Thm 1.3.4
0≤P(C)≤1, ∀c∈B
0=P(ϕ)≤P(c)≤P(C)=1
Thm 1.3.5
P(C1∪C2)=P(C1)+P(C2)−P(C1∩C2)
P(C1∪C2)=P(C1)+P(C1c∩C2) ⋅⋅⋅(1)
C2=(C1∩C2)∪(C1c∩C2)
P(C2)=P(C1∩C2)+p(C1c∩C2) ⋅⋅⋅(2)
(1) - (2) -->
P(C1∪C2)−P(c2)=P(C1)−P(C1∩C2)
P(C1∪C2∪C3)=P1−P2+P3
P1=P(C1)+P(C2)+P(C3)
P2=P(C1∩C2)+P(C1∩C3)+P(C2∩C3)
P3=P(C1∩C2∩C3)
In general,
P(C1∪C2∪C3∪⋅⋅⋅Ck)=P1−P2+P3−⋅⋅⋅+(−1)k−1Pk
pi:sum of all possible intersection of i sets
*C2,C3⋅⋅⋅:Mutually exclusive
Mutually exclusive sets C1,C2,⋅⋅⋅are called Exhaaustive(만약 Ck의 합이 표본공간인 경우)
* limn→∞Cn을 쓸 수 있는 특수한 경우
(1) Countable union and Increasing sets
⋃n=1∞Cn, Ci′s:increasing set(C1⊂C2⊂C3 ⋅⋅⋅⋅)
(2) Countable union and Decreasing sets
⋂n=1∞Cn, Ci′s:decreasing set(C1⊃C2⊃C3 ⋅⋅⋅⋅)
ex) Cn= {x:0<x<1−(n+1)1}
ex) Cn= {x:0<x<1+(n+1)1}
Thm 1.3.6
{Cn}: increasing set
limn→∞P(Cn) := P(limn→∞Cn)
pf)
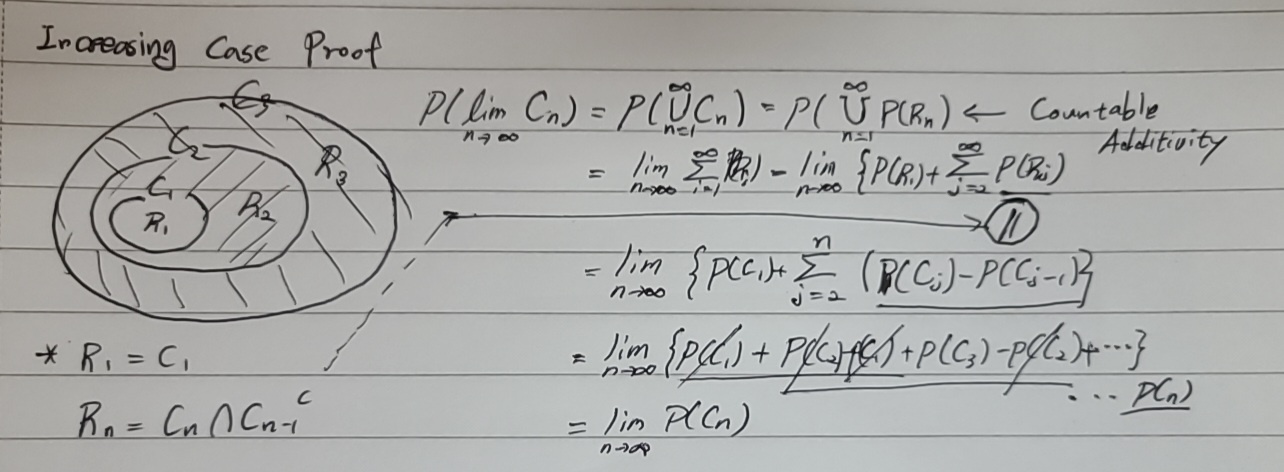
{Cn}: decreasing set
limn→∞P(Cn) := P(limn→∞Cn)
Thm 1.3.7(Boole's inequality)
{Cn}: arbitrary seg of sets
P(⋃n=1∞Cn)≤∑n=1∞P(Cn)
1.4 조건부확률과 독립성(Conditional Prob & Independence)
C1,C2∈C,
The conditional probability of C2 given C1
P(C2∣C1) := P(C1)P(C2∩C1)
∗P(C1)=ϕ
(1) P(C2∣C1)≥0(non−negativity)
(2) P(C2∣C2)=1(Normality)
(3) C2,C3,C4,⋅⋅⋅:mutually exclusive
-->P(⋃i=1∞Ci∣C1)=∑i=1∞P(Ci∣C1) <- countable additivity
ex1.4.1
포커 카드(52장)에서 비복원 추출 시, 6번째 뽑았던 것이 3번째 스페이드인 확률
--> 처음 5개 중 2개의 스페이드 출현
--> 마지막에 스페이드 출현
C1:two spades in the first five draws
C2:third spades in the sixth draws
what is P(C1∩C2)?
--> 교집합을 구하기 쉽지 않으니, 조건부확률로 구하자
P(C2∣C1) := P(C1)P(C2∩C1) >>> P(C1∩C2)=P(C2∣C1) *P(C1)
(1) P(C2∣C1)는 52개의 카드 中 5개를 뽑았는데 2개의 스페이드가 나온 상황에서 또 6번째로 스페이드를 뽑을 확률
(P(C2∣C1) = (52−5)(13−2) -> 47개의 패 중에서 스페이드를 뽑을 확률)
(2) P(C1)는 5개 뽑았는데 2개가 스페이드일 확률
P(C1)= 52C513C2
(1) * (2) = 0.064
Joint Prob을 Marginal Prob과 Conditional Prob의 곱으로 나타내기
P(C1∩C2)=P(C1)∗P(C2∣C1)
P(C3∣C1∩C2)= P(C1∩C2)P(C3∩C2∩C1)
P(C3∩C2∩C1) = P(C1∩C2)∗P(C3∣C1∩C2)
In general,
P(C1∩C2∩C3⋅⋅⋅)
= P(C1)∗P(C2∣C1)∗P(C3∣C1∩C2)⋅⋅⋅
Bayes Theorm
C1,C2,C3⋅⋅⋅: mutually exclusive & exhaustive
P(Ci)>0,∀i=1,⋅⋅⋅,k
P(Cj∣C) = ∑i=1kP(Ci)∗P(C∣Ci)P(Cj)∗P(C∣Cj)
- 좌변이 구하기 어려운 경우이거나 비현실적인 경우, 우변을 통해 우회하여 구하는 것
pf)
- P(Cj):Prior prob(사전확률)
- P(Cj∣C):Posterior prob(사후확률)
Def 1.4.1 독립의 정의(왜 독립인 두사건의 교집합은 곱으로 나타내는가)
C1,C2:(Statistical) independent iff
P(C1∣C2)=P(C1)
In general,
C1,C2,⋅⋅⋅independent
iff every collection of k event(2 ≤k≤n)
P(Ci1∩Ci2⋅⋅⋅∩Cik) = ∏j=1kP(Cij)
cf)
Ci,Cj:pairwise independent if
P(Ci∩Cj)=P(Ci)P(Cj), ∀i=j