TensorFlow
1. TensorFlow Basic
TensorFlow
- An open source software library for numerical computation using data flow
graphs
- Python! 기반
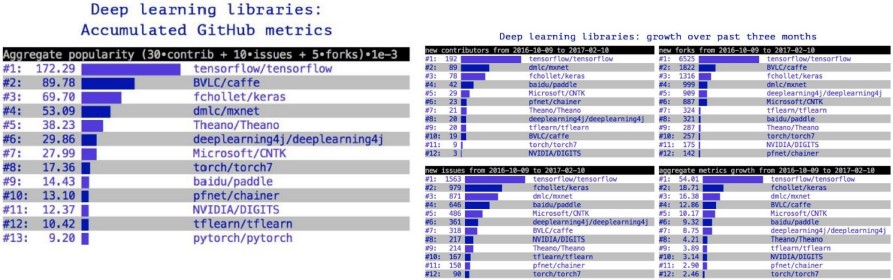
Data Flow Graph Computation
- Data Flow Graph
• 노드를 연결하는 엣지가 데이터를 노드는 데이터를 통해 수행하는 연산 역할을 하는 그래프 구조를 의미합니다.
- 데이터가 edge역할을 하여 node로 흘러가는 그래프 구조를 가지며 node에 지정된 연산을 하는 연산 방법입니다.
- 텐서플로우의 경우 이름에서 알 수 있듯 텐서(Tensors)가 기본 자료구조이기 때문에 텐서플로우의 엣지(Edge)는 텐서를 의미하며 엣지의 방향은 텐서의 흐름을 의미하고 노드(Node)는 곱하고, 나누는 등 텐서를 처리하는 연산을 의미합니다.
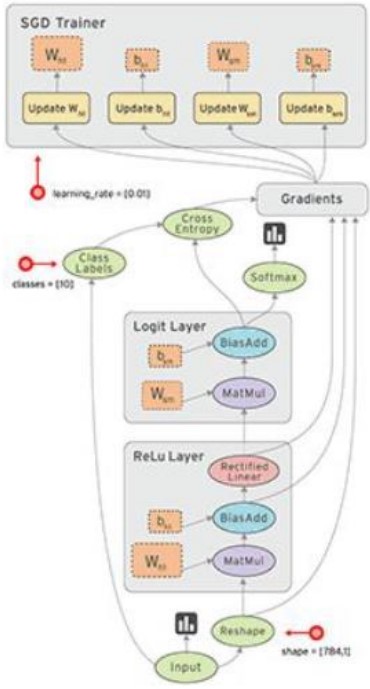
TensorFlow 설치
# 관리자모드에서
pip install tensorflow
pip install –upgrade tensorflow
# 위 방법이 안될 시
conda install tensorflow
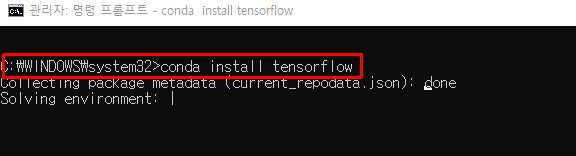
버전 확인
# 버전 확인
import tensorflow as tf
tf.__version__
example
# Tensorflow2 버전 설치 후 버전1 사용
import tensorflow.compat.v1 as tf
tf.disable_v2_behavior()
hello=tf.constant("Hello, TensorFlow")
sess=tf.Session()
print(sess.run(hello))
Tensors : Everything is Tensor
# a rank 0 tensor; this is a scalar with shape []
3
# a rank 1 tensor; this is a vector with shape [3]
[1. ,2., 3.]
# a rank 2 tensor; a matrix with shape [2, 3]
[[1., 2., 3.], [4., 5., 6.]]
# a rank 3 tensor with shape [2, 1, 3]
[[[1., 2., 3.]], [[7., 8., 9.]]]

Computational Graph
node1 = tf.constant(3.0, tf.float32)
node2 = tf.constant(4.0) # also tf.float32 implicitly
node3 = tf.add(node1, node2)
print("node1:", node1, "node2:", node2)
print("node3: ", node3)

sess = tf.Session()
print("sess.run(node1, node2): ", sess.run([node1, node2]))
print("sess.run(node3): ", sess.run(node3))

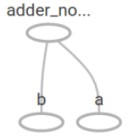
TensorFlow Mechanics
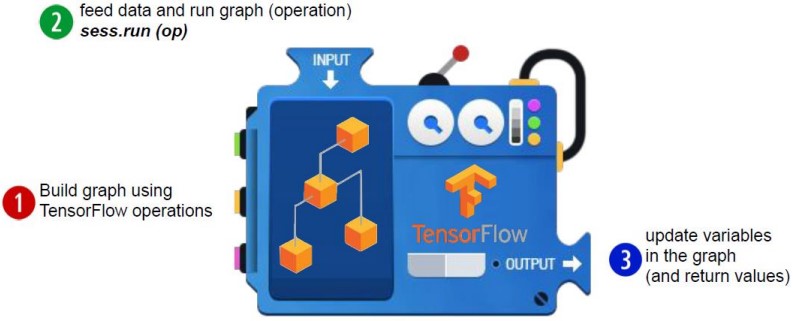
Placeholder
a = tf.placeholder(tf.float32)
b = tf.placeholder(tf.float32)
adder_node = a + b
print(sess.run(adder_node, feed_dict={a:3, b: 4.5}))
print(sess.run(adder_node, feed_dict={a:[1, 3, 4], b: [2, 4, 10]}))
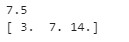
add_and_triple = adder_node * 3.
print(sess.run(add_and_triple, feed_dict={a:3, b: 4.5}))
print(sess.run(add_and_triple, feed_dict={a:[1, 3, 4], b: [2, 4, 10]}))
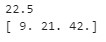
Tensor Ranks, Shapes, and Types : Ranks
- t = [[1, 2, 3], [4, 5, 6], [7, 8, 9]]
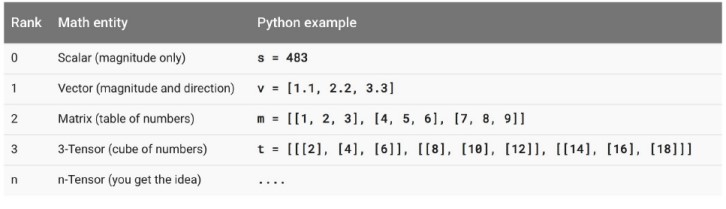
Tensor Ranks, Shapes, and Types : shapes
- t = [[1, 2, 3], [4, 5, 6], [7, 8, 9]]
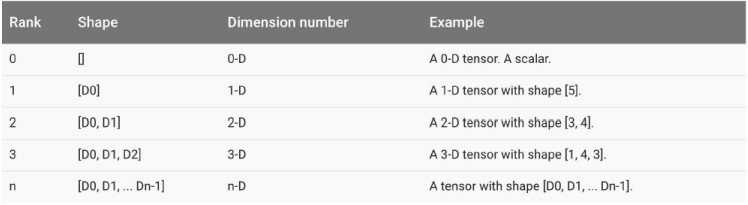
Tensor Ranks, Shapes, and Types : type
- t = [[1, 2, 3], [4, 5, 6], [7, 8, 9]]
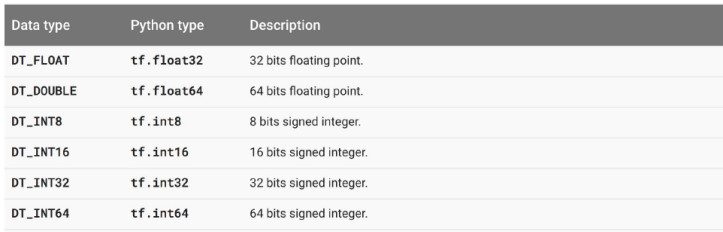
TensorFlow 2 - Deep Learning Libray
TensorFlow2
- 텐서(Tensor)를 흘려 보내면서(Flow) 딥러닝 알고리즘을 수행하는 라이브러리입니다.
- 2020년 현재 전 세계에서 자장 많이 사용됩니다.
- TensorFlow 2.0부터 직관적이고 쉽게 배울 수 있는 Keras를 High-Level API로 공식 지원함으로서, 이러한 영향력은 더욱 커질 것으로 예상됩니다.
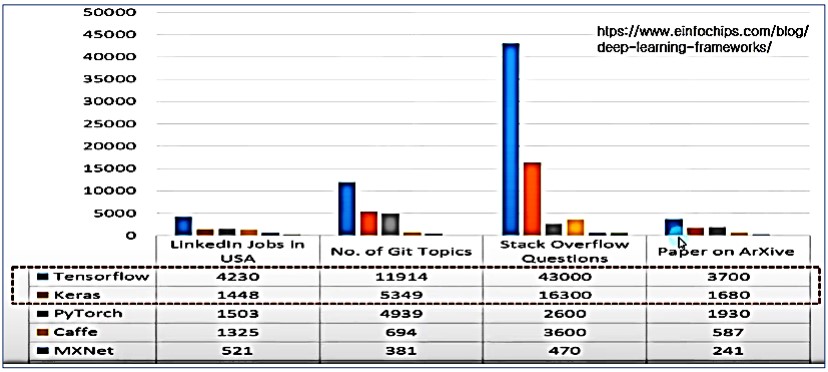
- 2019년 9월 30일에 TensorFlow 2.0 정식 Release 됨
- 즉시 실행 모드로 불리는 Eager Execution 적용되어 코드의 직관성이 높아졌으며
- 사용자 친화적이여서 쉽게 배울 수 있는 Keras만을 High-Level API로 공식 지원함
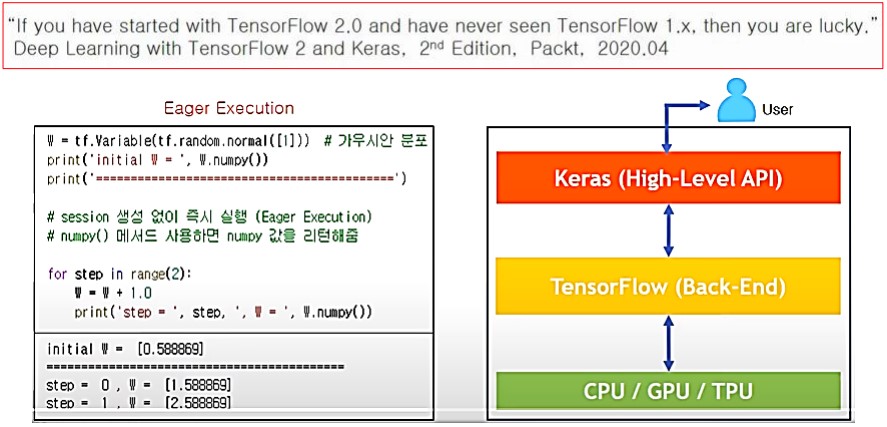
3. Eager Execution
Eager Execution(즉시 실행모드)
- 계산 그래프와 세션을 생성하지 않고 즉시 실행 가능한 Eager Execution 적용
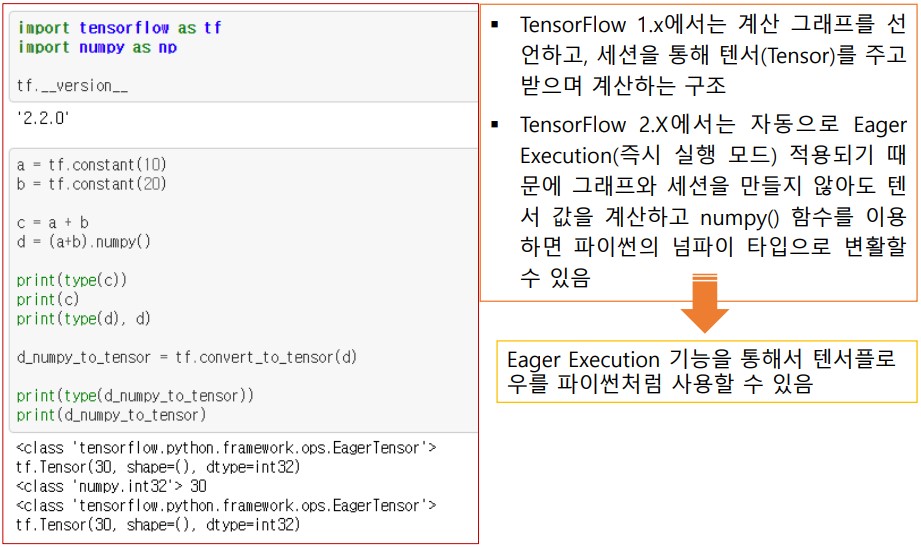
- TensorFlow 2.0에서는 오퍼레이션을 실행하는 순간 연산이 즉시 수행(Eager Execution)되기 때문에 오퍼레이션 실행결과를 numpy() 메서드를 통하여 바로 알 수 있다.
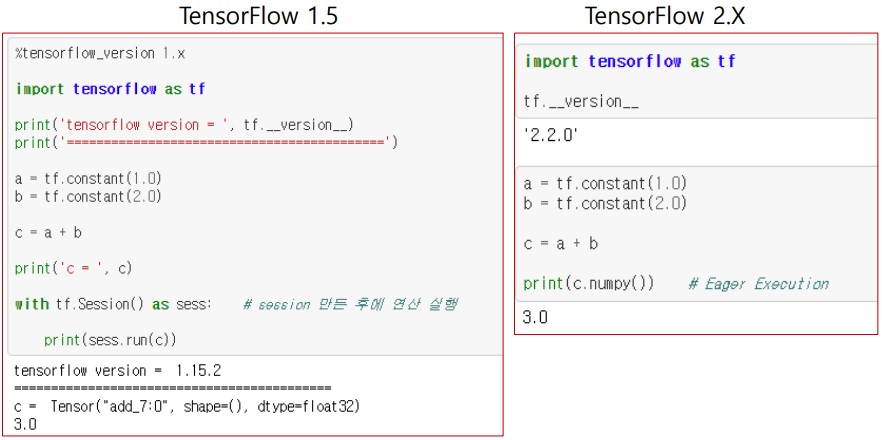
tf.Variable(…)
- TensorFlow에서 tf.Variable()값을 토기화하기 위해 세션내에서
tf.global_varivable_initializer() 과정이 필요 없으며, 변수를 정의함과 동시에 초기 값
이 할당됨(Eager Execution)
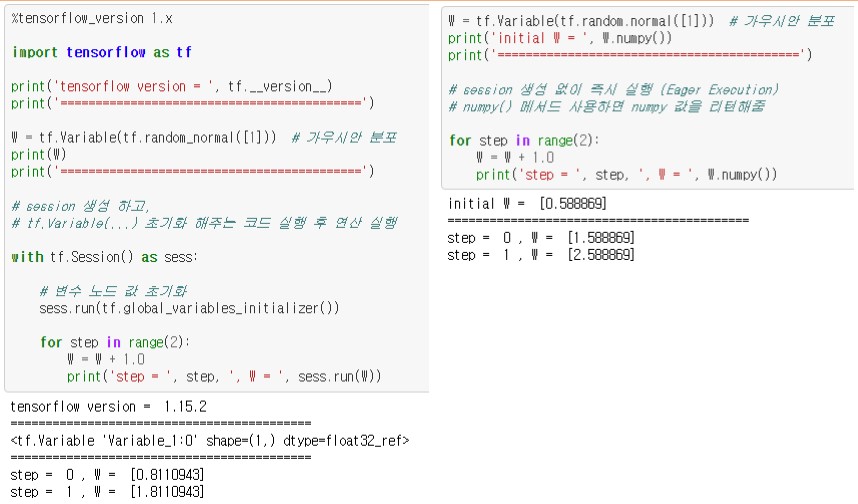
tf.placeholder(…) 삭제
- TensorFlow 1.x 버전에서 함수를 실행하여 결과를 얻기 위해서는 tf.placeholder()
에 입력 값을 주고, 그 값을 이용하여 함수에서 정의된 연산을 실행하였으니, TF 2.0
에서는 일반적인 python 코드와 마찬가지로 함수에 값을 직접 넘겨주고 즉시 결과를 얻을 수 있음(Eager Execution)
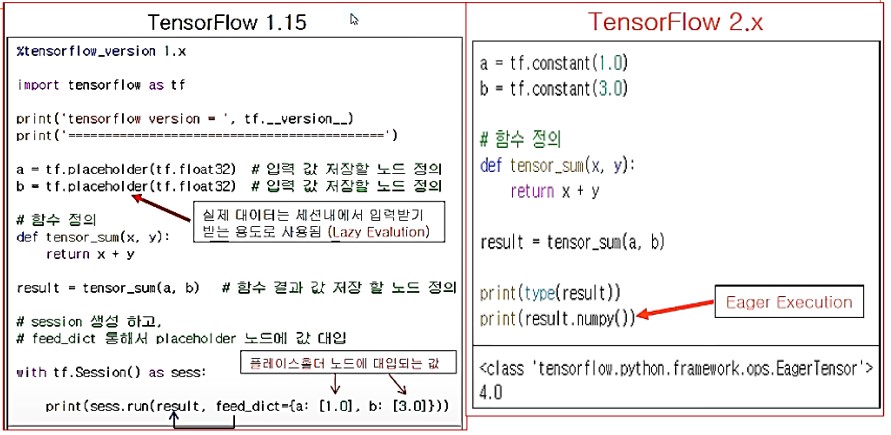
4. Keras as High Level API
Keras in TensorFlow 2.0
- Keras 창시자 프랑소와 솔레(Franceis Chollet)가 TF2.0 개발에 참여하였고, TF 2.0에서 공식적이고 유일한 High-Level API로서 keras를 채택함
- 프랑소와 숄레는 앞으로 native Keras 보다 tf.keras처럼 TF에서 케라스를 사용할 것을 권장함
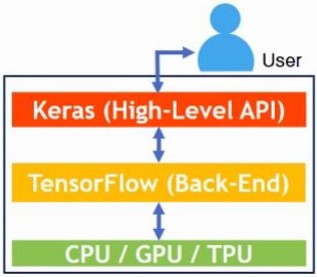
Keras 특징
- User Friendliness : Keras의 직관적인 API를 이용하면 일반 신경망(ANN), CNN, RNN 모델 또는 이를 조합한 다양한 딥러닝 모델을 (몇 줄의 코드만으로) 쉽게 구축 할 수 있음
- Modularity : Keras에서 제공하는 모듈은 독립적으로 설정 가능함, 즉 신경망 층, 손실함수, 활성화 함수, 최적화 알고리즘 등은 모두 독립적인 모듈이기 때문에 이러한 모듈을 서로 조합하기만 하면 새로운 딥러닝 모델을 쉽고 빠르게 만들어서 학습 시킬 수 있음
Keras 가장 핵심적인 데이터 구조 모델
Keras
Keras - 모델(Model)
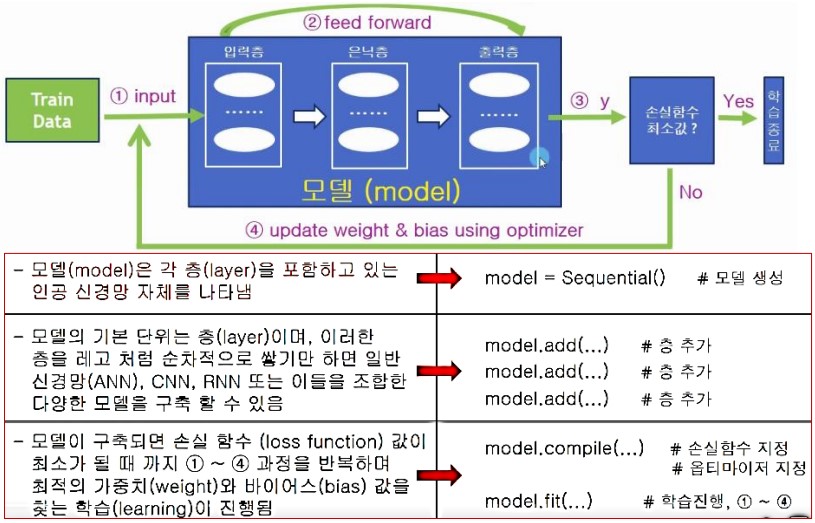
Keras - 개발과정(데이터 생성)
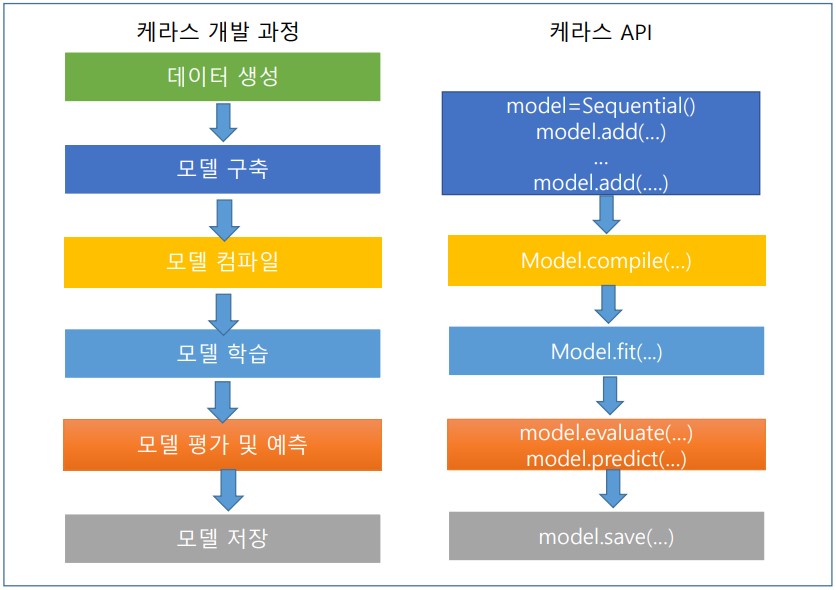
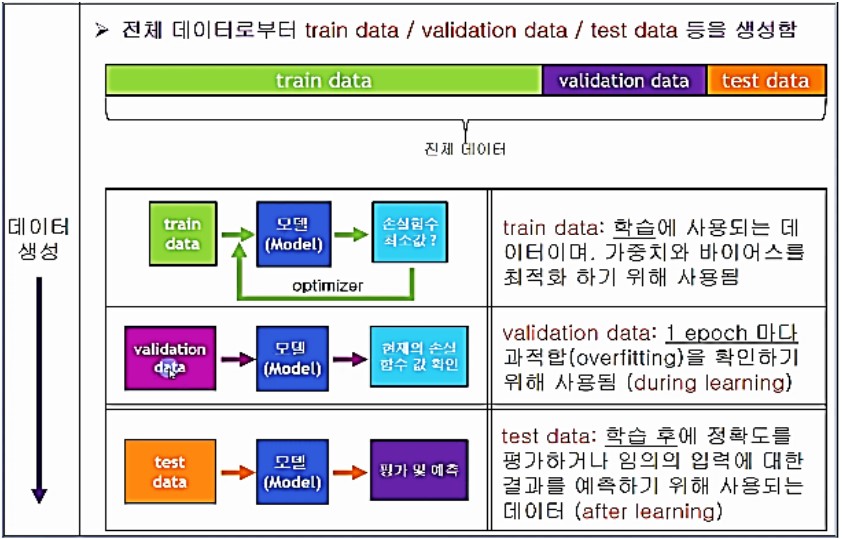
Keras - 개발과정(모델 구축)
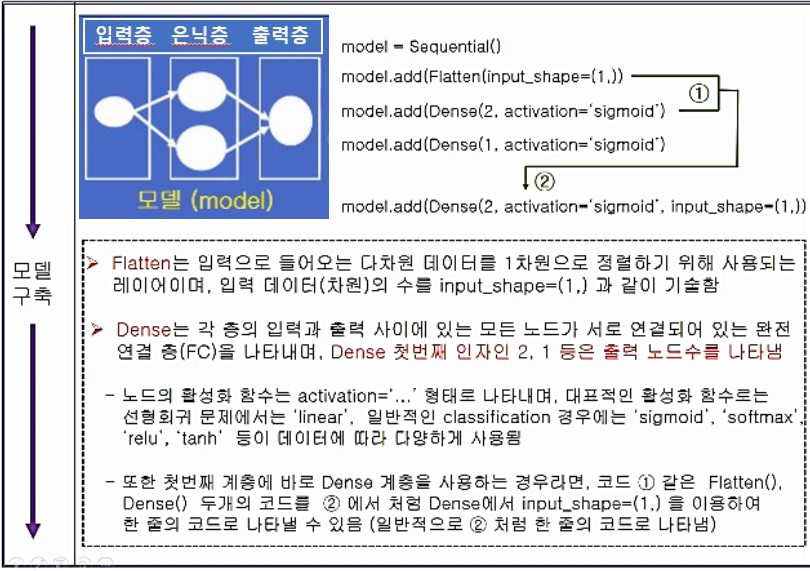
Keras - 개발과정(컴파일링, 모델학습)
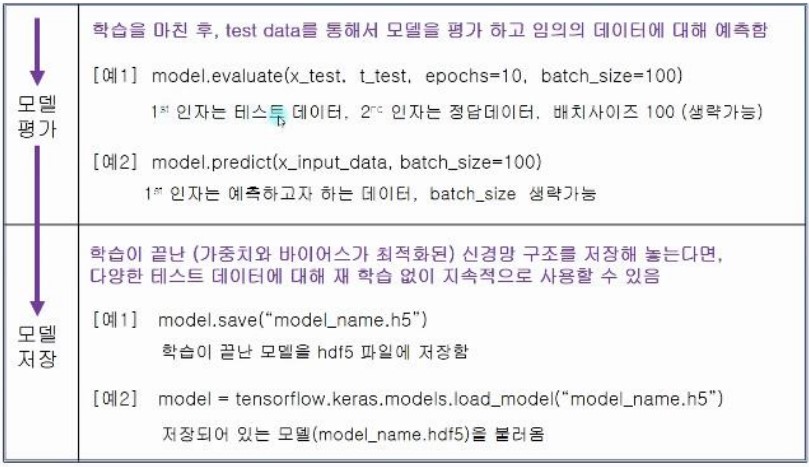
5. Keras-Simple LinearRegression Exercis
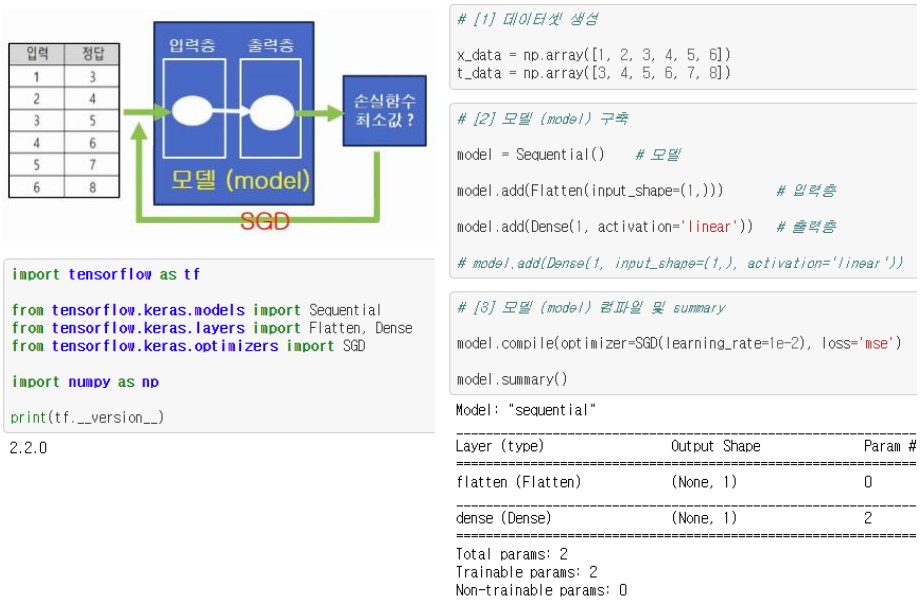
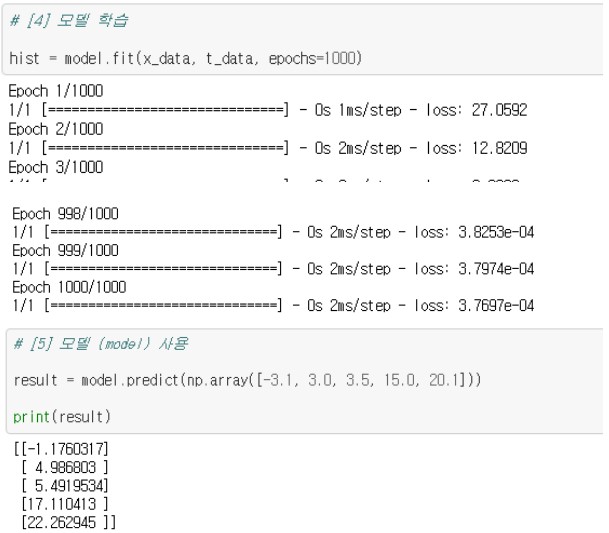