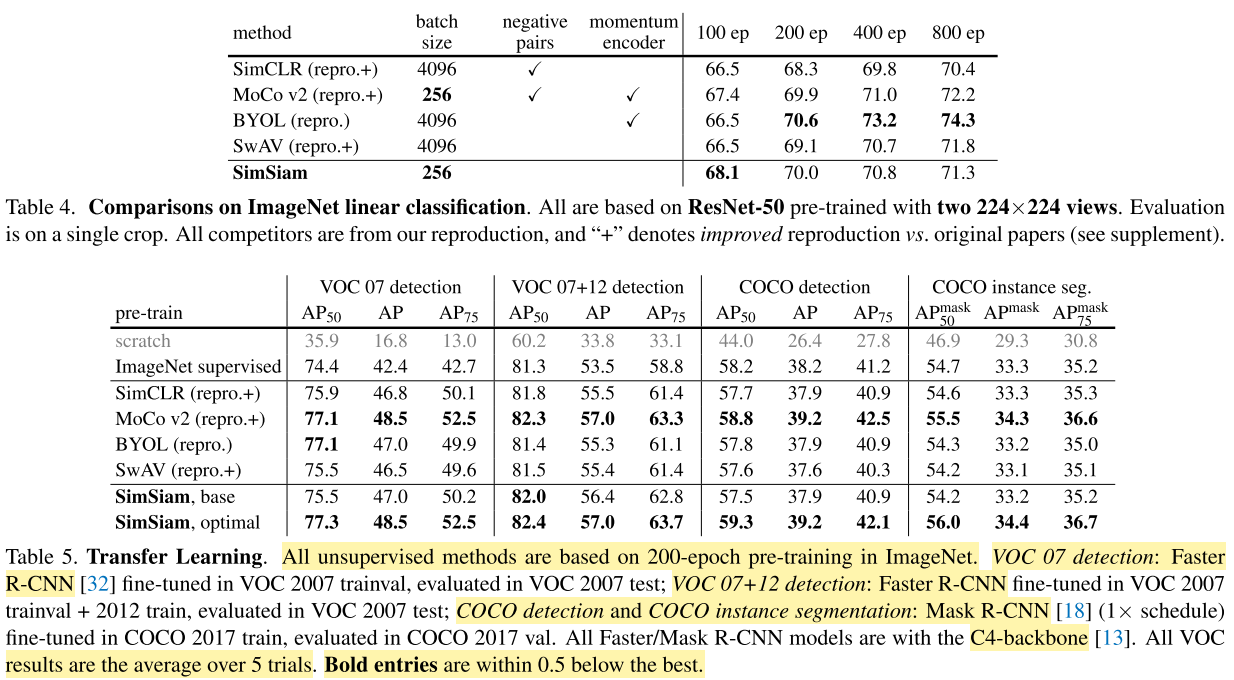
- SimSiam base가 기본 ImageNet pre-trained 모델
- SimSiam optimal은 lr=0.5, wd=1e-5인 optimal한 모델
- 둘다 ImageNet supervised pre-training보다 나은 성능
Conclusion
- 단순한 Siamese network구조에 간단한 수정을 더해 성능개선 성공
So...
- Section 5 - Hypothesis 이해를 위해 Clustering 개념 복습 필요
- Stop-gradient의 활용도에 대한 고민
- 그래서 predictor의 정확한 역할은 무엇인지?
TODO