Introduction to Time Series and Forecasting
Peter J. Brockwell, Richard A. Davis 를 바탕으로 작성되었습니다.
Definition of time series model
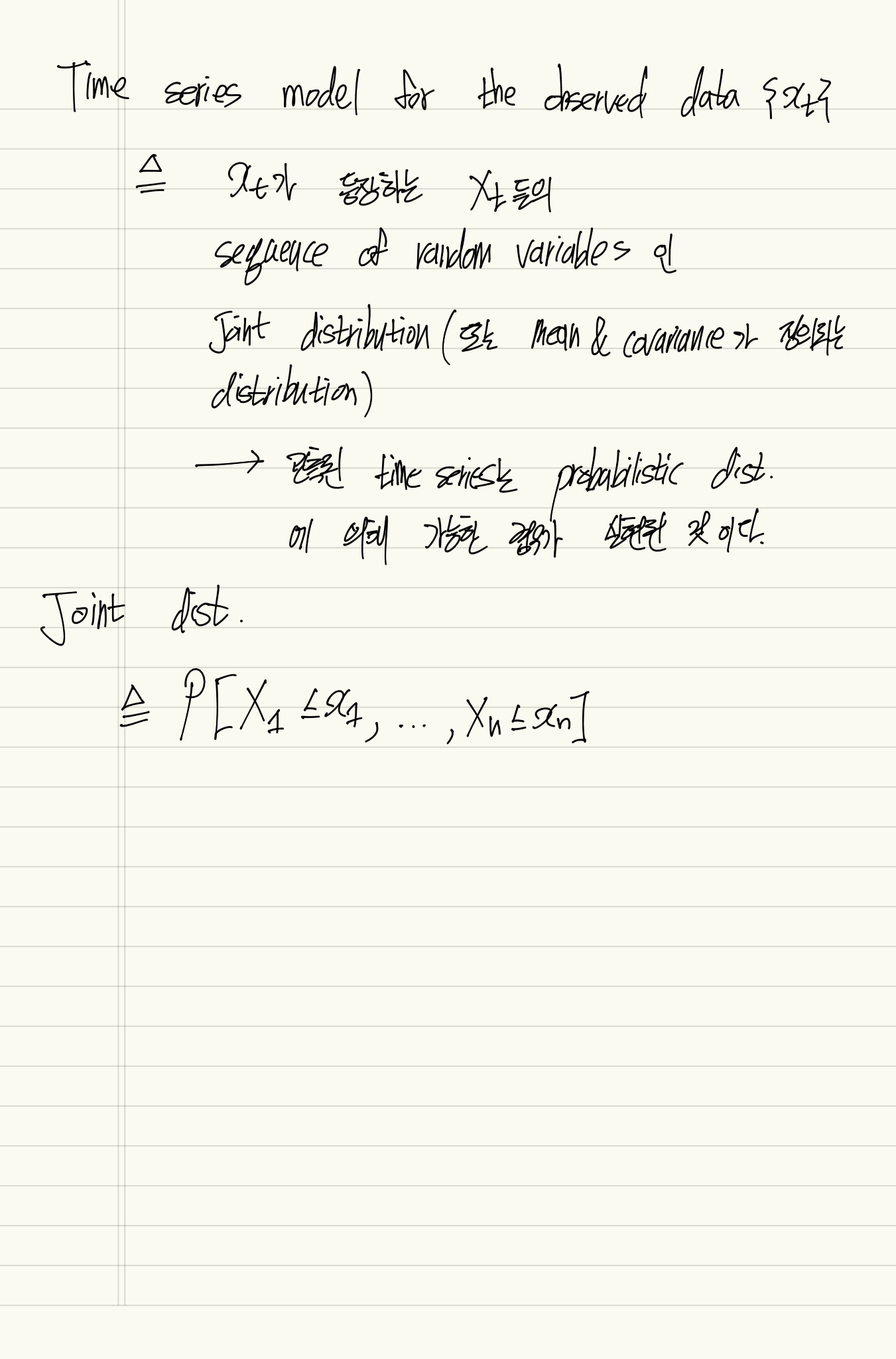
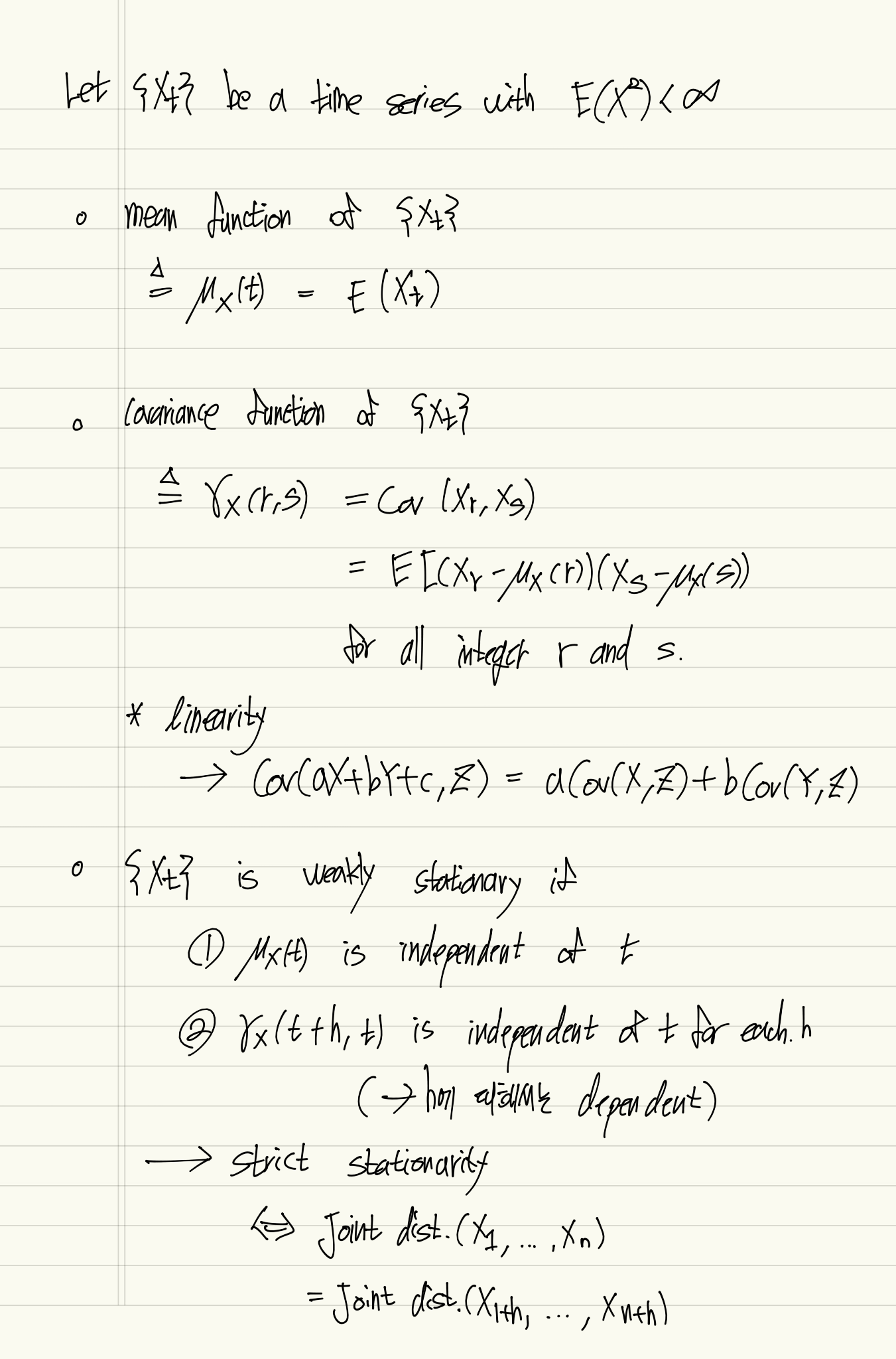
A General Approach to time series modelling
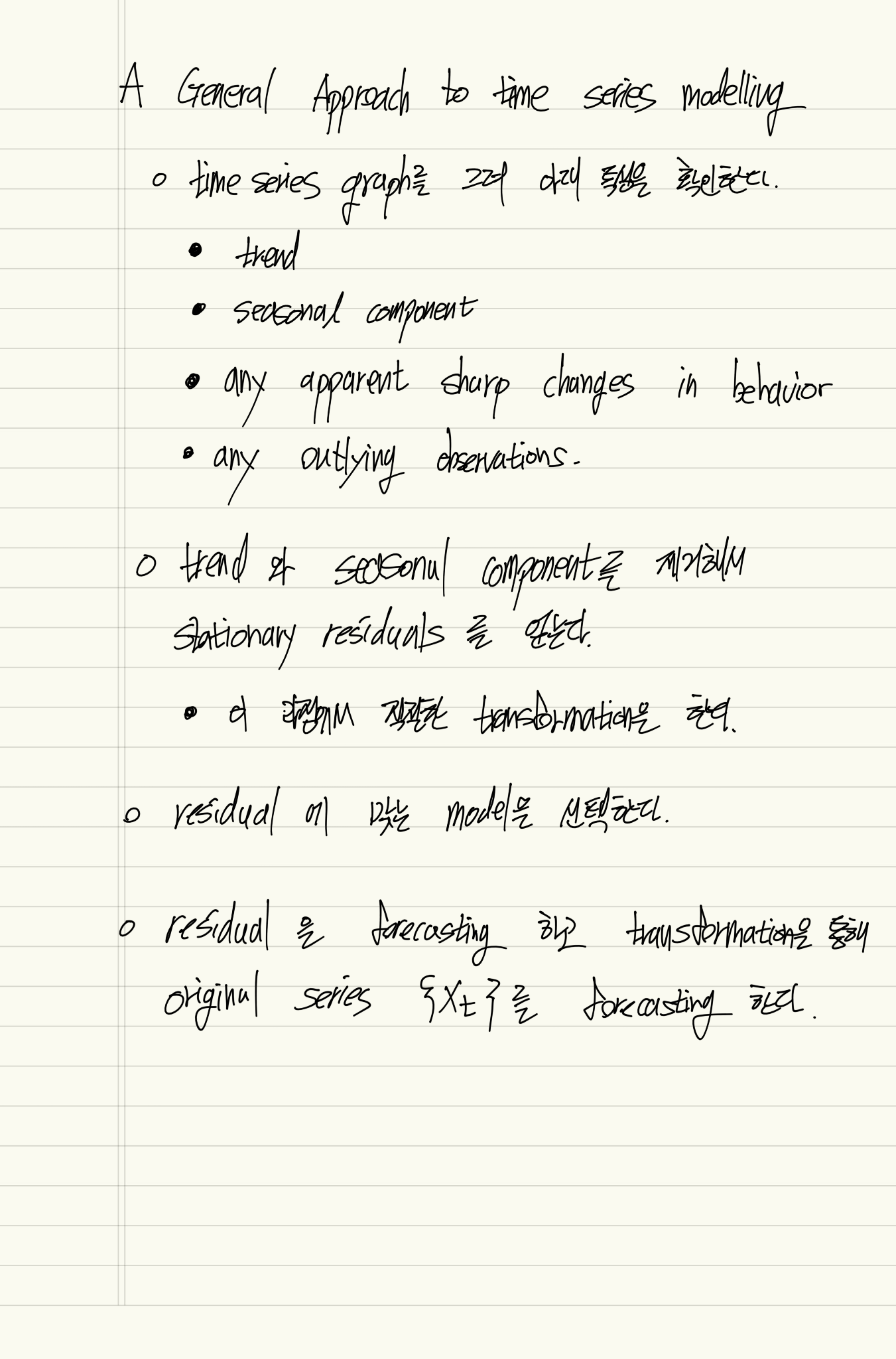
Autocovariance & Autocorrelation
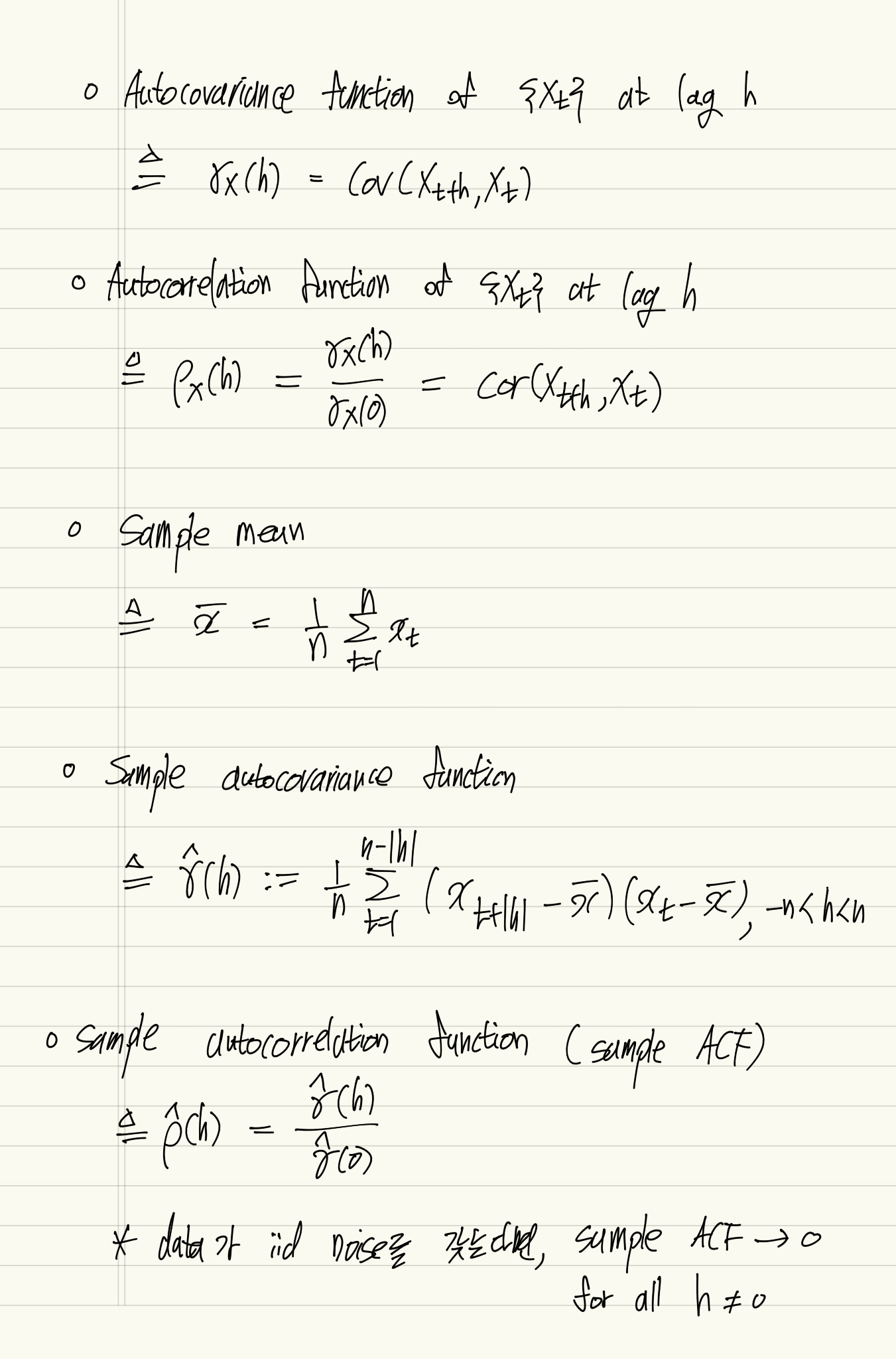
Esitmation and Elimination of Trend and seasonality
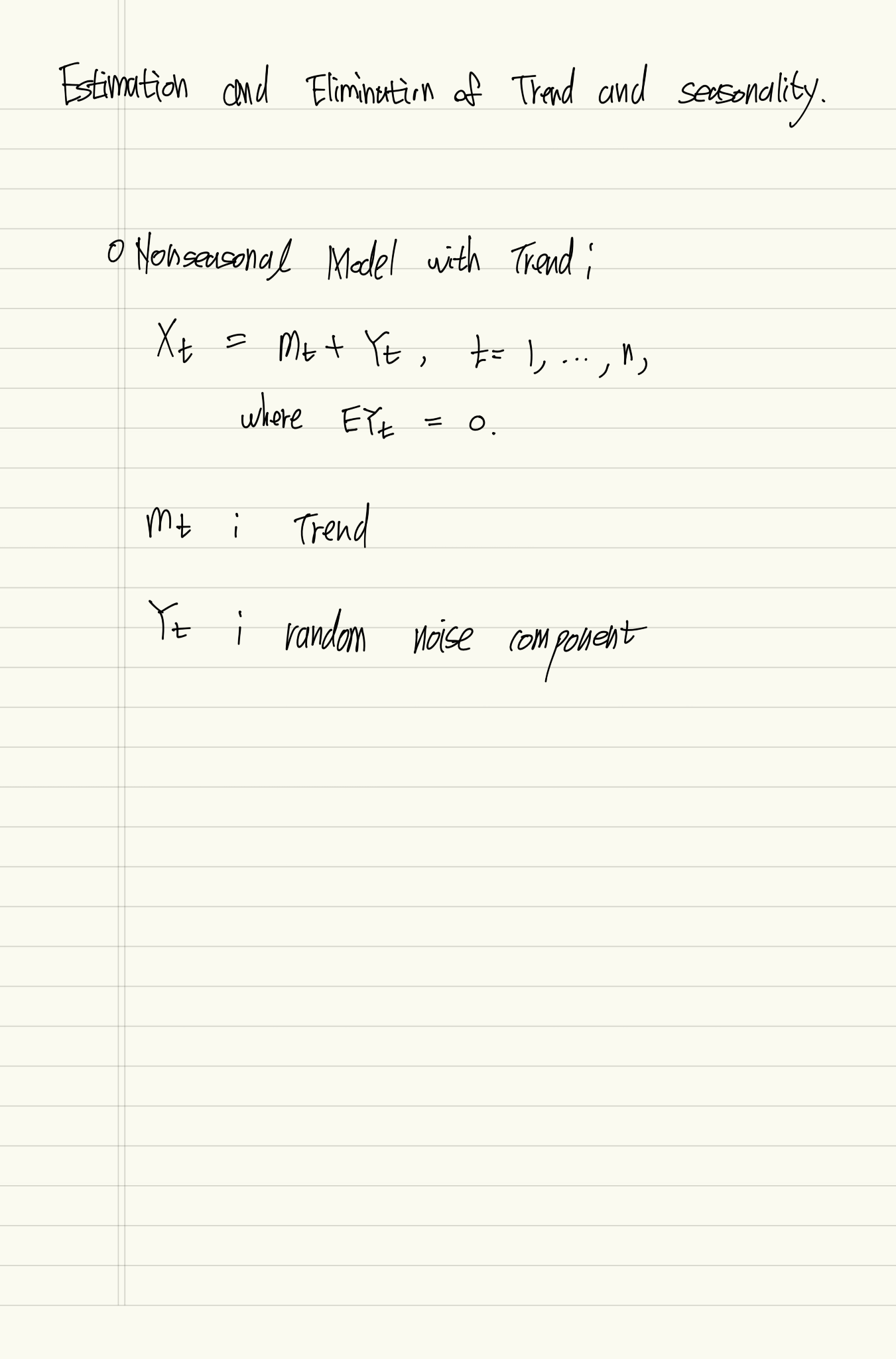
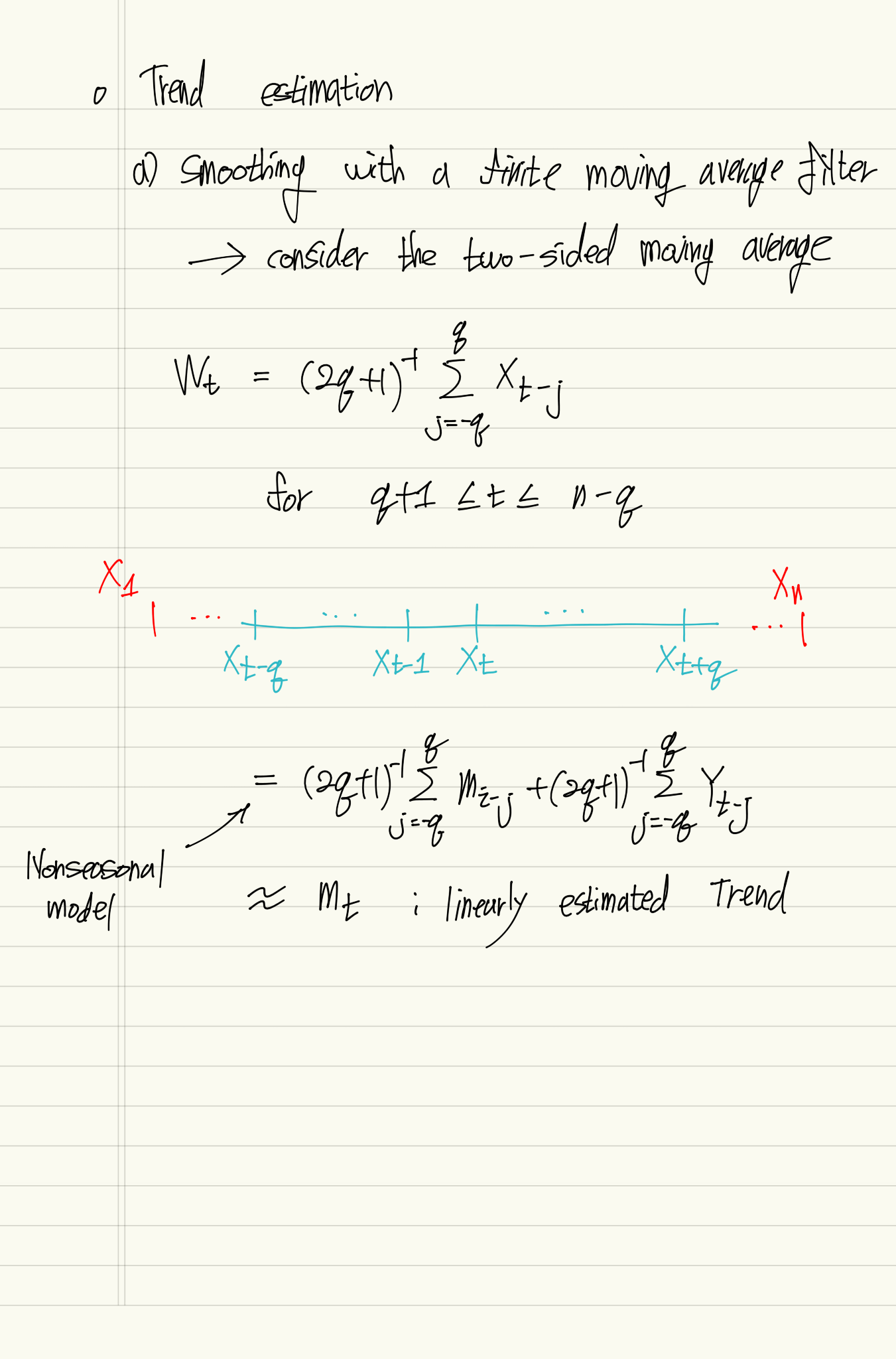
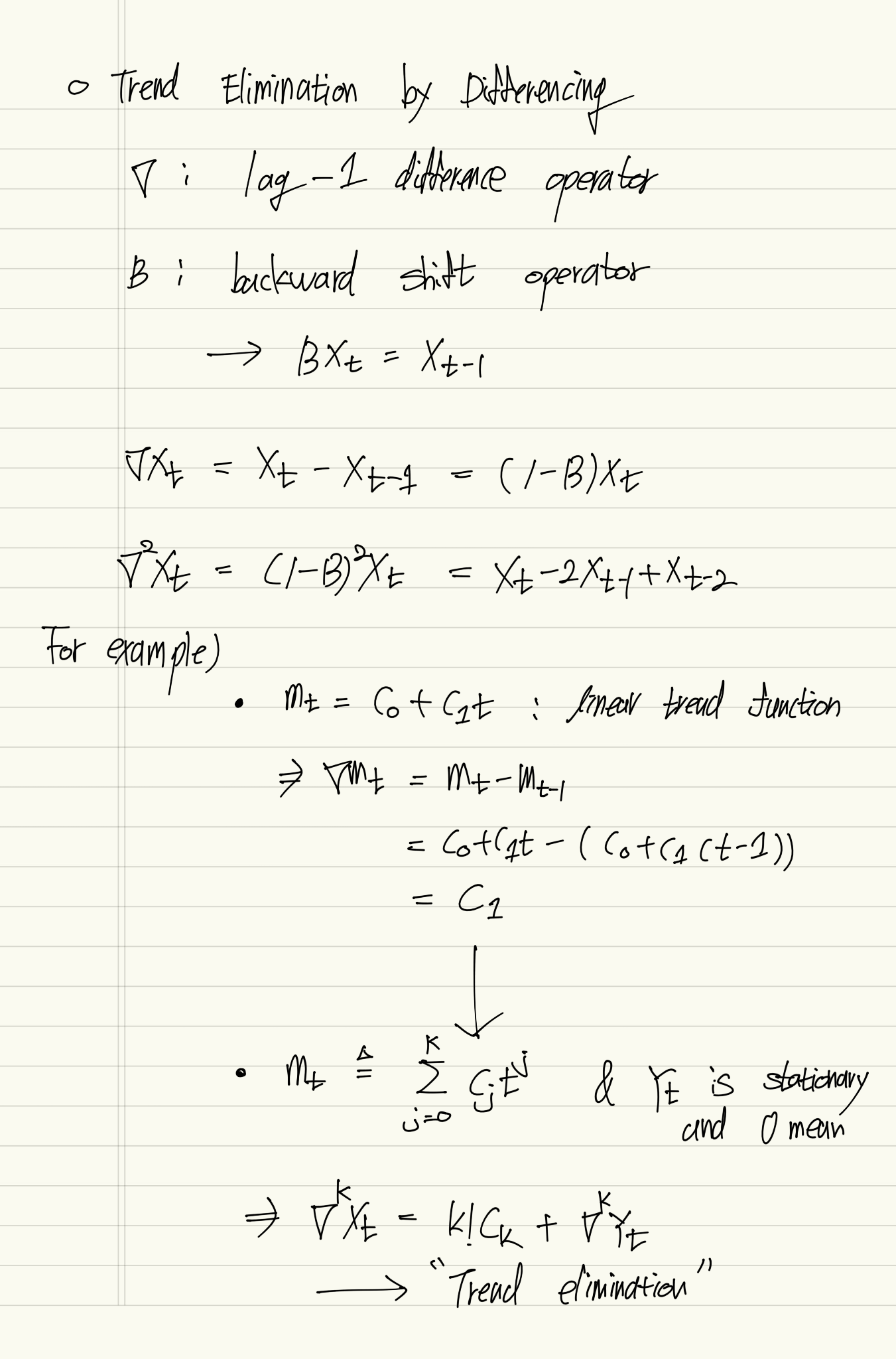
Estimation and Elimination of Both Trend and seasonality
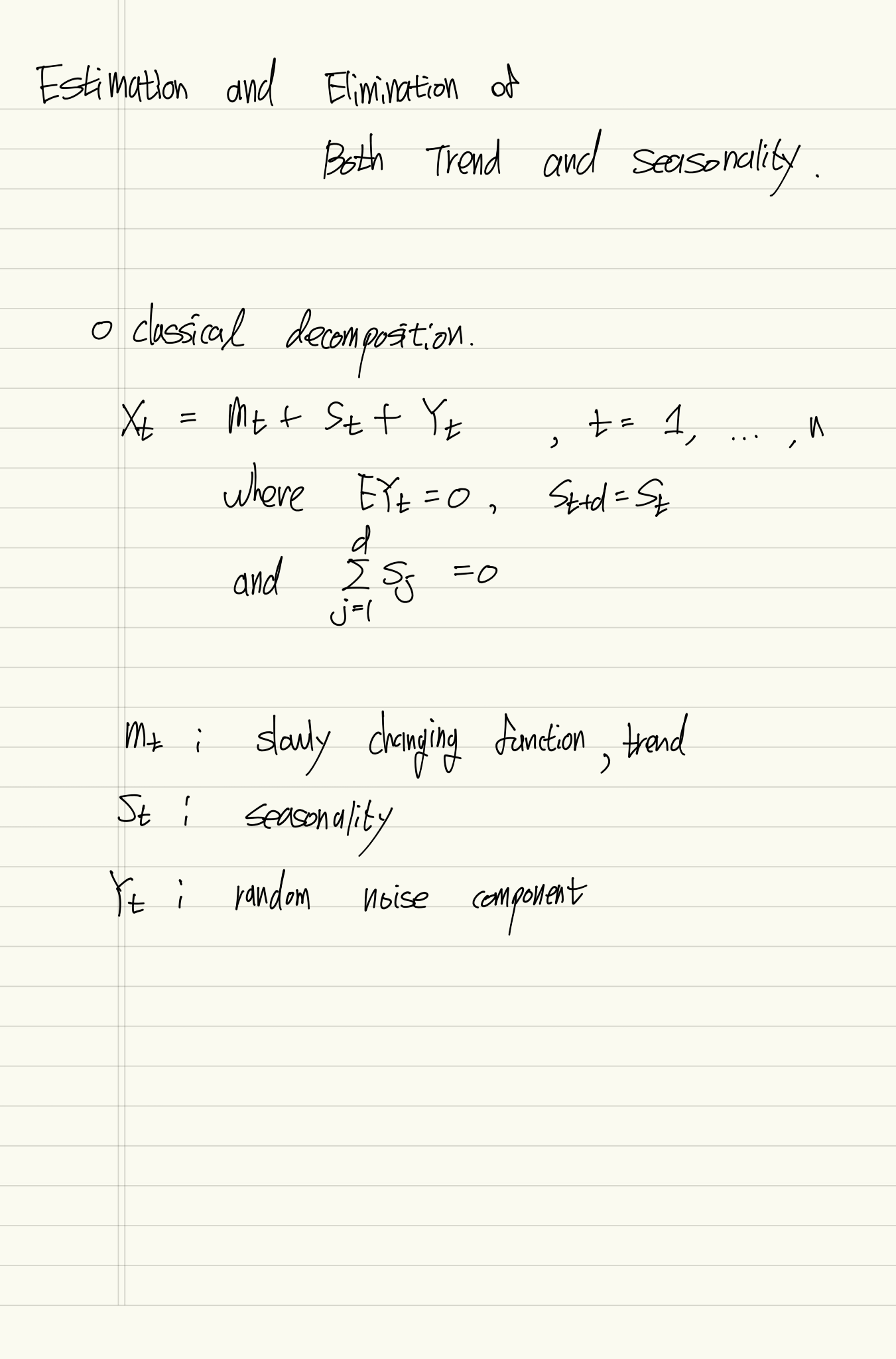
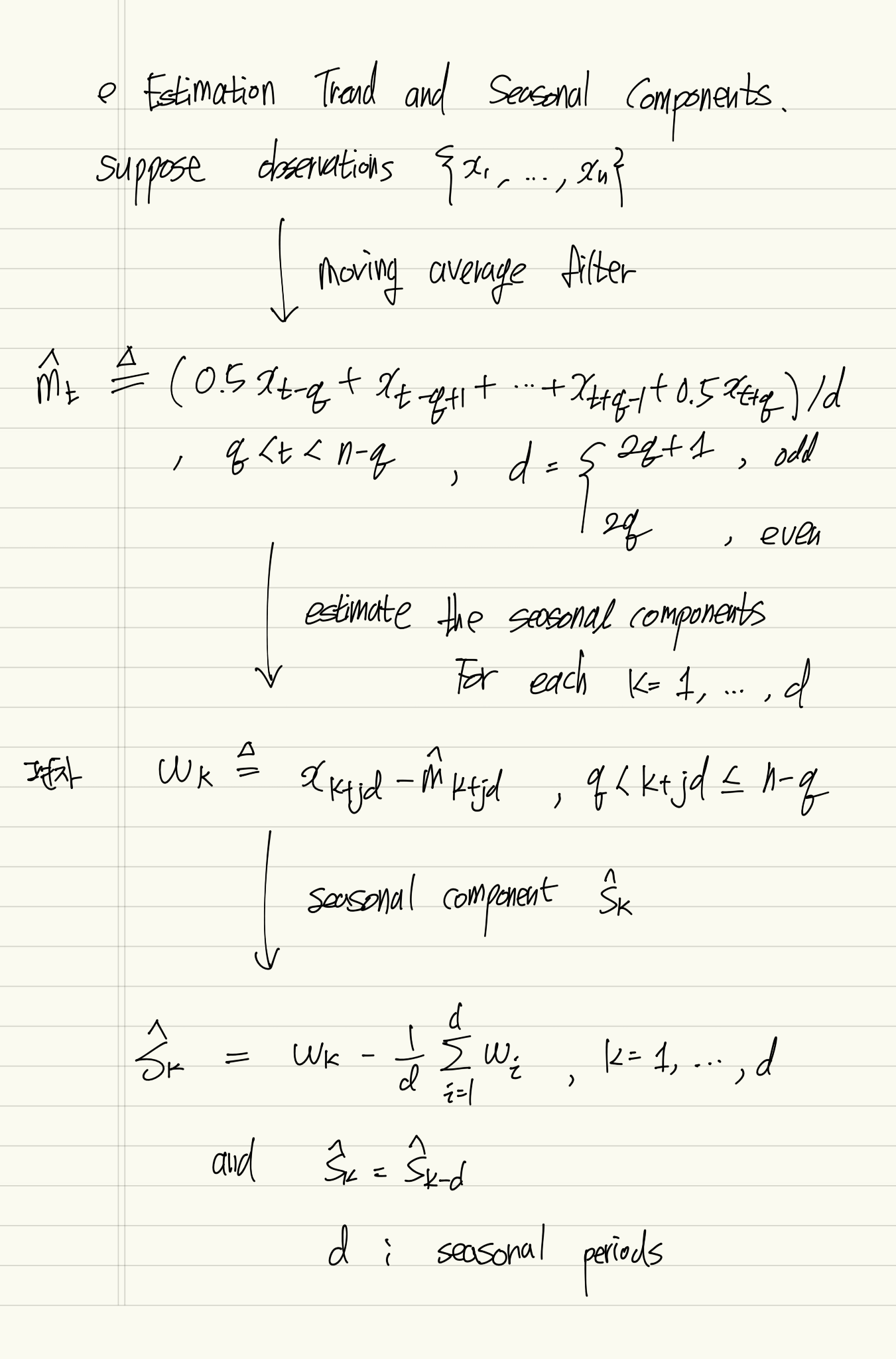
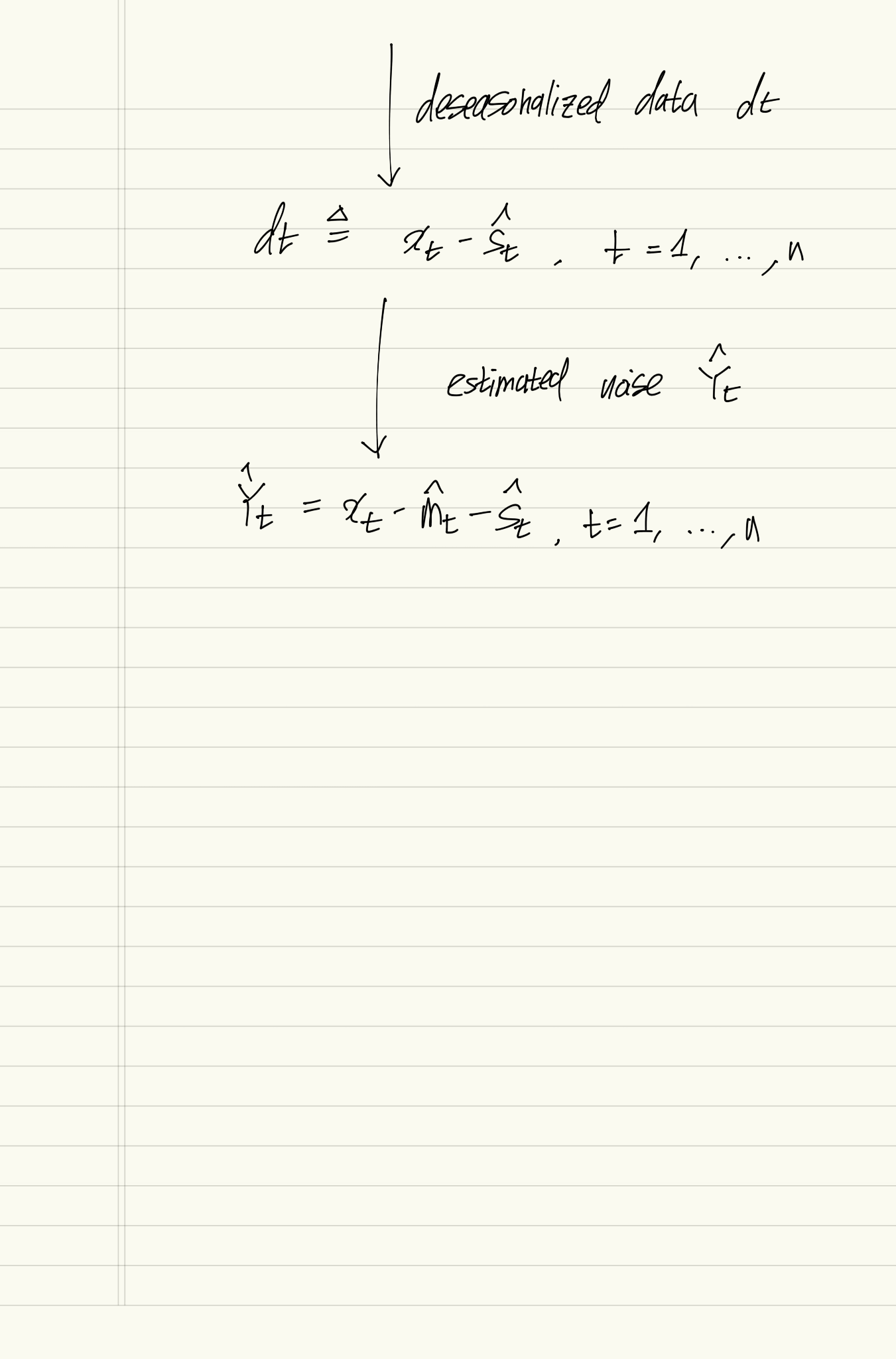
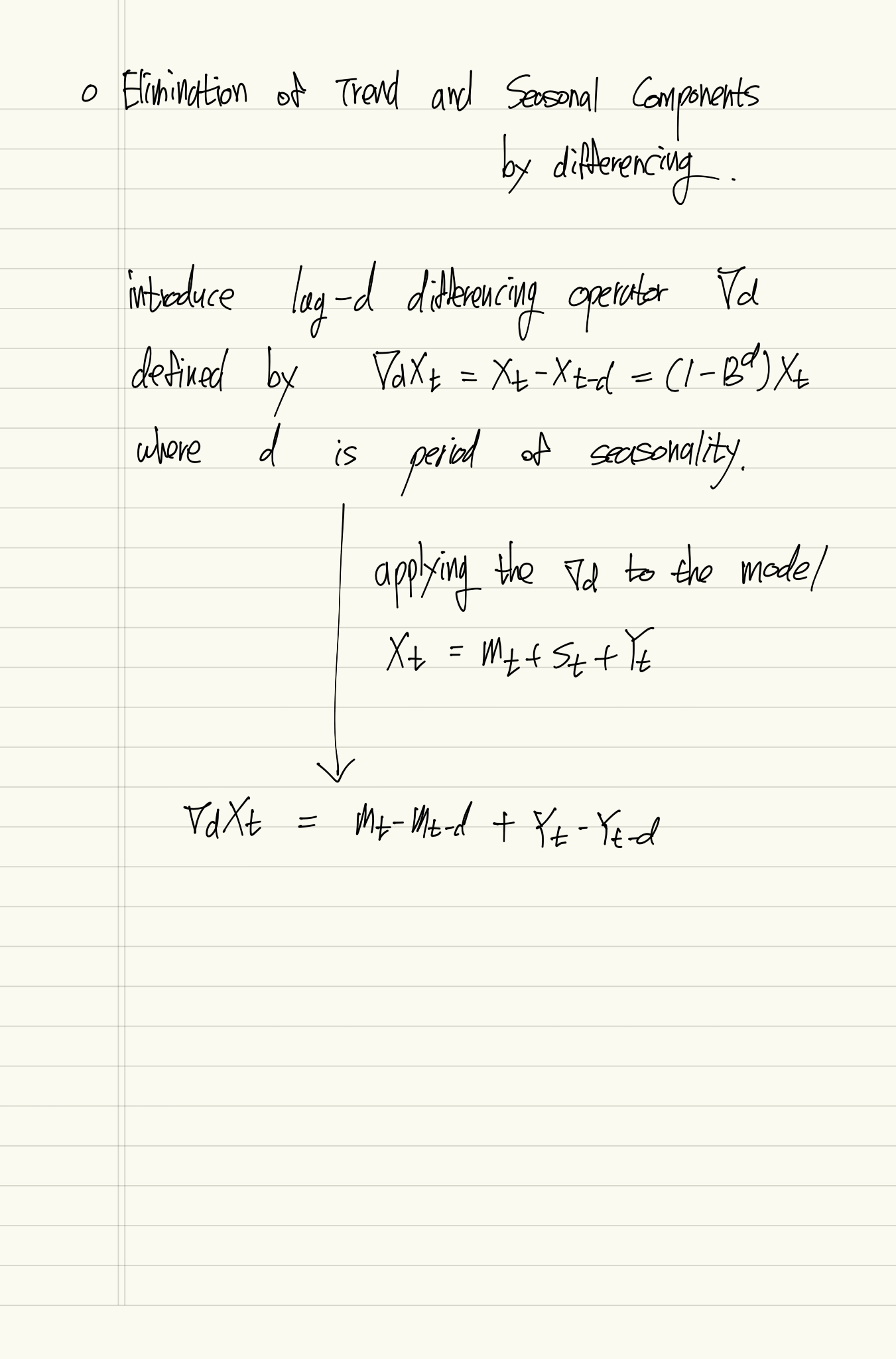
Autoregressive model (AR)
https://en.wikipedia.org/wiki/Autoregressive_model
자기자신을 통한 예측과정이라고 할 수 있고 AR(p)는 다음과 같이 정의된다.
Xt=i=1∑pϕiXt−i+Zt
Zt: white noise
혹은 이와 동등하게 다음과 같이 정의된다.
Xt=i=1∑pϕiBiXt+Zt
Bi: back shift operator (lag operator)
stationary condition은 characteristic equation을 통해서 구해진다.
https://m.blog.naver.com/PostView.naver?isHttpsRedirect=true&blogId=jahyone20&logNo=220933491078
Moving Average model (MA)
https://en.wikipedia.org/wiki/Moving-average_model
MA(q)는 다음과 같이 정의된다.
Xt=μ+i=1∑qθiZt−i+Zt
Z : white noise error term
μ : mean
back shift operator를 이용한 정의는 다음과 같다.
Xt=μ+(1+θ1B+⋯+θqBq)Zt
Autoregressive moving average model(ARMA)
Def of ARMA(p,q)
Xt=i=1∑qθiZt−i+Zt+i=1∑pϕiXt−i
Case of ARMA(1,1)
Xt−ϕXt−1=Zt+θZt−1
where {Zt} ~ WN(0,σ2) and ϕ+θ=0
or
ϕ(B)Xt=θ(B)Zt
Existence of stationary solution
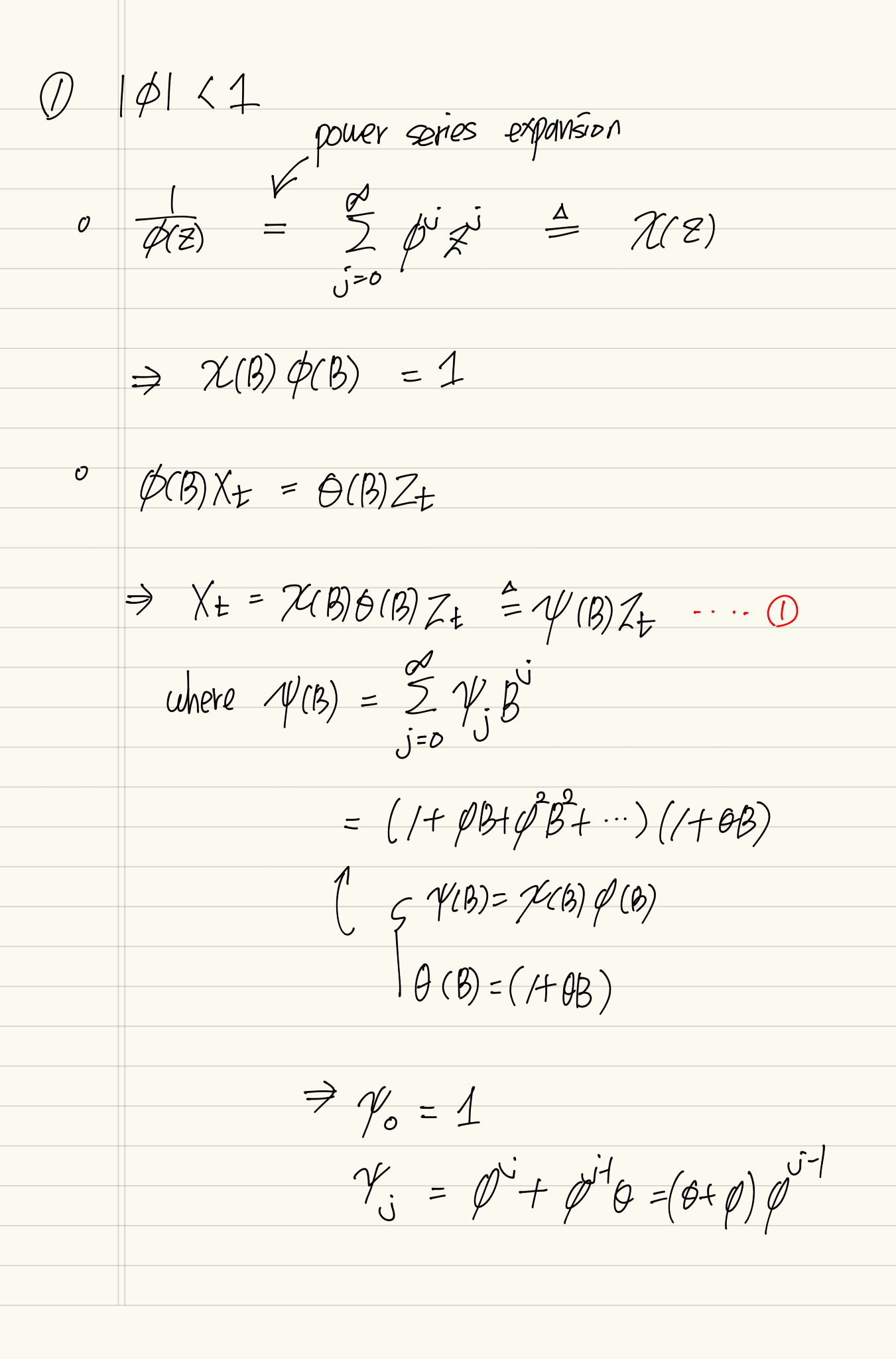
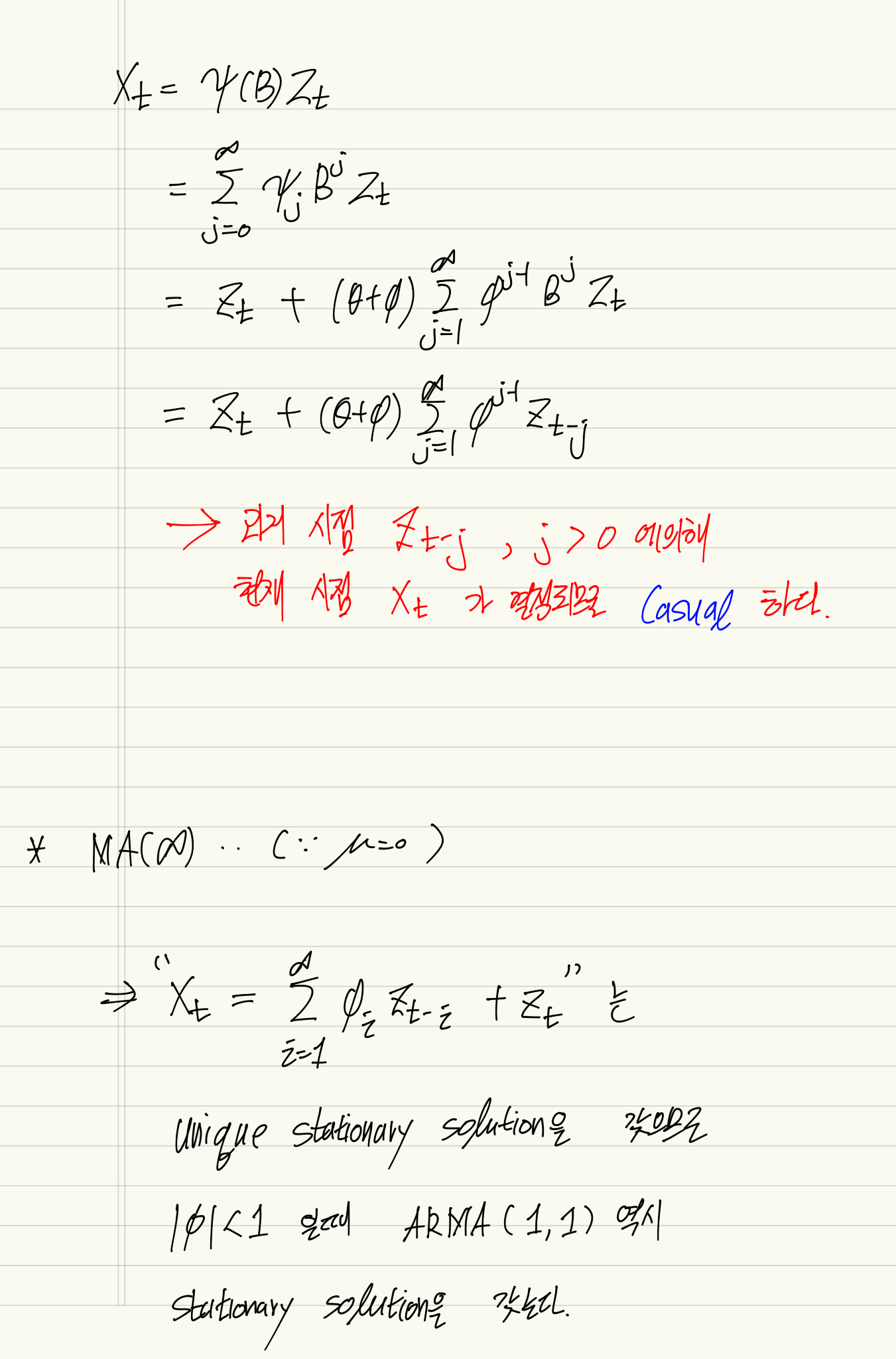
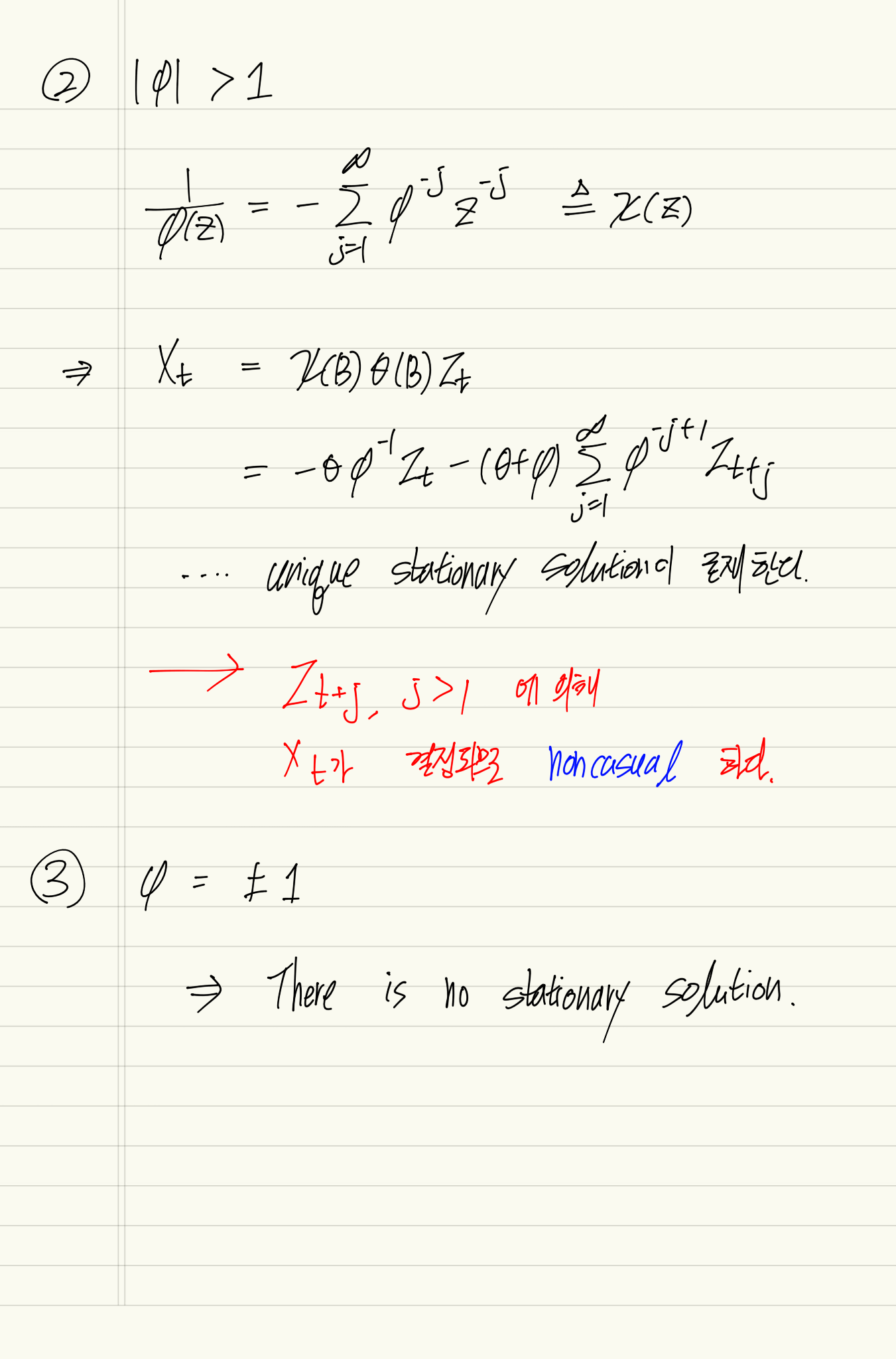
invertible / noninvertible
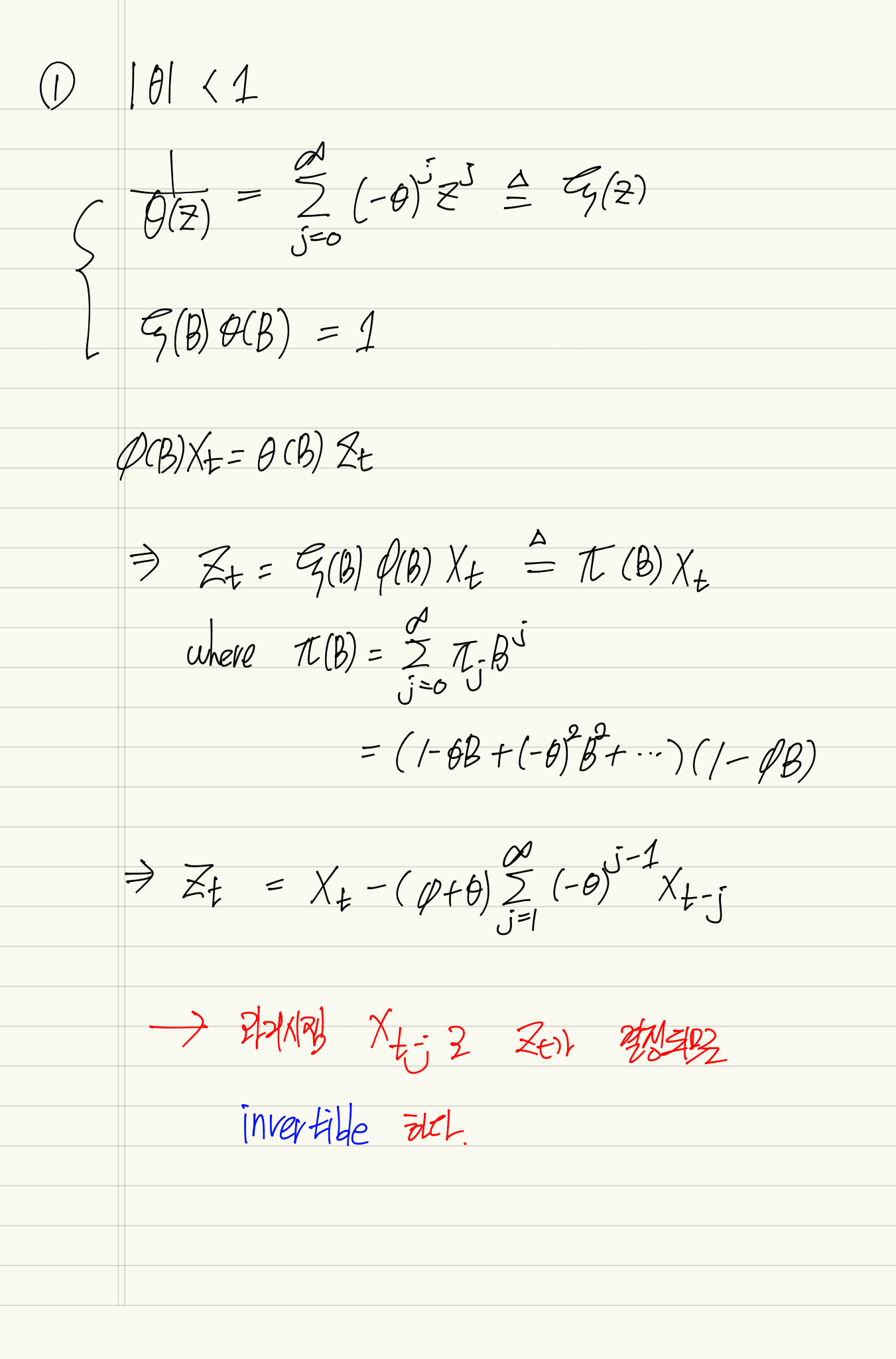
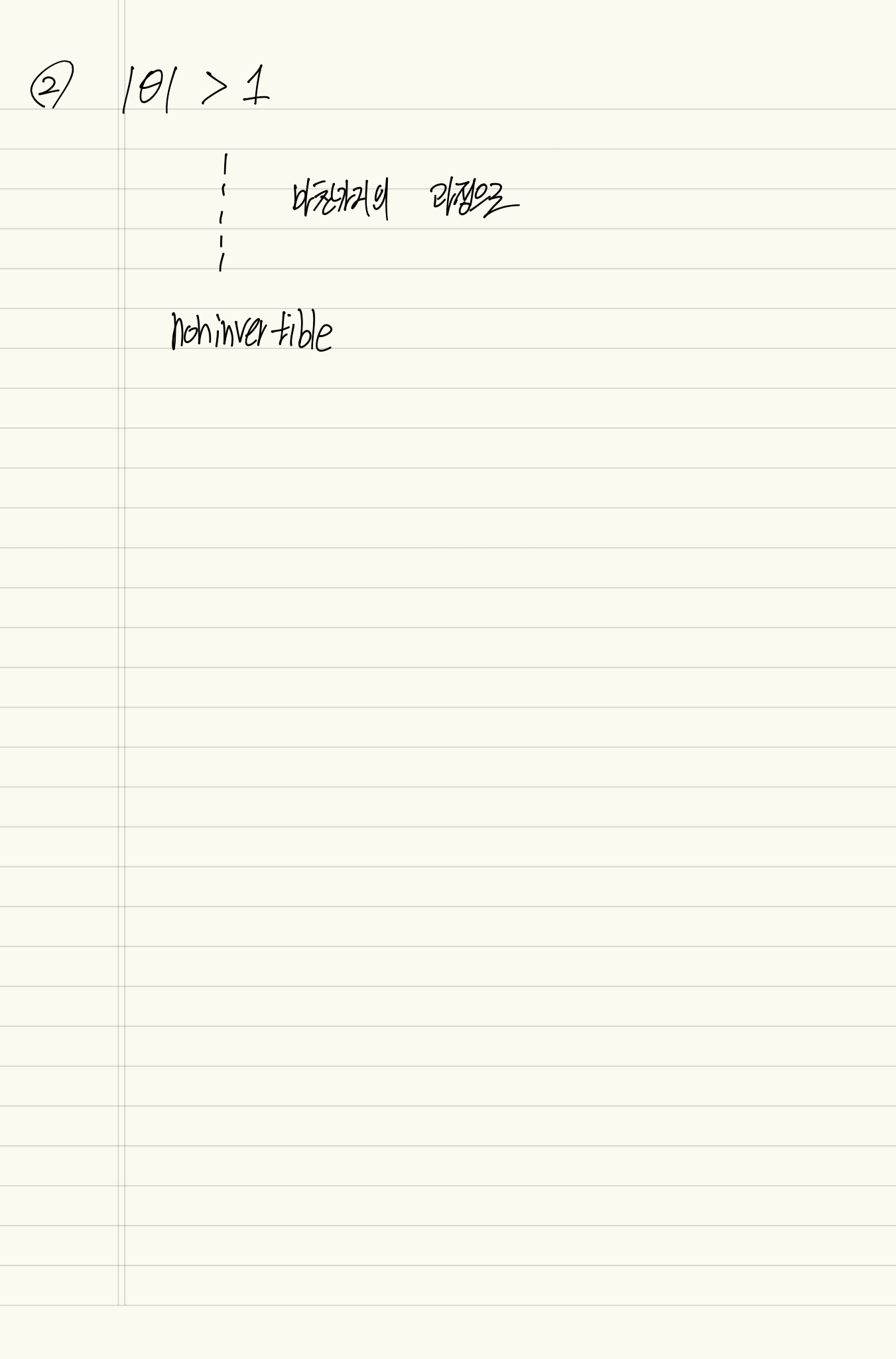
Yule-Walker Estimation
Yule-Walker Equation을 통해 time series model의 parameter를 추정하는 방법이다.
AR model의 경우는 다음과 같다.
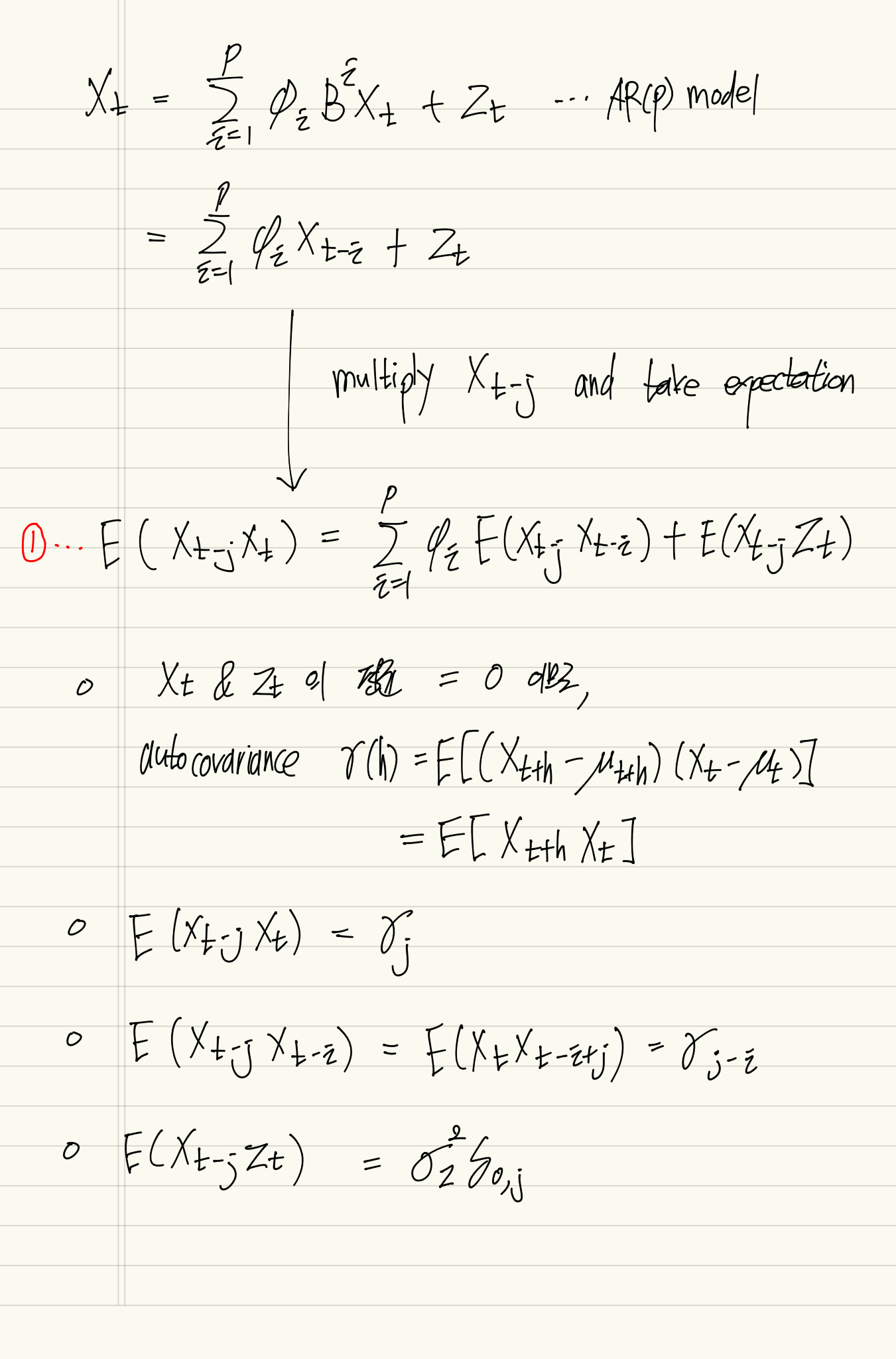
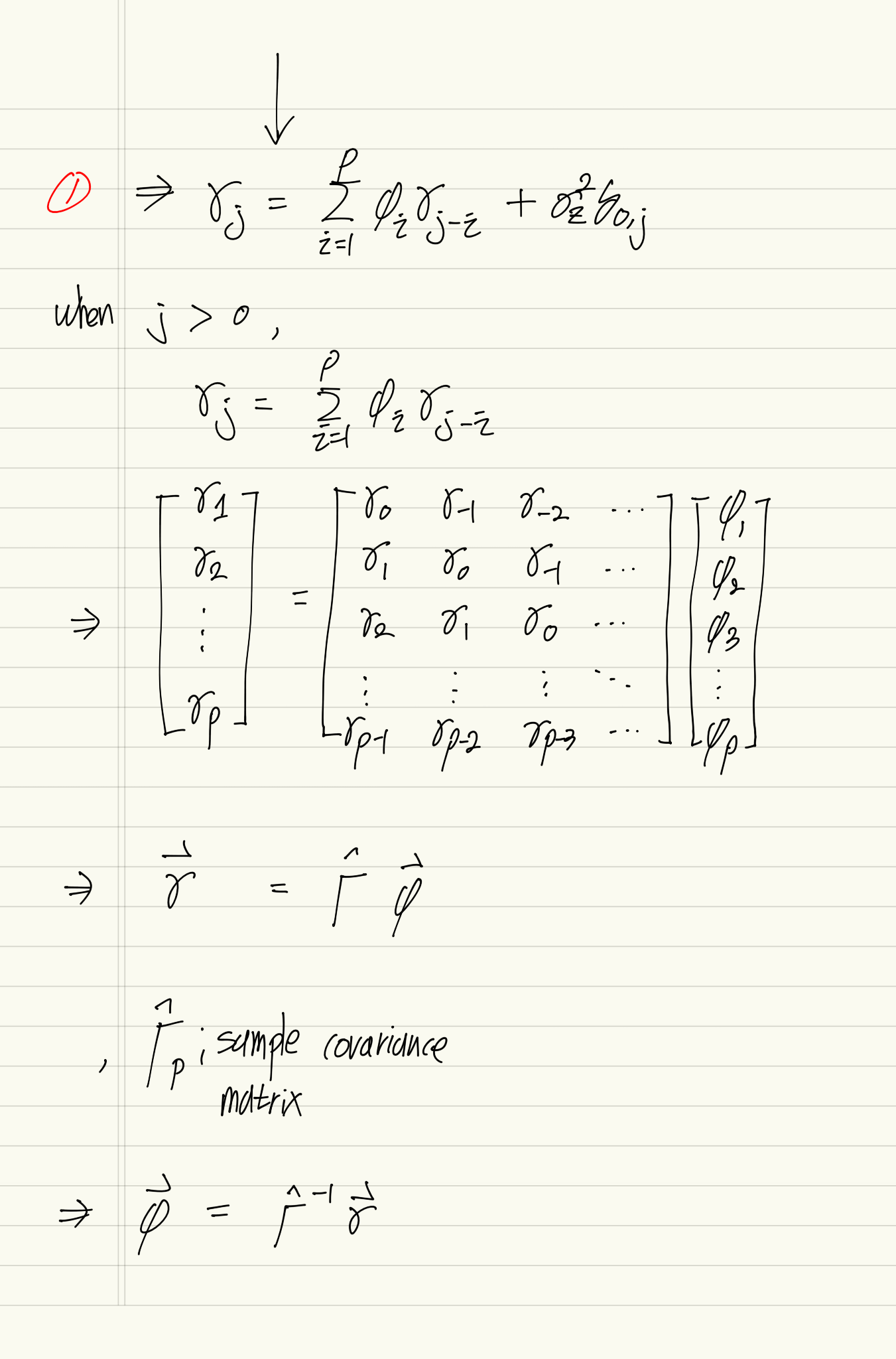
...
Multivariate ARMA
...
State-Space Representations
State-Space model은 아래 두개의 방정식으로 구성되는
time series {Yt, t=1,2,…} 를 말한다.
Yt=GtXt+Wt, t=1,2,… : observation equation
Xt+1=FtXt+Vt, t=1,2,… : state equation
where
{Wt}∼WN(0,{Rt})
, {Vt}∼WN(0,{Qt})
, Gt is sequence of w x v matrices
, Ft is sequence of v x v matrices
, Xt is v-dimensional state variable
, Yt is w-dimensional observation
and Vt is uncorrelated with Wt ( i.e E(WtVtT)=0 for all )
ARMA(1,1) process