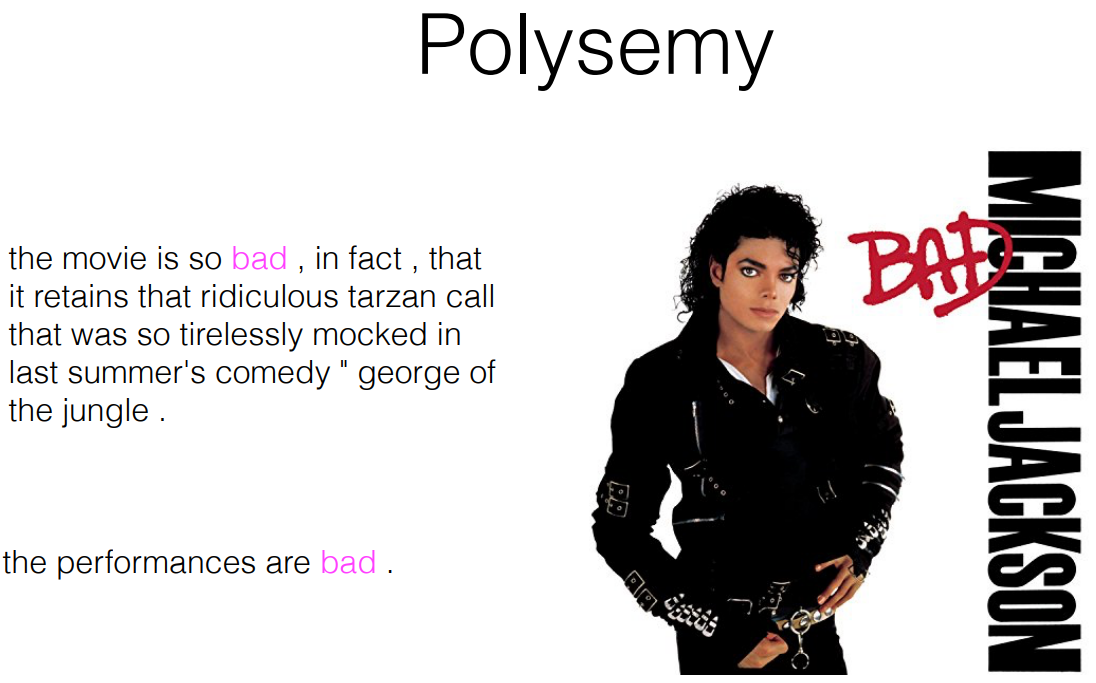
=> bad 는 좋은 의미로 쓰일 수도 있고 나쁜 의미로 쓰일 수도 있다.
여러 의미를 가지는 단어 중에 어떤 뜻을 선택할 것인가? -> Word Sense 로 파악
Word senses
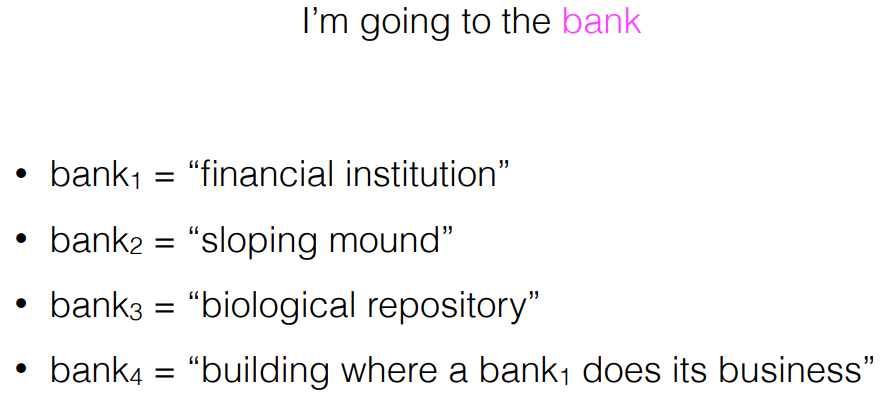
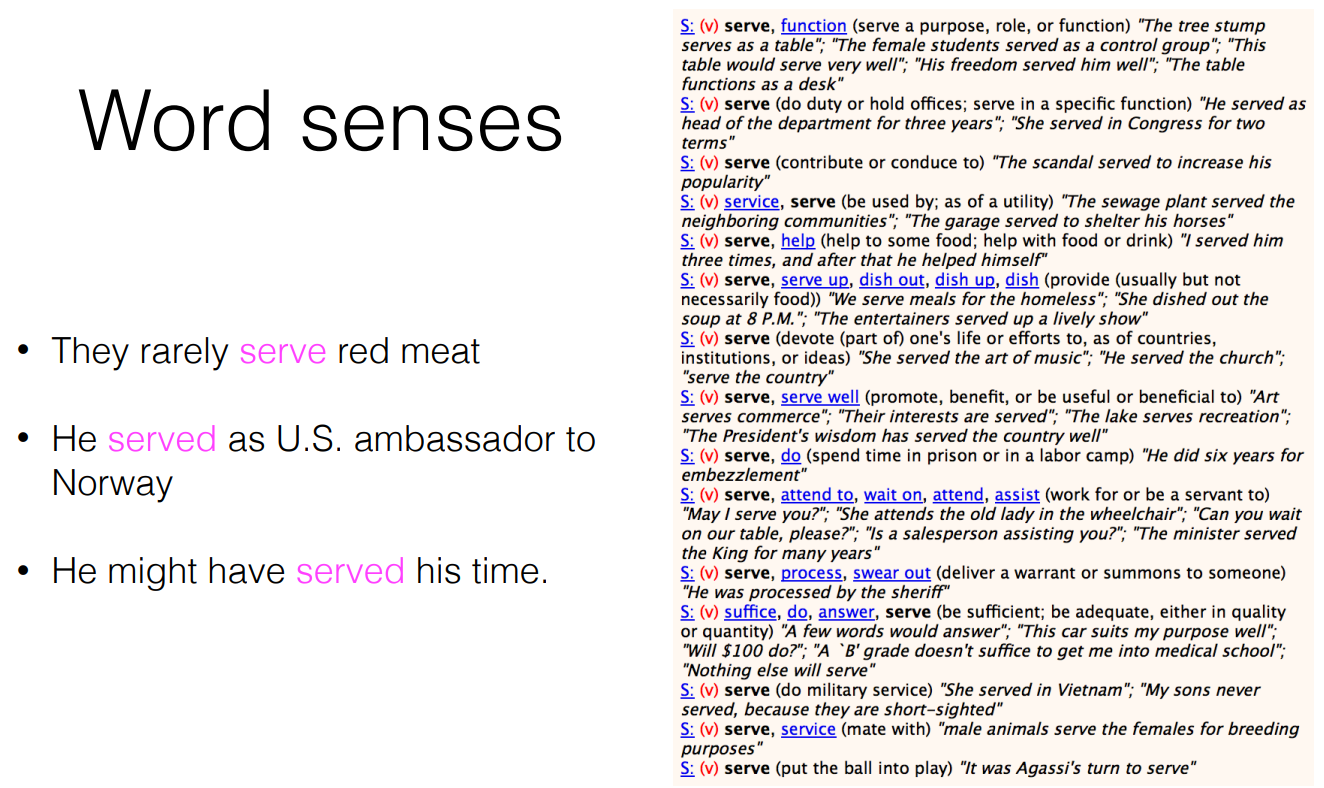
=> serve 의 여러 가지 의미 중에 무슨 의미일까? -> word sense 로 파악
Relationship between senses
-
Symomymy/antonymy (동의성/반의성)
-
Hypernymy (의미적 포함관계)
-
Metonymy (어떤 특정 단어로 전체 지칭하는 경우)
-
Meronymy (물리적 포함관계)
-
상위-하위 개념일 때, 상위개념이 하위개념을 포함
ex) bihike(상위) 와 car(하위), bike(하위)
-
전체-부분 관계일 때
ex) car(전체) 와 wheel(부분), window(부분)
Synonym
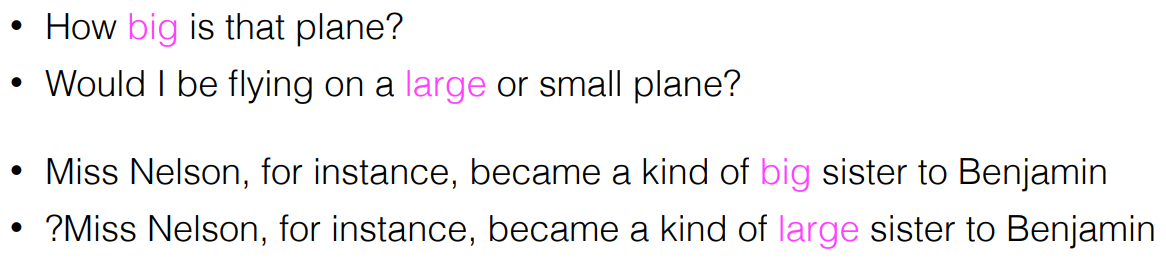
=> word sense로 big, large 중에 뭐 고를래?
- Synonymy 는 cosine similarity 가 비슷
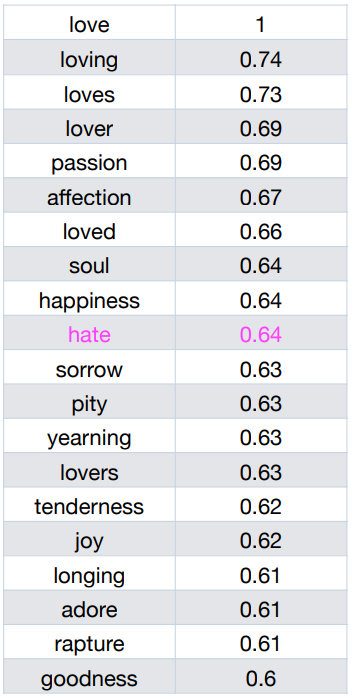
Antonymy
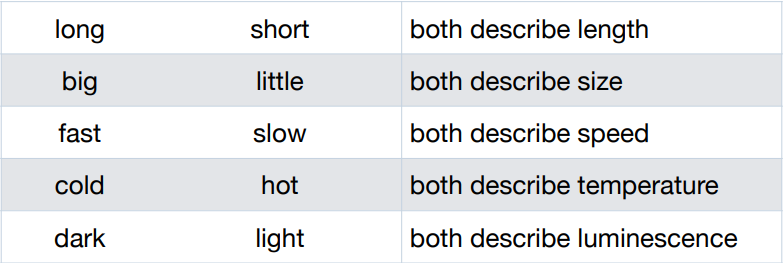
=> word sense로 long, short 중에 뭐 고를래?
Hyponymy
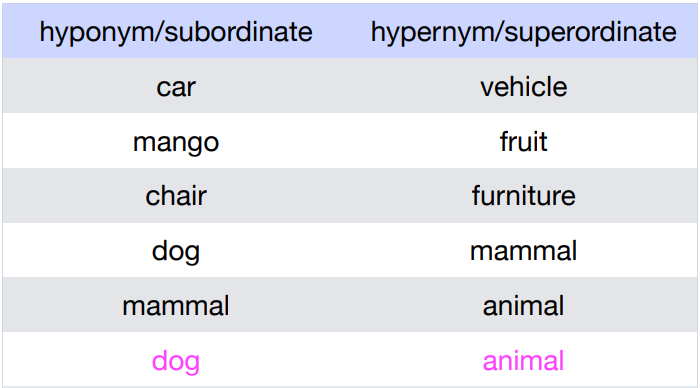
Meronymy
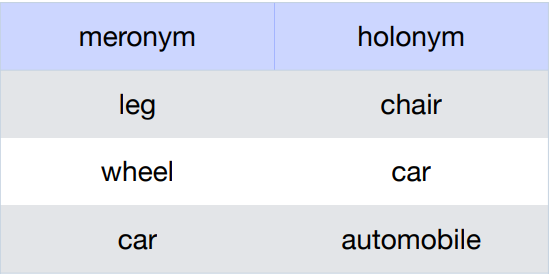
Dataset
WordNet
- 언어학자들이 명사/형용사/동사 .. 등 전부 다 분석해놓음
- Lexical database for nouns, verbs and adjectives/adverbs
- Each word sense is arranged in a synset and each synset is related to others in terms of their sense relations
Relations : word 와 word의 관계를 본다
![]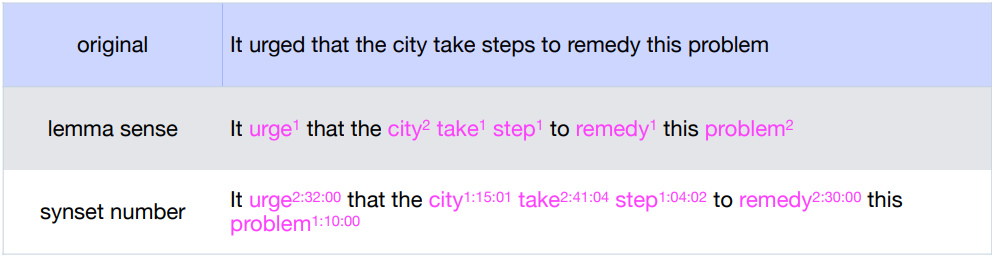
(https://velog.velcdn.com/images/passion_man/post/5d6461de-02c3-4c4c-8354-f76dcad3d028/image.png)
Synsets : synymy 기반의 data word-sense
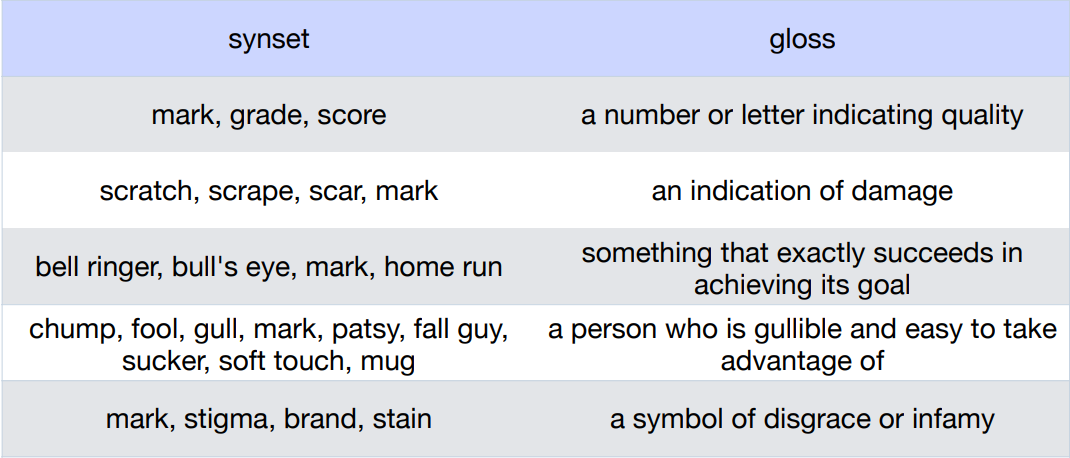
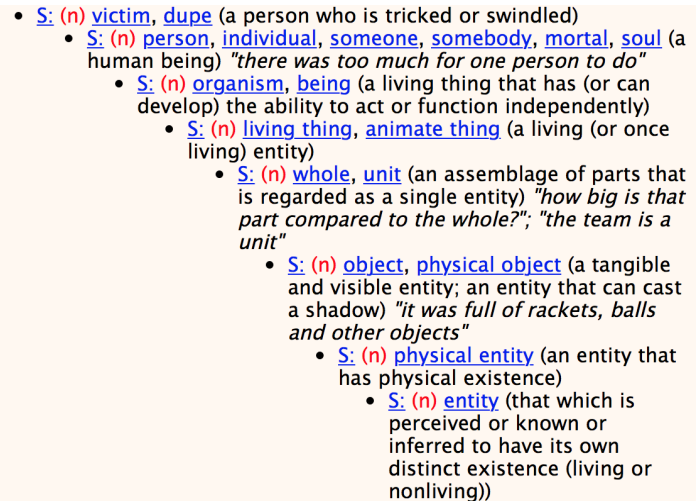
-> 위로 올라갈 수록 상위개념
WordNet
- WordNet encodes human-judged measures of similarity. Learn distributed representations of words that respect WordNet similarities -> WordNet의 similarity 를 이용해서 Word vector 를 만듬
Semcor (dataset)
- 200K + words from Brown corpus tagged with WordNet senses.
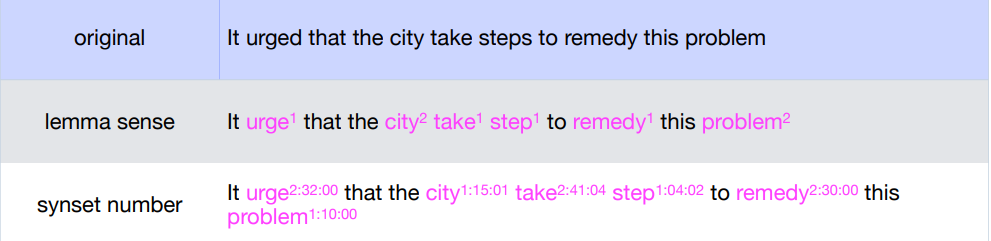
=> 위의 그림에서 숫자 1, 숫자 2는 WordNet Problem의 1번째 혹은 2번째 problem을 사용한다 라는 뜻이다.
Word Sense Disambiguation (WSD)
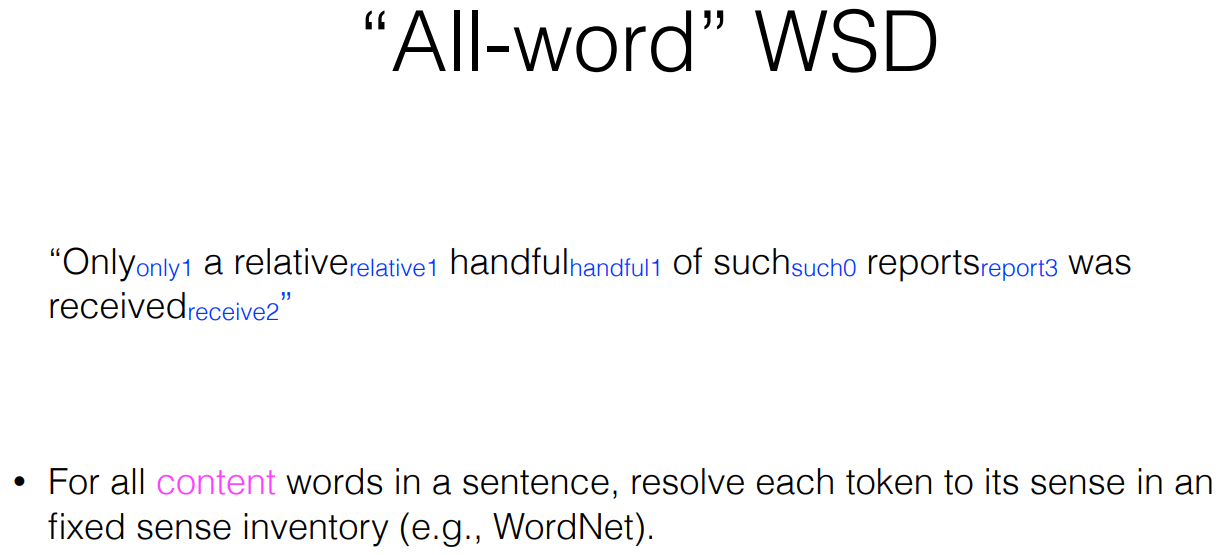
- WSD 의 여러 가지 방식
1) Dictionary methods (Lesk)
2) Supervised (machine learning)
3) Semi-supervised (Bootstrapping)
Dictionary methods
- Predict the sense a given token that has the highest overlap between the token's context and sense's dictionary gloss -> Sense1,2,3 중 classification -> context와 gloss 사이에 overlap이 가장 큰 것으로 선택
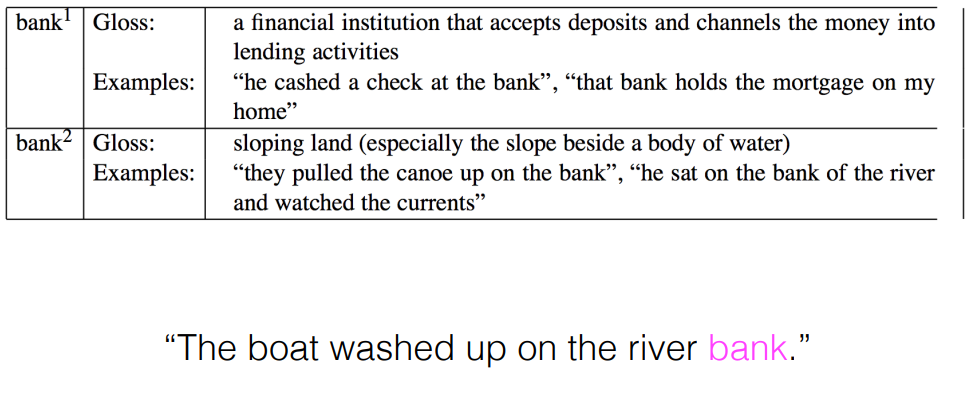
=> 각 단어 word vector를 만들어서 bank1, bank2의 word vector 중 어떤 것과 가까운지 파악한다.
Supervised WSD
- We have labeled training data; let's learn from it
- Decision trees
- Naive Bayes, long-linear classifiers, support vector machines
- Bidirectional LSTM
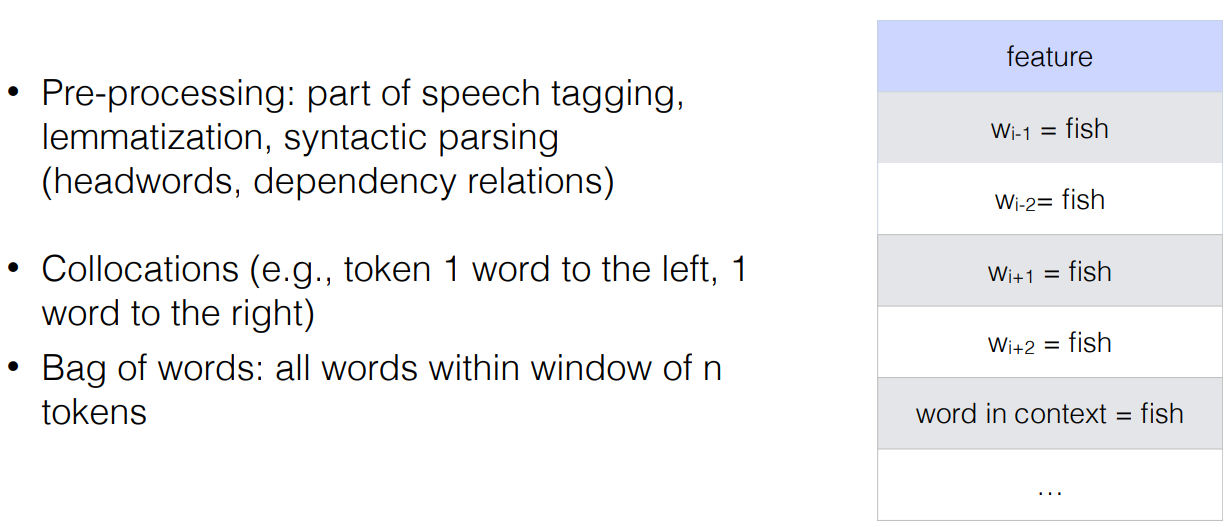
Evaluation
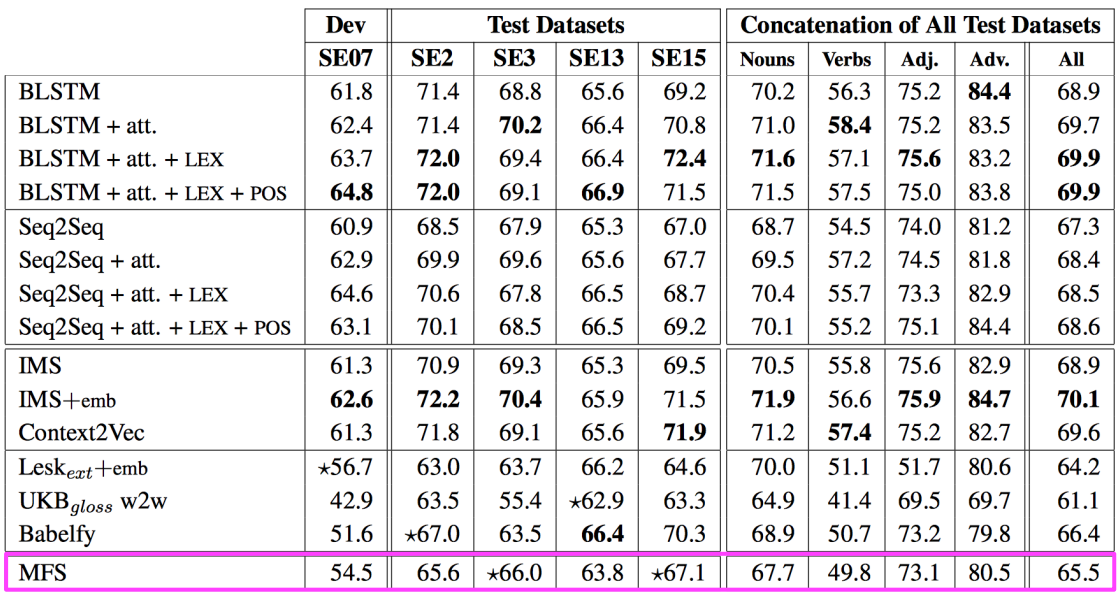
=> 맨 아랫 줄(Baseline)의 MFS는 Most Frequent Sense 로 통계적으로 가장 많이 나온 단어를 선택했을 때 결과값이다. 어떤 방식을 쓰든 이것보단 값이 높아야 한다.
if a word appears multiple times in a document, it's usually with the same sense -> 언어의 특징
ex) Articles about finacial banks don't usually don't talk about river banks
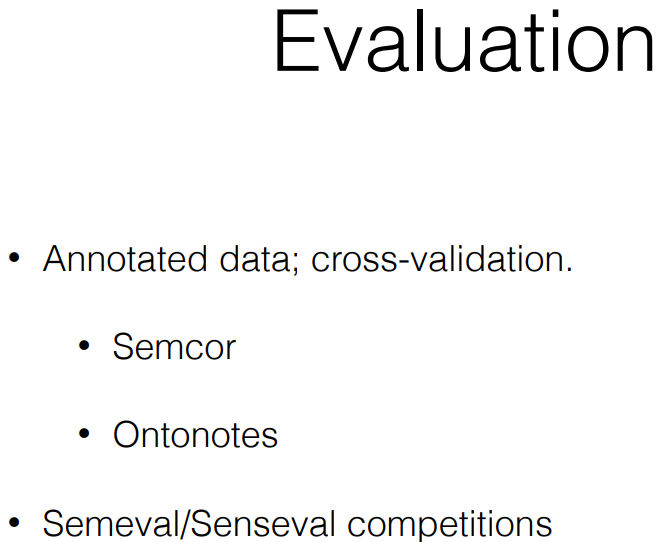
hyponymy 의 word sense
Supersense tagging
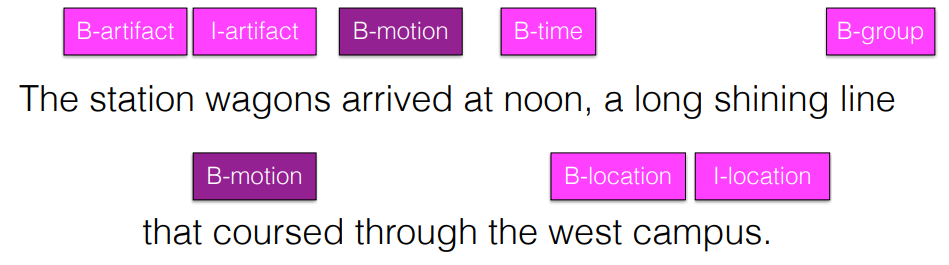
=> 하위개념을 상위개념으로 Mapping 해준다