Positional Encoding
- Positional encoding을 이용하여 Sequence의 순서를 고려해주는 vector를 생성해준다.
Residual connection
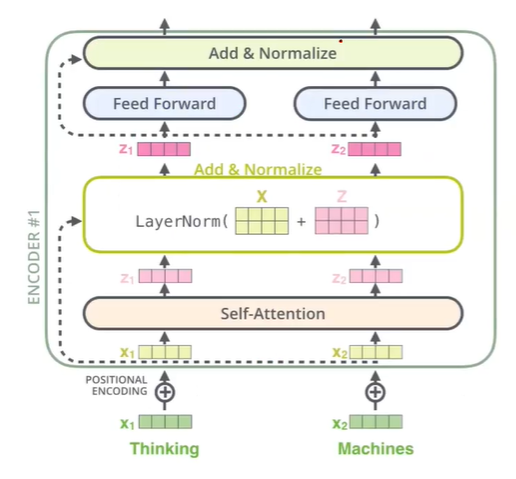
- Positional encoding이 된 vector을 self-attention의 output의 concat 해준다. 이는 기존 일반적인 Neural Net의 residual 효과를 이용한 것이다. 또한, Feed Forward 후에 또 residual을 이용해준다.
지금까지 Encoding파트를 보았고, 이제 Decoding 파트를 보자
Decoder
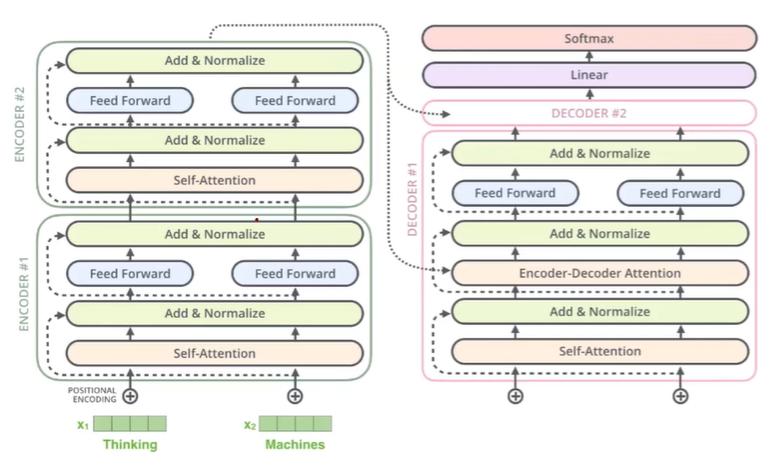
-
기본적으로 Encoding 파트의 network와 매우 유사하다.
-
다른점은 Self Attention이 끝난 이후에, Encoder의 output을 이용하여 Encdoer-Decoder Attention 부분이 추가가 된다.
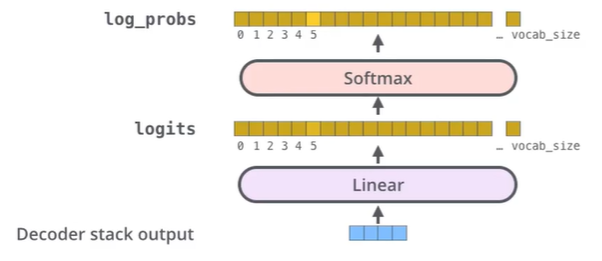
- Decoder의 output이 생성되면, 다음에 나오는 단어가 어떤 단어일지, 예측하는 Linear layer와 Softmax function이 사용된다.
Summary
- Encoder 파트가 Decoder보다 중요하여, 많은 설명이 있으며, Decoder 파트는 Encoder파트와 거의 유사하다.
- Transformer Network의 Encoder만 사용한 모델이 BERT, Decoder만 사용한 모델이 GPT이다. 두 모델 모두 NLP에서 좋은 성능을 보여준다.
- "What Does BERT Look At? An Analysis of BERT's Attention"에서 각각에서의 Self-Attention이 무엇을 집중하여 이해하는지 분석한다.