Category of Machine Learning
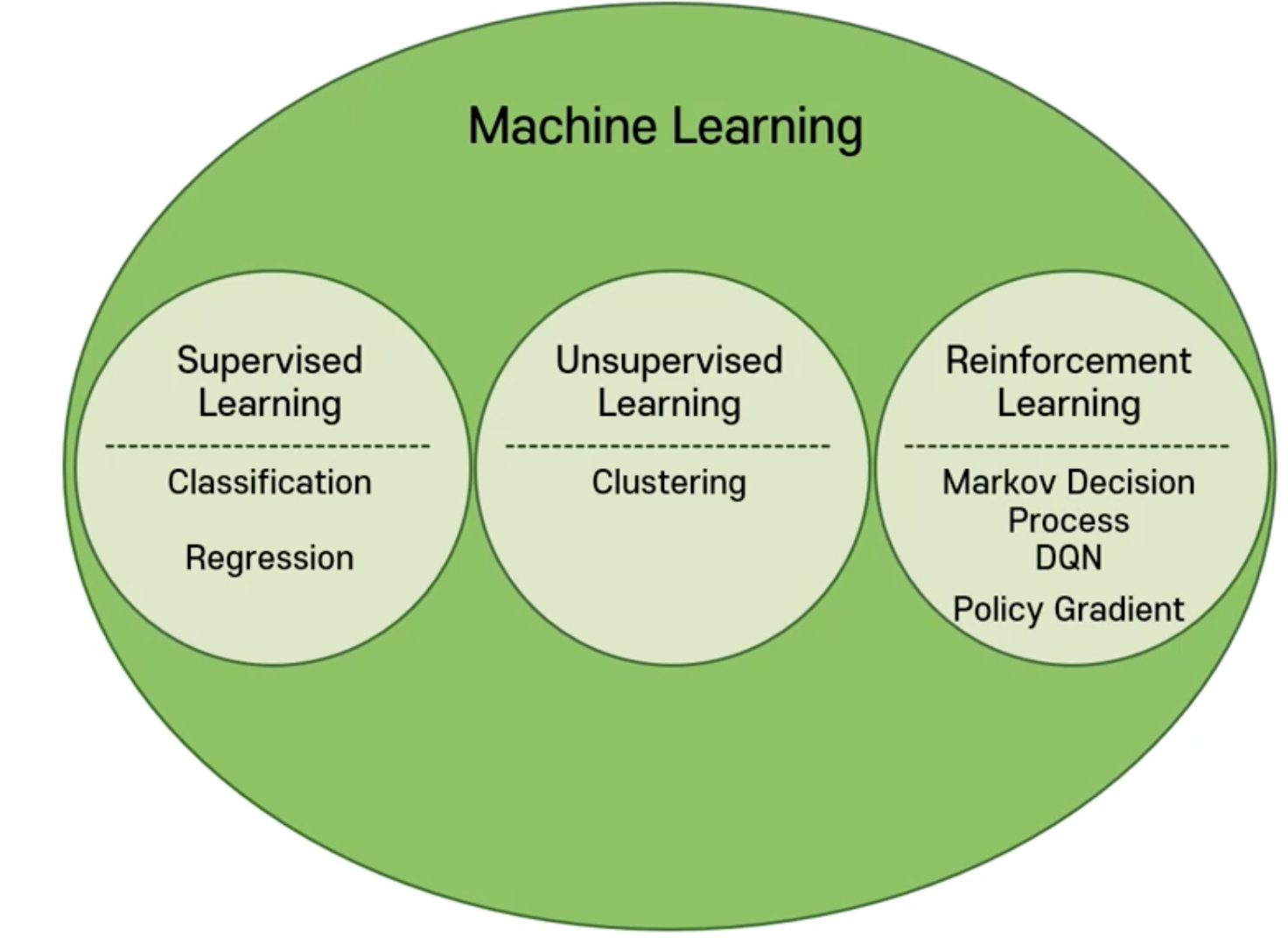
지도학습
- Classification
- Regreesion
비지도학습
강화학습
Supervised Learning
- Output y is categorical variable : Classification
- Output y is continuous : Regression
Regression
- Ouput(y) , Input(x)
- x = n*p matrix 형태
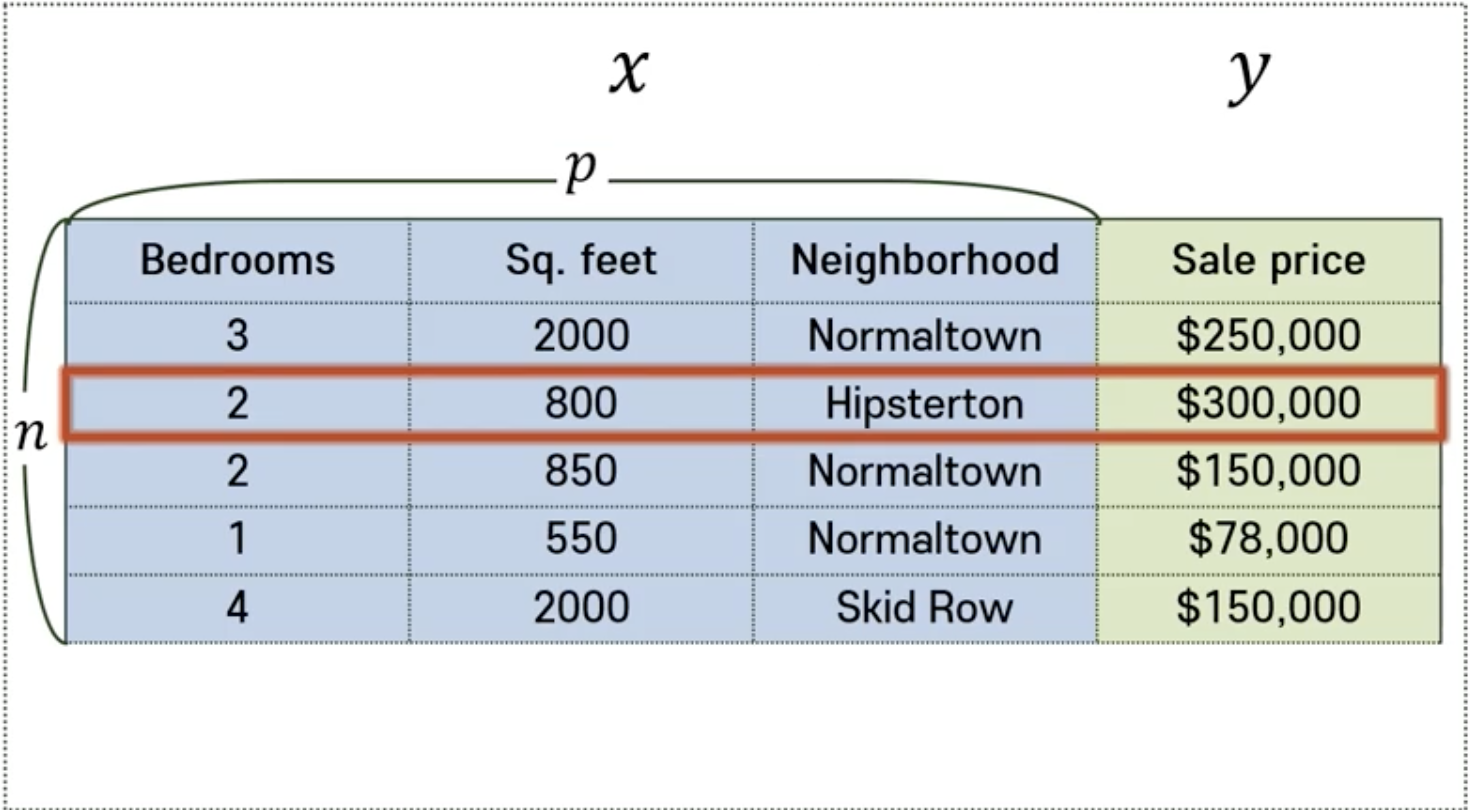
Classification
- Output(y), Input(x)
- Each image is an input and corresponding class is output
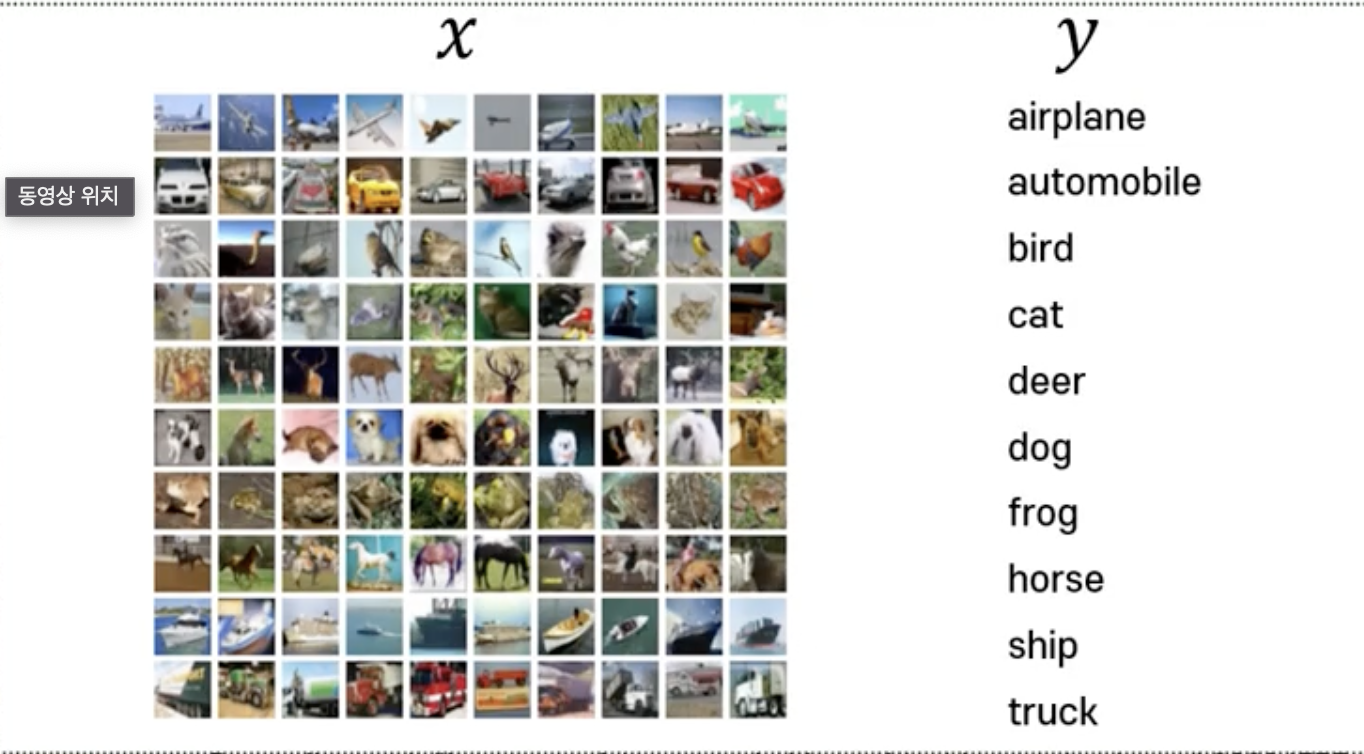
- We must train the function for appropriately demonstrating the relationship between input and output variables
- The word 'appropriately' means the difference between results from real data and model's prediction is small
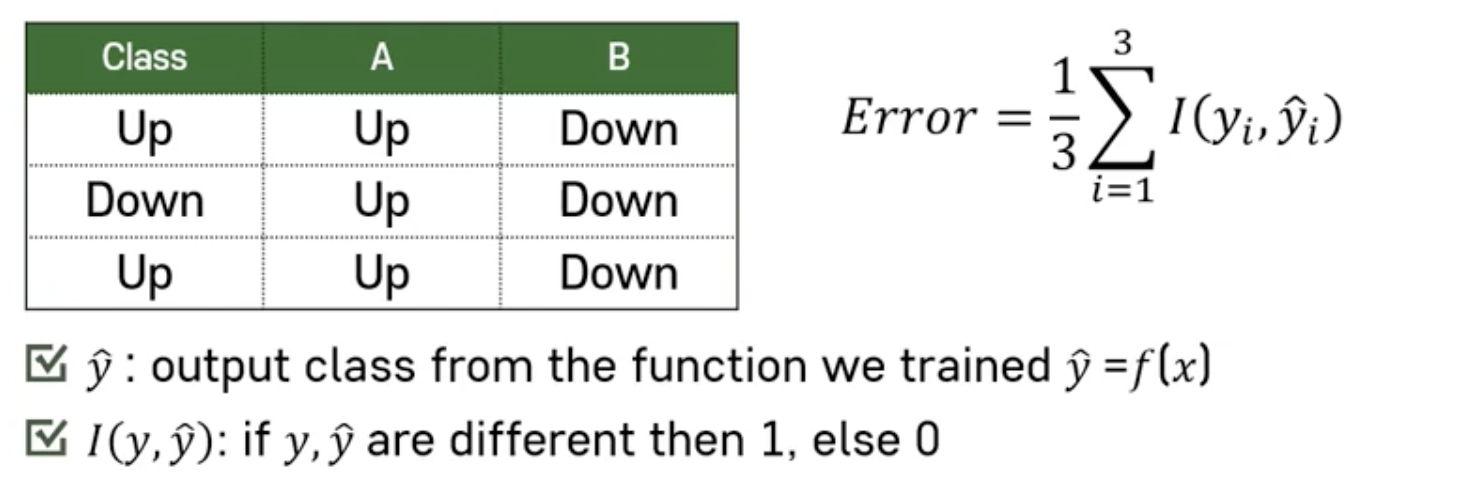
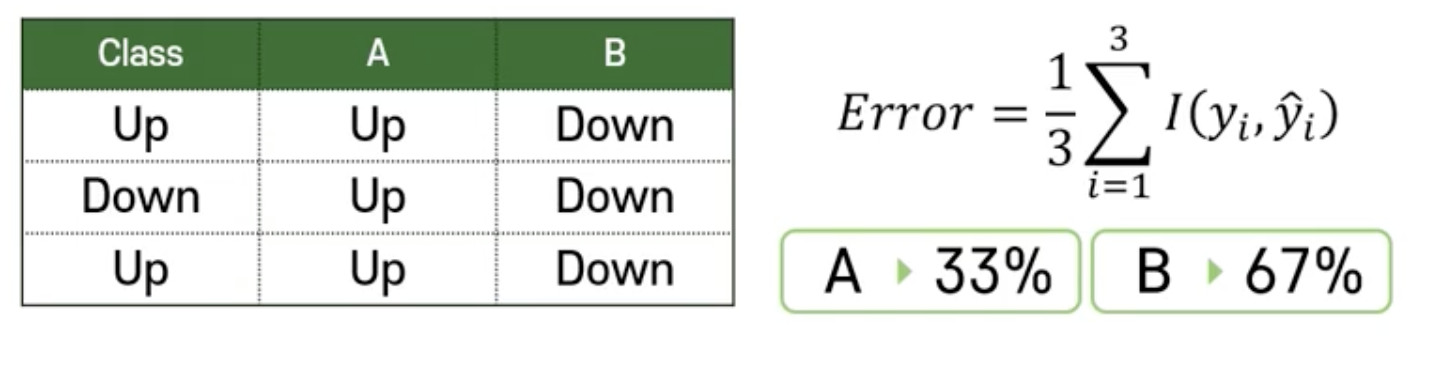
-> which model is better than the other? => A
Machine Learning and Optimization
- Machine learning models optimize the function by minimizing the loss
- The process of optimization for the machine learning functions represents learning
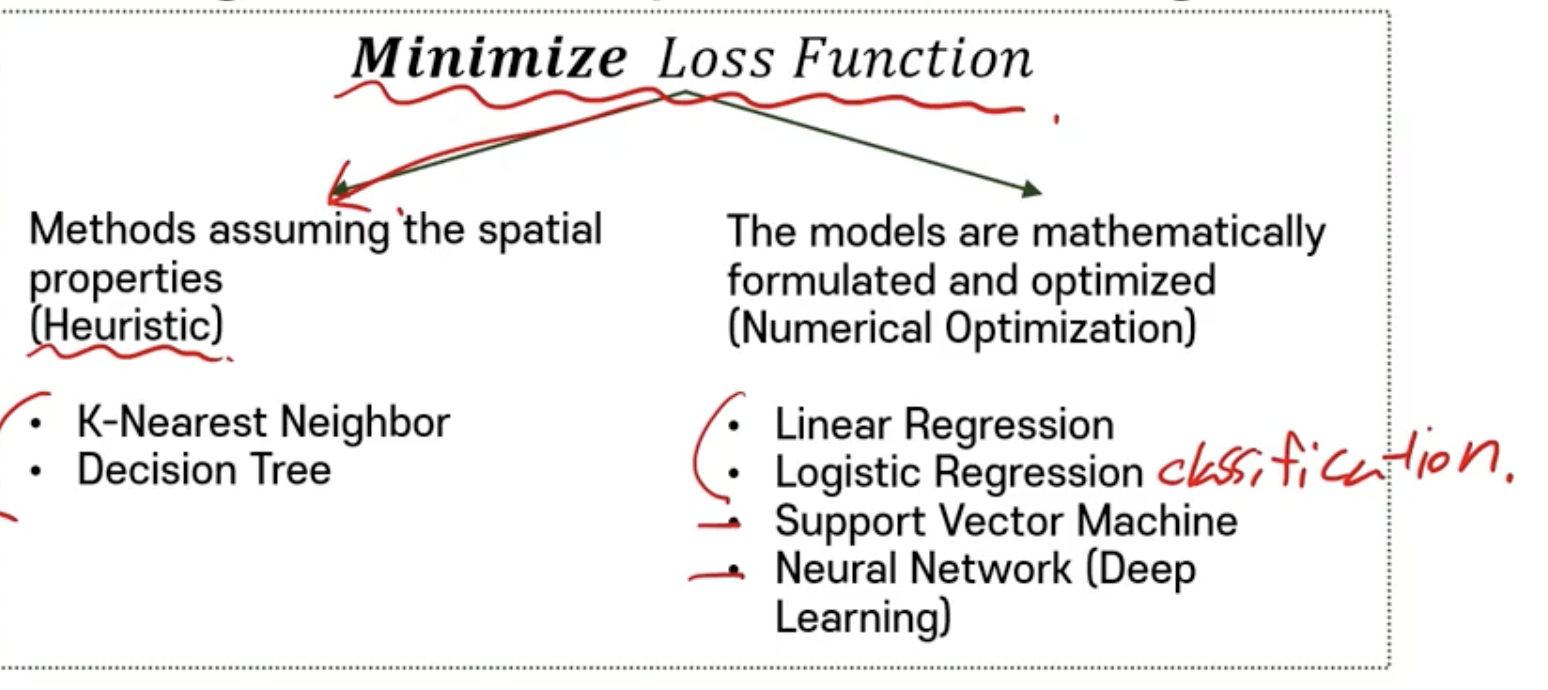
Generalization Error and Hyperparameter
-
Training error
- prediction error to training data(Loss)
-
Validation error
- prediction error to validation data(Generalizaion error)
-
As the complexity of the model increases,
the training error decreases but the validation error increases from a critical point 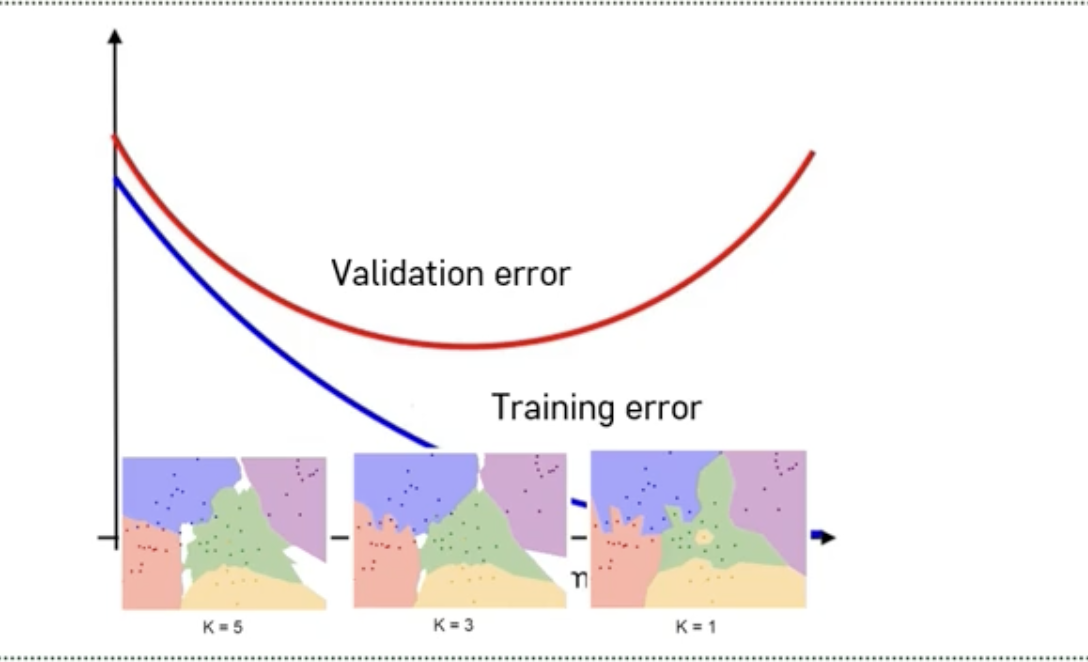
-
Machine learning model's goal is predicting unseen data accurately
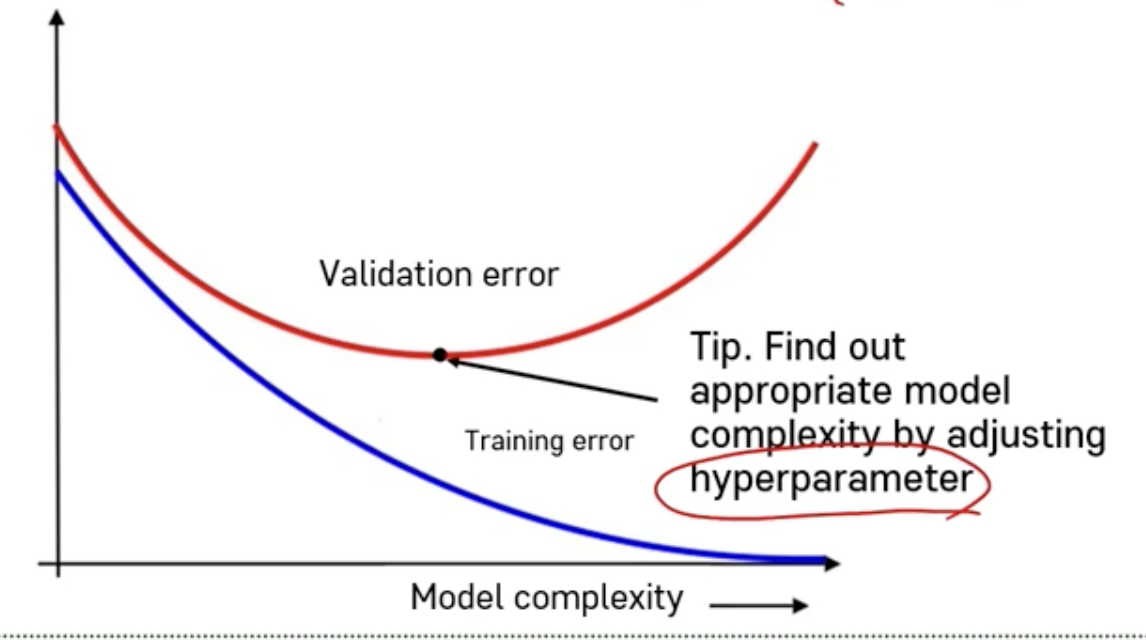
Model Validation with Data
- Validation with training data only (Bad)
- Training, Testing data (After parameter tuning)
- Training, Validation, Testing data (Best)
- Cross-Validation(with small number of data)
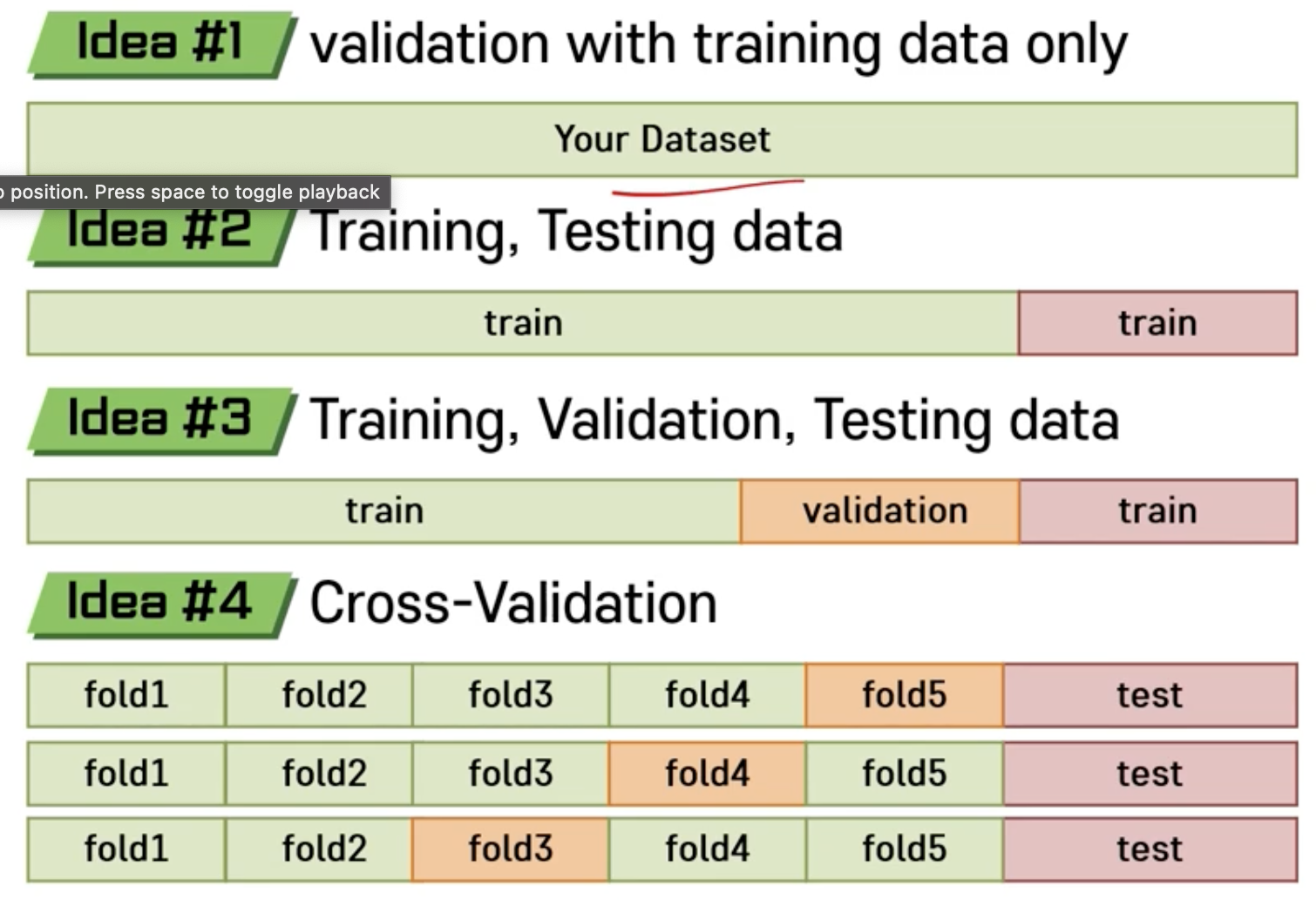
reference : K-MOOC 실습으로 배우는 머신러닝