[Point Review] Let 2D Diffusion Model Know 3D-Consistency for Robust Text-to-3D Generation
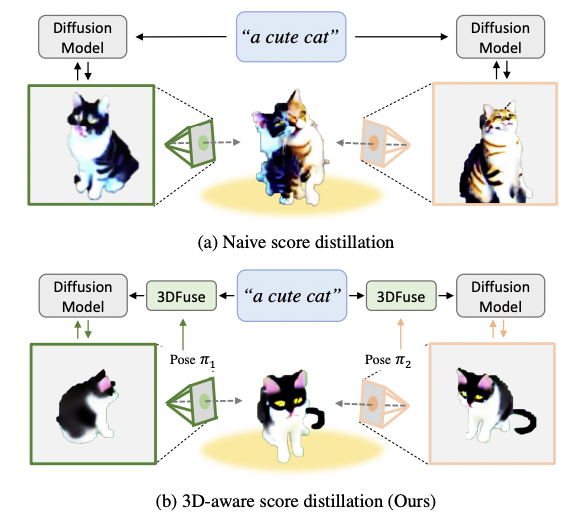
- Diffusion model에는 3D 정보가 없으므로 bias에 의해 front view를 더 많이 생성하게 되고 3D로 볼 때 여러 pose에서 face가 나타나는 현상이 있음
- 또한, text ambiguity에 의해 같은 prompt여도 다른 이미지가 생성될 수 있음
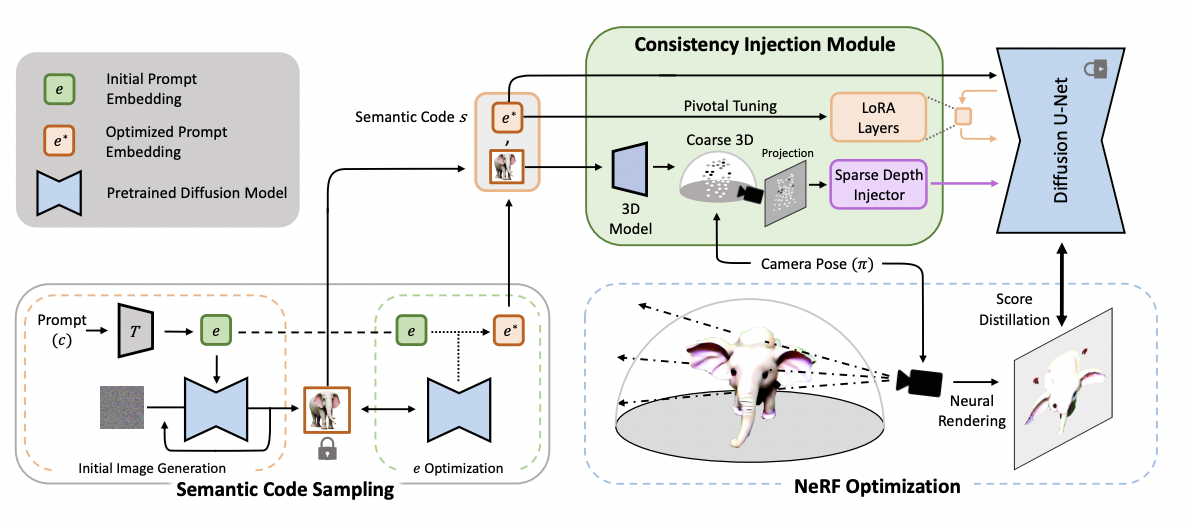
- Initial image로부터 text prompt embedding을 optimize하여 만든 semantic code를 diffusion model에 condition으로 주어 text ambiguity 문제를 해결
e∗=eargmin∥ϵθ(x^t,e)−ϵ∥22,
- Initial image의 depth를 추정하여 point cloud를 만들고 이를 다른 view에 projection한 결과를 condition으로 주어 diffusion model이 3D 정보를 알 수 있도록 함