1. 와인데이터 받아오기
import pandas as pd
red_url = 'https://raw.githubusercontent.com/PinkWink/ML_tutorial/master/dataset/winequality-red.csv'
white_url = 'https://raw.githubusercontent.com/PinkWink/ML_tutorial/master/dataset/winequality-white.csv'
red_wine = pd.read_csv(red_url, sep= ';')
white_wine = pd.read_csv(white_url, sep= ';')
red_wine['color'] = 1.
white_wine['color'] = 0.
wine = pd.concat([red_wine, white_wine])
X = wine.drop(['color'], axis=1)
y = wine['color']
2. 레드/화이트 와인 분류기의 동작 Process
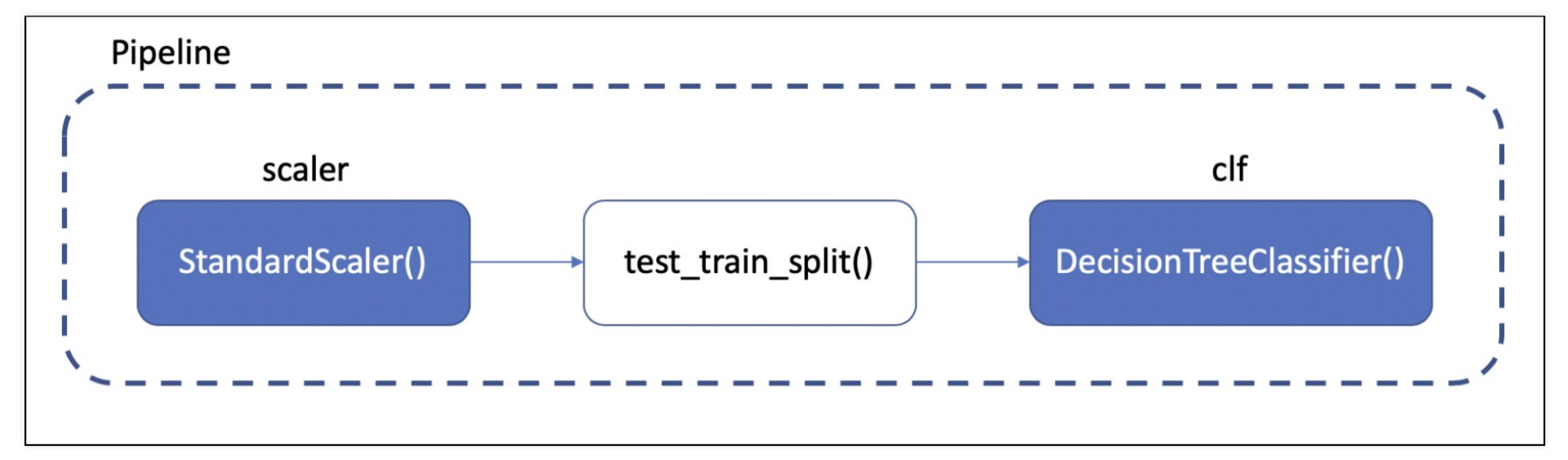
- 여기서 test_train_split은 Pipeline 내부가 아니다.
3. 위 그림을 Pipeline을 코드로 구현
from sklearn.pipeline import Pipeline
from sklearn.tree import DecisionTreeClassifier
from sklearn.preprocessing import StandardScaler
estimators = [
('scaler', StandardScaler()),
('clf', DecisionTreeClassifier())
]
pipe = Pipeline(estimators)
pipe.steps
# [('scaler', StandardScaler()), ('clf', DecisionTreeClassifier())]
4. set_params
pipe.set_params(clf__max_depth = 2)
pipe.set_params(clf__random_state=13)
# Pipeline(steps=[
('scaler', StandardScaler()),
('clf', DecisionTreeClassifier(max_depth=2, random_state=13))
])
5. Pipeline을 이용한 분류기 구성
from sklearn.model_selection import train_test_split
X_train,X_test,y_train,y_test = train_test_split(X,y, test_size=0.2, random_state=13, stratify=y)
pipe.fit(X_train, y_train)
# 성능 확인
from sklearn.metrics import accuracy_score
y_pred_tr = pipe.predict(X_train)
y_pred_test = pipe.predict(X_test)
print('train Acc : ',accuracy_score(y_train, y_pred_tr))
print('test Acc : ',accuracy_score(y_test, y_pred_test))
# train Acc : 0.9657494708485664
# test Acc : 0.9576923076923077