아래에 글은 NAVER의 2023 DEVIEW에서 SYMBIOTE AI의 김태훈님께서 발표해주신 '값비싼 Diffusion model 저비용 MLOps'에 대해 개인적으로 공부하고 리뷰, 정리하여 공유한 글 입니다.
참고
요약
- diffusion model 이란 multimodal image generation model with diffusion process.
- diffusion model의 단점은 학습, 추론 속도가 느리다는 것. 즉, latency와 scalability가 중요함.
- 레이턴시 개선을 위해 GPU 하드웨어적인 방법으로써 GPU의 SRAM과 HBM 사이에 I/O를 줄여주는 FlashAttention이 있음.
- 또한 추론 step을 줄여 레이턴시를 줄이는 distillation 방법도 있음.
- FlashAttention과 유사한 방법으로 TensorRT + Triton을 활용함. 이는 GPU 연산 중에 삼각함수 연산 같은 I/O가 비효율적인 활성 함수들을 개선해주는 방법.
- 추론 인프라의 경우, nvidia triton inference server로 ensemble model과 scheduler를 사용해 비동기적인 추론을 구현하여 추론 속도를 개선함.
개요
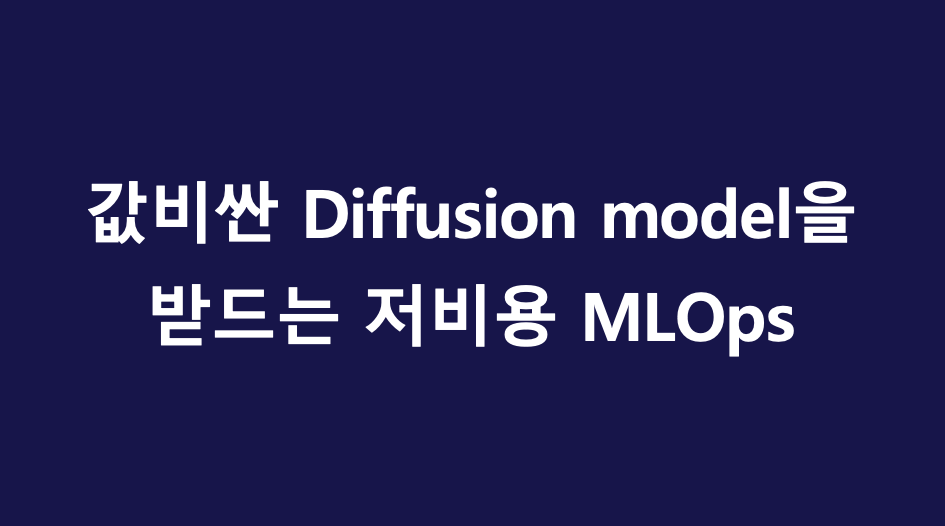
Diffusion model에 대해
Diffusion model이란?
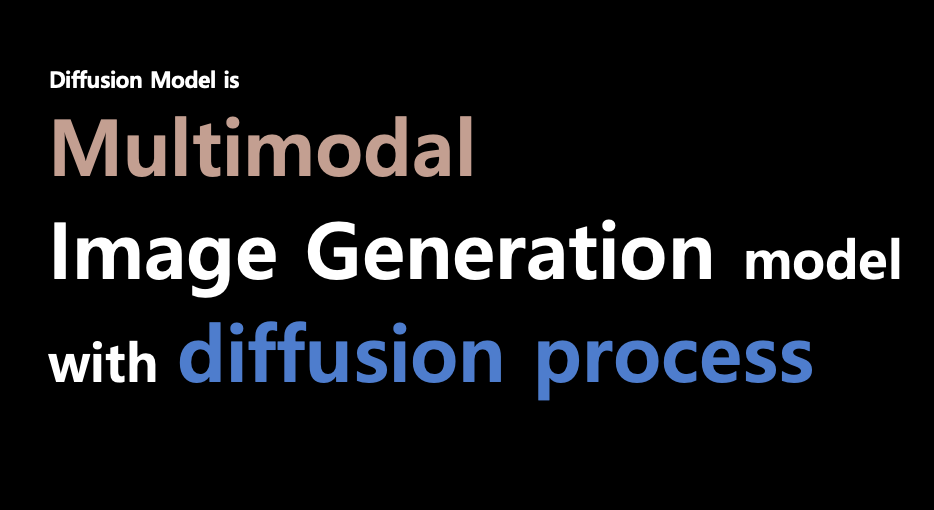
- Diffusion model은 Multimodal Image Generation model with diffusion process를 말함.
Image Generation model 이란?
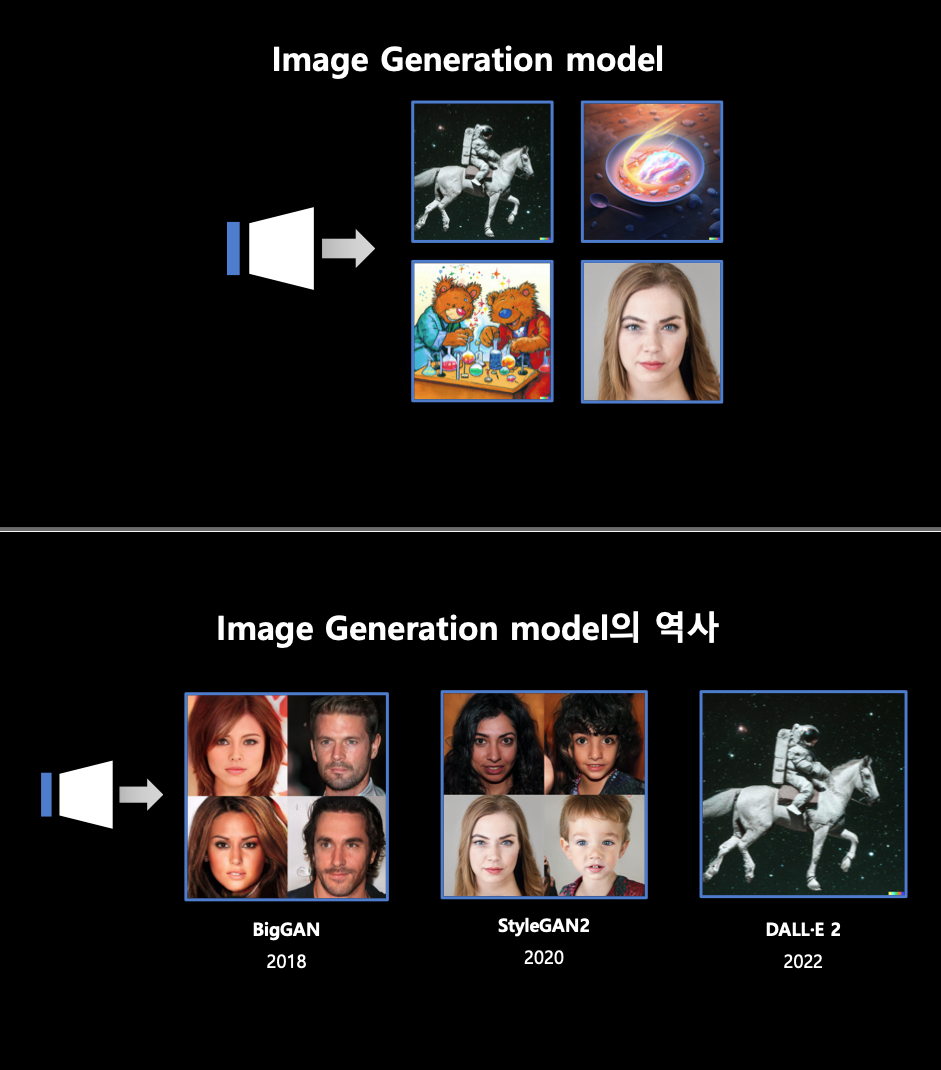
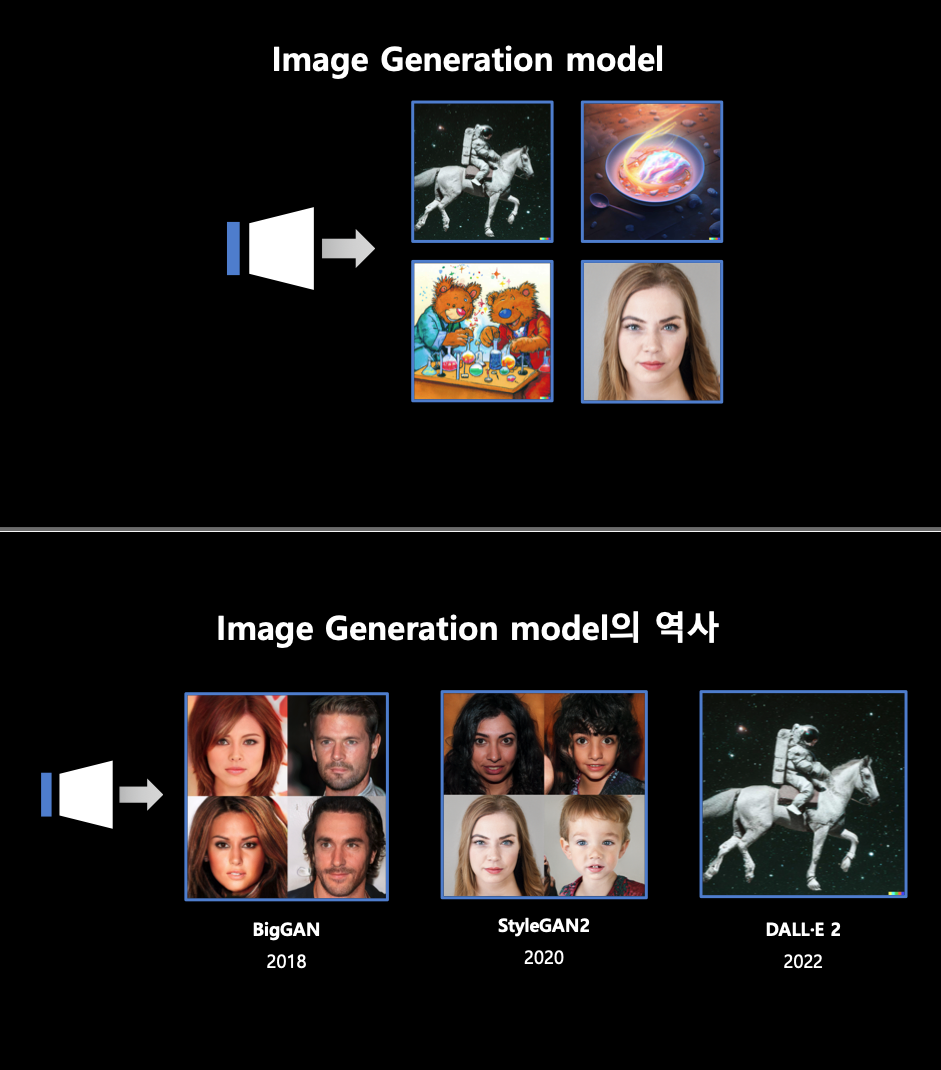
Multimodal 이란?
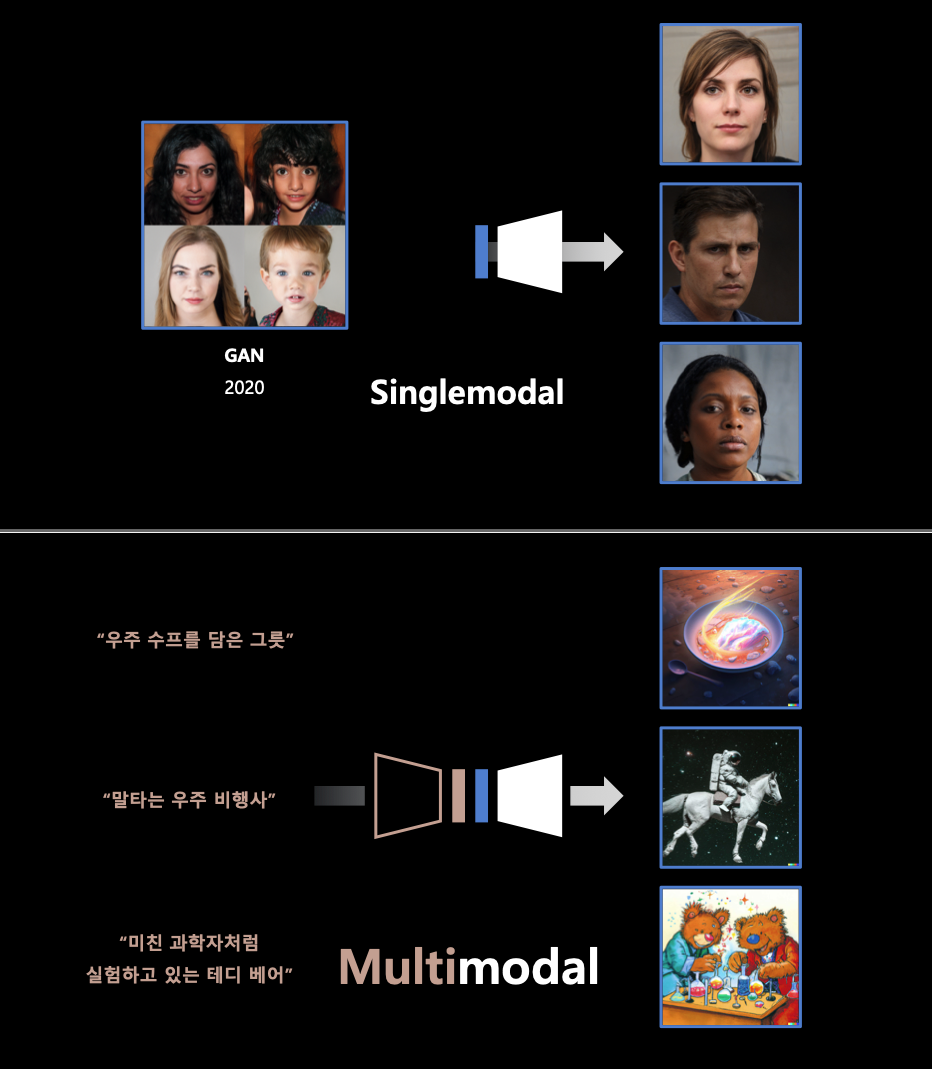
Diffusion model 이란?
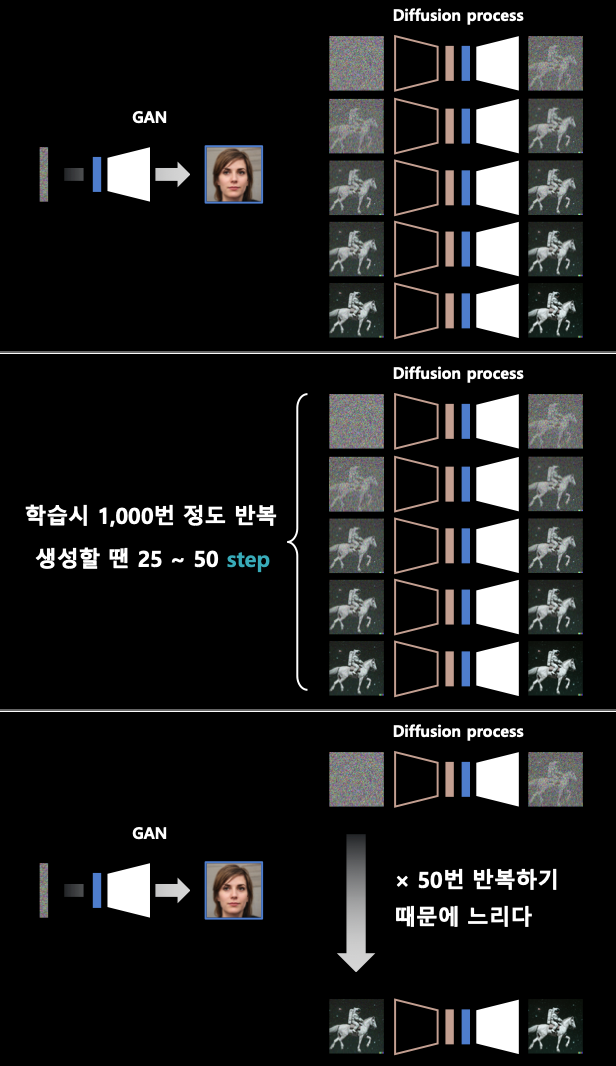
- 추가적으로 별도의 자료 서칭
- Diffusion model은 데이터를 만들어내는 deep generative model 중 하나로, data로부터 noise를 조금씩 더해가면서 data를 완전한 noise로 만드는 forward process(diffusion process)와 이와 반대로 noise로부터 조금씩 복원해가면서 data를 만들어내는 reverse process
를 활용한다.
Diffusion model의 단점
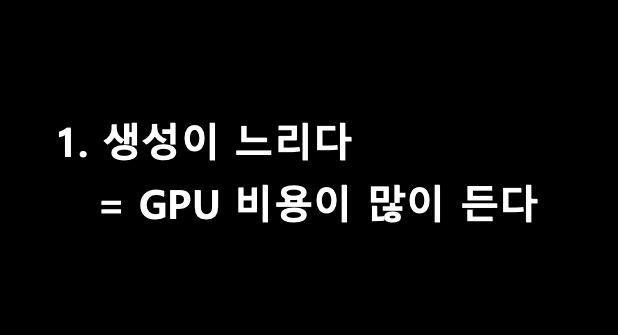
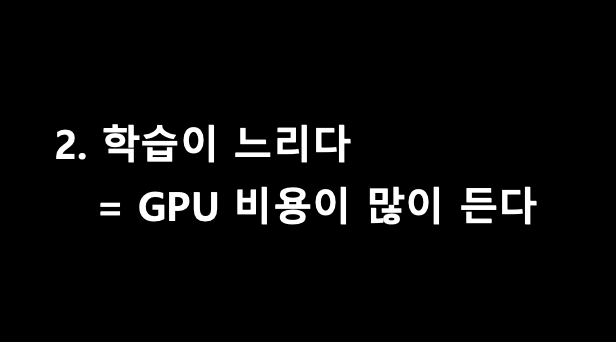
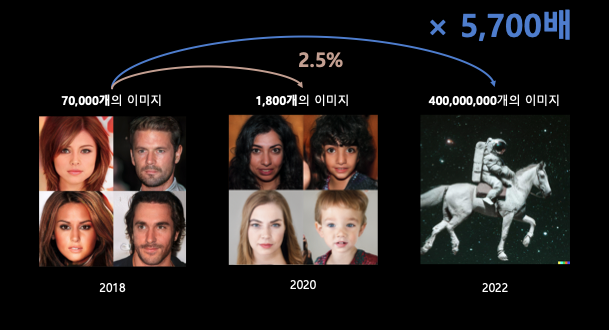
- 학습하려는 데이터의 규모에 대한 트렌드도 점점 작게 하려다가 이전보다 엄청 큰 규모를 활용하는 추세.
Diffusion model의 발전 과정
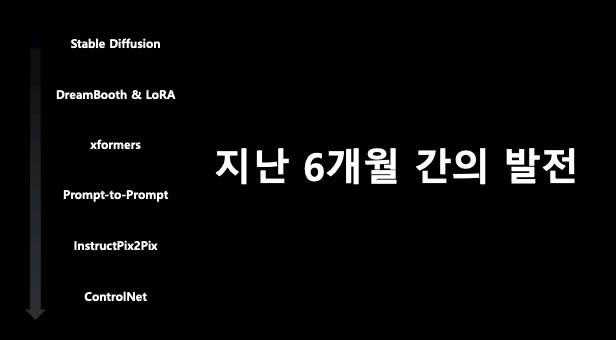
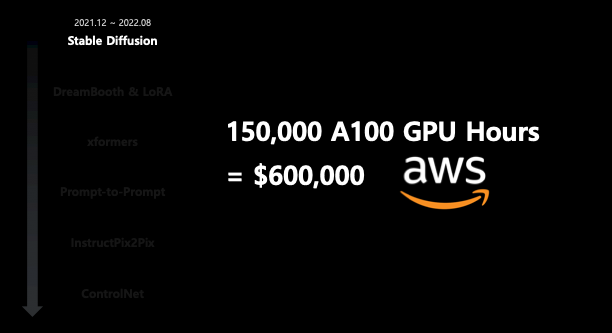
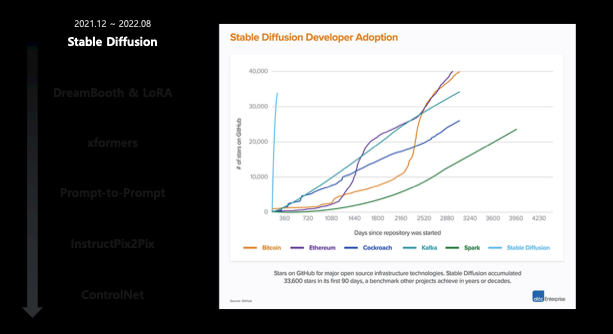
- OSS 중에 Stable diffusion의 역대급 github star 수 증가 추세.
stable diffusion의 학습 시간
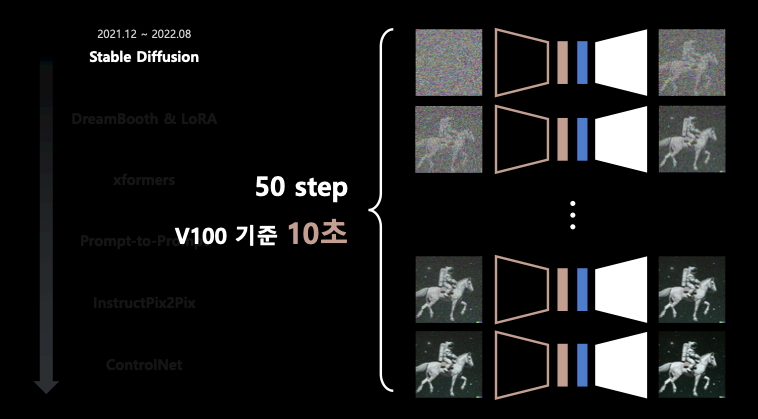
DreamBooth & LoRA
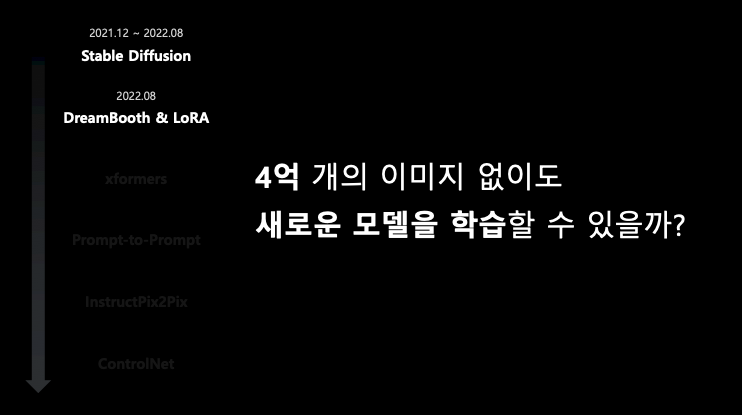
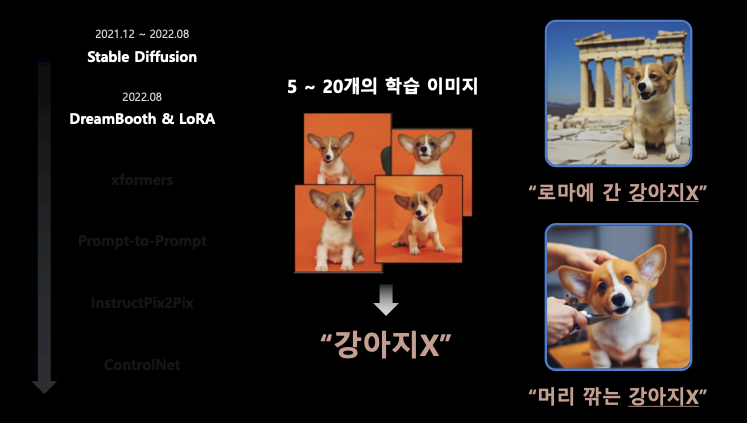
- 적은 데이터로 빠르게 학습하는 서비스도 등장.
FlashAttention
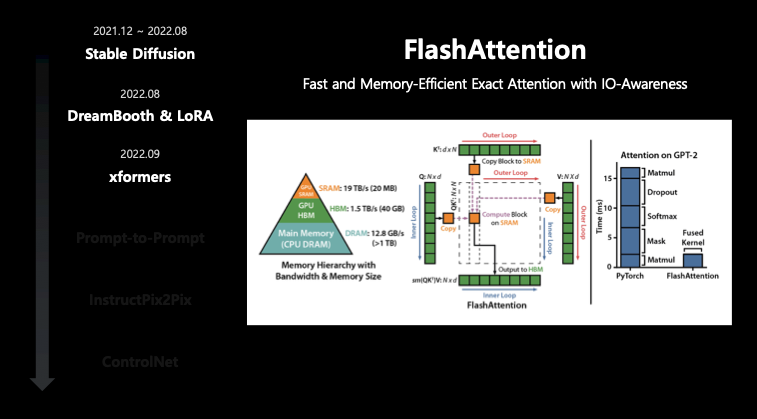
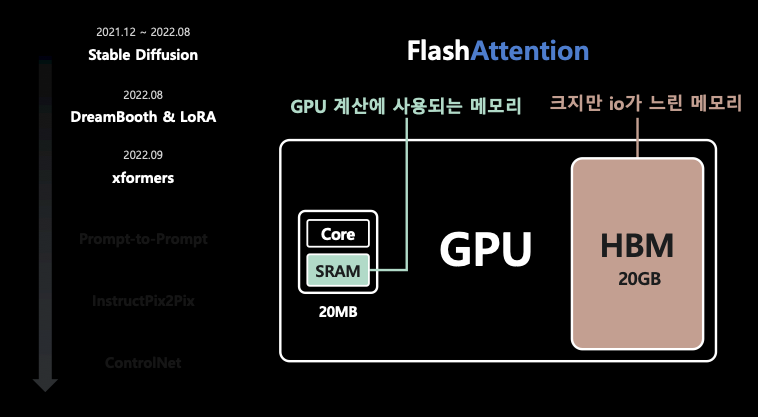
- GPU의 내부 구조는 CUDA Core - SRAM - HBM으로 이루어져 있음.
- 추가 자료 조사
- CUDA Core : GPU에서 다수의 스트림 프로세서(Streaming Processor, SP) 중 하나로 각 SP는 작은 SRAM 캐시와 몇 개의 연산 유닉을 가지고 있음.
- SRAM : Static Random Access Memory. 휘발성 메모리이며 적은 양의 데이터를 저장할 수 있지만 CPU의 캐시 메모리와 비슷하게 매우 빠른 속도로 데이터에 액세스할 수 있는 고속 캐시이므로 주로 CUDA Core의 로컬 메모리로 사용됨. (↔ DRAM과 반대되는 특성이 있음.)
- HBM : High Bandwidth Memory
- 여기서 CUDA 연산을 수행하기 위해 HBM 메모리에 데이터를 올리고 SRAM에 조금씩 옮겨서 Core가 연산을 하는 구조. 즉, HBM과 SRAM의 I/O가 있음. 그런데, SRAM은 20MB 밖에 안 됨. 그래서 SRAM과 HBM 간에 트랜잭션이 굉장히 빈번하고 결국 latency를 만들기 때문에 이 I/O를 줄이는게 속도를 높이는데 있어 중요함.
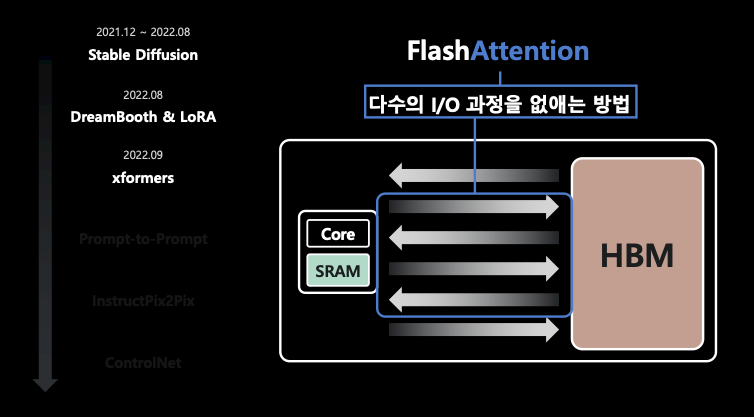
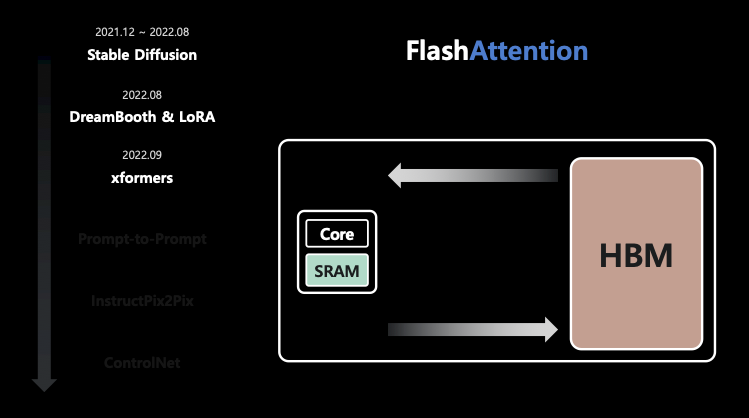
- 이런 다수의 I/O 과정을 줄이기 위해 FlashAttention 등을 사용하곤 함.
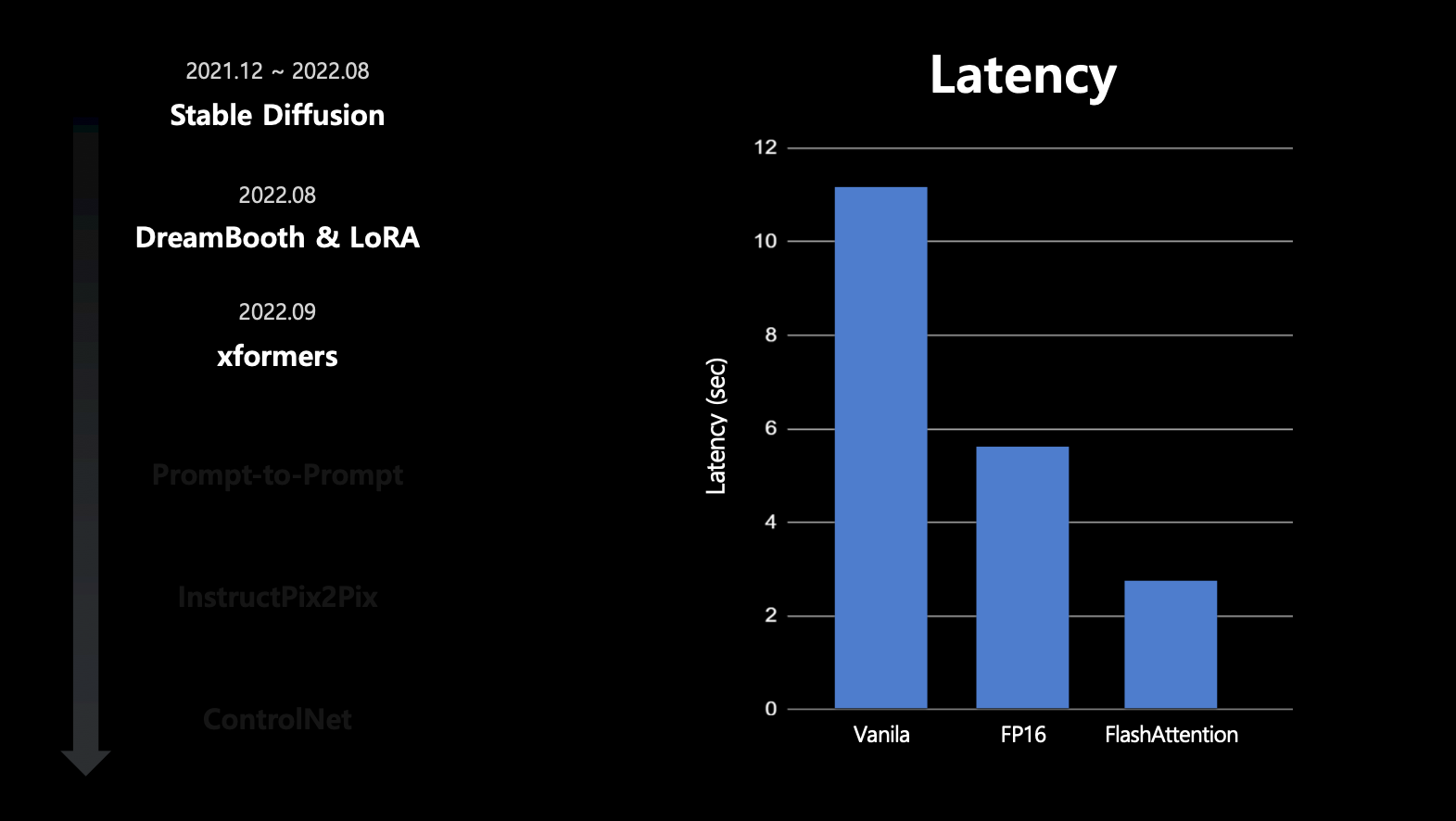
- FlashAttention 사용 시에 급격히 latency가 감소함.
prompt-to-prompt
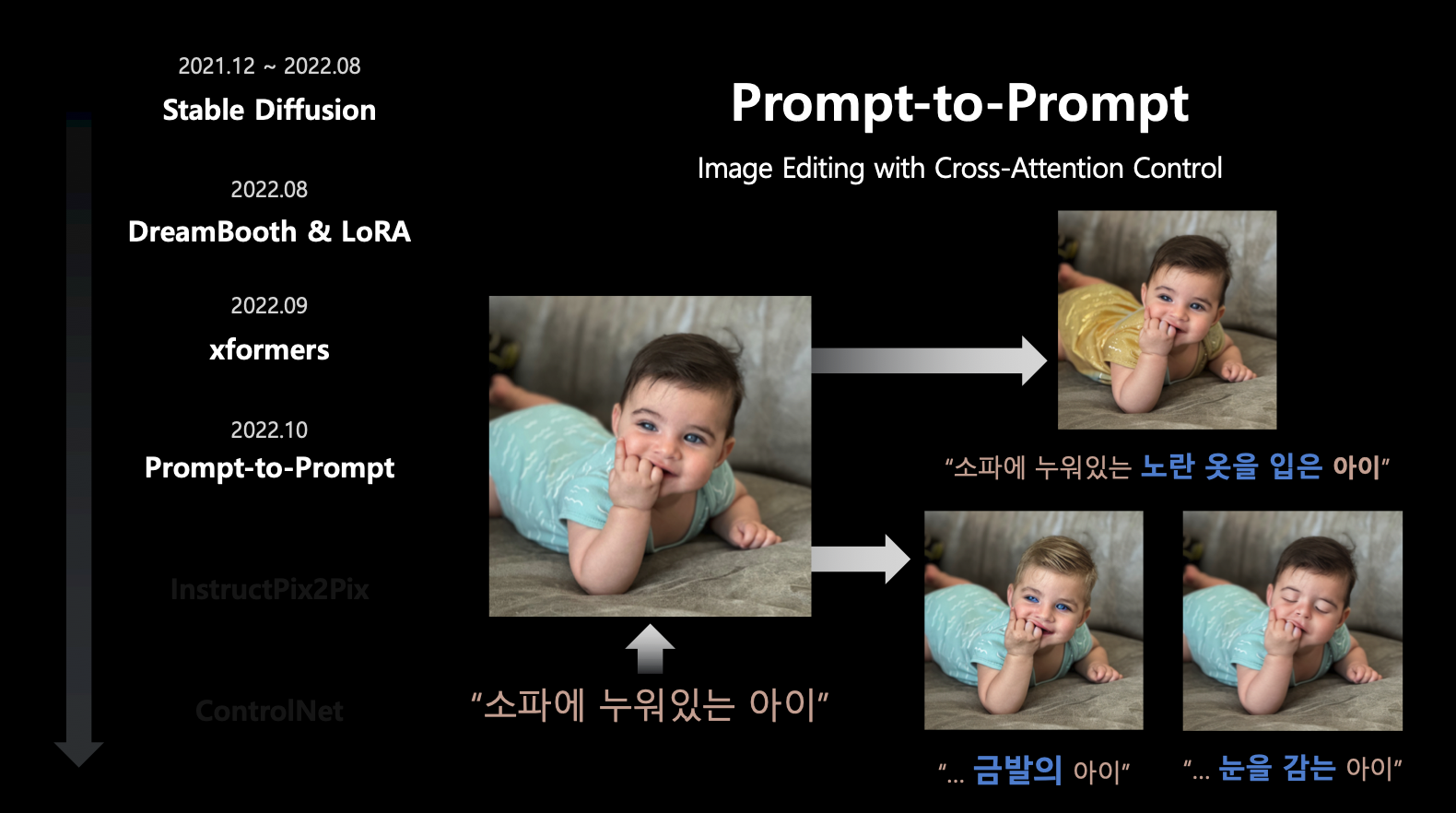
- prompt-to-prompt는 이미지에 작은 수정을 하기 위함.
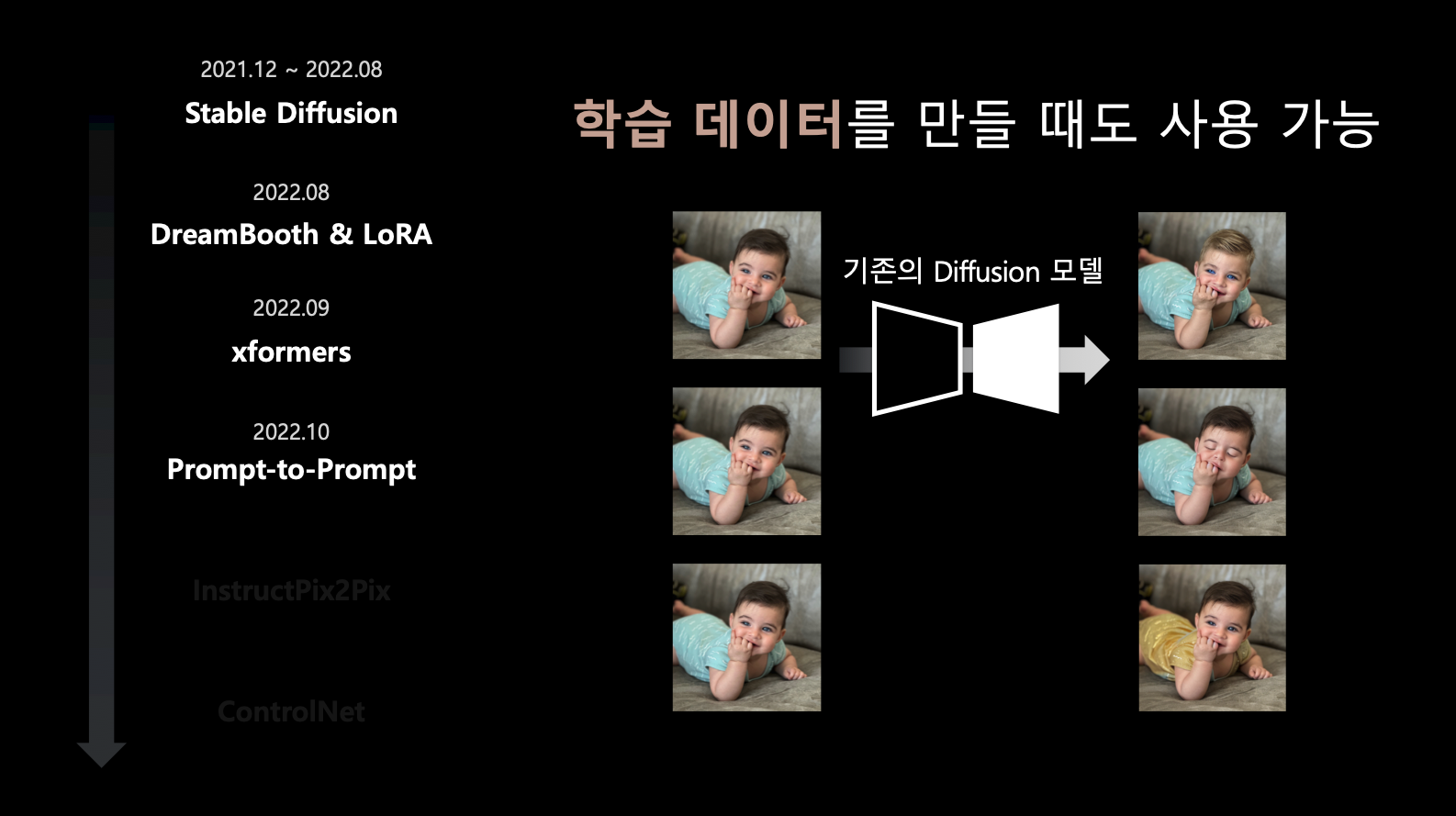
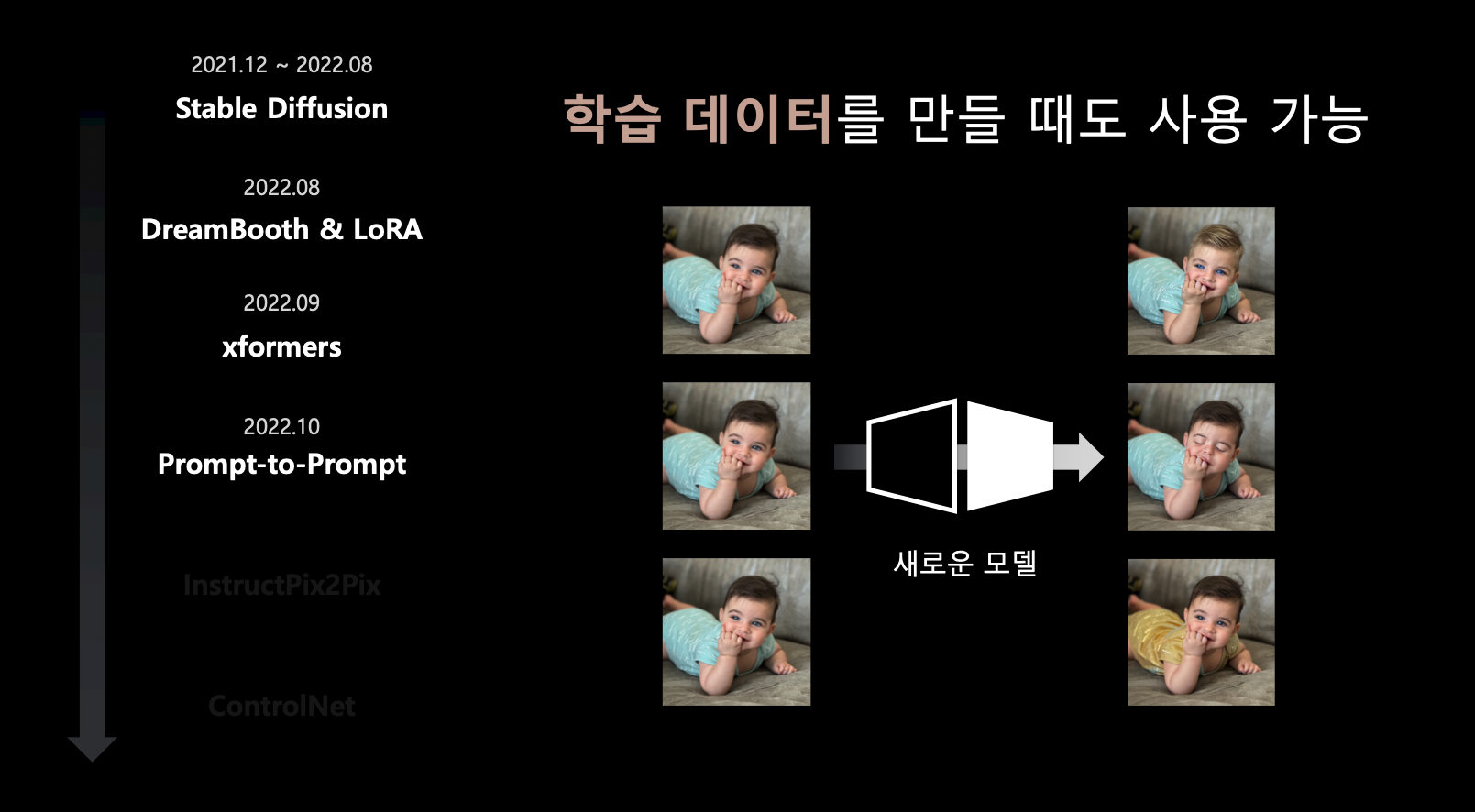
- 재밌는 점은 이 모델로 만든 이미지들을 학습 데이터로 사용 가능하다는 것.
InstructPix2Pix
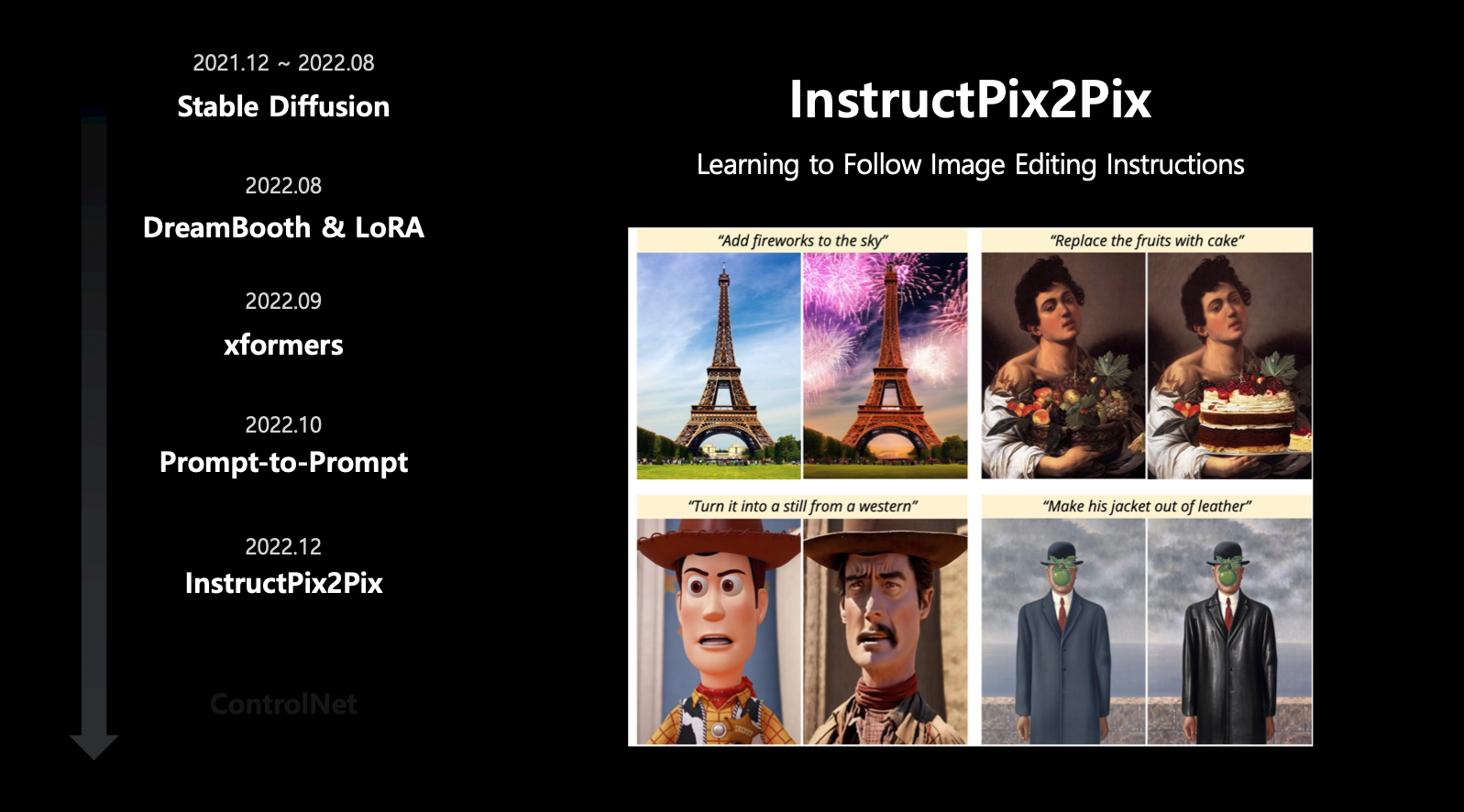
- InstructPix2Pix는 앞서 본 Prompt-to-Prompt를 학습 데이터로 활용한 기술.
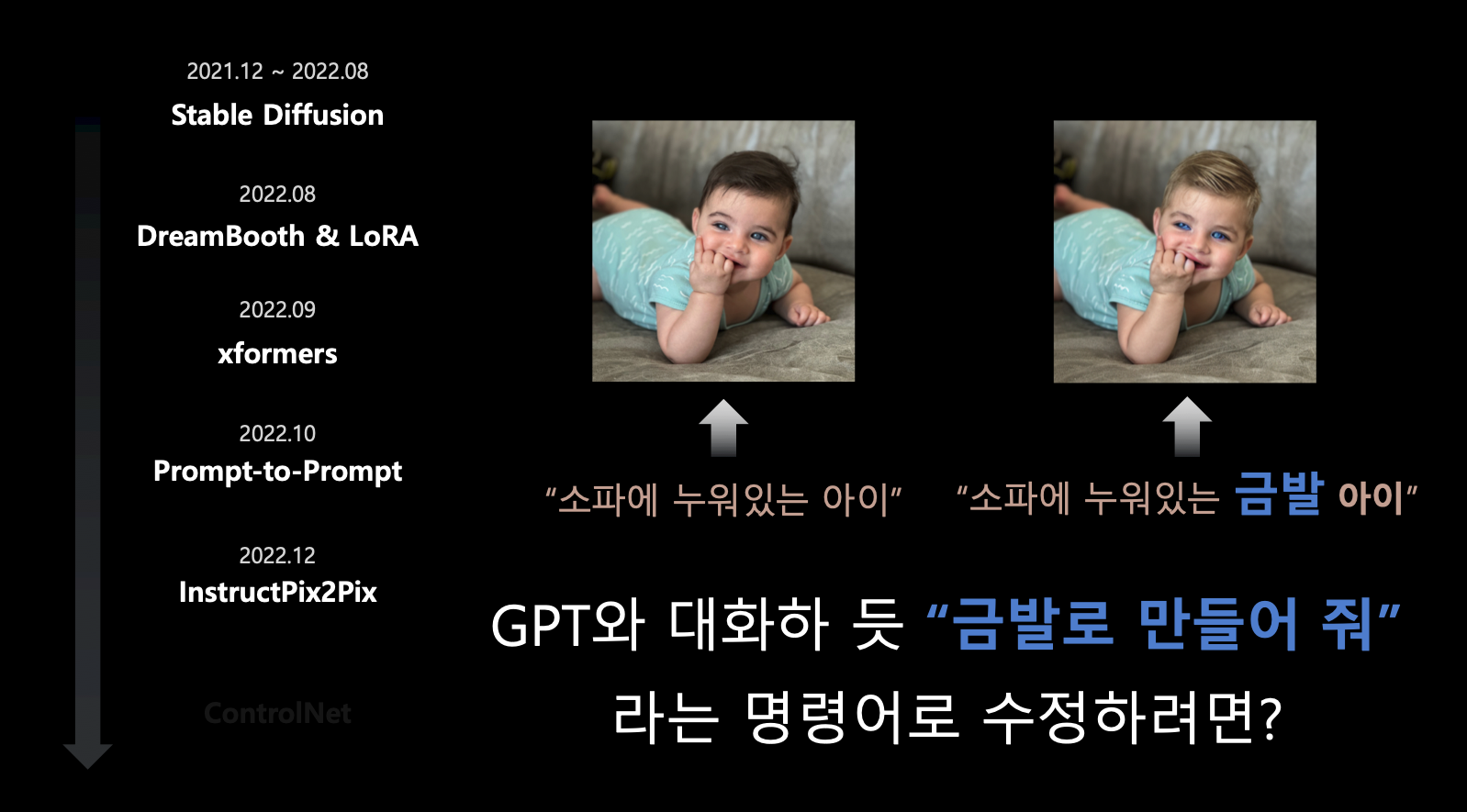
- Prompt-to-Prompt와 GPT를 함께 사용해서 대화형 프롬프트로 이미지 수정을 구현함.
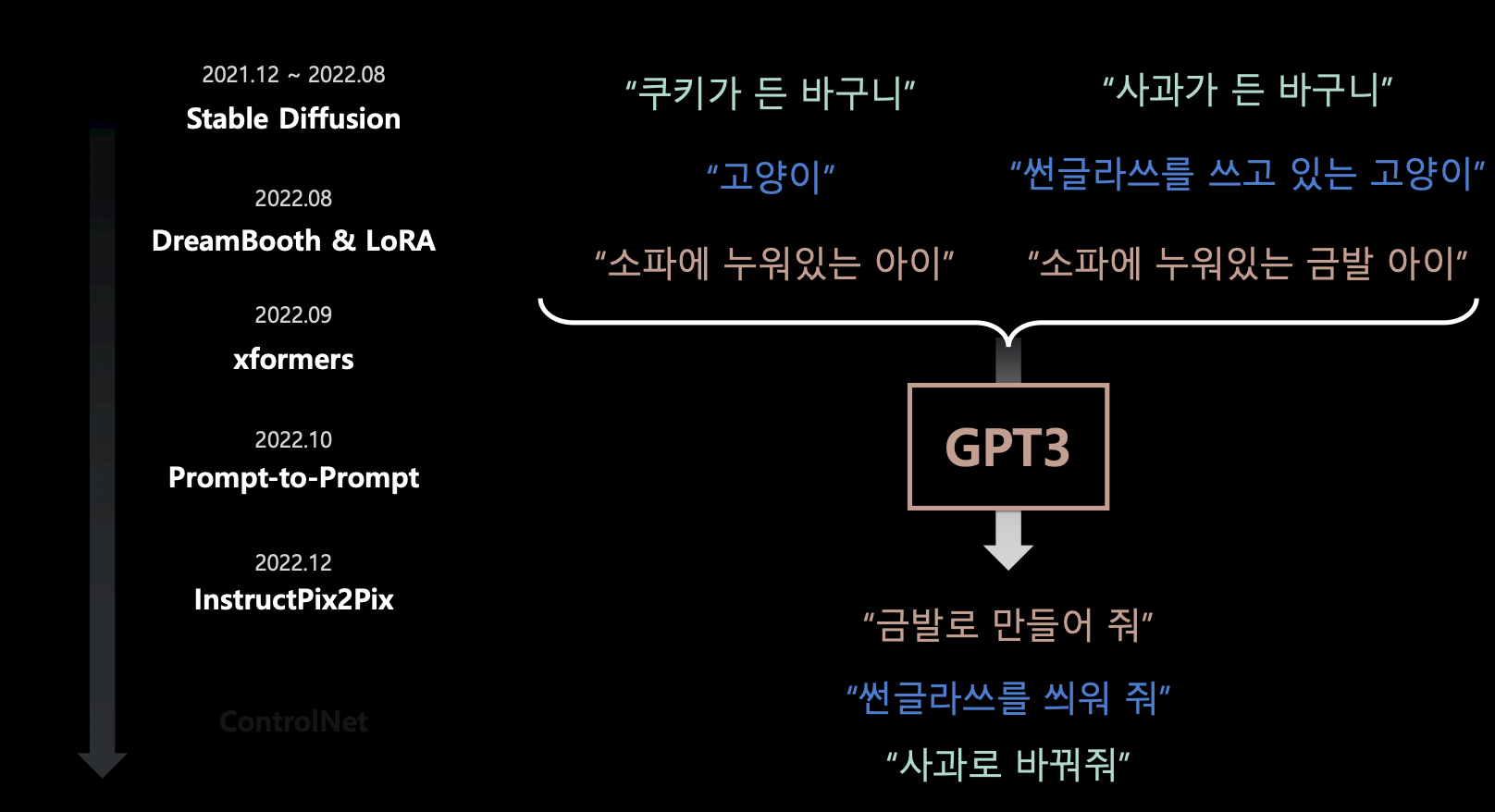
- 즉, 인풋 이미지들과 이 이미지를 기반으로 Prompt-to-Prompt로 수정된 이미지들을 비교하면서 나타난 차이점들을 텍스트 데이터를 만들어 GPT3 프롬프트 명령어로 학습시킴. 이로 인해, 사용자는 GPT3 프롬프트 명령어를 인풋으로 주면 프롬프트 명령어에 따라 기존 이미지에 대한 원하는 수정을 할 수 있음.
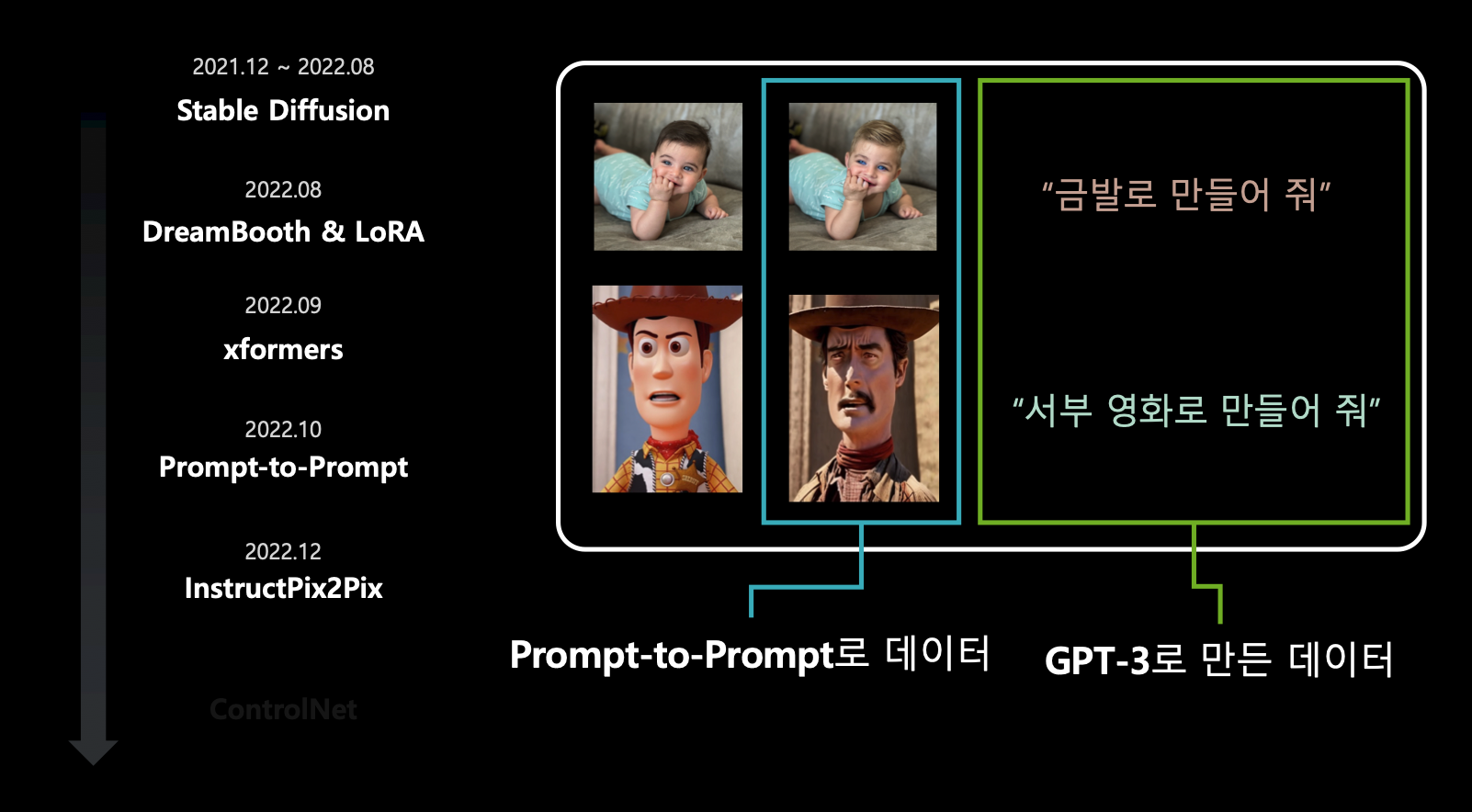
- 다시 말해, 학습 데이터는 기존 이미지, Prompt-to-Prompt 데이터, GPT-3 데이터 세 가지.
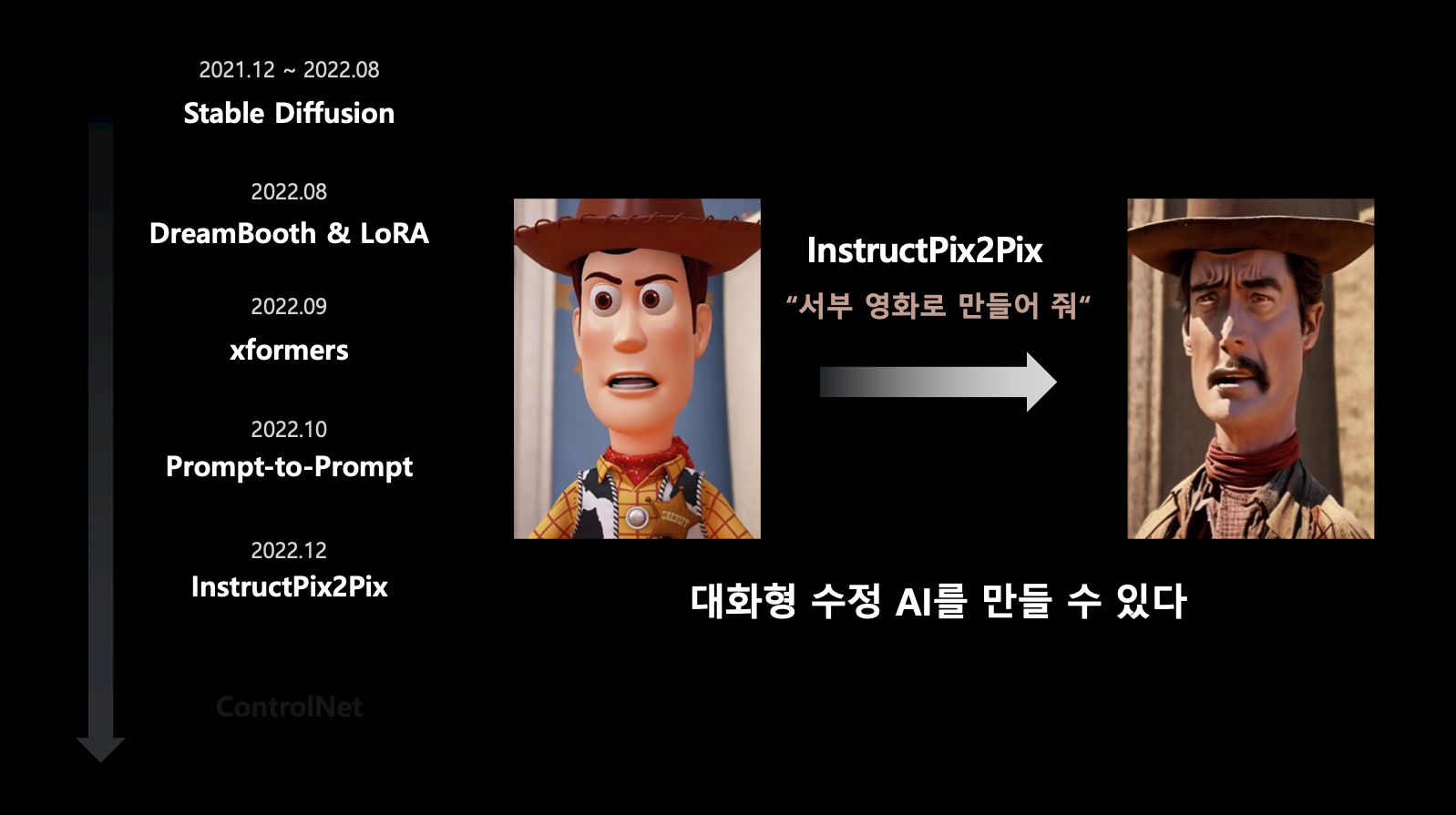
ControlNet
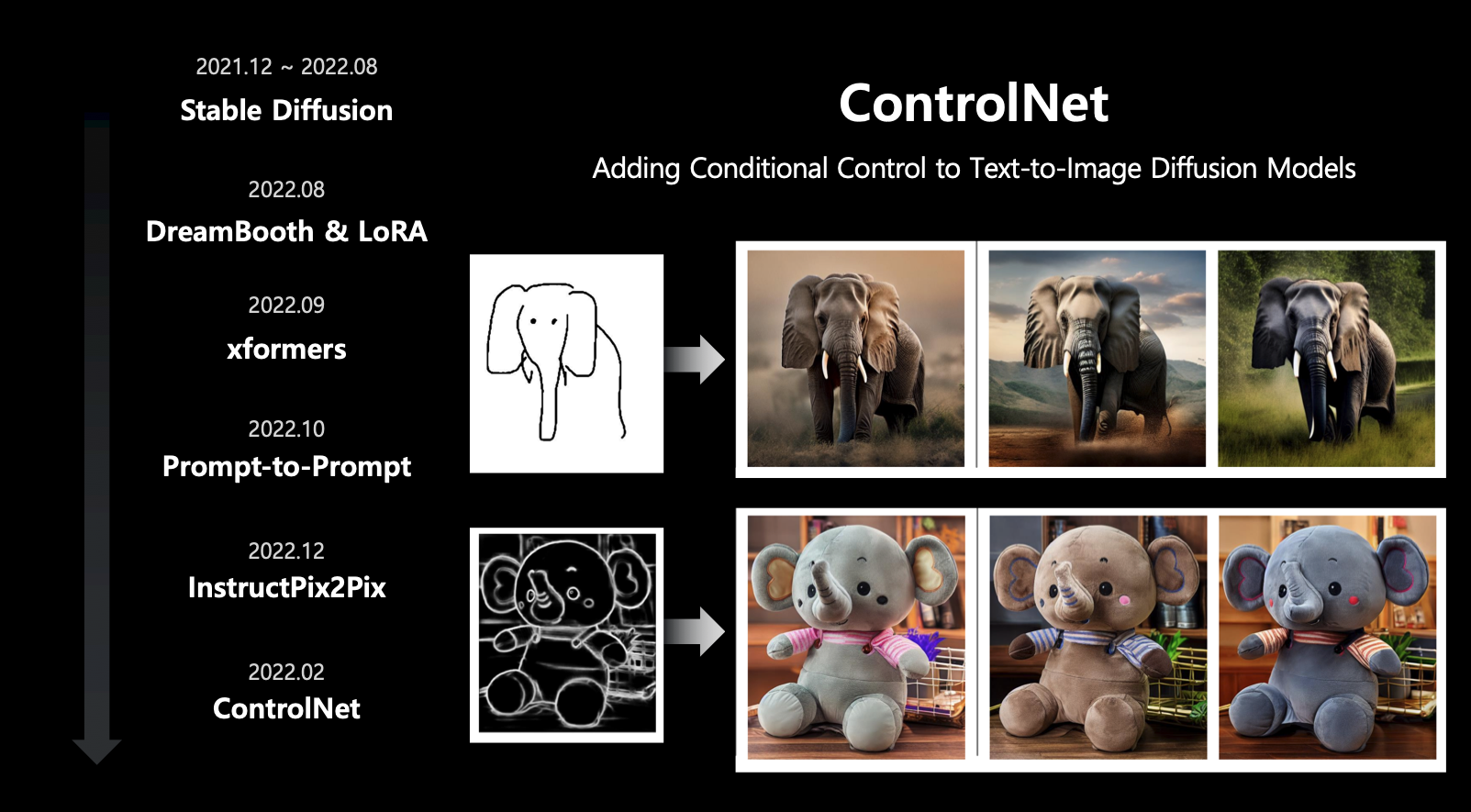
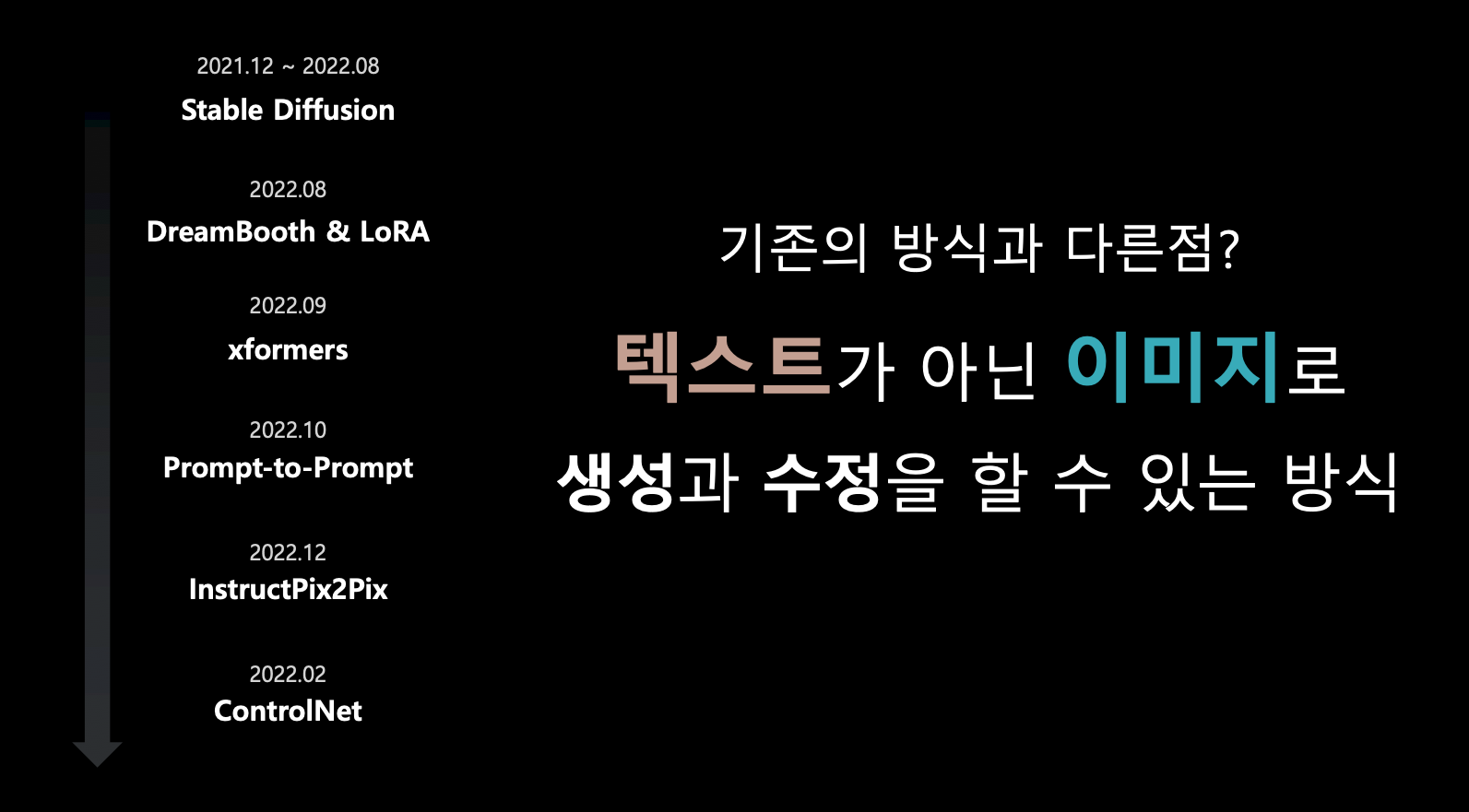
- ControlNetd은 기존 텍스트 기반 이미지 생성이 아니라 레퍼런스 이미지 기반으로 이미지 생성하는 기술.
지난 6개월 간 diffusion model의 발전
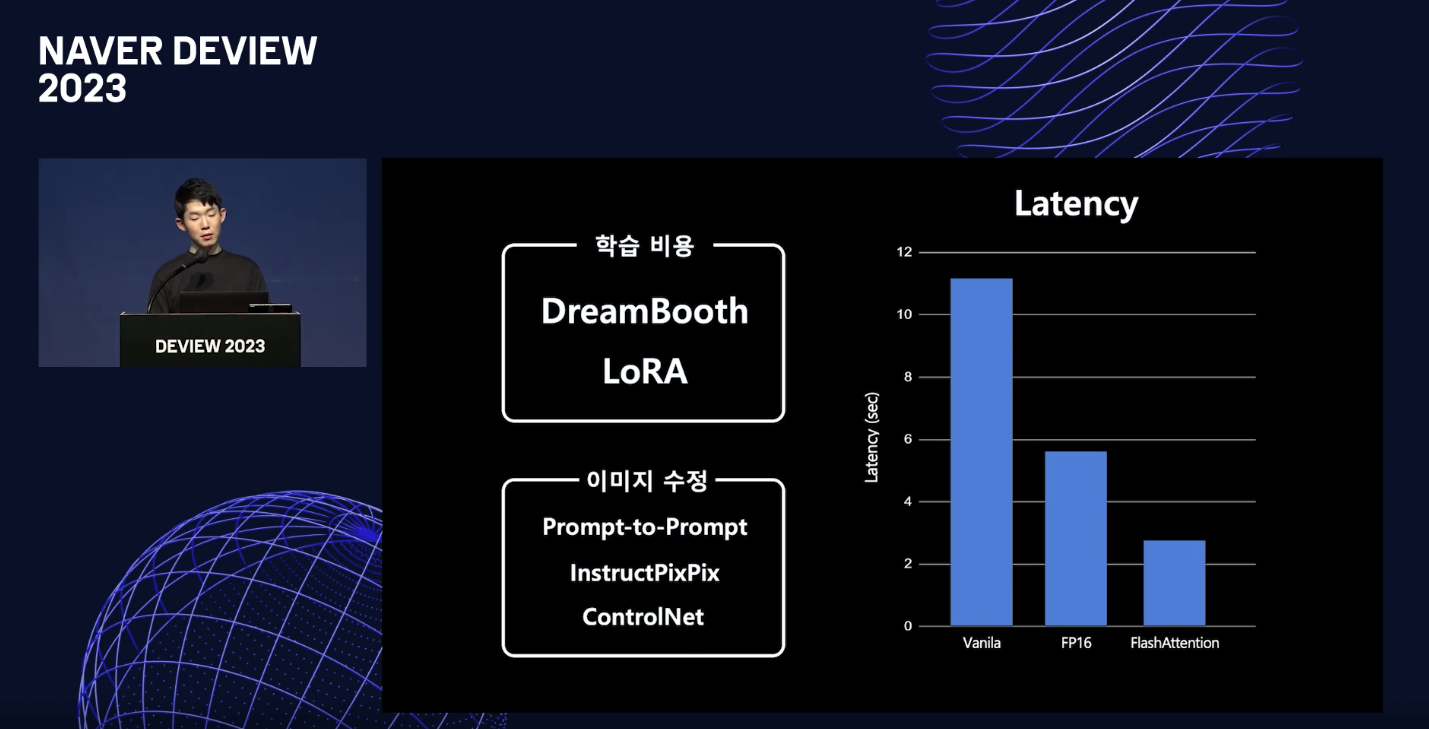
GPU 비용에 대해
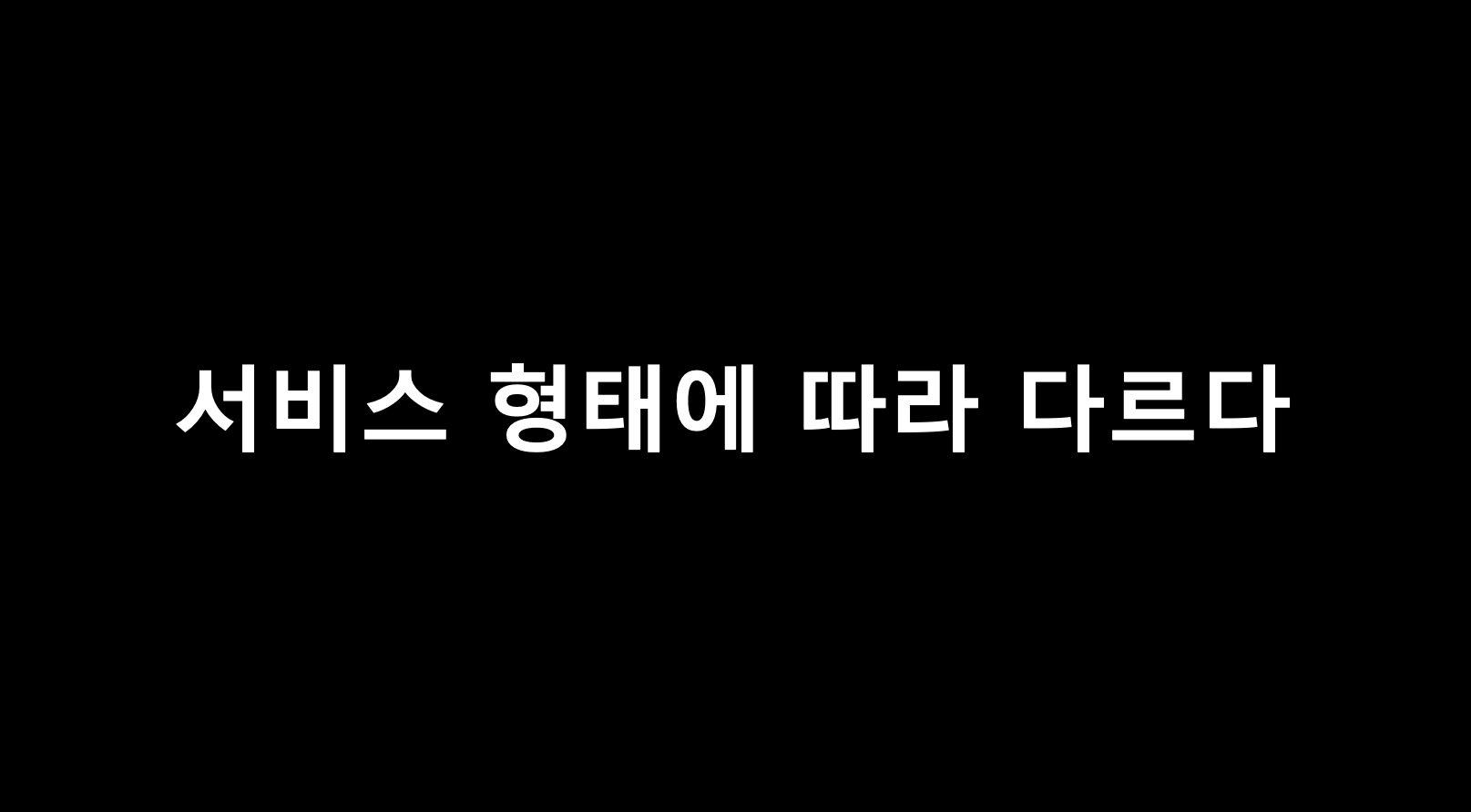
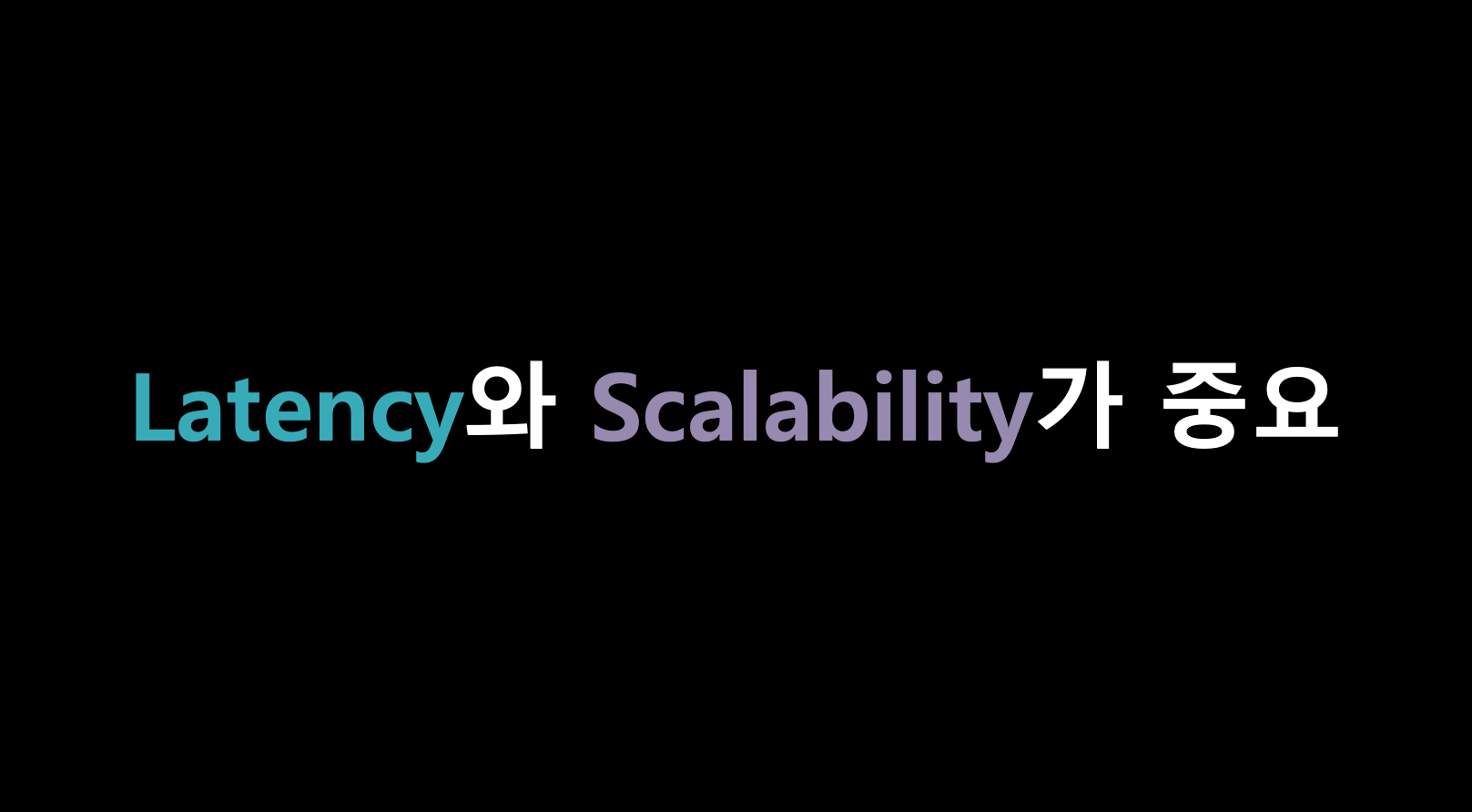
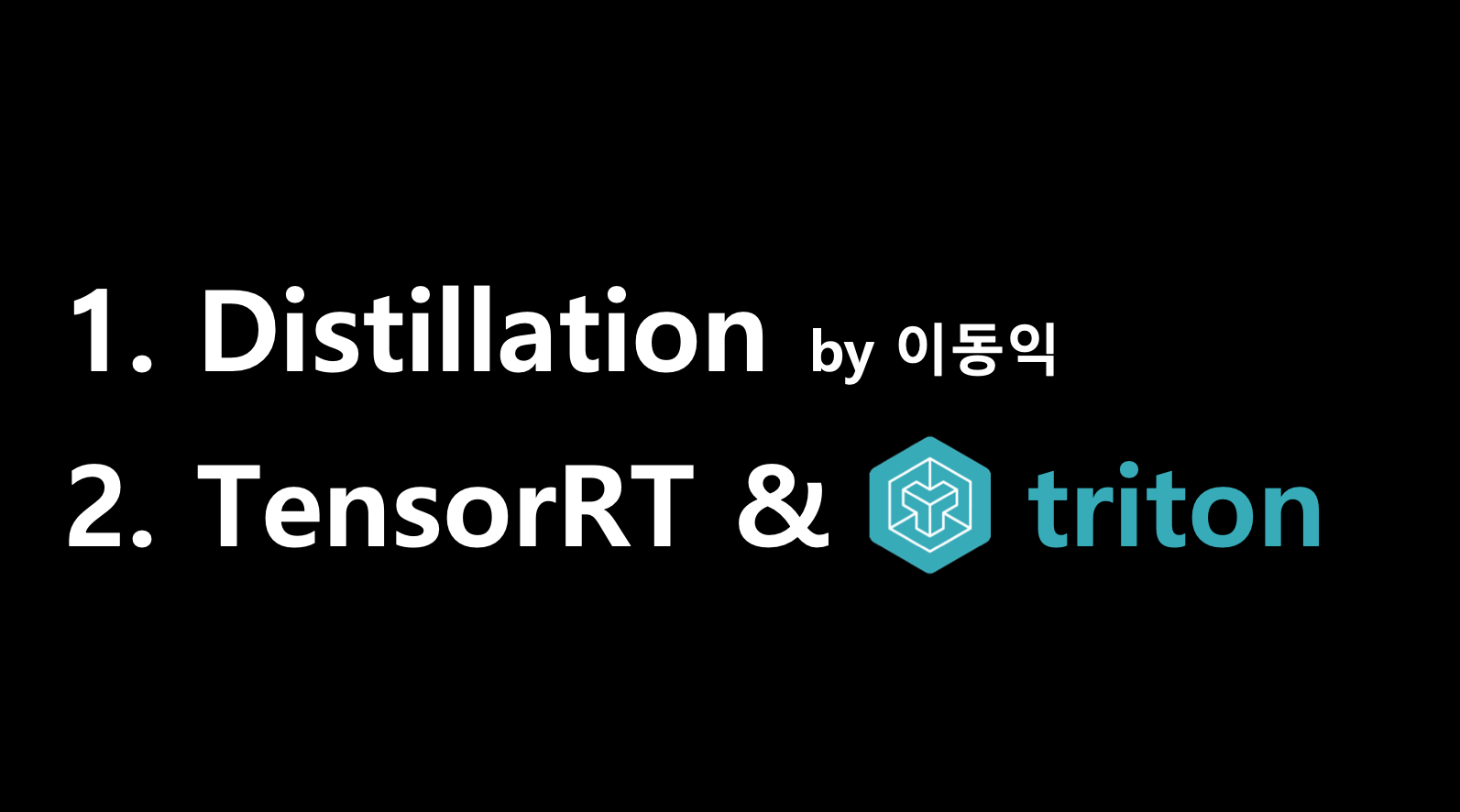
- latency와 scalability를 높이기 위해 distillation과 tensorRT&triton을 활용.
Distillation
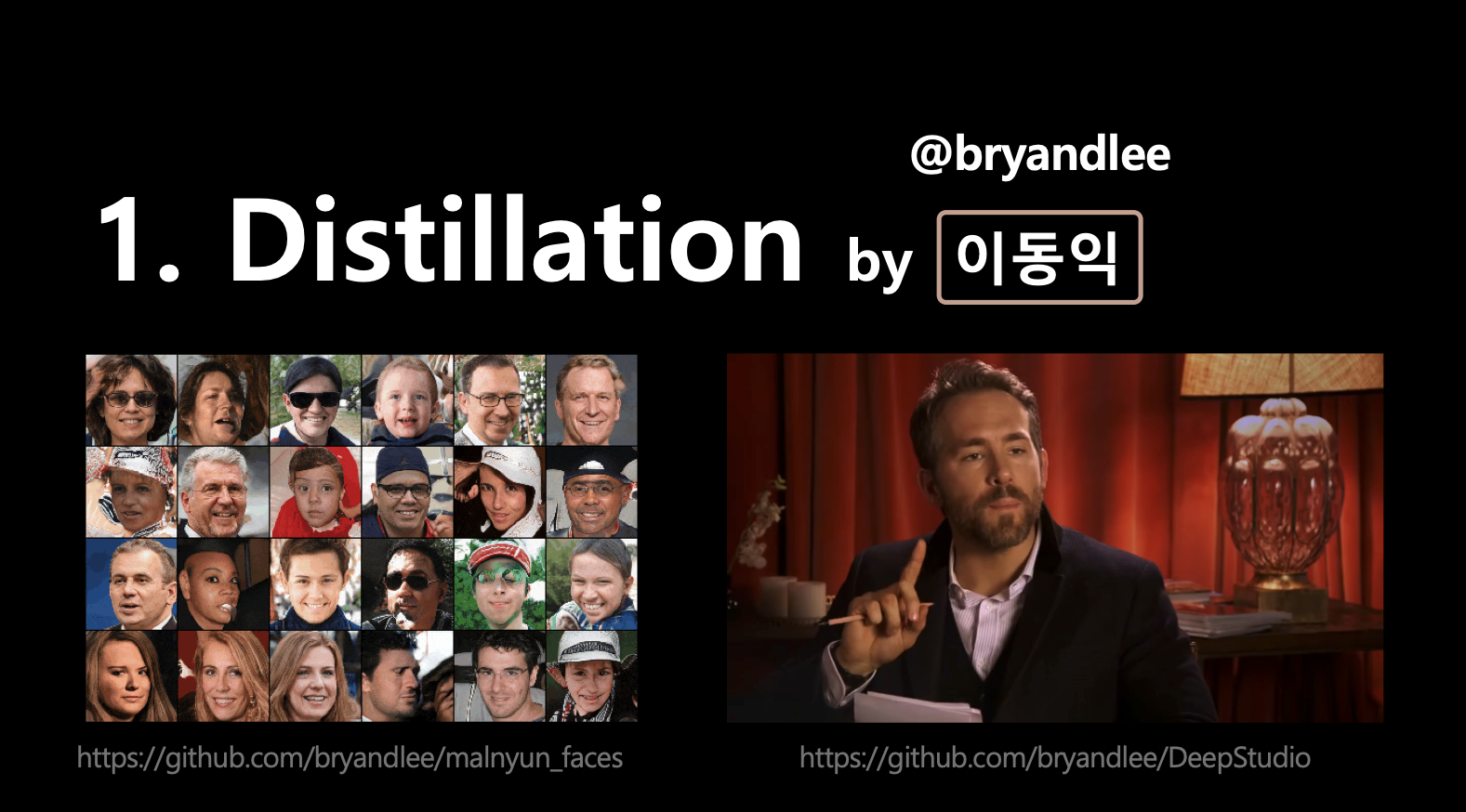
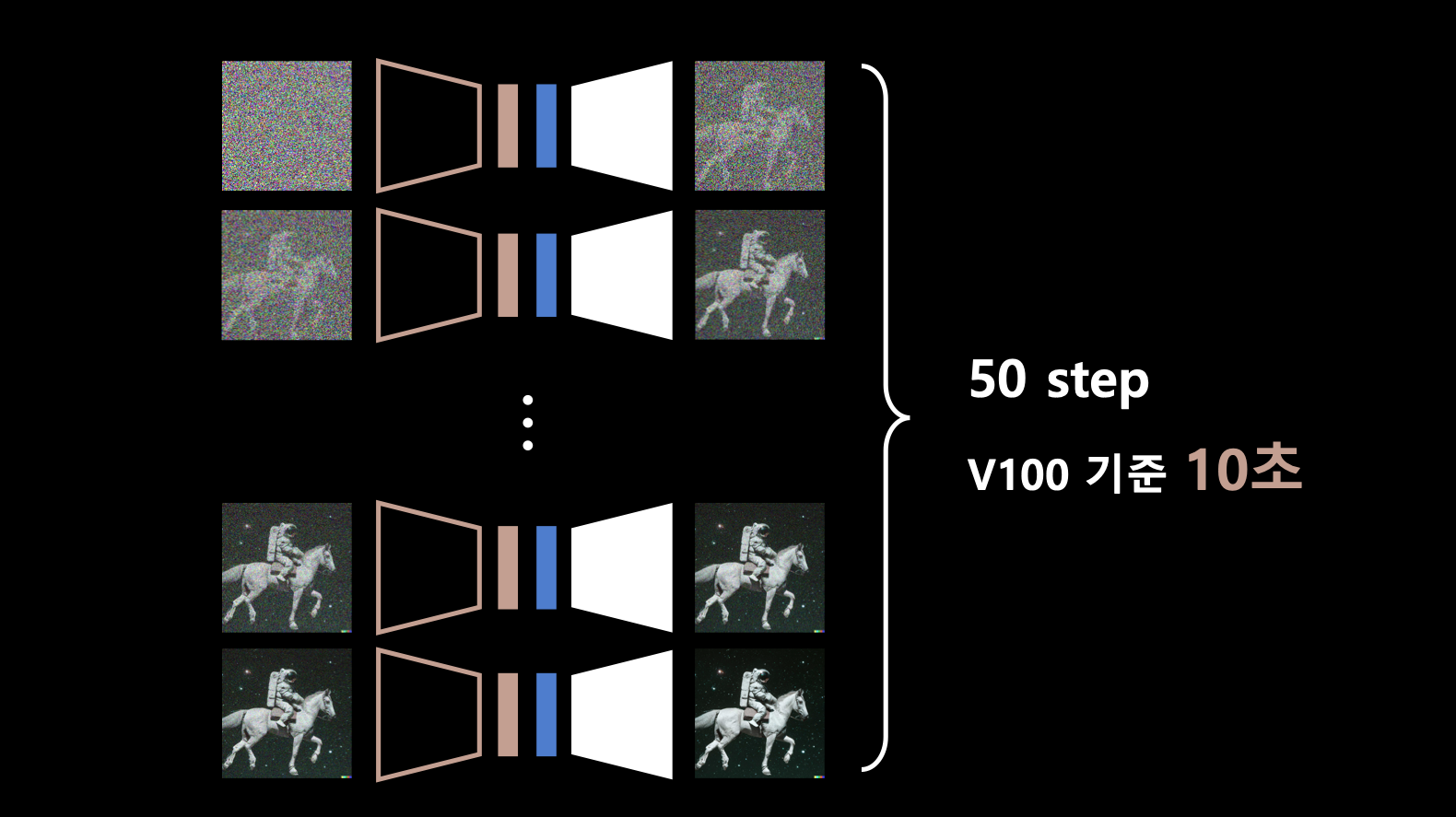
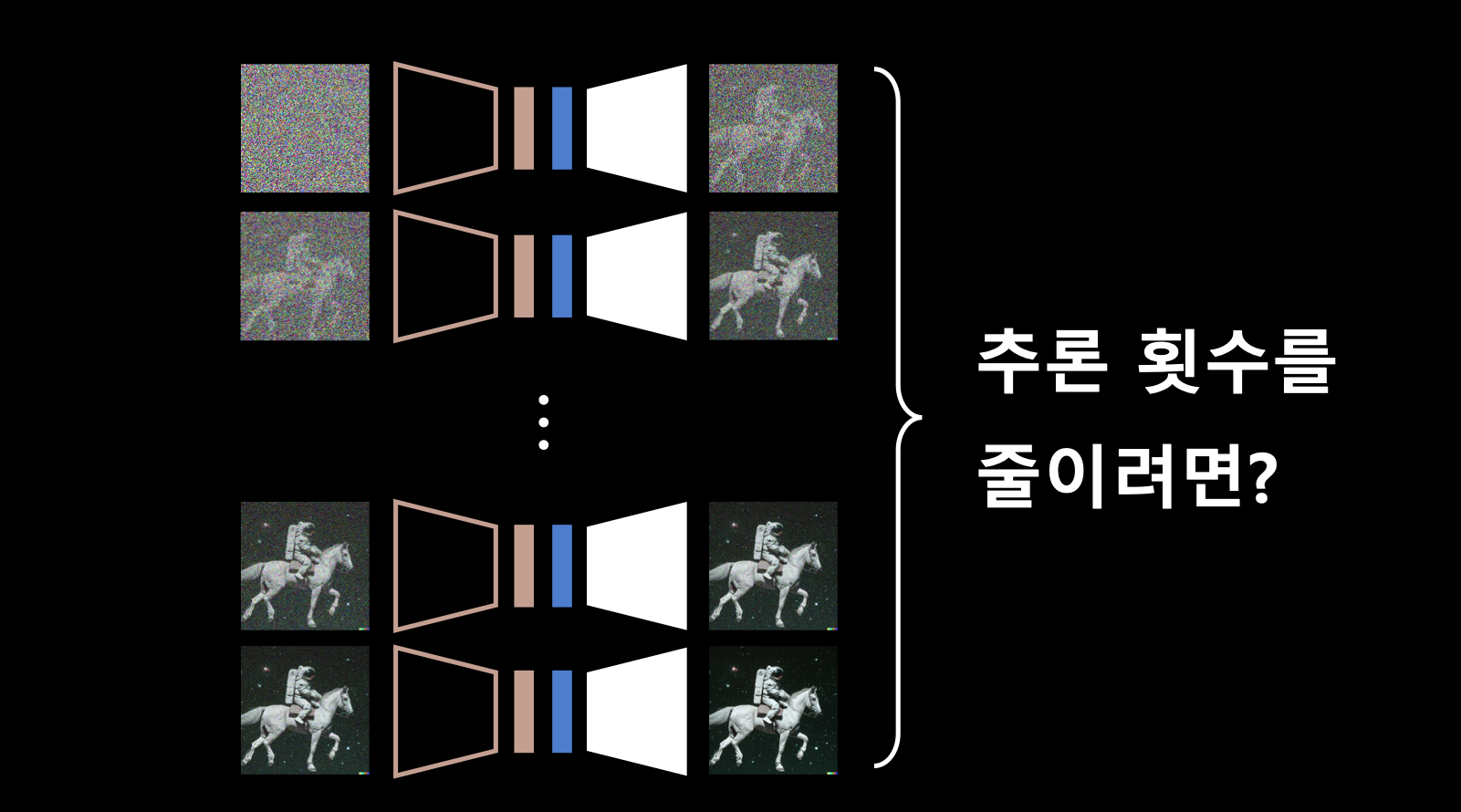
- 모델의 추론 속도를 줄이기 위해 distillation을 활용할 수 있음.
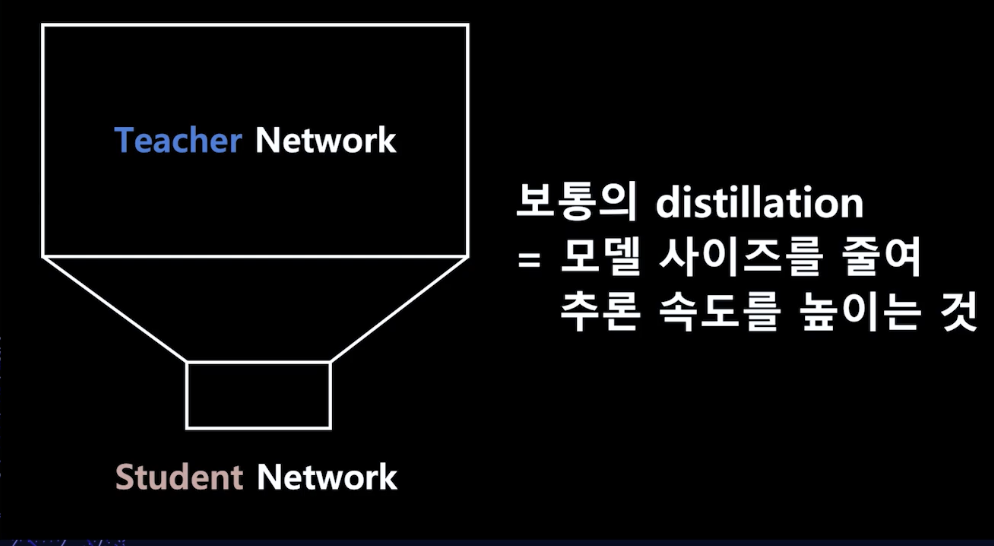
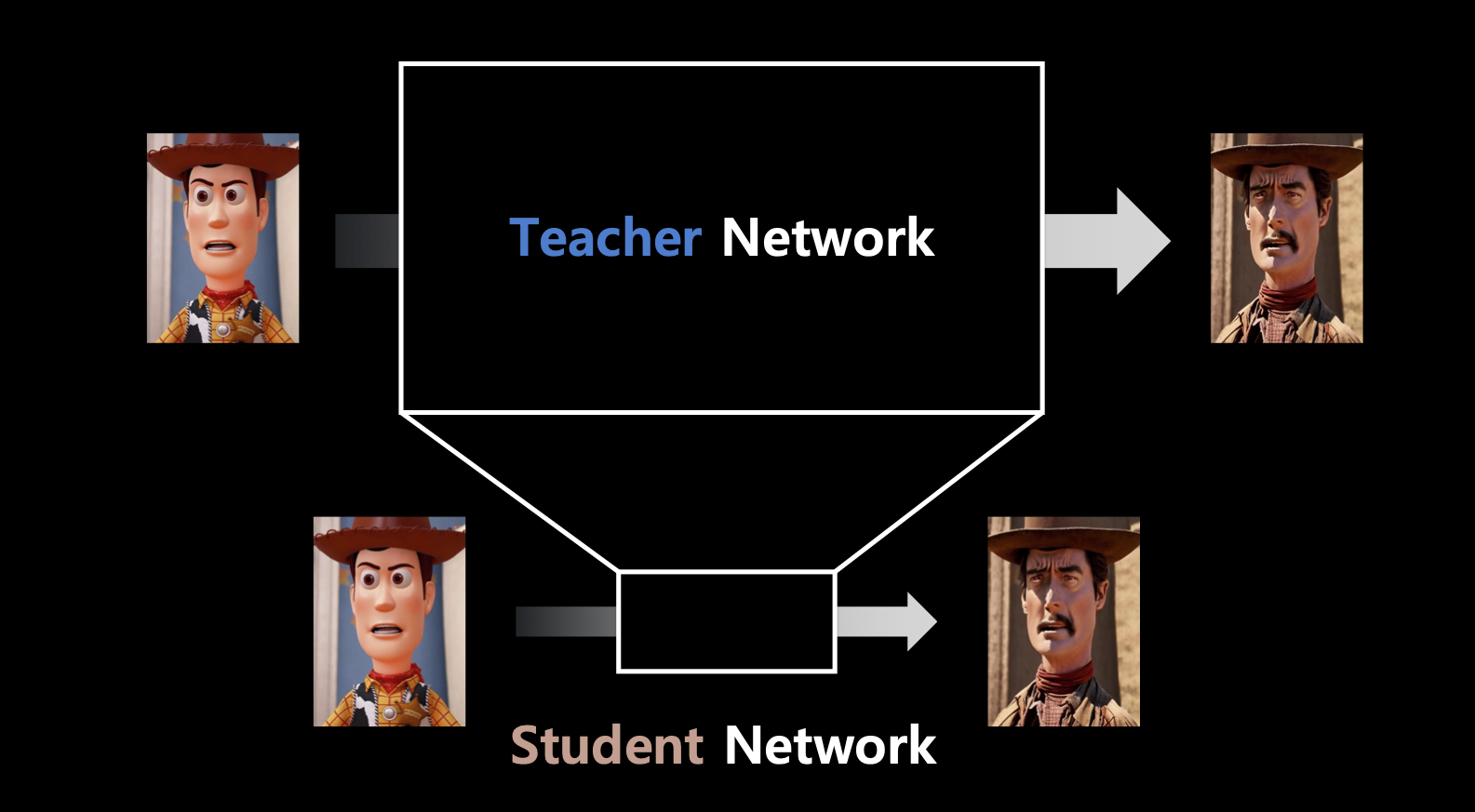
- 기존의 distillation은 큰 모델을 작은 모델로 사이즈를 줄여서 추론 속도를 높이는 방법.
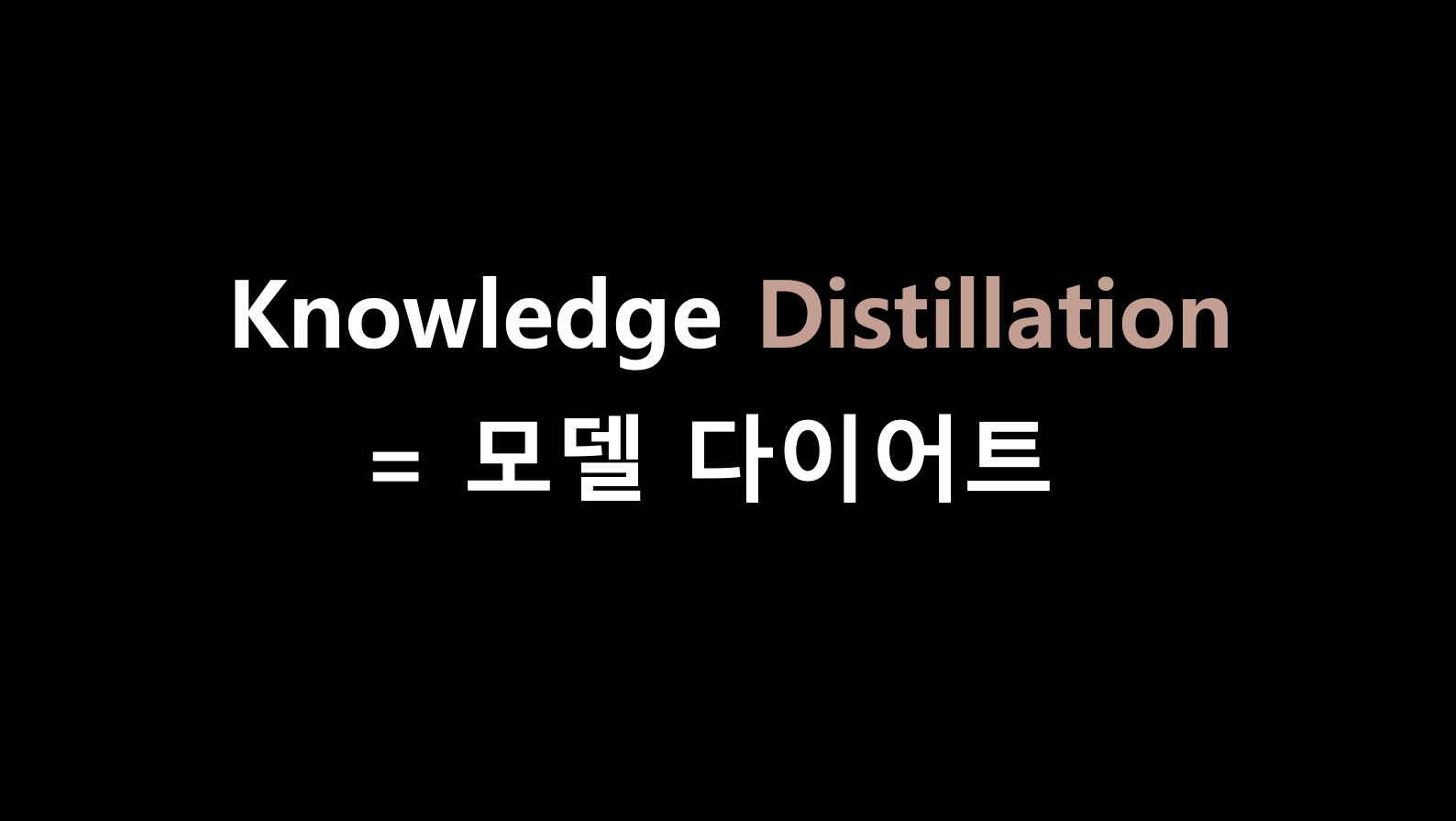
- 즉, 기존 distillation은 모델 다이어트라고 할 수 있음.
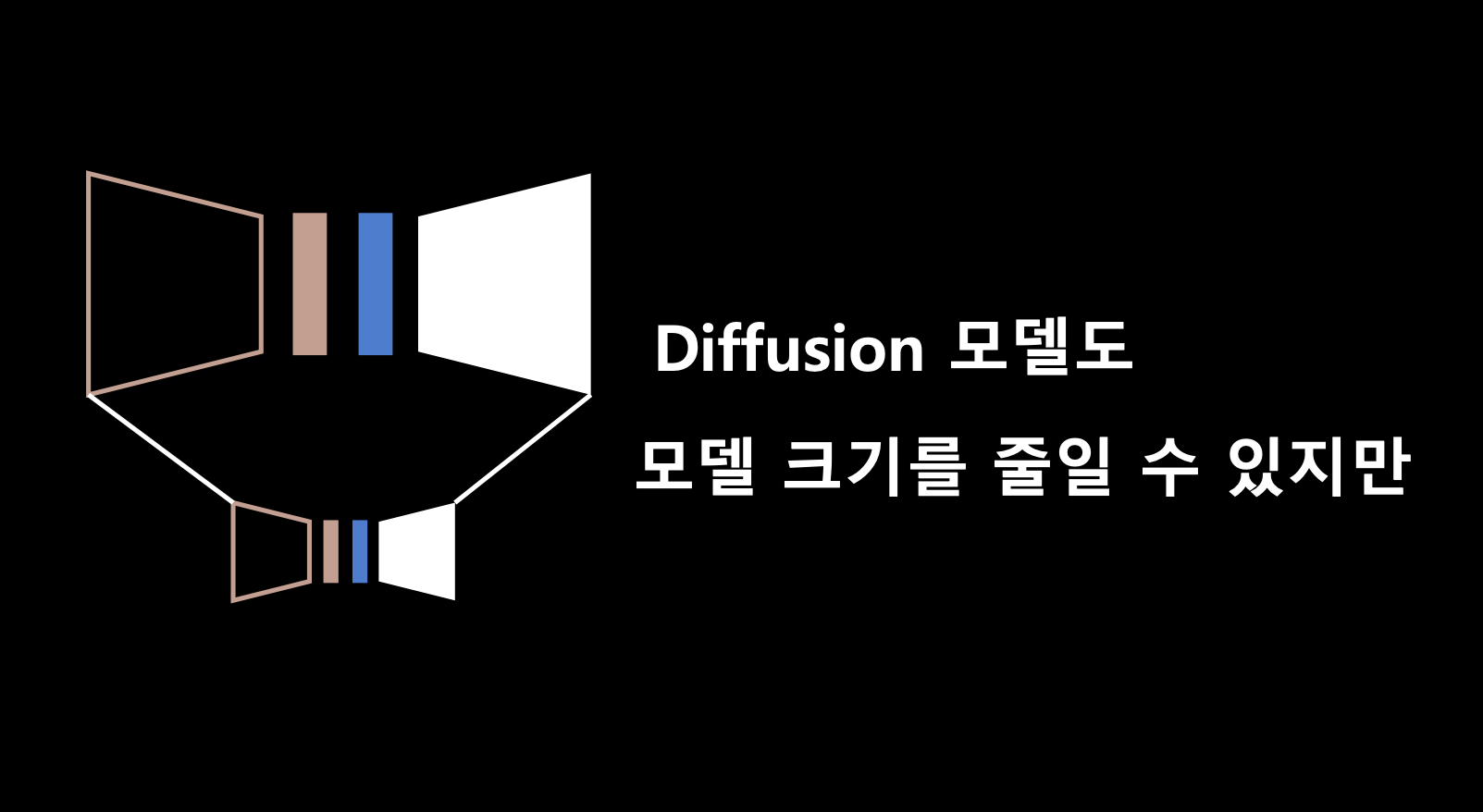
- diffusion model도 모델 사이즈를 줄이는 distillation을 할 수 있었지만 우리가 추론 결과로 받을 이미지가 만족스럽지 못할 가능성이 있기 떄문에 다른 방법을 시도함.
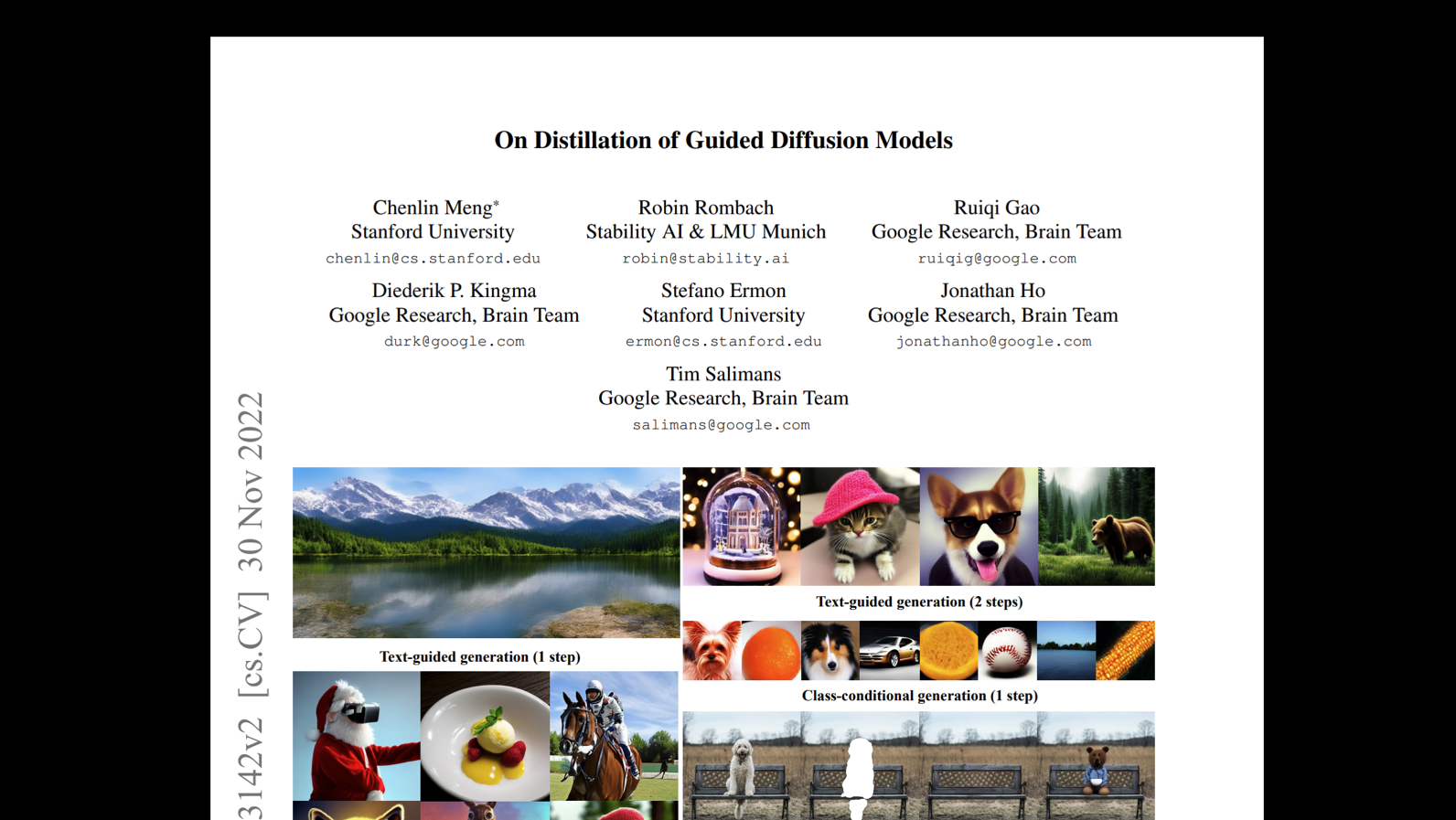
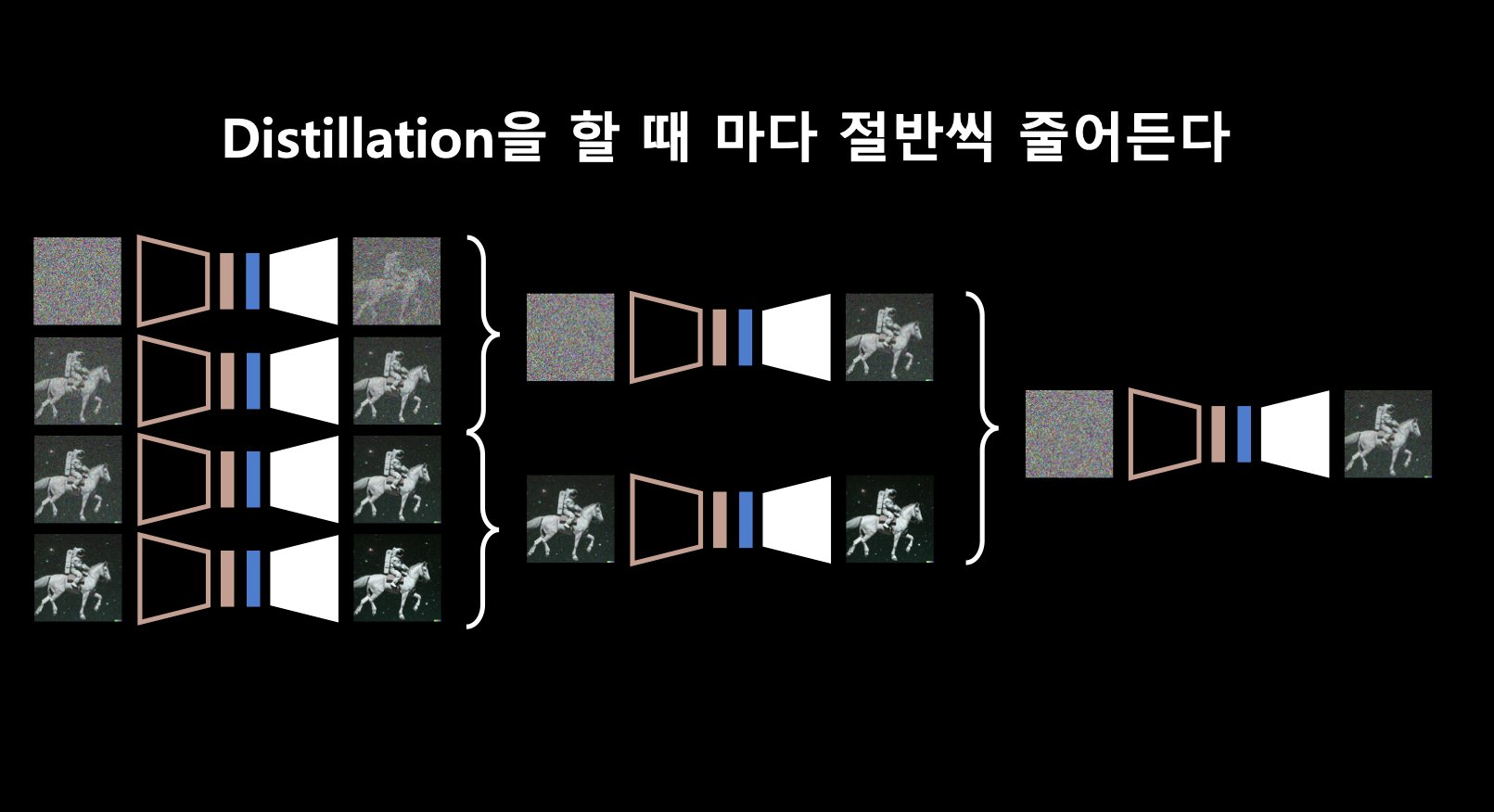
- 그래서 diffusion process의 step을 2n 으로 줄일 수 있도록 재학습 시키는 방법을 시도함. (논문)
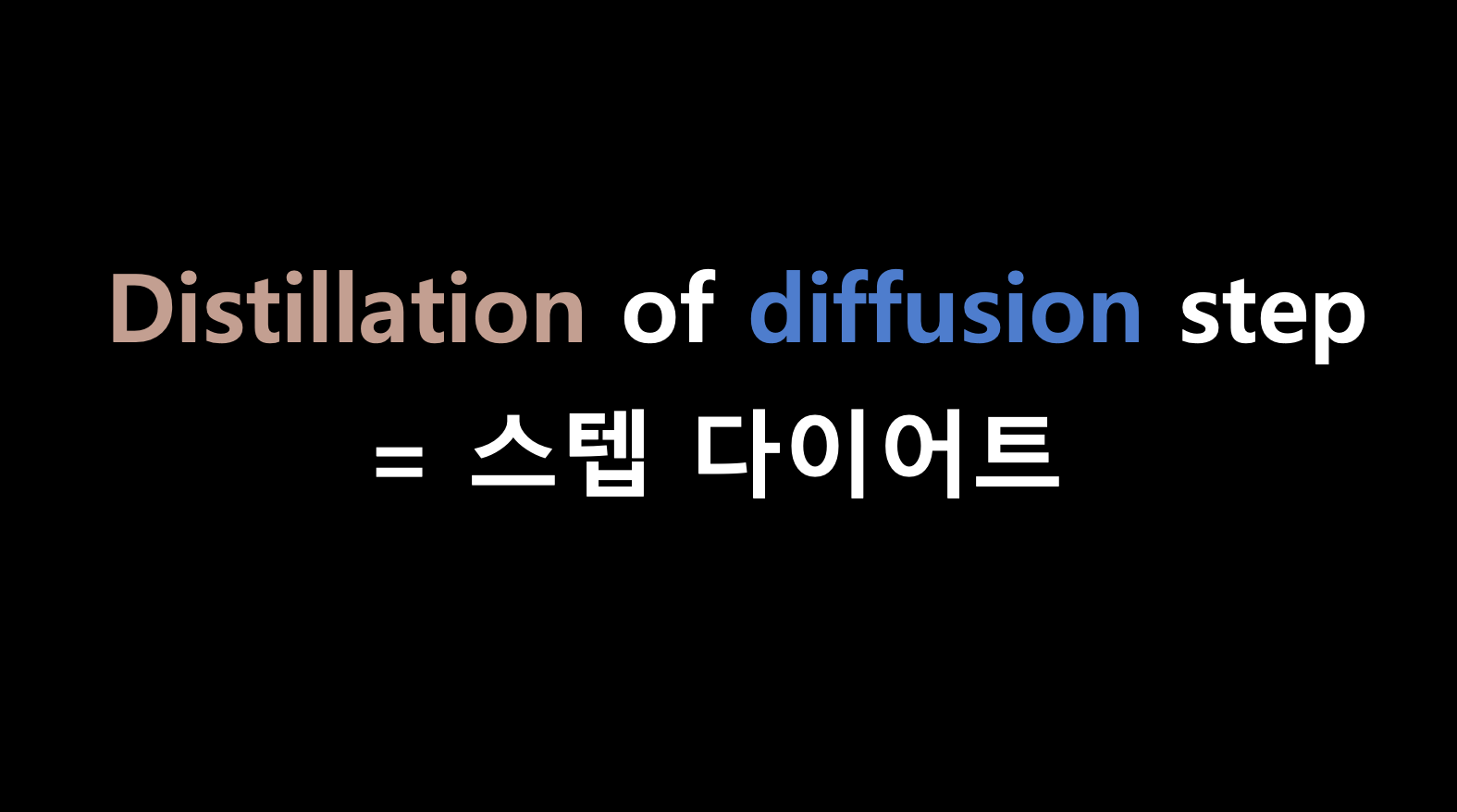
- 즉, diffusion step에 대한 distillation이므로 스텝 다이어트라고 할 수 있음.
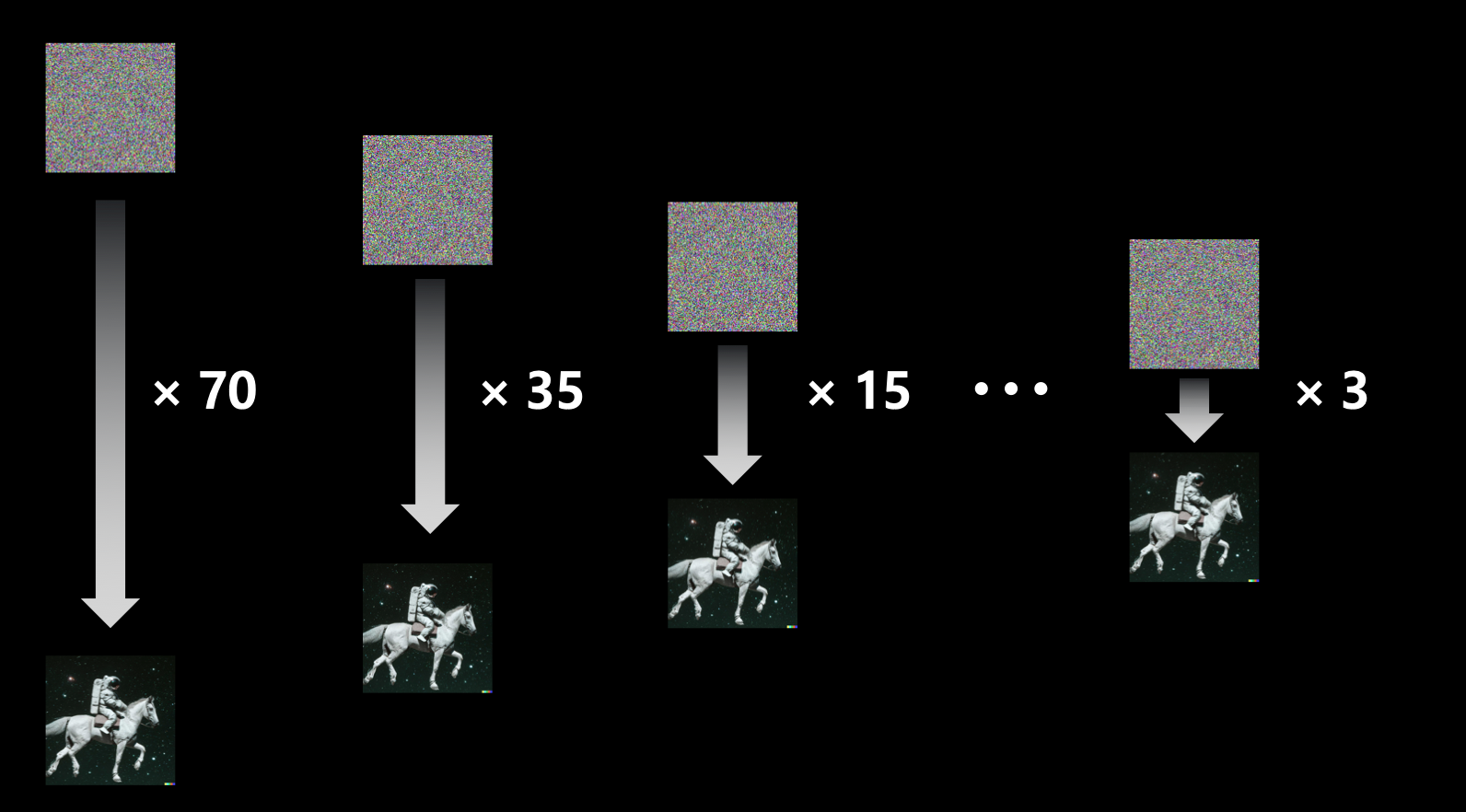
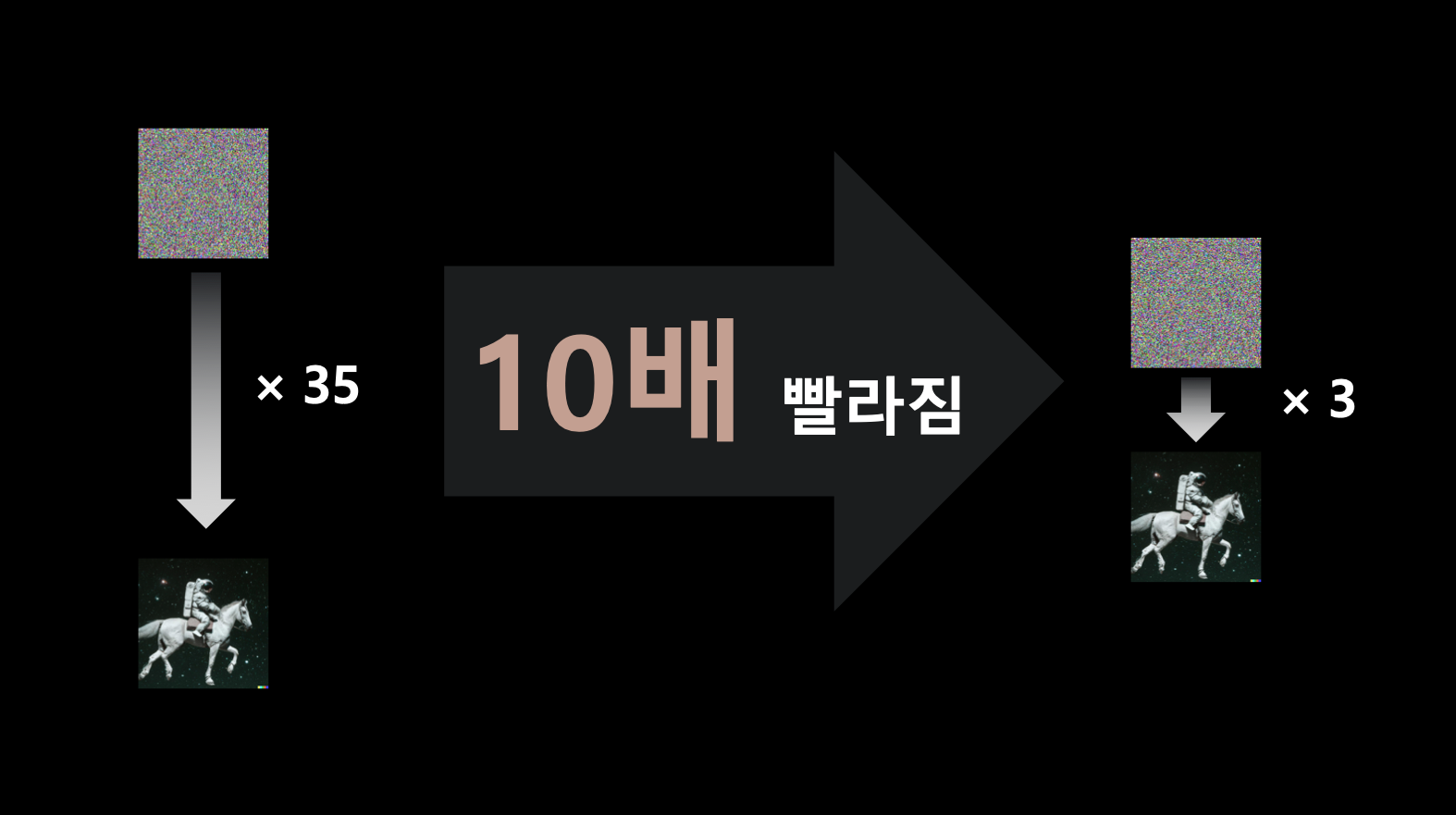
실험 결과
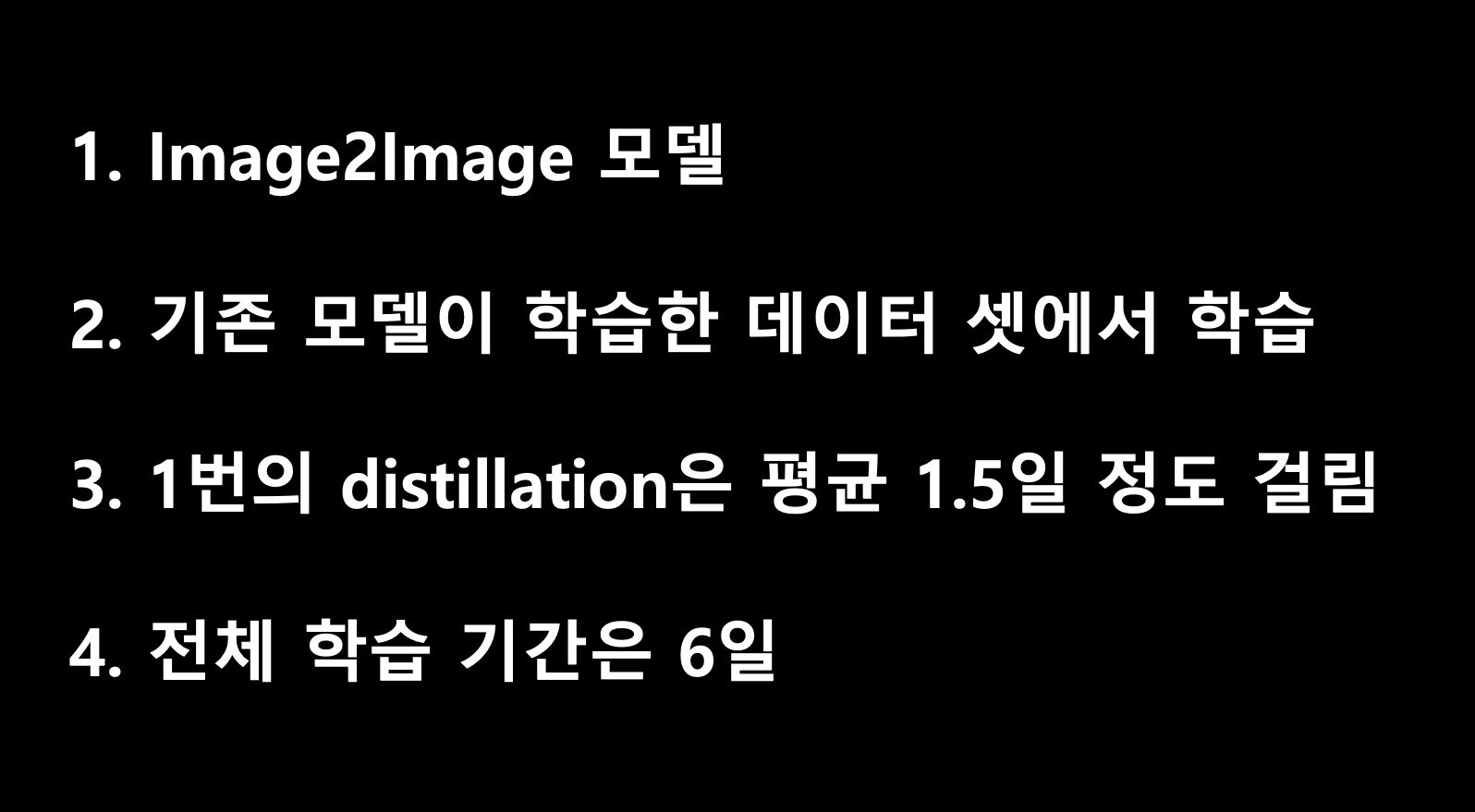
- Image2Image는 이미지를 넣어 다르게 바꿔주는 모델.
- 6번 정도의 distillation을 했다고 함.
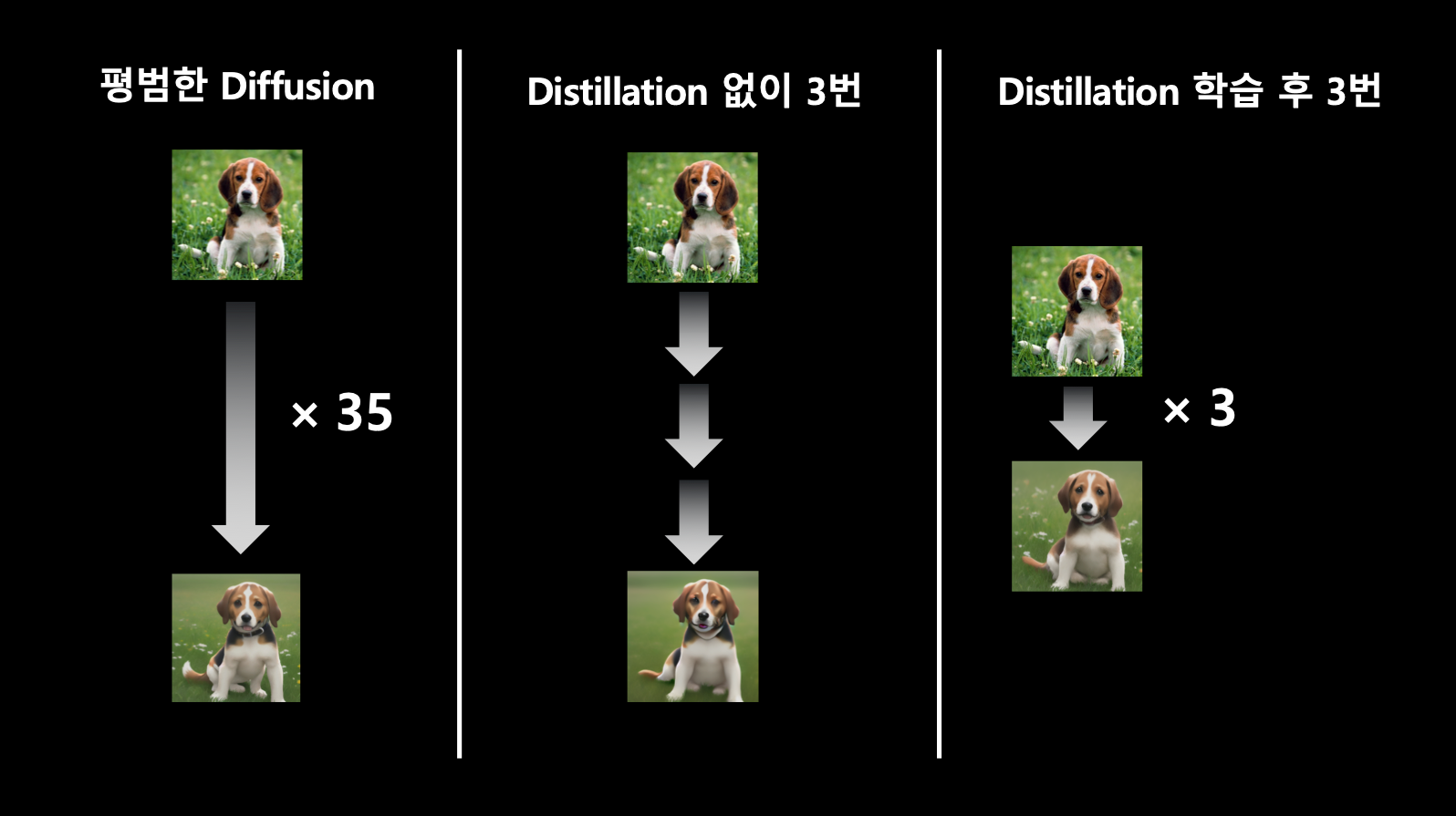
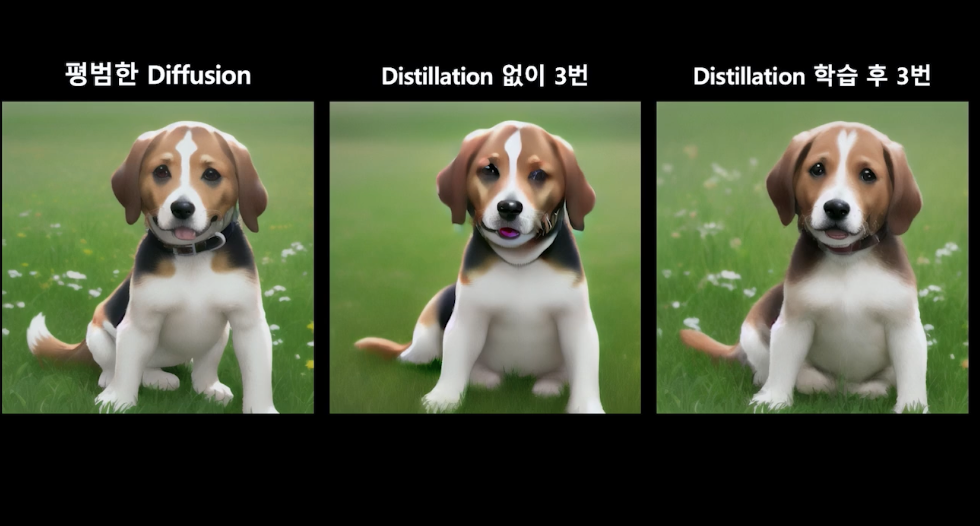
- 현실의 사진을 애니메이션으로 바꿔주는 모델에 적용했을 때.
- 제일 왼쪽과 같이 원래는 35번의 과정이 필요한데 가운데 처럼 distillation 없이 3번만 수행 후 나온 결과와 distillation 학습 후 3번 수행한 뒤 결과에 대한 차이점을 눈으로 확인할 수 있음. 즉, 같은 3번의 수행을 하더라도 distillation 학습을 한 경우가 원래 추론하는 35번의 수행과 아주 근접함.
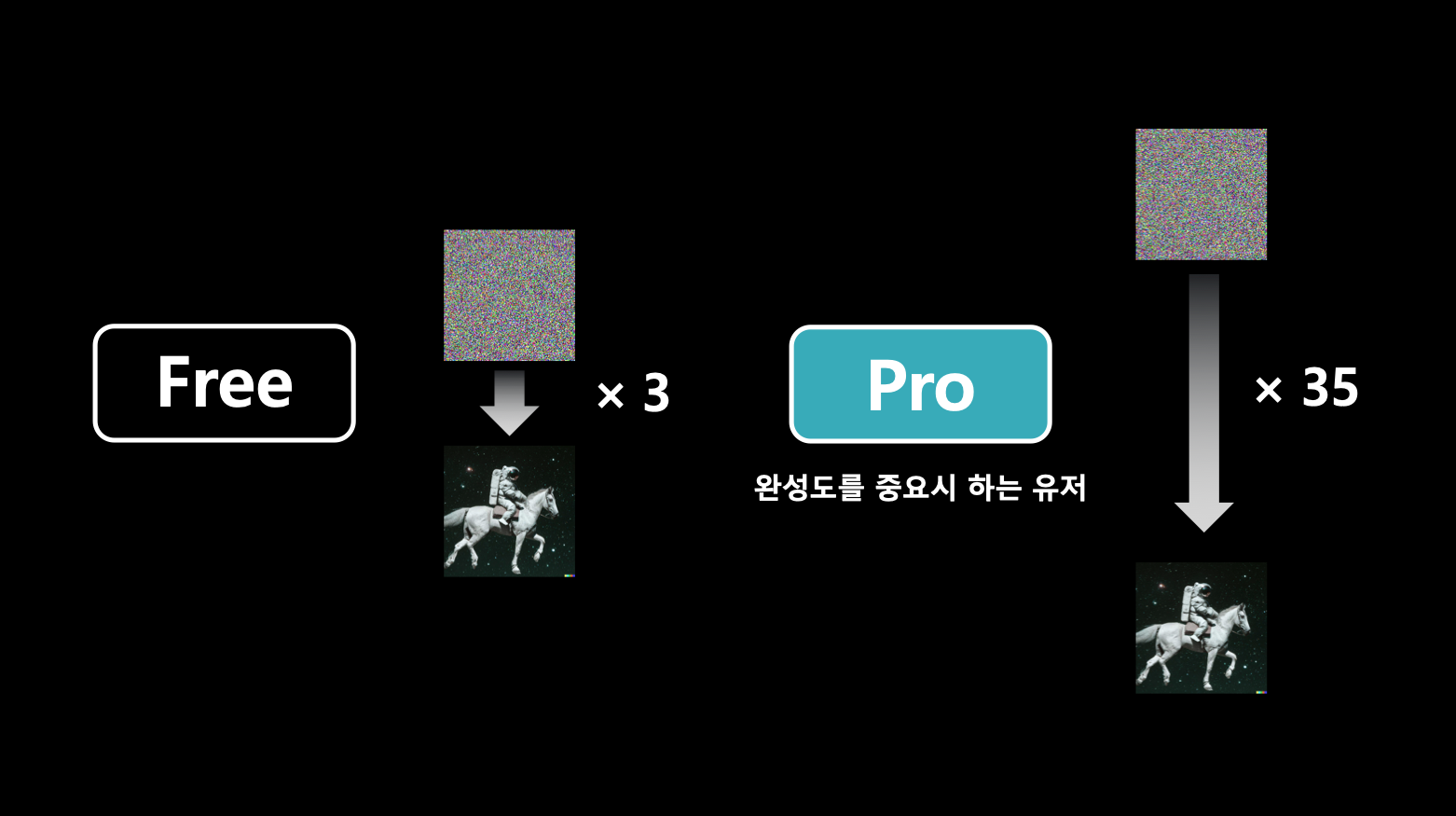
- 또한 이 회사는 추론 step 횟수에 따라 속도와 품질을 달리하여 서비스한다고 함.
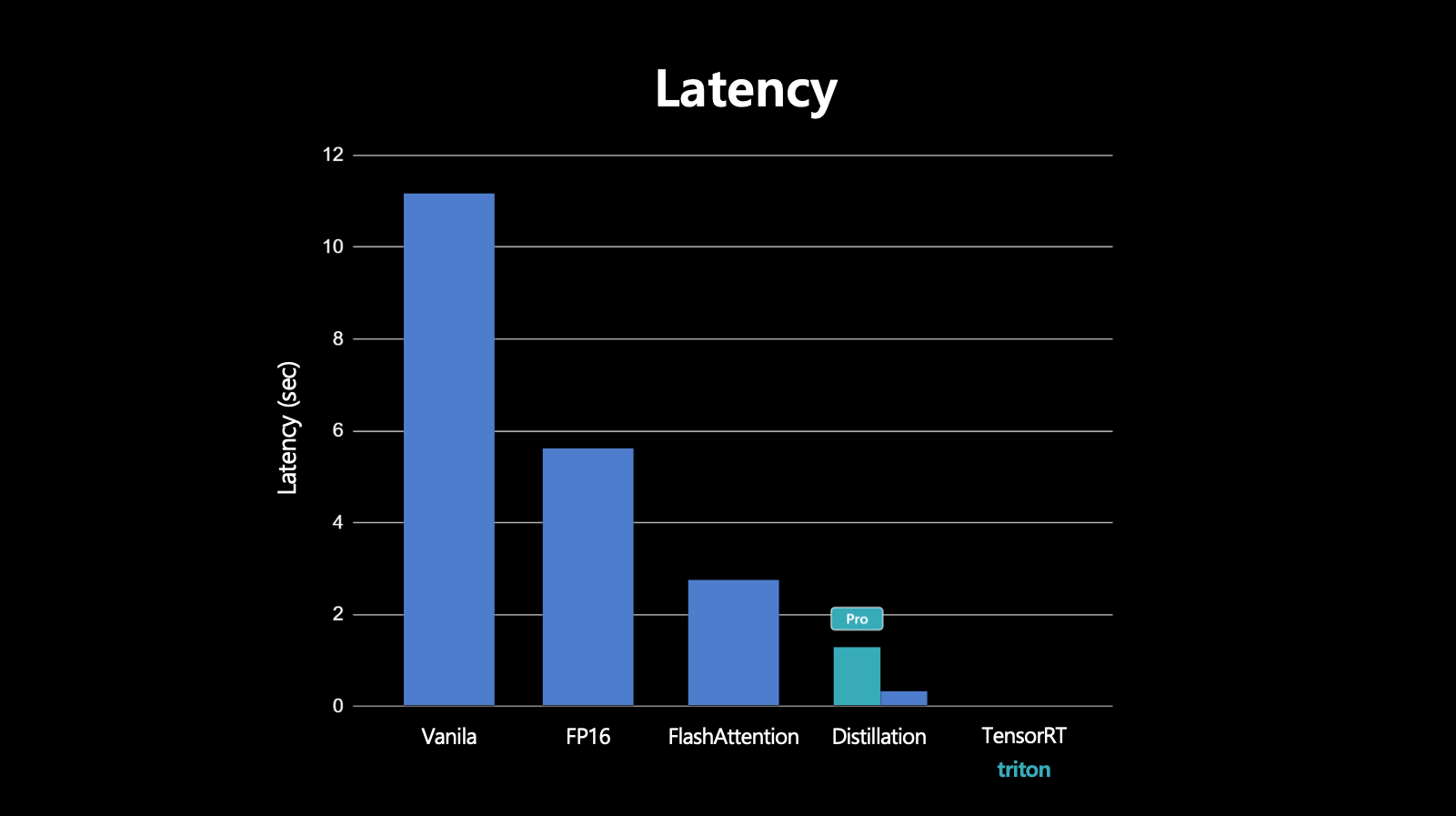
- 이렇게 distillation을 통해 latenct가 많이 줄어들었음.
TensorRT & triton
앞서 살펴본 GPU 하드웨어적인 개선 방법 FlashAttention
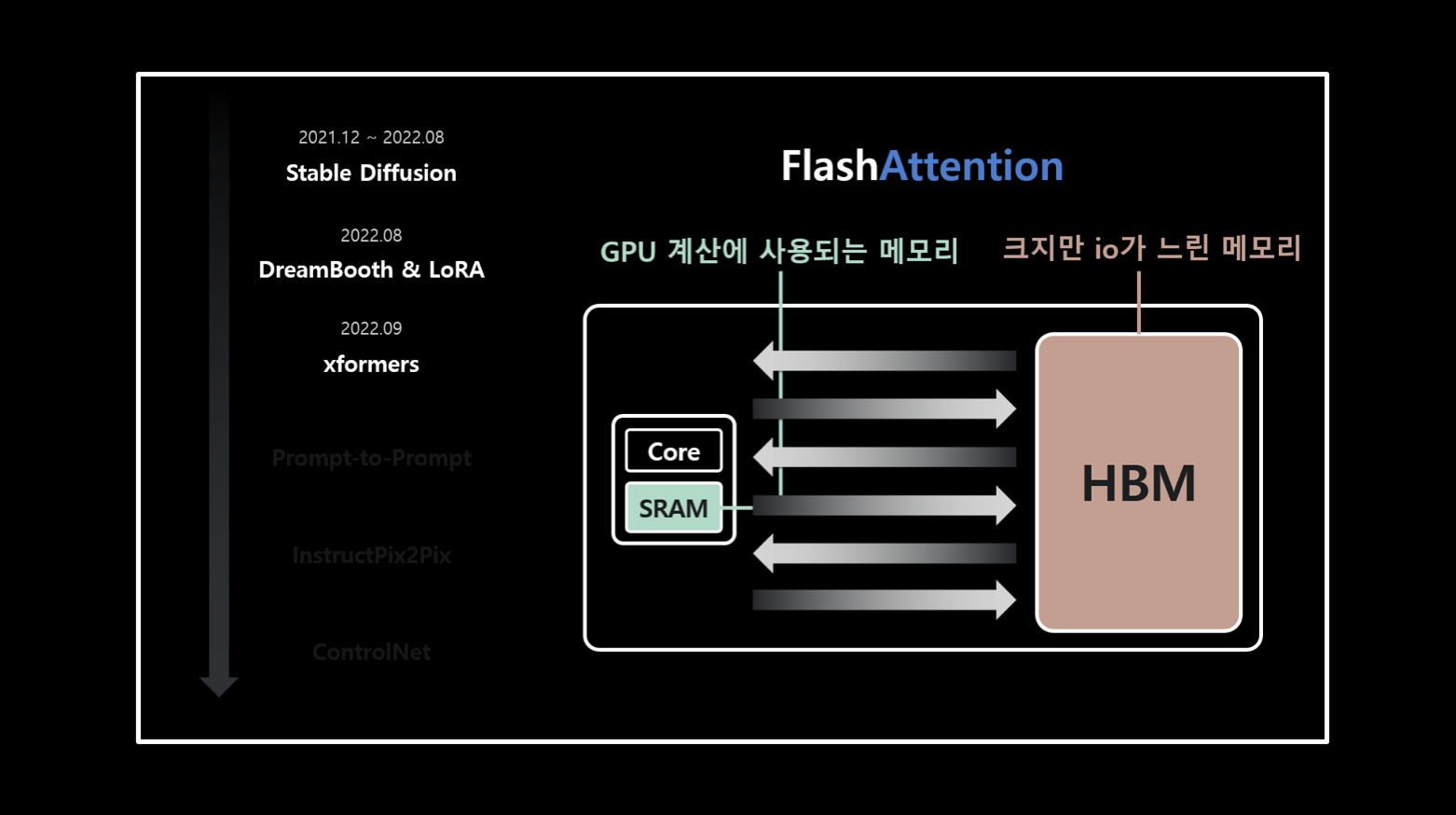
- 앞서 본 FlashAttention은 GPU 하드웨어 구조에서 발생하는 I/O를 줄여서 속도를 빠르게 한 것.
- TensorRt와 triton은 쓰는 것도 비슷하다고 함.
GPU 연산 과정과 모델 학습에서의 장점
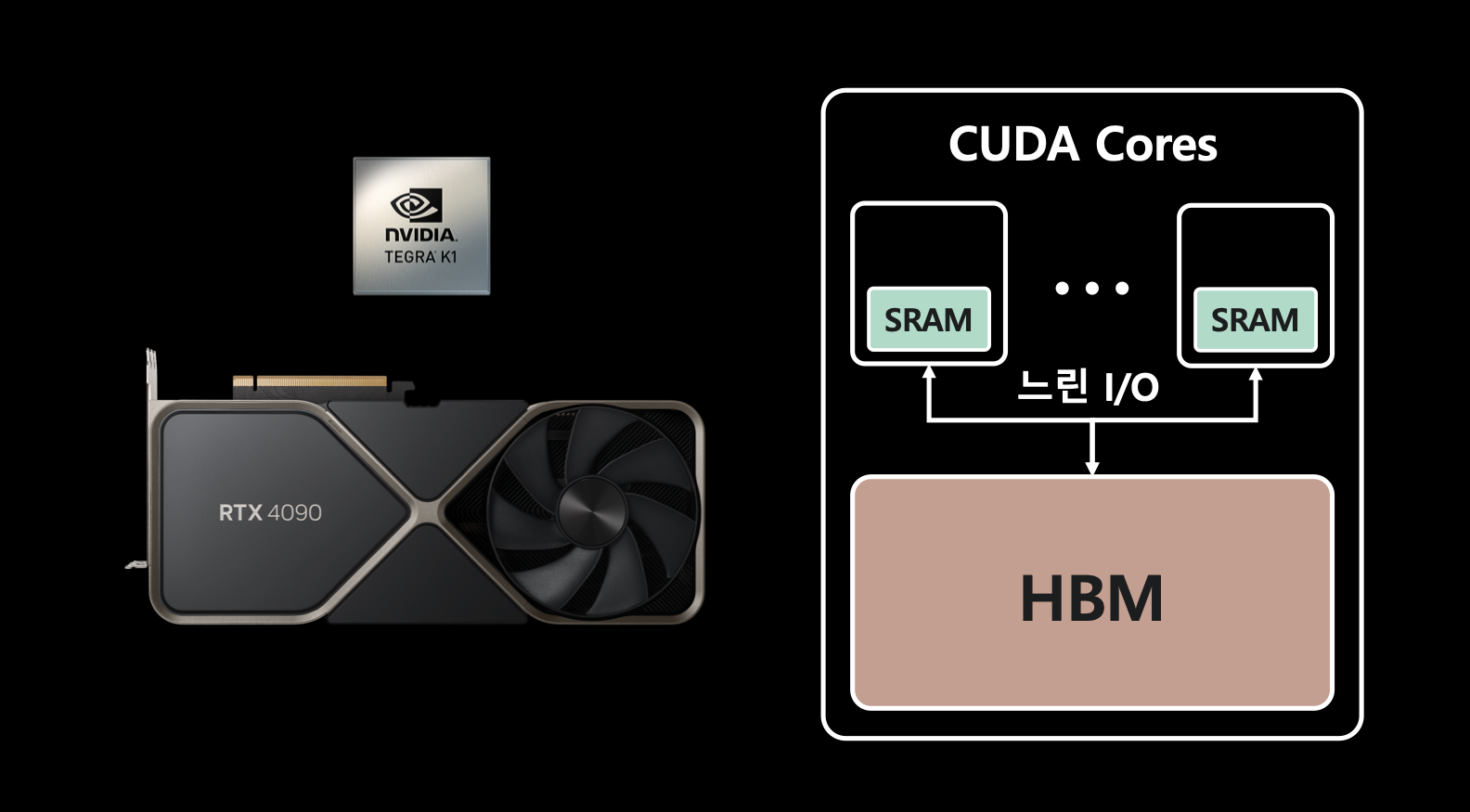
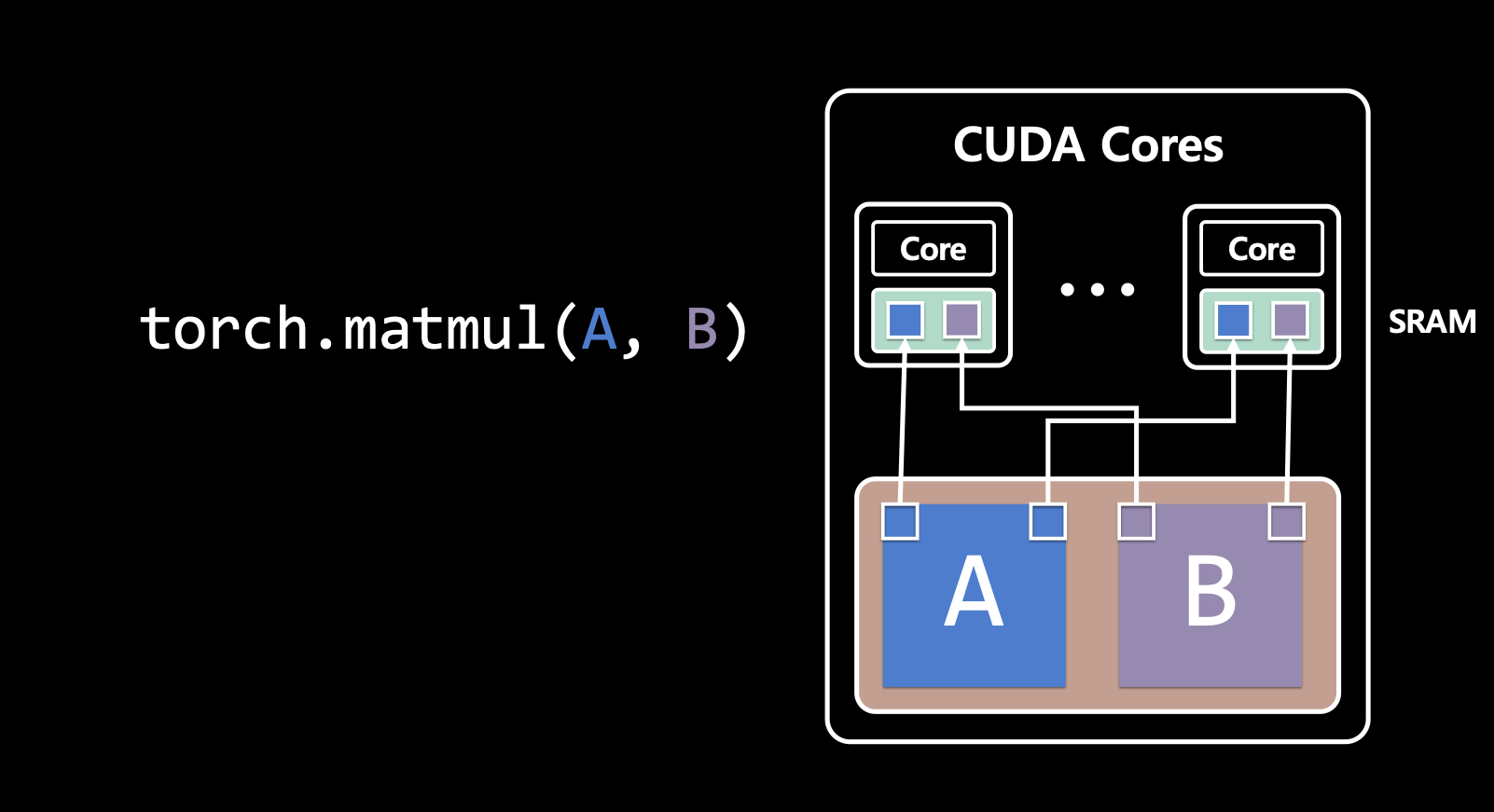
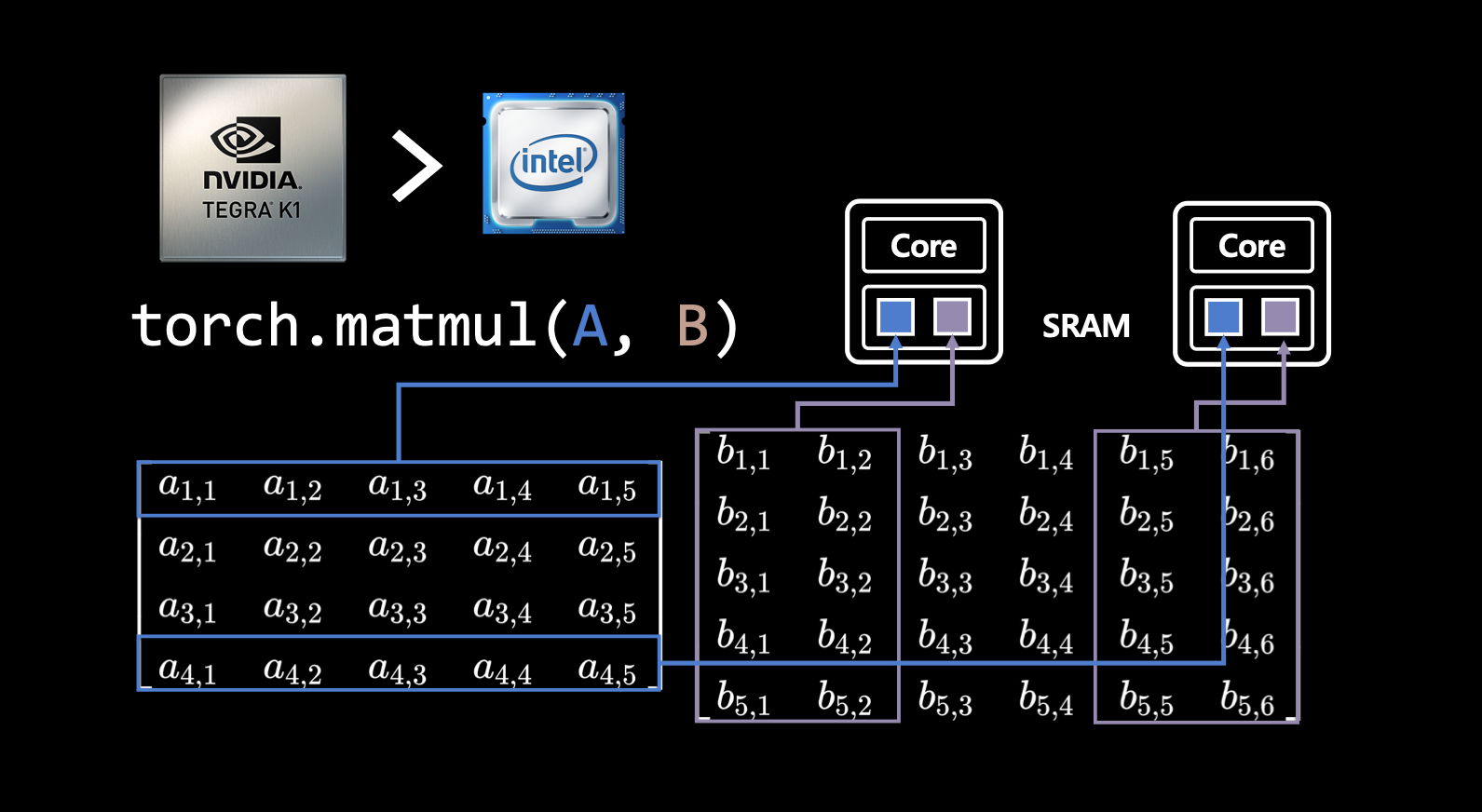
torch.matmul(A, B)
를 사용하면 행렬 A와 B를 곱하는 연산을 수행함. 먼저, GPU의 HBM에 A와 B 행렬을 올림. 그 후, 행렬 곱셈을 하기 위해서 A, B 메트릭의 값들이 부분적으로 SRAM에 들어가 CUDA Core 연산이 시작 됨. 이런 연산들은 병렬적으로 수행됨.
- 즉, GPU가 머신러닝 모델 학습에 빠른 이유는 학습 시에 핵심이 되는 행렬 곱셈을 병렬 처리할 수 있기 때문.
- 다시 말해, 동시에 여러 개의 수많은 코어들 사이에서 이 메트릭을 잘게 쪼개서 곱셈을 할 수 있기 때문.
CPU보다 GPU 연산이 느린 작업들
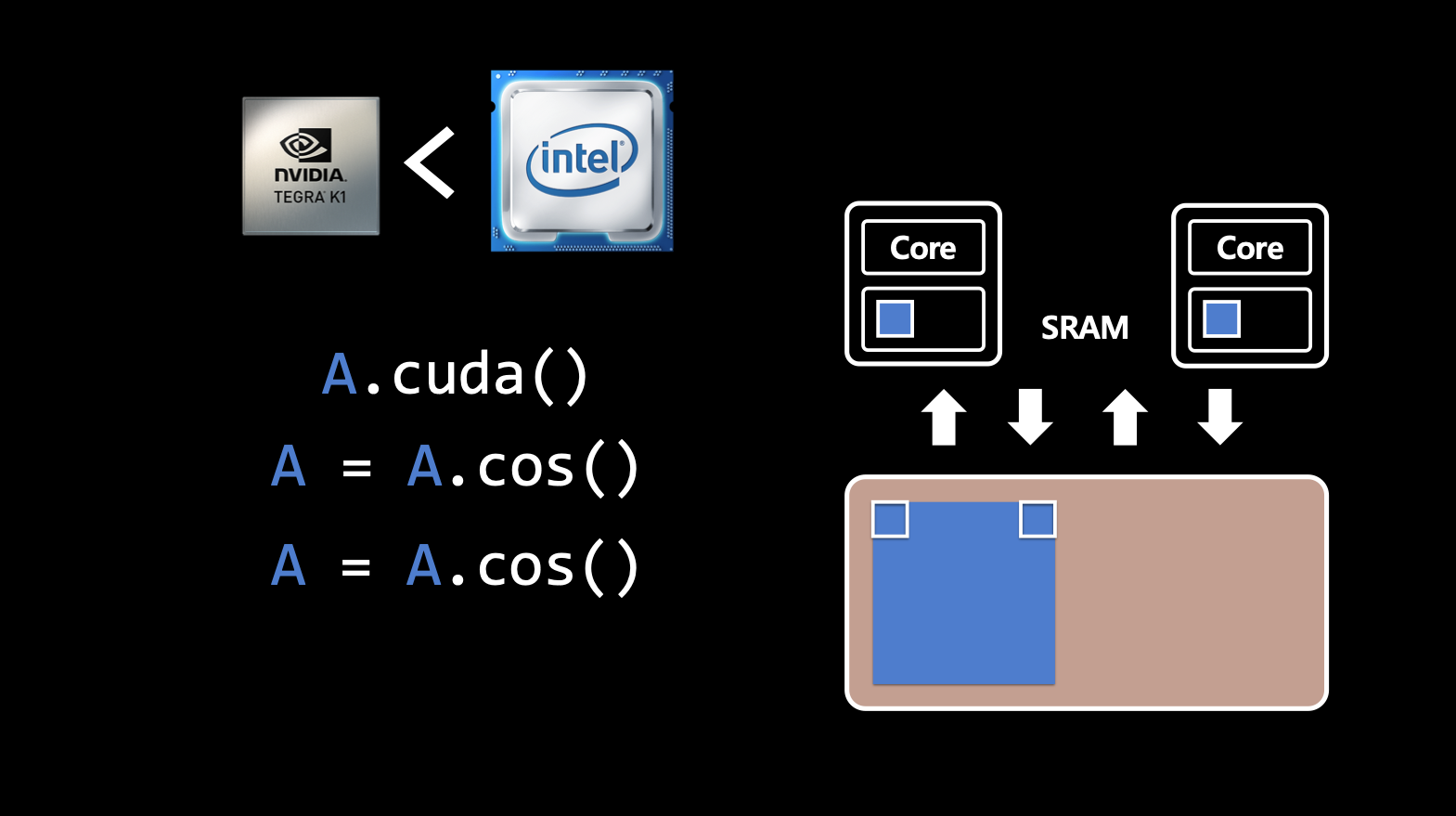
- 예를 들어, A 메트릭에 대한 cos 값 계산을 수행했을 때,
HBM에 메트릭 저장 → SRAM 에 불러와 → Core 연산 → 다시 HBM에 저장
과정을 수행하는데 A.cos().cos() 같은 작업을 했을 때, 이런 작업들이 반복적으로 늘어남.
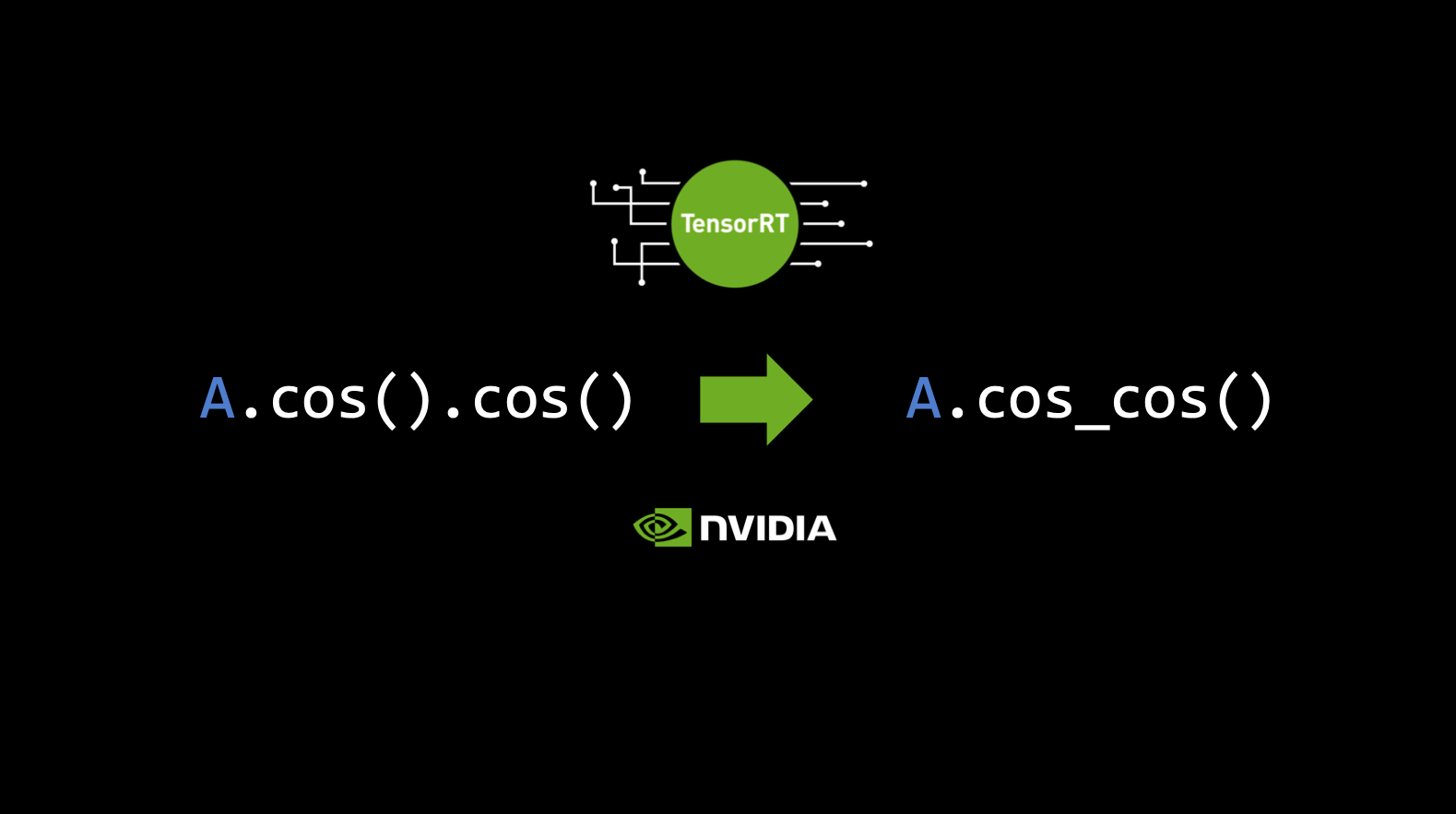
- 그래서 이렇게 읽고 저장하고 일고 저장하고 두 번 하는 작업을 한 번으로 줄여주는 작업이 nvidia가 만든 TensorRT 라이브러리의 역할임.
TensorRT를 쓰는 방법
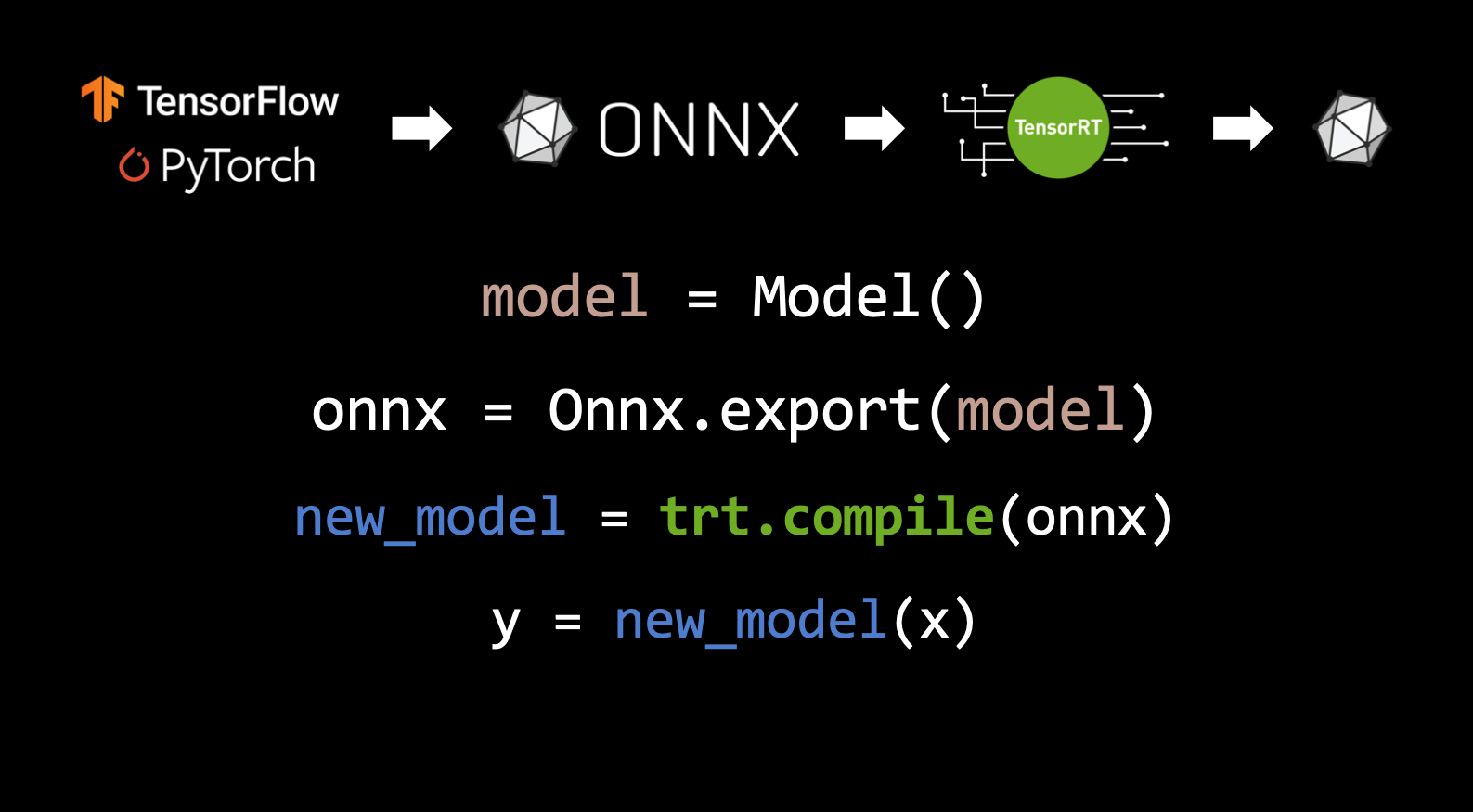
- TensorFlow나 Pytorch로 모델 개발 → ONNX로 번역 → TensorRT로 컴파일
그럼에도 TensorRT가 해결하지 못하는 문제(?)
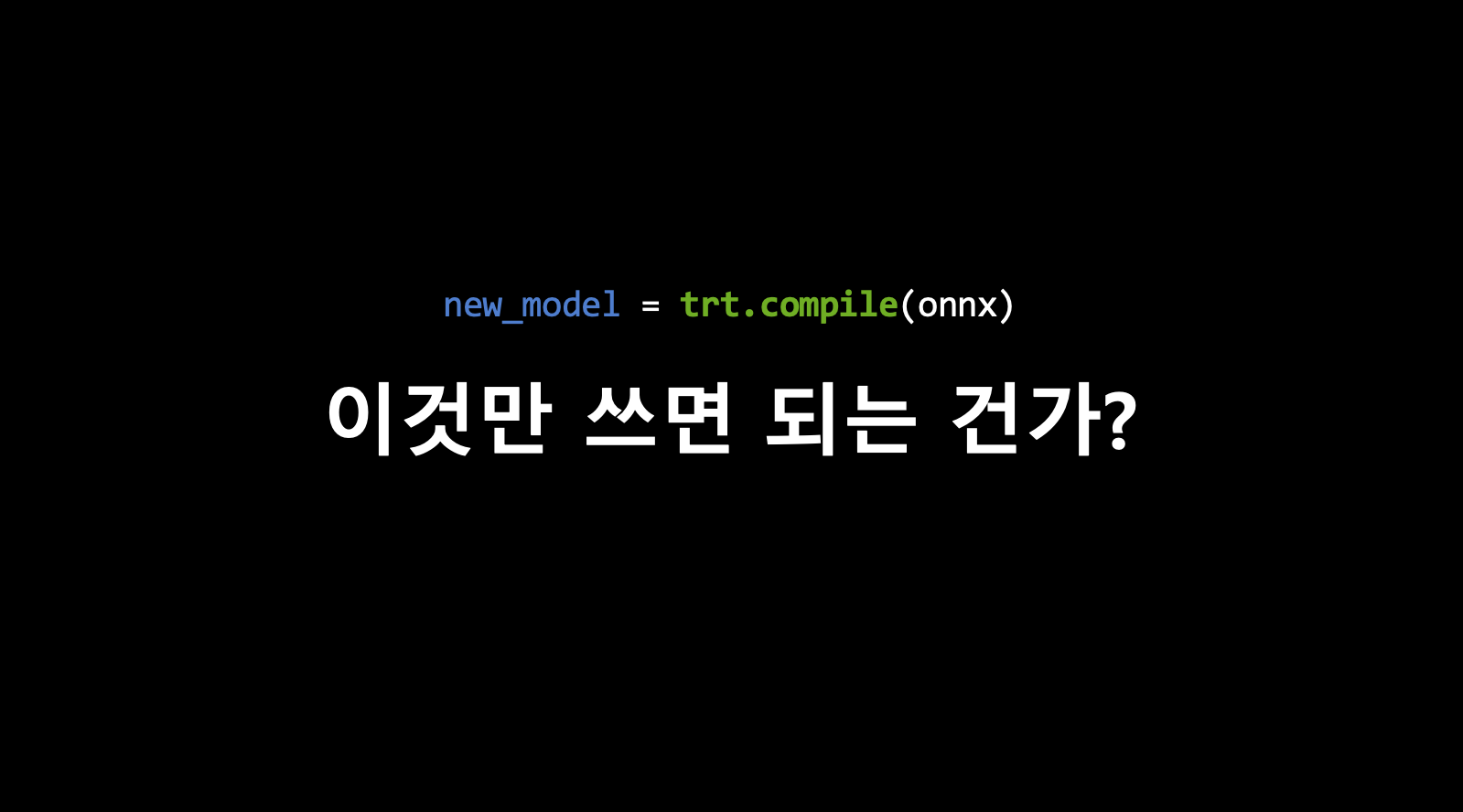
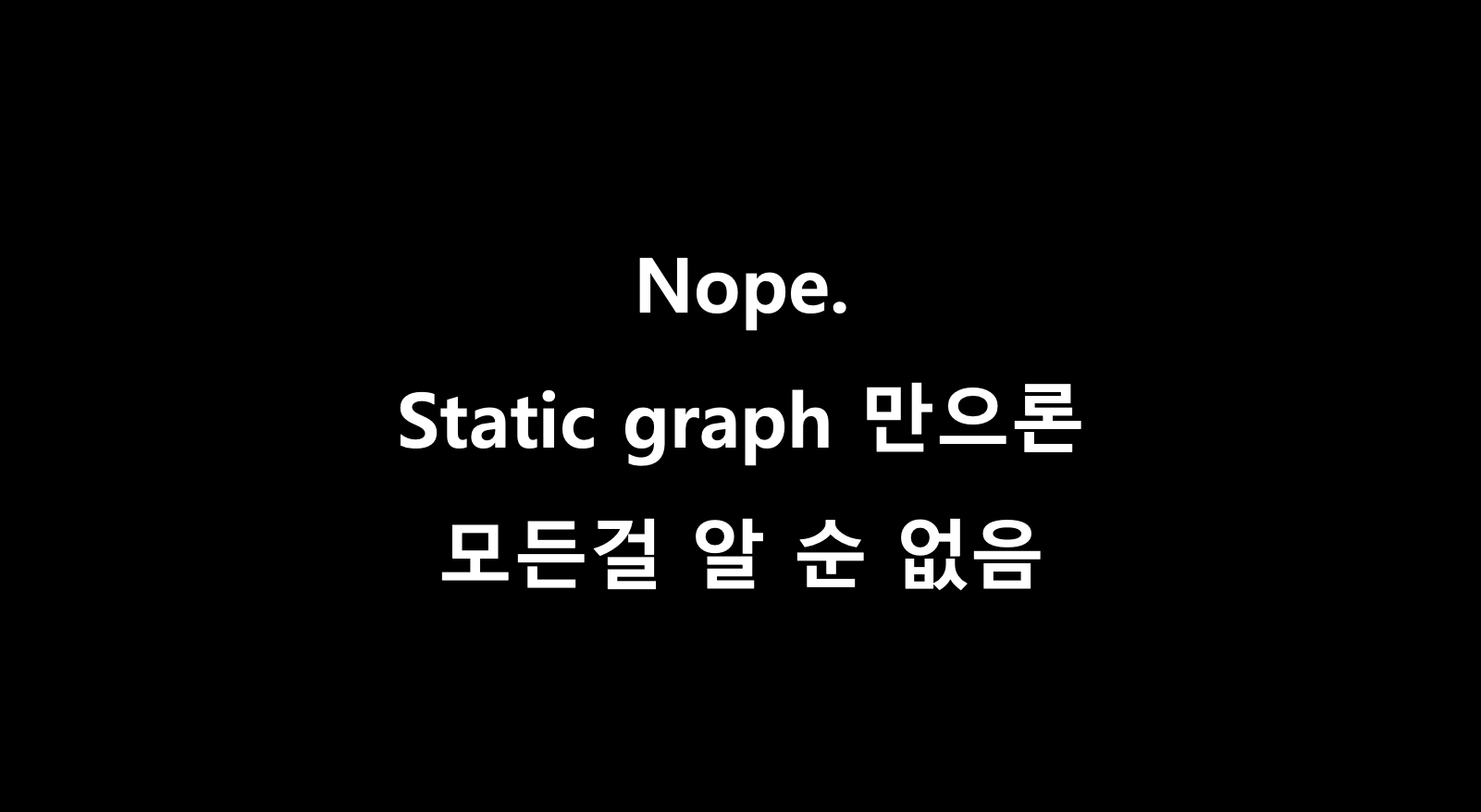
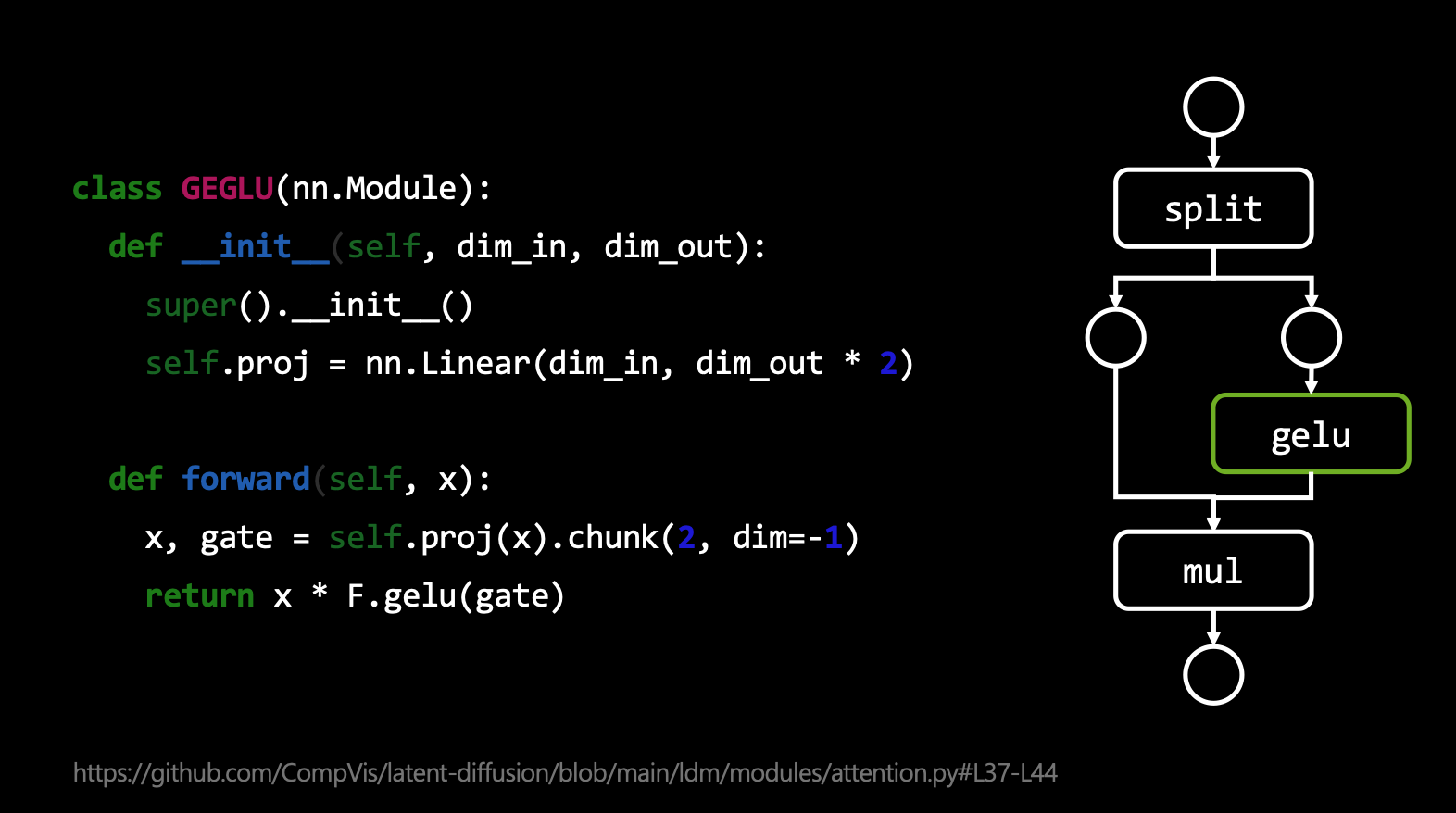
- 예를 들어, stable diffusion의 활성 함수 GEGLU가 있는데 이는 x라는 인풋값을 받으면 반반으로 쪼개어 반은 gelu 함수로 계산하고 이 값을 나머지 반과 곱해서 결과물을 내는 과정을 수행하는데 이 작업을 엄청 반복되는 오퍼레이터임.
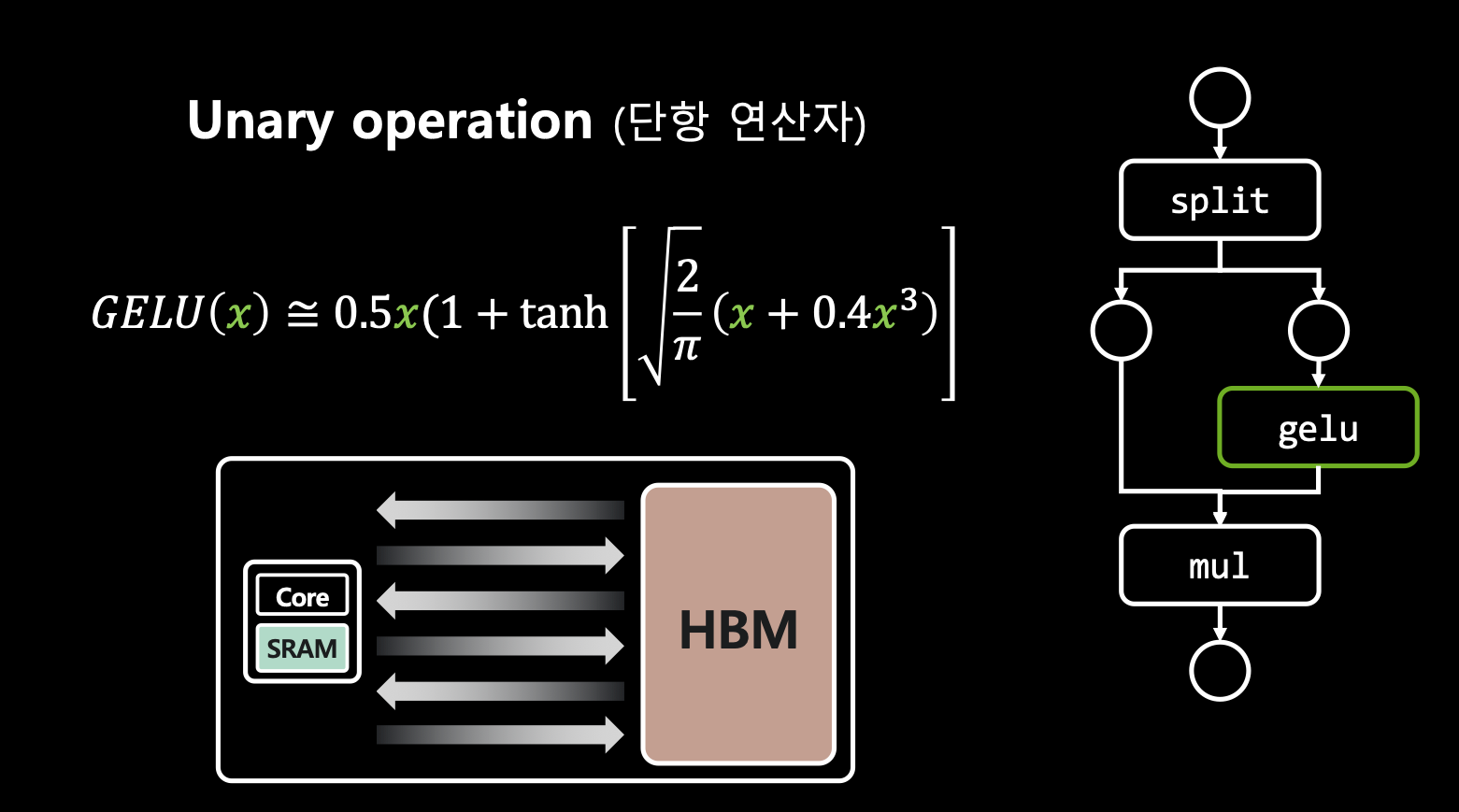
- 아무튼 여기서 GELU는 단항 연산자(unary operation)임. 인풋으로 x 하나만 받음. 그런데 얘는 위와 같이 엄청 많은 반복을 함.
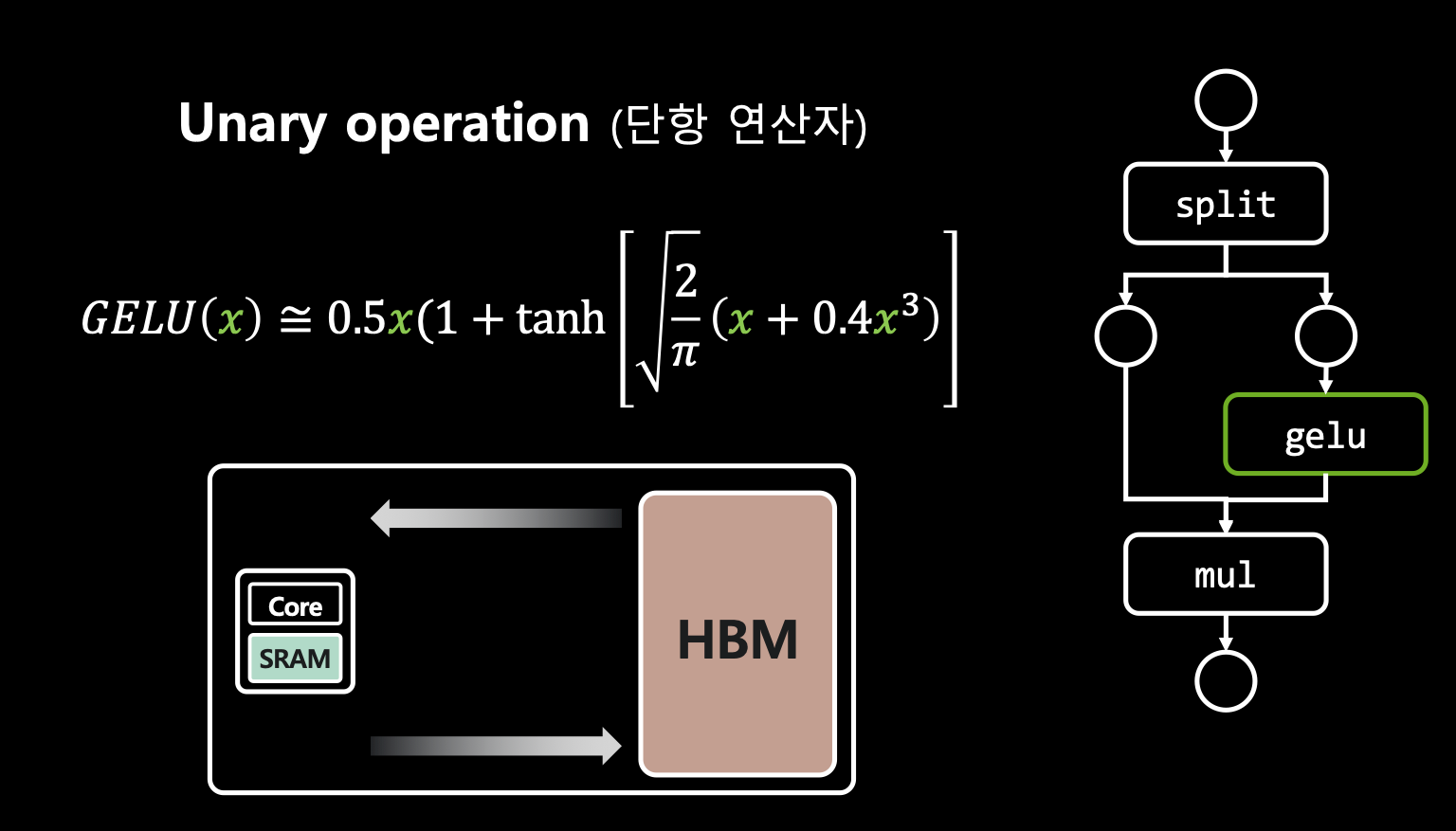
- 그래서 이 과정은 반복이 엄청 됨. 그러므로 TensorRT로 개선할 수 있음.
- 특히나 gelu는 unary operation 이기 때문에(?) 굉장히 효율적으로 개선을 할 수 있다고 함.
- 게다가 gelu는 유명한 함수이므로 TensorRT가 알아서 변환을 해줌.
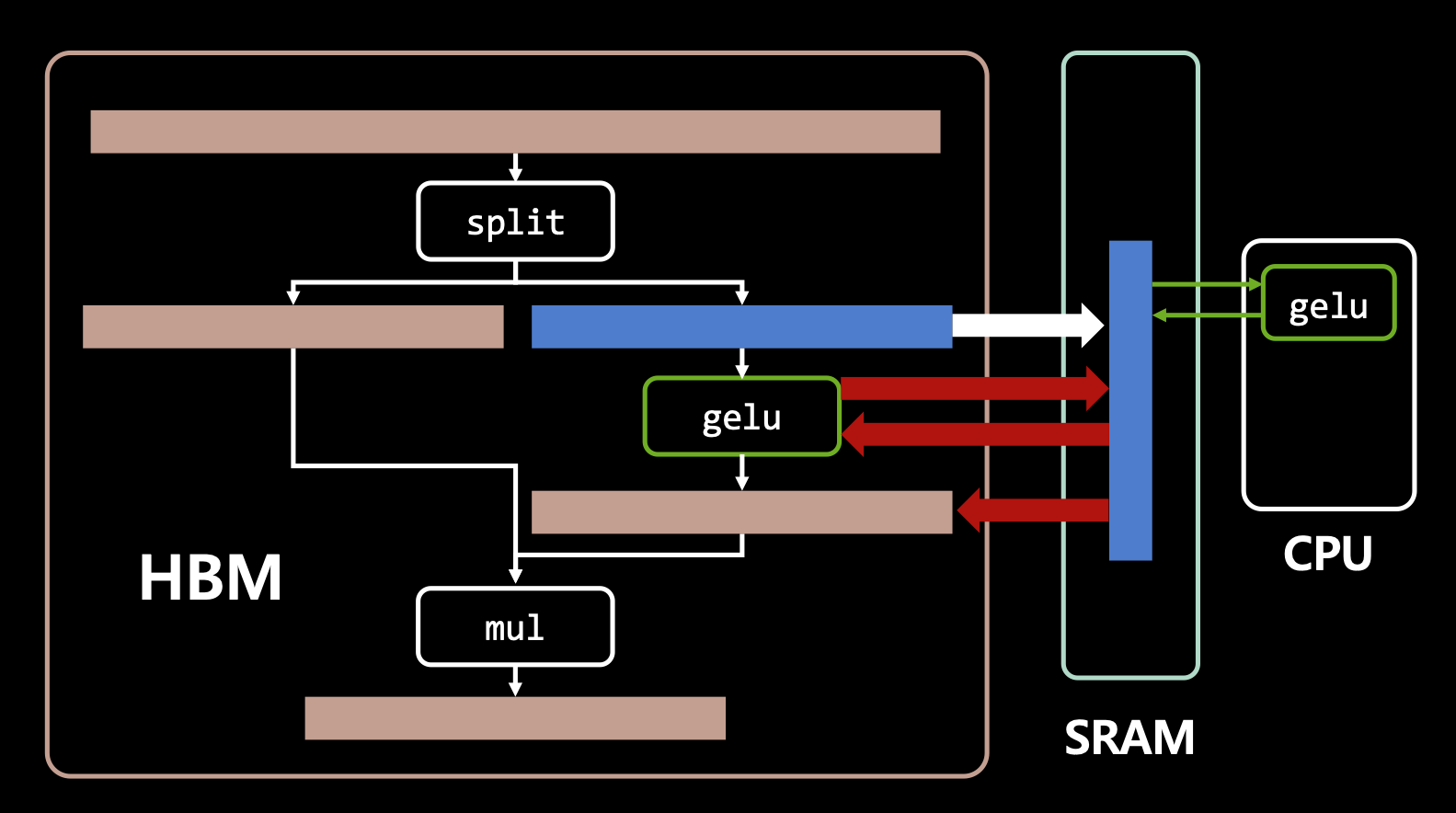
- 다시 말해, 먼저, 많은 데이터 중에 gelu도 HBM에 업로드 되고 SRAM을 타면서 Core로 연산 됨. 여기서 과도한 연산으로 레이턴시가 생김.
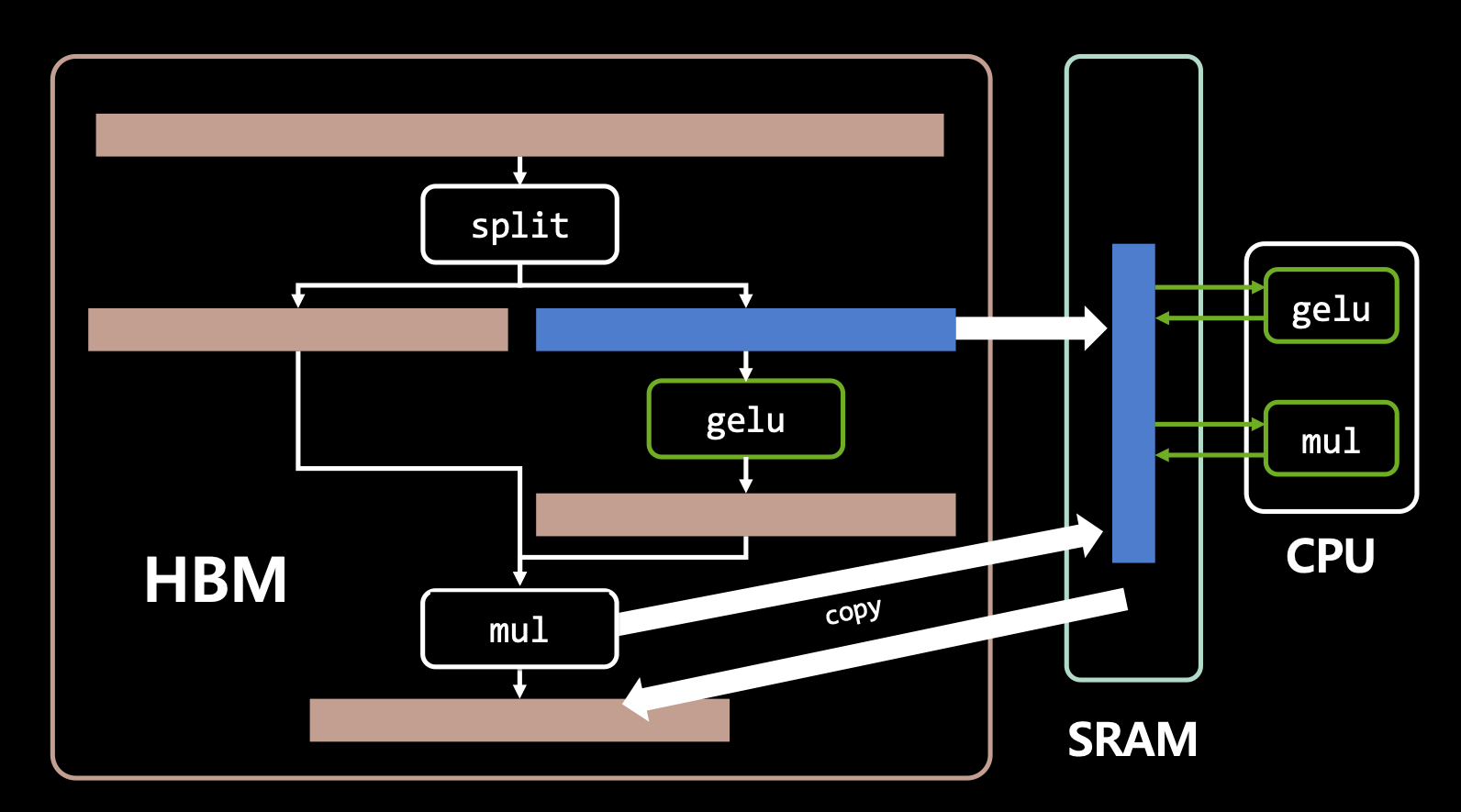
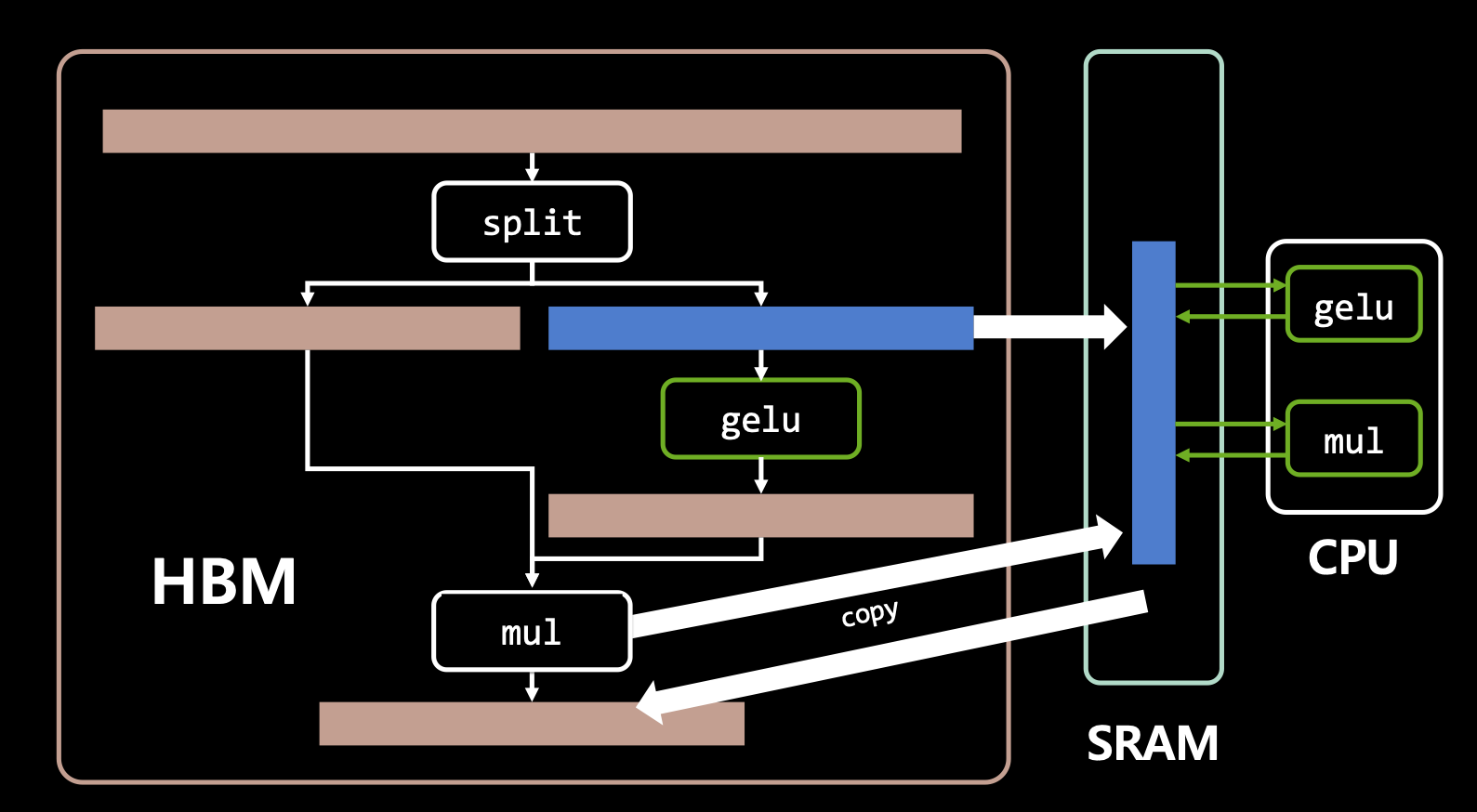
- 그러나 tensorRT를 사용하면 효율적인 I/O로 레이턴시가 개선됨.
이러한 문제를 해결하는 triton(?)
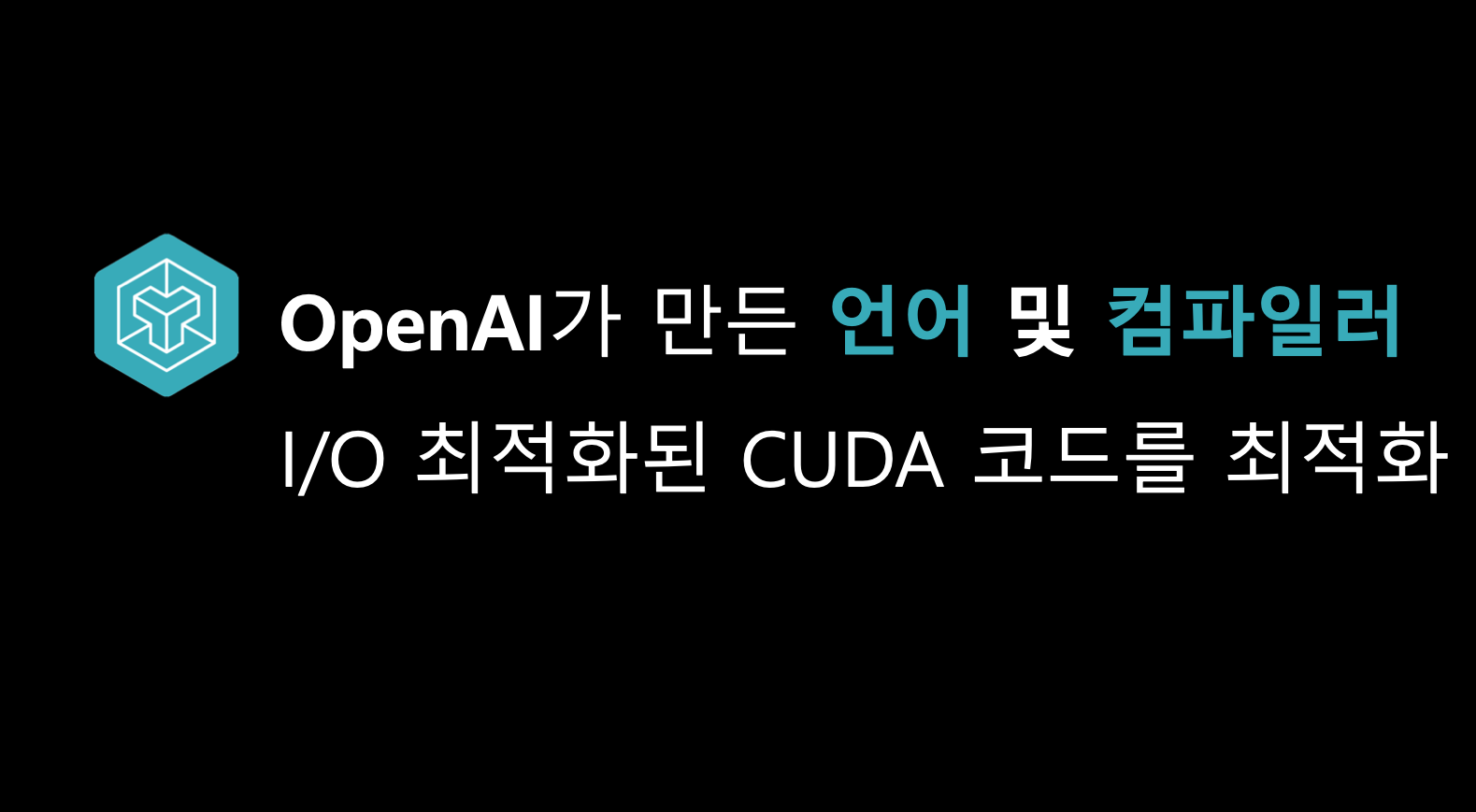
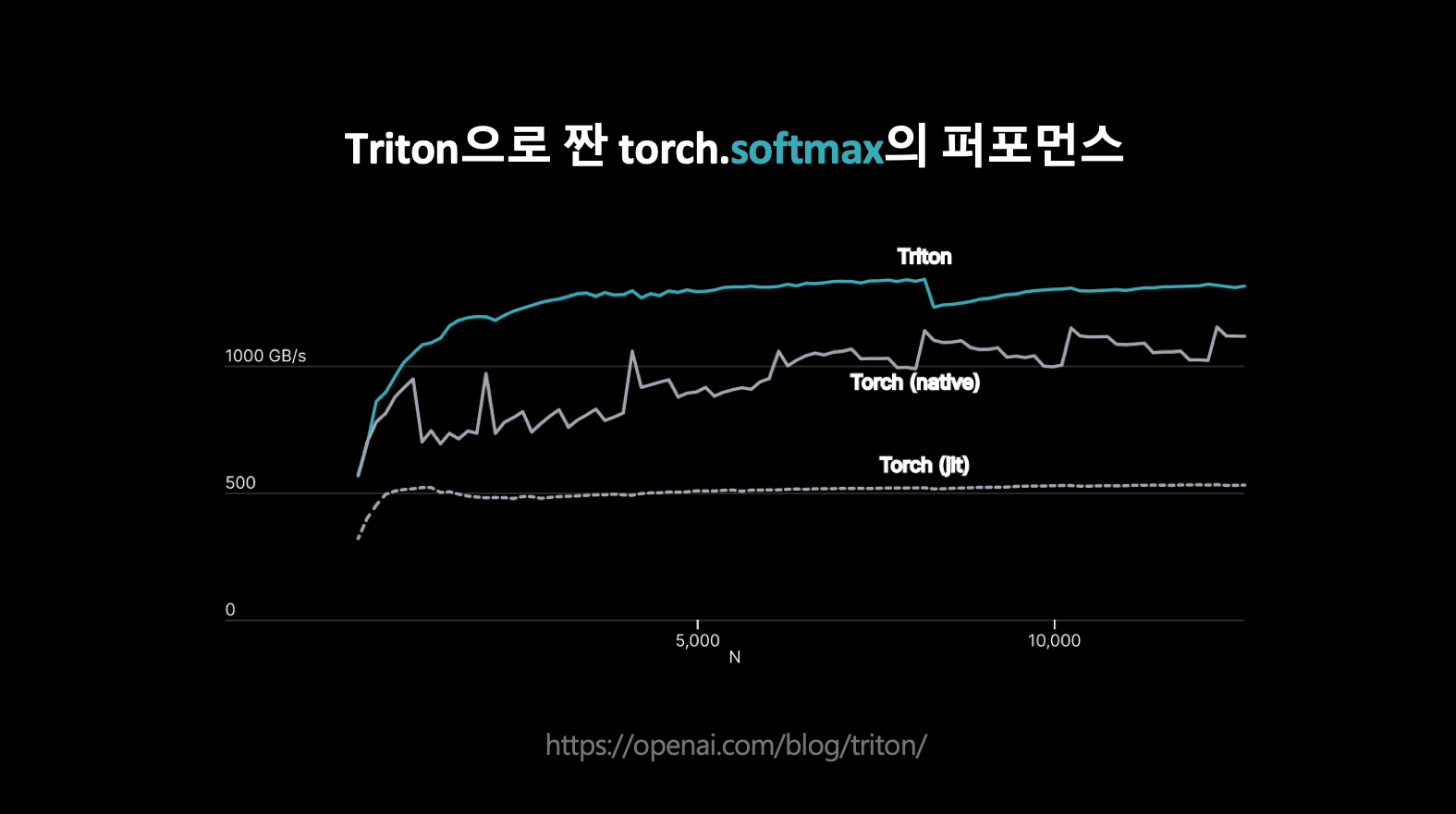
- torch에서 softmax에 적용했을 때 성능.
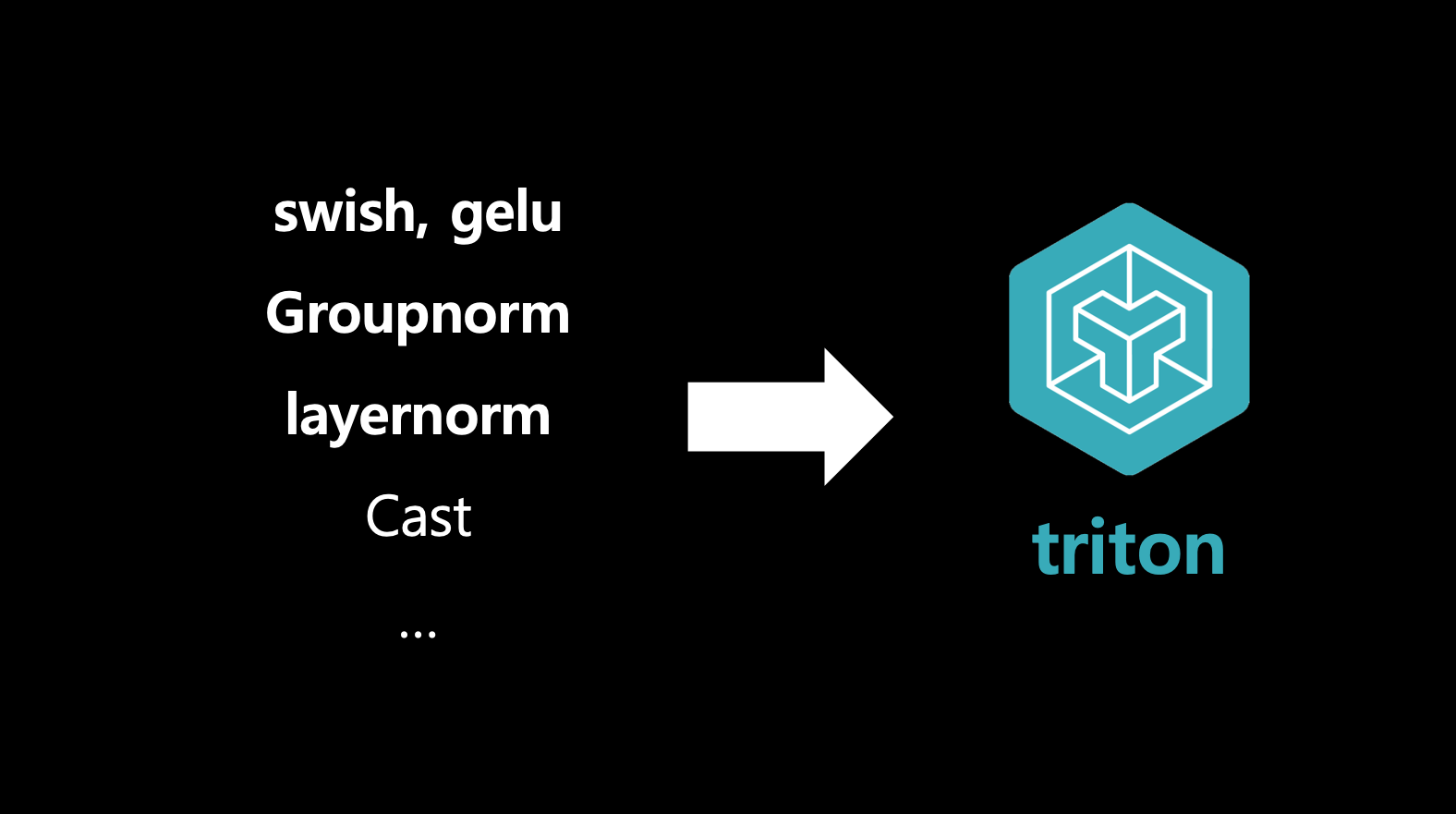
- 즉, 딥러닝에서 tensorRT가 많이 쓰이긴 하지만 그 안에 효율적으로 짜여져 있지 못한 코드들을 개선하는데 triton을 사용함.
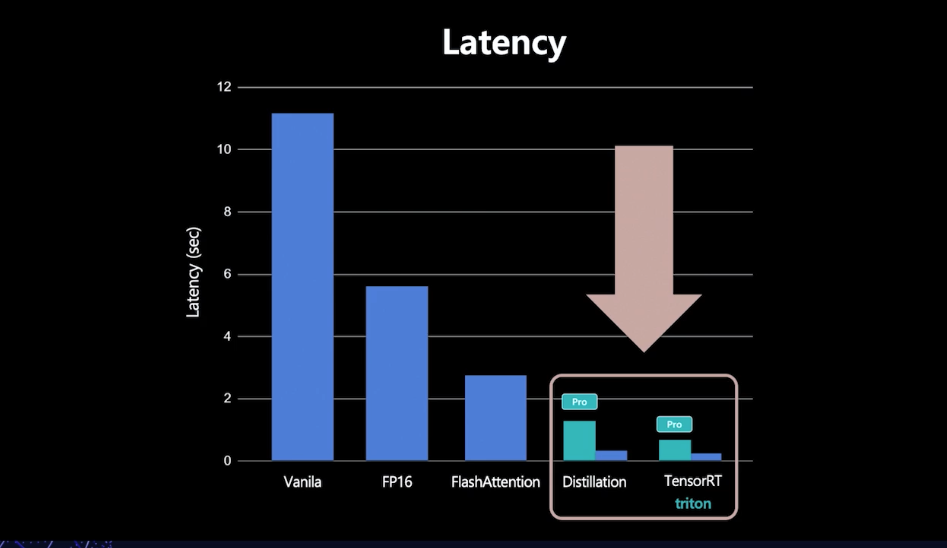
어떻게 serving 했는가?
인프라 구조
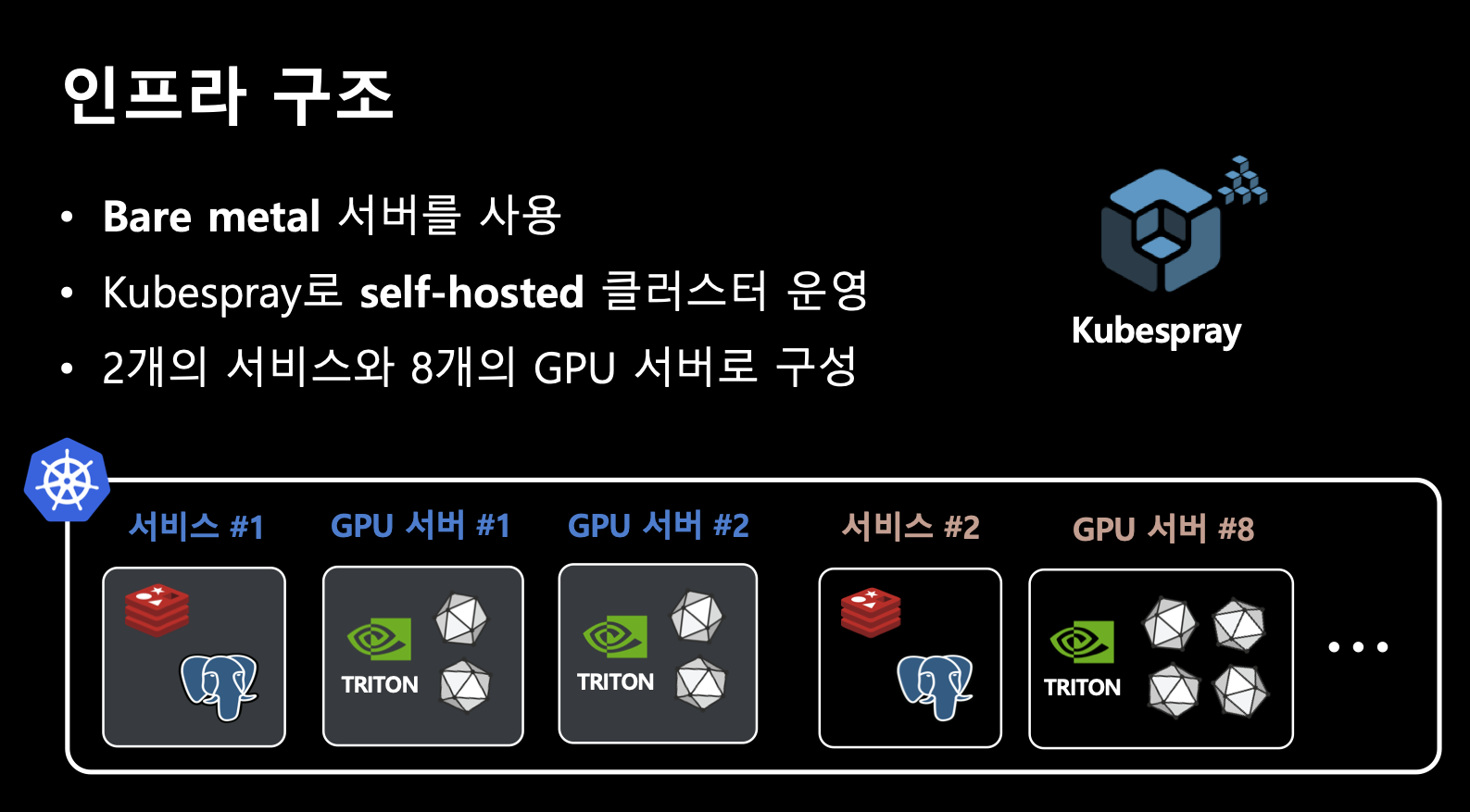
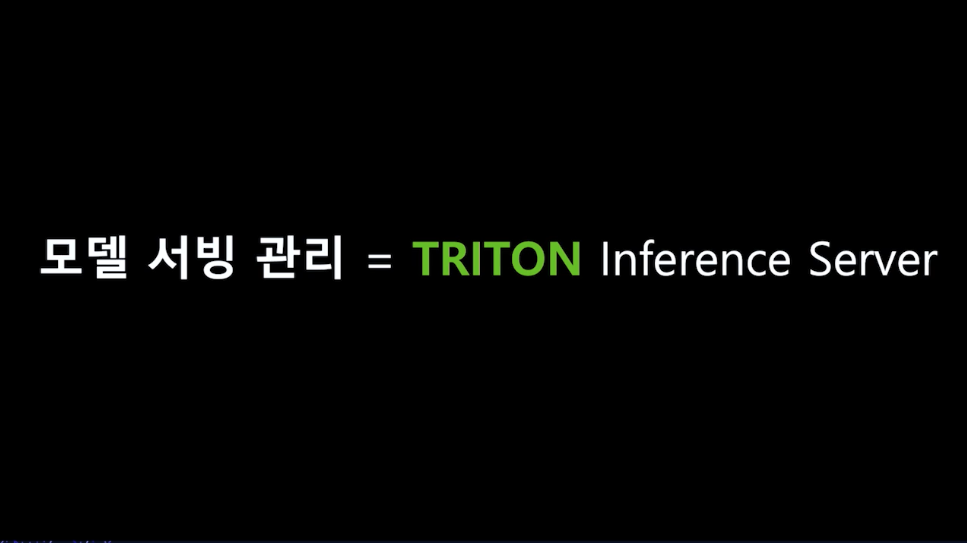
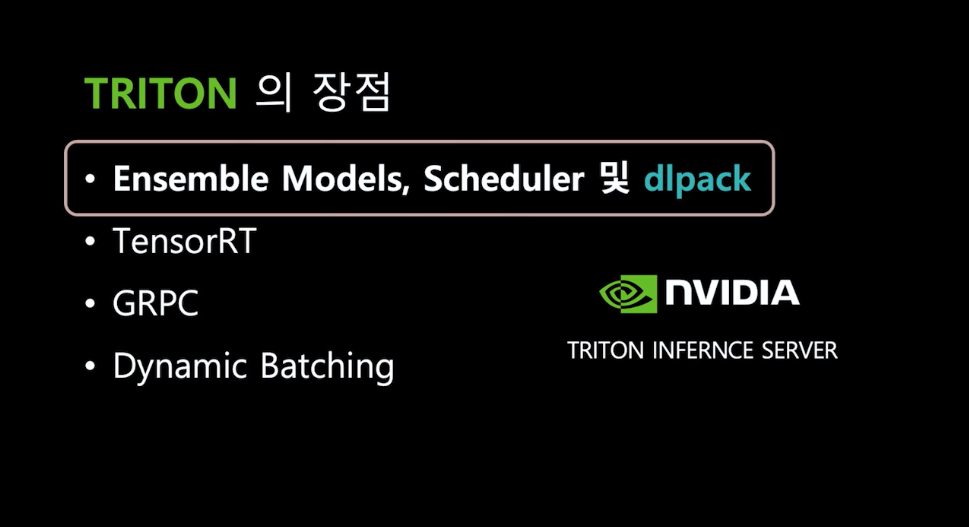
latency를 줄이려 했던 이유
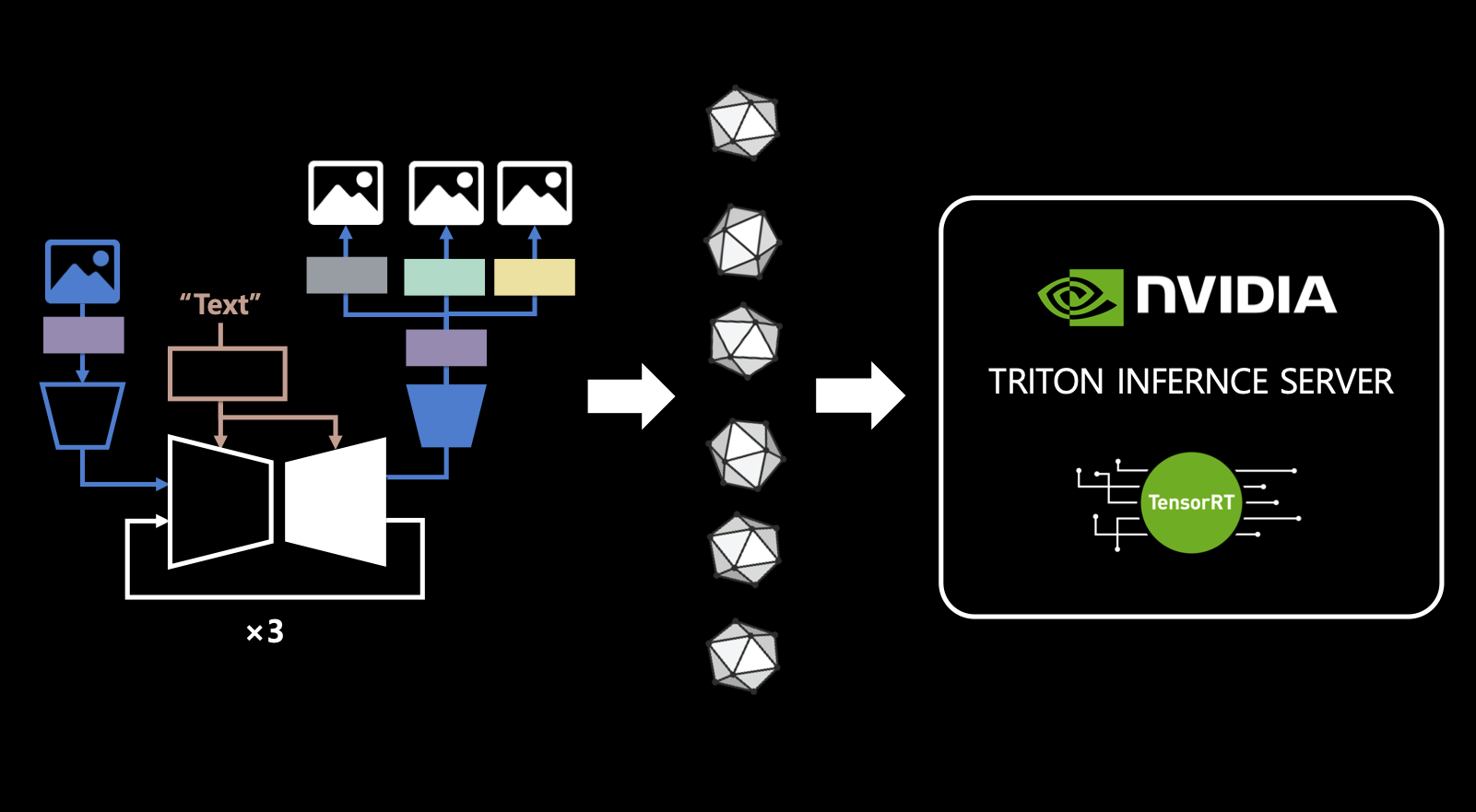
- 여러 모델을 통한 서비스를 위함. 그리고 이 모든 모델들에 대해 tensorRT와 triton inference server를 활용함.
- 주의 : 앞서 연산 최적화 triton과 여기에 inference server triton은 다른 것임.
비동기적인 인퍼런스
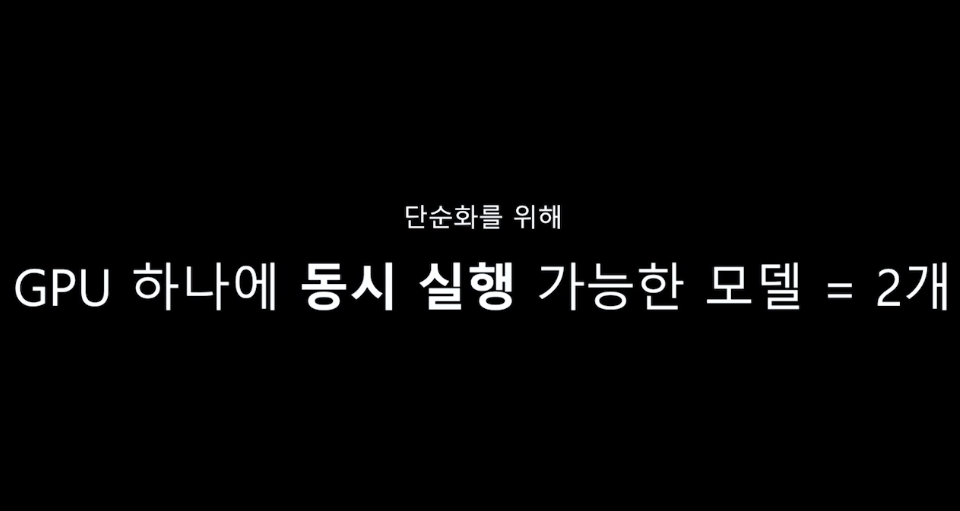
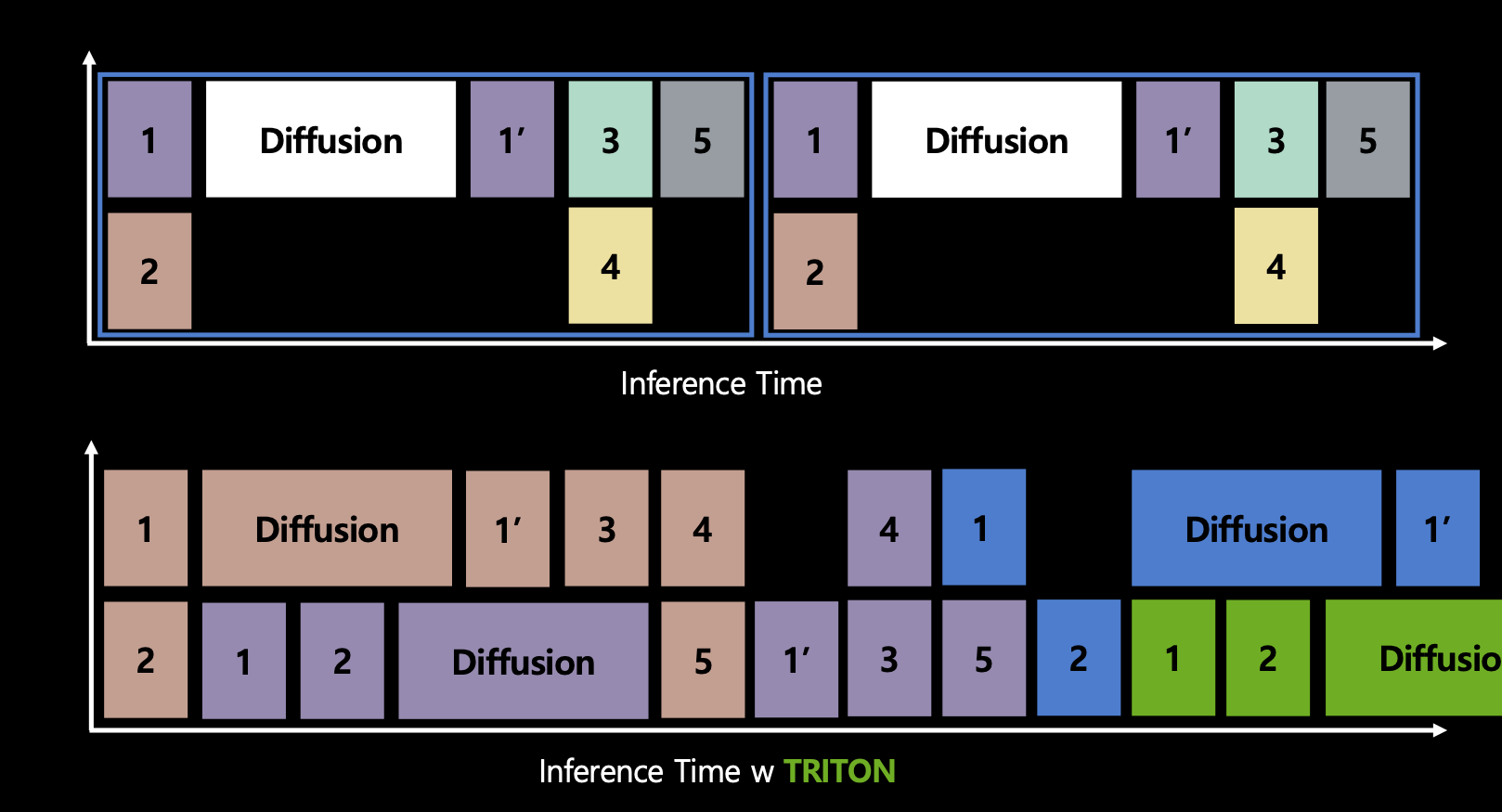
- GPU 하나에 여러 개 모델을 실행할 순 없을까?
- nvidia의 triton inference server로 ensemble model과 scheduler를 사용하면 graph dependency를 계산하여 요청이 연속으로 오더라도 하나의 요청을 처리하는 도중에 다른 요청에 대해 동시에 처리할 수 있음.
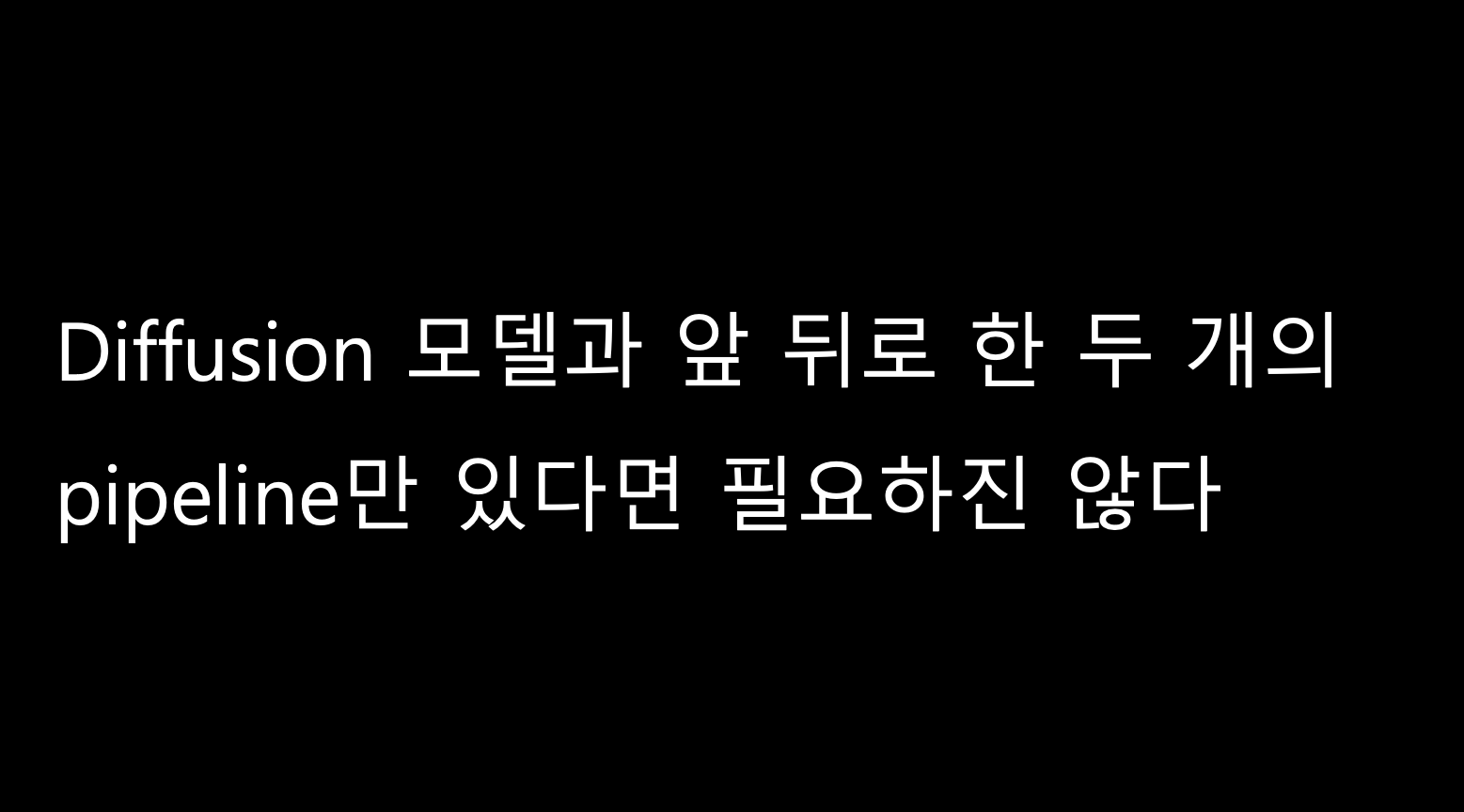
결론
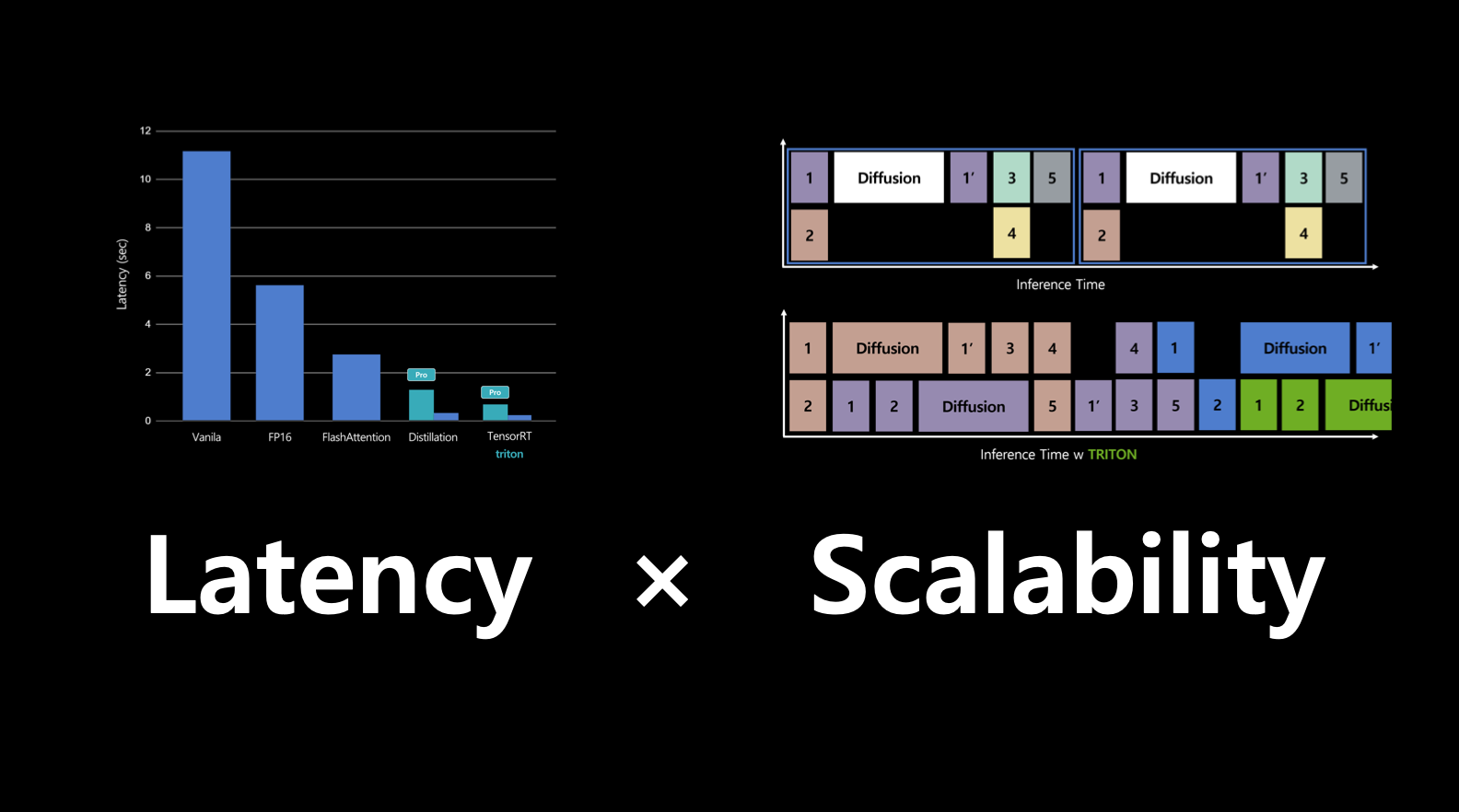
- TensorRT + Triton으로 inference latency를 줄임.
- nvidia Triton inference server로 scalability를 높임.