📖 Introduction
- What make you a good deep learner?
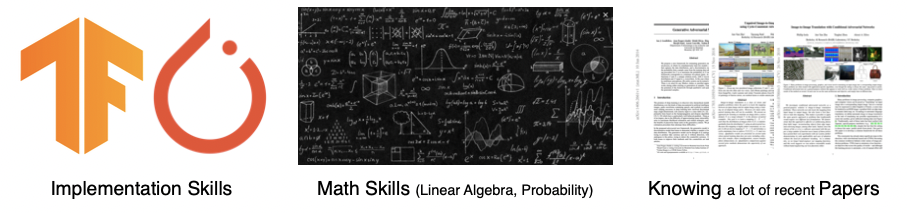
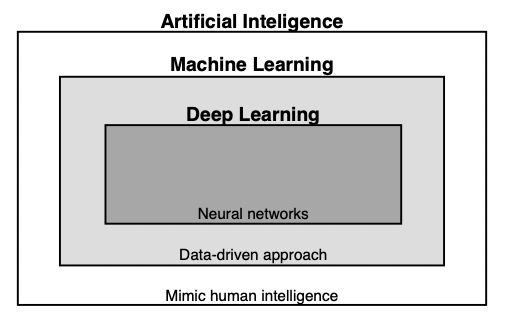
- Deep Learning(Neural networks)
⊂ Machine Learning(Data-Driven approach)
⊂ Artificial Intelligence(Mimic human intelligence)
- Key Components of Deep Learning
- The data that the model can learn from
- The model how to transform the data
- The loss function that quantifies the badness of the model
- The algorithm to adjust the parameters to minimize the loss
- (참고) 새로운 논문 혹은 연구를 봤을 때, 이 4가지 항목에 비추어서 논문 및 연구를 바라보면 기존 연구에 대해서 어떤 장점이 있고 어떤 기여가 있는지 이해하기가 쉬움
📖 Data
- Data depend on the type if the problem solve
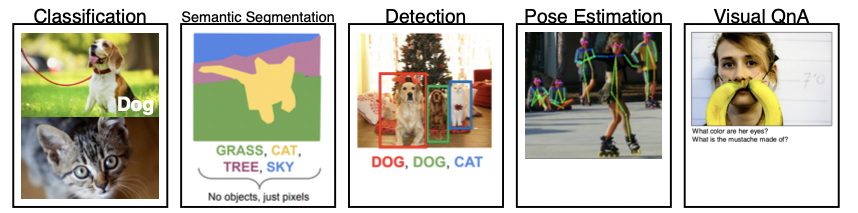
📖 Model
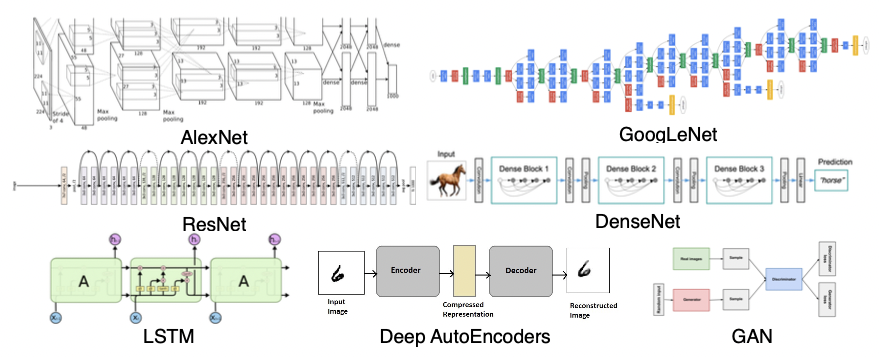
- example : AlexNet, ResNet, LSTM, GoogLeNet, DenseNet, DeepAutoEncodes, GAN and so on.
- 같은 task가 주어졌다하더라도 model의 성질에 따라서 좋은 결과가 나올 수도, 좋지 않은 결과가 나올 수도 있음
📖 Loss
- The loss function is a proxy of what we want to achieve.
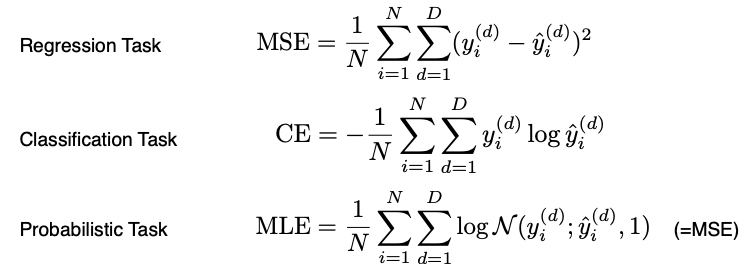
- loss function의 값을 줄인다 하여 우리가 원하는 답을 항상 이룬다는 보장은 없음
📖 Optimization Algorithm
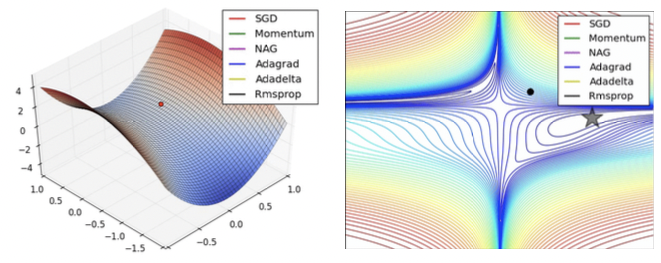
- Regulerizer : Dropout, Early stopping, k-fold validation, Weight decay, Batch normalization, MixUP, Ensemble, Bayesian Optimization
- 네트워크가 단순히 train data에서만 잘 동작하는 것이 아니라 한 번도 보지 못한 test data 혹은 실제 환경에서도 잘 동작하는 목표로 이용하는 알고리즘
<이 게시물은 최성준 교수님의 '딥러닝 기본 용어 설명' 강의 자료를 참고하여 작성되었습니다.>
본 포스트의 학습 내용은 [부스트캠프 AI Tech 5기] Pre-Course 강의 내용을 바탕으로 작성되었습니다.
부스트캠프 AI Tech 5기 Pre-Course는 일정 기간 동안에만 운영되는 강의이며,
AI 관련 강의를 학습하고자 하시는 분들은 부스트코스 AI 강좌에서 기간 제한 없이 학습하실 수 있습니다.
(https://www.boostcourse.org/)