Abstract
- two contexts
- long-term intra-speaker context
- temporal dependencies of the same speaker
- short-term inter-speaker context.
- interactions of speakers in the same scene.
- self-attention for long-term intra-speaker
- Effectiveness in modeling long-range dependencies
- convolutional blocks for short-term inter-speaker context.
- capture local patterns
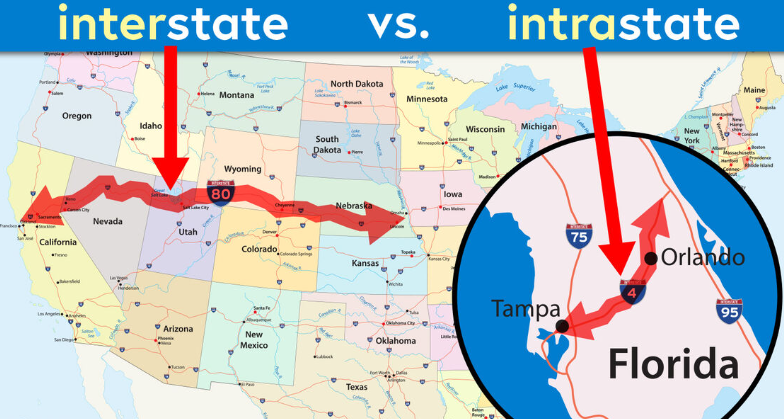
1. Introduction
ASD importance / applications
- Interactive computer vision systems in real-world scenarios need to recognize not only objects and people but also social properties, such as how individuals interact.
- Active Speaker Detection (ASD) is a fundamental task that involves identifying the current speaker in a complex scene with multiple people.
- ASD is important for various applications, including human-robot interaction, speech diarization, and video retargeting.
Long-term Intution & Existing Method Problem
- Determining if someone is speaking involves examining visual cues like mouth movement and eye direction, as well as correlating these movements with observed audio.
- This speaker-independent evidence can be learned by comparing a person's behavior during speaking and non-speaking times.
- Generally, at least 6 seconds of intra-person information is needed to observe both activities.
- However, most existing ASD methods only consider short video segments of around 200ms-500ms. (ASC, ASDNet)
- Long-term intra-speaker context allows models to learn simple cues for speaking activity detection, such as mouth movement and eye direction.
Short-term(multi-speaker) Intuition
- In complex videos, a person's face may be occluded, facing away, off-frame, or too small, making it difficult to determine if they are speaking based on direct visual information alone.
- However, the behaviors of others in the scene can provide evidence about a target person's speaking status. (from HCI Review Paper)
- For example, if other people turn their heads towards the target person and don't open their mouths, it can be inferred that the target person is speaking.
- This inter-speaker context requires only a short-term temporal window, as speaking activities of speakers are more correlated within a short time range than over longer periods.
Existing method problem / Proposed
- Existing ASD methods have not fully utilized long-term intra-speaker and short-term inter-speaker context information.
- ASCNet models both, while other methods focus on long-term intra-speaker and single-frame inter-speaker context, lacking dynamic inter-speaker context.
- TalkNet only considers long-term intra-speaker context without accounting for other speakers.
- These limitations hinder the effectiveness of these methods in challenging situations.
- In contrast, the proposed method incorporates
- both long-term intra-speaker context for capturing speaker-independent speaking indicators
- short-term inter-speaker context to infer speaking activity from other speakers.
- These two contexts complement each other, providing more reliable information for ASD
Proposal
- LoCoNet is proposed as an end-to-end Long-Short Context Network that addresses the limitations of existing ASD methods by combining long-term intra-speaker context, short-term inter-speaker context, and end-to-end training.
- The self-attention mechanism is used for modeling long-range dependencies in long-term intra-speaker context, while convolutional blocks capture local patterns in short-term inter-speaker context.
- Unlike most ASD methods that use vision backbones for audio encoding, LoCoNet introduces VGGFrame, a modification of VGGish that leverages pretrained AudioSet weights to extract per-frame audio features. This approach is the first to combine these elements to improve ASD performance.
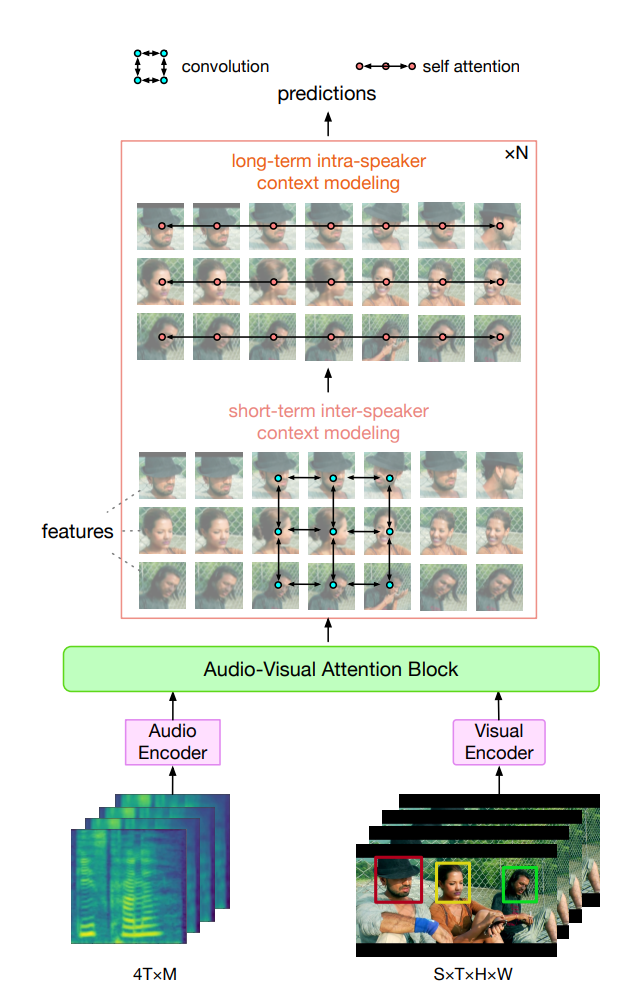
3. Approach
Input stacked consecutive face crops of the target speaker and context speakers in a video clip of length T, and corresponding audio signals
3.1 Encoders
Visual Encoder
- first sample S − 1 speakers from all candidate speakers that appear in the same scene
- stack the face crops of all sampled speakers together
- The target speaker is always the first element along the S axis for the convenience of evaluation
- All the face tracks are stacked and converted to gray scale
- V∈RS×T×H×W×1
- Given face crop of each Speaker
- Vi∈RT×H×W×1
- Visual encoder yields a time sequence of visual embeddings
- evi∈RT×Cv
- The stacked visual embeddings of all the sampled speakers
- ev∈RS×T×Cv
Audio Encoder
- per-frame audio and video features to do per-frame classification
- most pretrained audio encoders for audio classification
- have a high degree of temporal downsampling, which makes it difficult to be applied in our case.
- VGGFrame
- fully utilize pretrained VGGish
- remove the temporal downsampling layer after block-4
- instead add a deconvolutional layer to upsample the temporal dimension
- concatenate the intermediate features with the upsampled features to extract a hierarchy of representations
- Input
- Mel-Spectrogram A∈R4T×M
- Audio Embedding: ha∈RT×Ca
- To Align S speaker, repeat the S times: ea∈RS×T×Ca
Audio-Visual Attention Block
- Dual-attention (same with TalkNet) <= 근데 왜 Reference 안달아두었지
- hv=MLP(MHA(ev,ea,ea))
- ha=MLP(MHA(ea,ev,ev))
- u=Concat(hv,ha),u∈RS×T×(Cv+Ca)
3.2 Long-Short Context Modeling (LSCM)
LSCM contains N blocks, each of which consists of a Short-term Inter-speakerModeling (SIM) module and a Long-term Intra-speaker modeling (LIM) module sequentially
Short-term Inter-speaker Modeling (SIM)
- For a given moment in the video, the speaking activity of a target person can be inferred from the behavior of other people in nearby frames, but behaviors of other people in distant frames is not useful.
- short temporal receptive field can better learn dynamic patterns in interactions.
- EASEE, ASDNet, MAAS
- capture speaker-interaction in each individual frame or fuses it with long-term modeling
- This means that the model needs to capture local temporal information instead of long-term dependencies
- employ a small convolutional network
- usl=LayerNorm(Convs×k(ul))
- ul∈RS×T×C: output of pervious layer l
- usl∈RS×T×C: output of this layer l
- s is set to 3, which is the same as the number of context speakers,
- k is set to 7 frames
- usl=MLP(usl)+ul
Long-term Intra-speaker Modeling (LIM)
- Long-term intra-speaker context models an individual person’s behavior over a longer time period
- model needs to have large capacity and the ability to learn long-term dependencies
- Transformer Layer
- hl=LayerNorm(SelfAttention(usl)+usl)
- ul+1=LayerNorm(MLP(hl)+hl)
- ul+1=LIM(SIM(ul))
- Previous Work
- ASC, EASEE, ASDNet, MAAS
- jointly model inter-speaker and intra-speaker context
- SIM + LIM (Disjoint Model)
- first focuses on short-term inter-speaker context and then focuses speaker-dependent pattern learning in longterm intra-speaker context
- easier to optimize and can learn more discriminative features
- repeated N times to further refine and distill more informative features.
3.3 Loss Function
5. Experiment
5.1 Context Modeling
Long-term Intra-speaker Context
Short-term Inter-speaker Context
-
To investigate the importance of short-term inter-speaker modeling, the temporal length is kept at 200 frames while varying the number of speakers from 1 to 3.
- More than 3 speakers were not tested, as over 99% of the AVA-ActiveSpeaker dataset videos have 3 or fewer speakers in the same frame.
-
Results in Table 1b show that
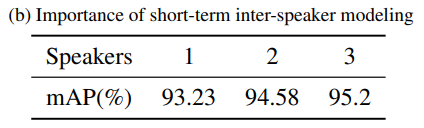
- mAP consistently improves with more speakers included in training.
- This suggests that modeling multiple context speakers in short-term inter-speaker context is beneficial for the ASD task due to the correlation between speakers in the same scene.
5.2. Comparison with State-of-the-Art
AVA-ActiveSpeaker dataset
- [Explain]
- less challenging due to larger faces, static backgrounds, and only 1.6 speakers per frame on average.
- LoCoNet achieves 95.2% mAP, outperforming the state-of-the-art EASEE by 1.1%.
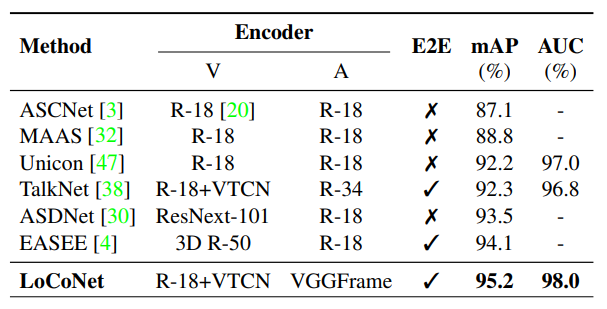
Columbia ASD datase
- [explain]
- A small-scale ASD dataset from an 87-minute academic panel discussion with 5 speakers and 150,000 face crops.
- It has non-overlapping and clear voices, large face sizes, static motion, simple backgrounds, and a small set of speakers, making it less diverse.
- LoCoNet outperforms TalkNet by 22% in average F1 score, demonstrating its robustness and generalizability compared to long-term-only context modeling.
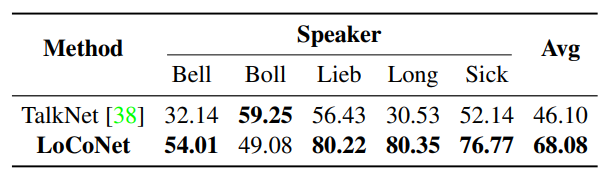
Talkies set
- [Explain]
- The first in-the-wild ASD dataset with 23,507 face tracks from 421,997 labeled frames, focusing on challenging scenarios with more speakers per frame, diverse actors and scenes, and more off-screen speech.
- LoCoNet outperforms the previous best methods by 1.7%, 2.5%, and 2.7% in different settings, showcasing the efficacy of the proposed Long-Short Context Modeling.
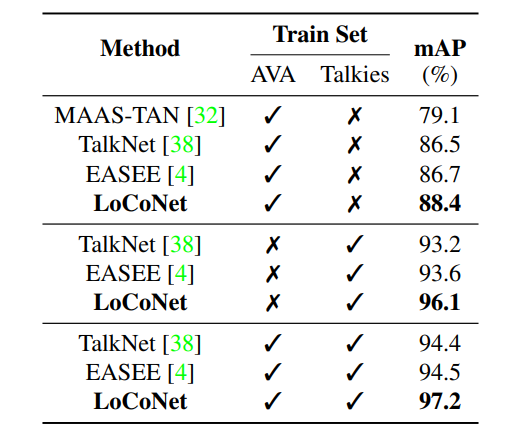
Ego4D dataset
- [Explain]
- The first egocentric ASD dataset with 3,670 hours of unscripted daily activities in diverse environments.
- Unlike other datasets, wearable camera videos in Ego4D have dynamic backgrounds, and active speakers are often off-center, making it massive in scale and highly challenging.
- LoCoNet outperforms the previous state-of-the-art by 0.56% under zero-shot settings.
- When trained on Ego4D, LoCoNet achieves an mAP of 59.69%, outperforming TalkNet by 8.03%.
- The significant improvement indicates that inter-speaker context modeling, which TalkNet lacks
- is useful for egocentric videos with jittery backgrounds and less clear visual cues.
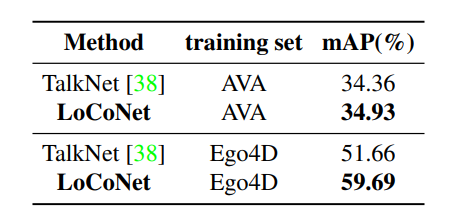
5.3. Challenging scenario evaluation
Quantitative analysis
- LoCoNet is validated for its efficacy in Long-Short Context Modeling by analyzing difficult cases:
- small faces in videos and scenes with many people.
- Face tracks are divided into three categories based on size and by the number of speakers present in the frame.
- LoCoNet consistently performs the best across all scenarios.
-
The improvement over other methods is more evident when there are three faces or when face size is small, outperforming the second-best(ASDNet) method by about 3.5% in both cases.
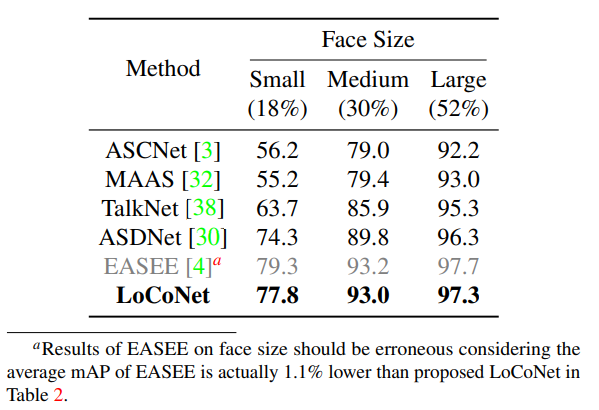
-
This highlights the importance of short-term inter-speaker modeling, as LoCoNet effectively models context speaker interactions even when the active speaker is not salient or when there are many speakers in a frame.
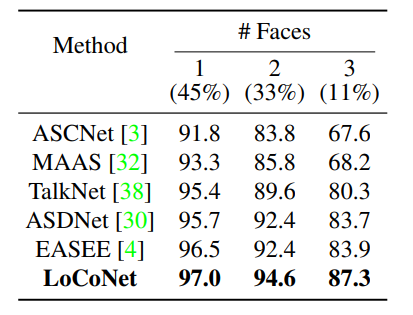
Qualitative analysis.
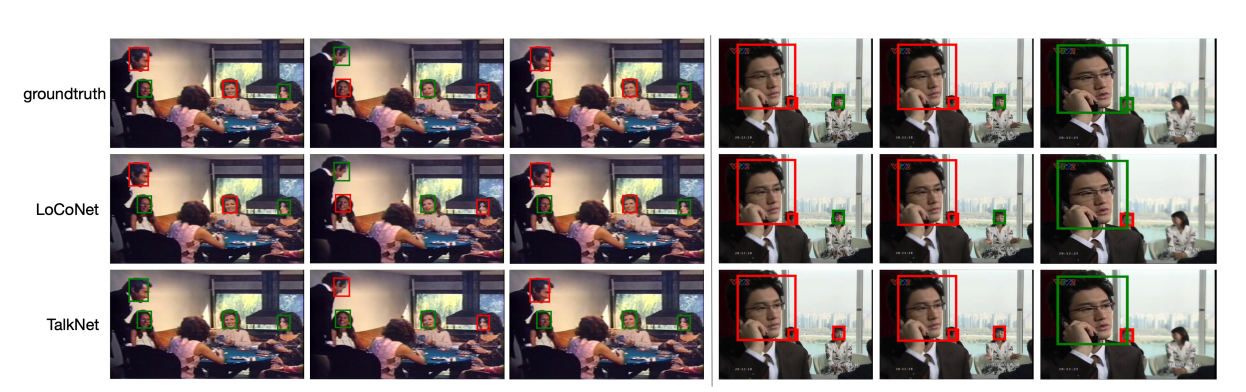
- In cases with multiple visible speakers conversing, LoCoNet successfully identifies the active speaker,
- In a video clip where the active speaker has a small visible face in the background and another person with a larger visible face isn't speaking, LoCoNet accurately identifies the active speaker,
- However, both methods fail to recognize an active speaker with a small visible face at the back of separate conversations, which is an especially challenging scenario.
5.4. Design Choices
Audio Encoder
- The study compares ResNet-18 pretrained on ImageNet with VGGFrame pretrained on AudioSet and finds that using VGGFrame as the audio encoder improves performance by about 1% mAP, suggesting that an audio encoder pretrained on audio datasets is more effective than a common vision encoder
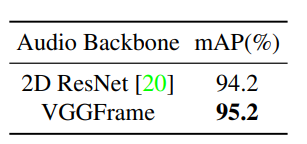
Context Modeling
-
Different context modeling approaches are investigated,
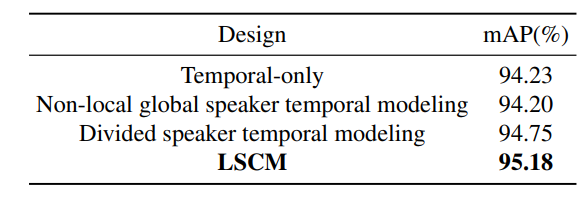
- temporal modeling only (wo CNN)
- non-local global speaker-temporal modeling
- captures pairwise relationships between all the speakers across all the temporal frames (From ASC, and Non-local neural networks.)
- divided speaker-temporal modeling [ref]
-
additional 1% mAP compared to the network using only temporal modeling.
-
The study also finds that replacing the convolutional network with local self-attention in modeling short-term inter-speaker context results in a similar performance to that of LSCM,
- further validating the effectiveness of combining long-term intra-speaker modeling and short-term inter-speaker modeling.