<References>
Understanding Convolutions on Graphs
The Challenges of Computation on Graphs
- Lack of Consistent Structure
- ex. molecule; 각각 다른 수/종류의 atom을 포함하고 다른 수/종류의 connection을 가짐
- Node-Order Equivariance
- 원래는 노드에 순서가 없지만, 임의로 부여 ⇒ node-order equivariant
- Scalability
- very large graph, but sparse
Problem Setting and Notation
- Node Classification: Classifying individual nodes.
- Graph Classification: Classifying entire graphs.
- Node Clustering: Grouping together similar nodes based on connectivity.
- Link Prediction: Predicting missing links.
- Influence Maximization: Identifying influential nodes.
→ 문제 해결에 선행하여 node representation learning이 필요
- Undirected Graph
- xv : node features for node v∈V
- hv(k) : kth layer(iteration)을 거친 뒤 node v의 representation
- Mv : matrix M에서 node v의 property
Extending Convolutions to Graphs
ordinary convolutions : not node-order invariant
- 이미지의 경우 pixel의 위치에 영향을 받음
⇒ How to generalize convolutions to general graph?
Polynomial Filters on Graphs
- CNN에서 주변 pixel에대해 localized filter를 적용하는 것처럼 주변 노드들에 대해 polynomial filter적용하기
The Graph Laplacian
- A : Adjacency Matrix
- D : Degree Matrix
Dv=u∑Avu
- L = Graph Laplacian, n×n matrix
Polynomials of the Laplacian
- Graph Laplacian을 이해하기 위한 polynomial 만들기
- polynomial : CNN에서의 필터에 해당하는 느낌
- coefficient w : 필터의 가중치
pw(L)=w0In+w1L+w2L2+⋯+wdLd=i=0∑dwiLi
이 polynomial의 계수들을 다음의 벡터로 나타낼 수 있다. → w=[w0,…,wd]
- 모든 w, pw(L)은 n×n matrix
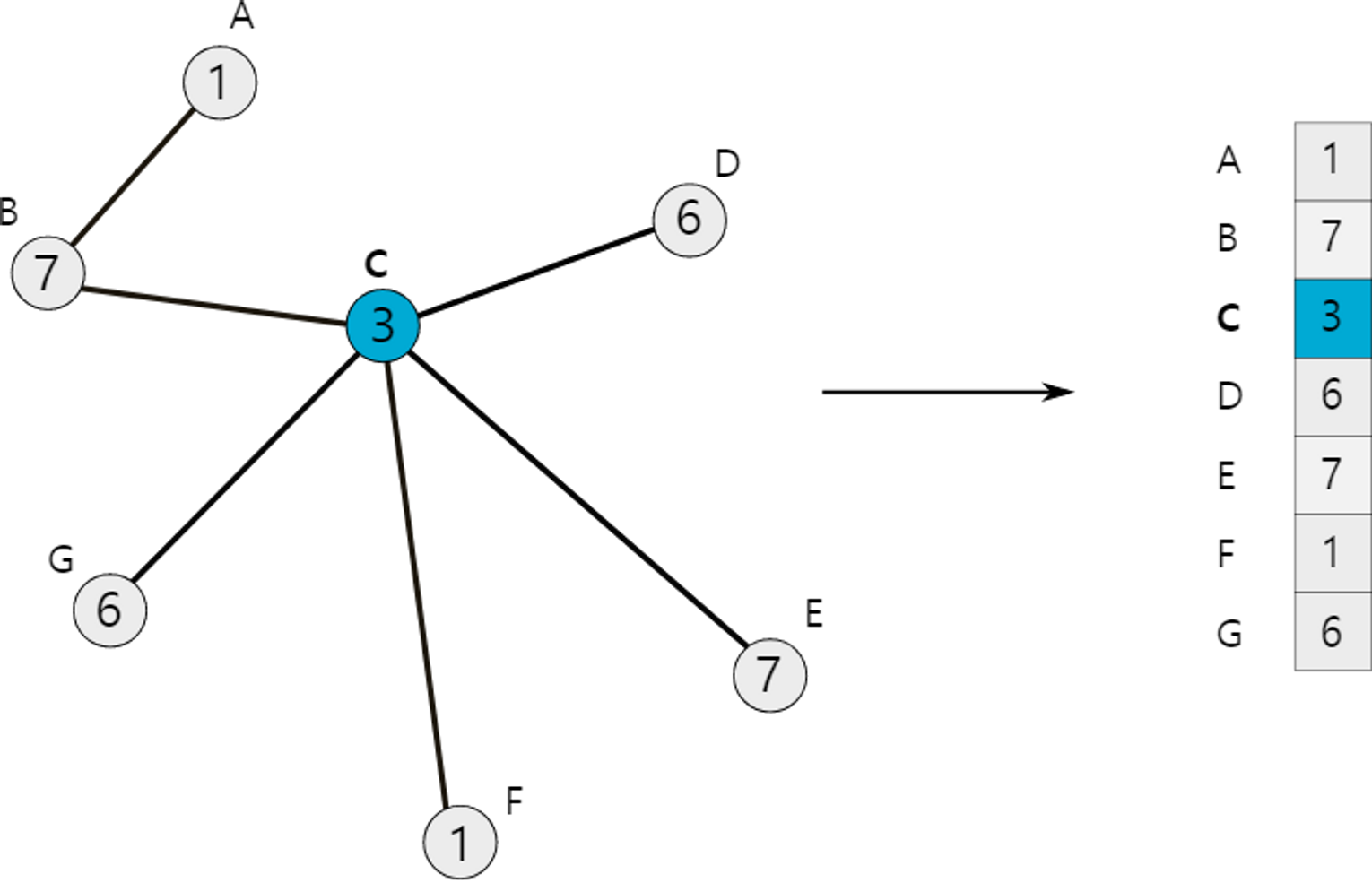
Fixing a node order (indicated by the alphabets) and collecting all node features into a single vector x
- node feature xv가 실수라고 할때, 이를 stacking하여 single vector x를 만들 수 있고, 이때 x∈Rn이다.
- feature vector x에 대해 polynomial filter pw로 convolution을 적용하면 다음과 같이 나타낼 수 있다.
x′=pw(L) x
💡 1. pw(L)=∑i=0dwiLi 에서 계수 w가 convolution에 어떤 영향을 미치는가?
- ex1. w0=1, wi=0 for i ≥1 일때
x′=pw(L) x=i=0∑dwiLix=w0Inx=x
- ex2. w1=1, wi=0 for i=1 일때
x′v=(Lx)v=Lvx=u∈G∑Lvuxu=u∈G∑(Dvu−Avu)xu=Dvxv−u∈N(v)∑xu
- Adjacency matrix * x → 이웃 노드들의 feature합계
💡 2. Polynomial의 차수 d는 convolution에 어떤 영향을 미치는가?
-
Lemma 5.2 from Wavelets on graphs via spectral graph theory
Wavelets on graphs via spectral graph theory
Let G be a weighted graph, L the graph Laplacian (normalized or non-normalized) and s > 0 an integer. For any two
vertices m and n, if dG(m,n)>s then (Ls)m,n=0.
Proof.
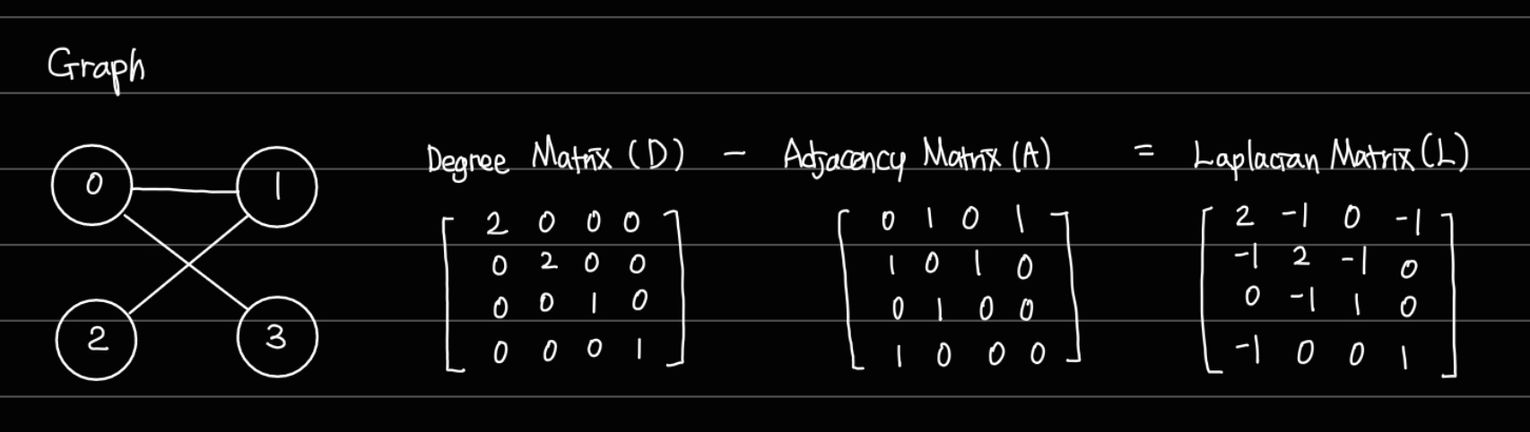
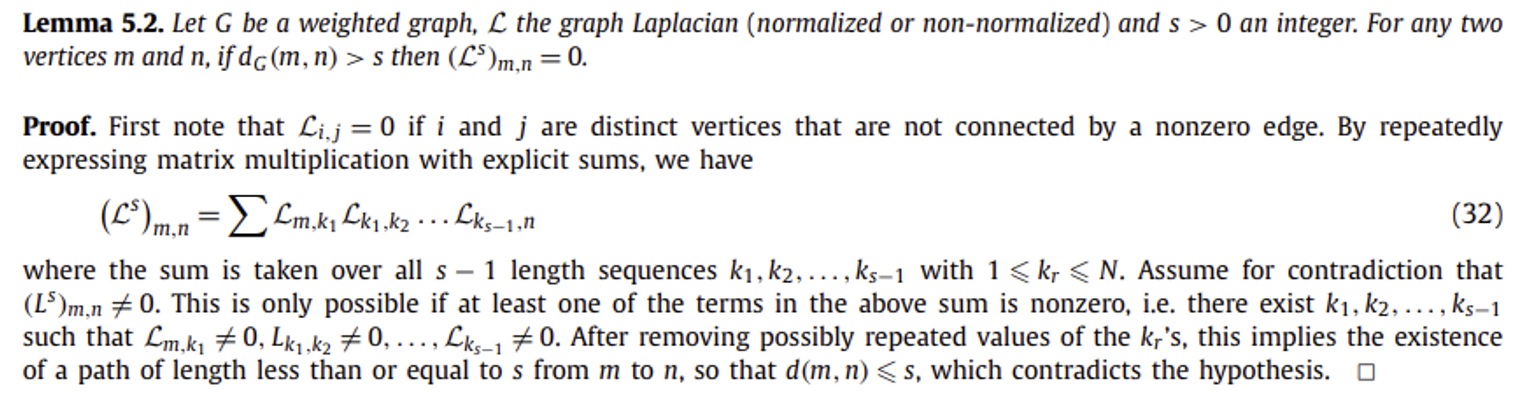
- s-1 길이의 sequence k1,k2,…,ks−1 with 1≤kr≤N
- 노드 m,n사이의 Laplacian의 s제곱((Ls)m,n)은 m에서 n까지 도달하는 s-1길이의 path들의 노드들사이 Laplacian의 곱의 합(path들간의 합)이라고 할 수 있음
- 증명하려는 것과 반대로, (Ls)m,n가 non-zero이려면, sum이 진행되는 term(Laplacian matrix의 곱)들 중 적어도 하나가 non-zero이어야 한다.
- 즉, Lm,k1=0,Lk1,k2=0,…,Lks−1,n=0. 인 k1,k2,…,ks−1(path)이 존재한다. ⇒ 노드 m,n사이에 길이가 s인 path가 존재한다.
- 반복되는 노드를 제거할경우, “노드 m,n사이에 길이가 s보다 작은 path가 존재한다”고 정리할 수 있다.
- 즉, (Ls)m,n=0 이려면, 노드 m,n사이에 길이가 s보다 짧은 path가 존재하지 않는다. ⇒ 두 노드 사이 거리는 s보다 크다.
다시 돌아와서 Polynomial의 차수 d가 convolution에 미치는 영향에 대해 보면,
distG(v,u)>i⇒Lvui=0
x′를 얻기 위해 x를 차수가 d인 polymonial px(L)로 대체하면,
xv′=(pw(L)x)v=(pw(L))vx=i=0∑dwiLvix=i=0∑dwiu∈G∑Lvuixu=i=0∑dwi u∈G, distG(v,u)≤i∑xu
- 노드 v에서의 convolution은 d hop보다 멀지 않은 노드들에 대해서만 적용됨
- localized polynomial filters, d의 크기에 따라 localization의 정도 변동
ChebNet
위에서 사용했던 polynomial filter는 다음과 같이 정의됨
pw(L)=i=0∑dwiLi
ChebNet에서는 polynomial filter를 다음의 형태로 수정하여 사용
pw(L)=i=1∑dwiTi(L~)
- Ti : i차의 1종 체비세프 다항식
- L~ : L의 가장 큰 eigen value를 사용해 정의된 normalized Laplacian
L~=λmax(L)2L−In
- L → positive semi-definite : L의 모든 eigenvalue들은 0보다 작지 않다.
- λmax>1 이라면, L의 제곱들은 급격하게 크기가 커지게 되는데, L을 효율적으로 scale-down한 L~의 경우 eigenvalue들이 [-1,1]의 구간에 있음을 보장하여 제곱값의 크기가 끝없이 커지는 것을 막는다.
- unnormalized Laplacian L에 대해 높은 차원의 계수의 크기 제한
- normalized Laplacian L~에 대해서는 더 큰 값의 계수 허용
- chebyshev polynomial → interpolation을 수치적으로 안정적이게 만드는 특성
Polynomial Filters are Node-Order Equivariant
Polynomial filter
- node order에 independent ← pw의 차원이 1이고, x가 이웃 노드의 feature를 aggregate한 value인 경우를 떠올리면 이해하기 쉬움(ex. 합계는 순서와 상관이 없음)
- proof. permutation matrix P : 정사각 이진 행렬, 각 행에 값이 1인 요소가 하나 있고, 나머지는 0의 값을 가짐. 다른 matrix A 를 곱해주었을 때 A의 각 row를 permutating해주는 것과 같은 효과라고 이해하면 됨.(=행 교환을 수행하는 행렬)
PPT=PTP=In function f : node-order equivariantf(Px)=Pf(x) permutation matrix P를 활용해 새로운 node-order를 만들어내면, 다음과 같은 변환이 수행된다.xLLi→Px→PLPT→PLiPT f(x)=pw(L)x 와 같은 polynomial filter가 있을 때, 다음과 같이 정리할 수 있다.f(Px)=i=0∑dwi(PLiPT)(Px)=Pi=0∑dwiLix=Pf(x)
Embedding Computation
- K different polynomial filter layer
- kth layer의 learnable weights w(k)
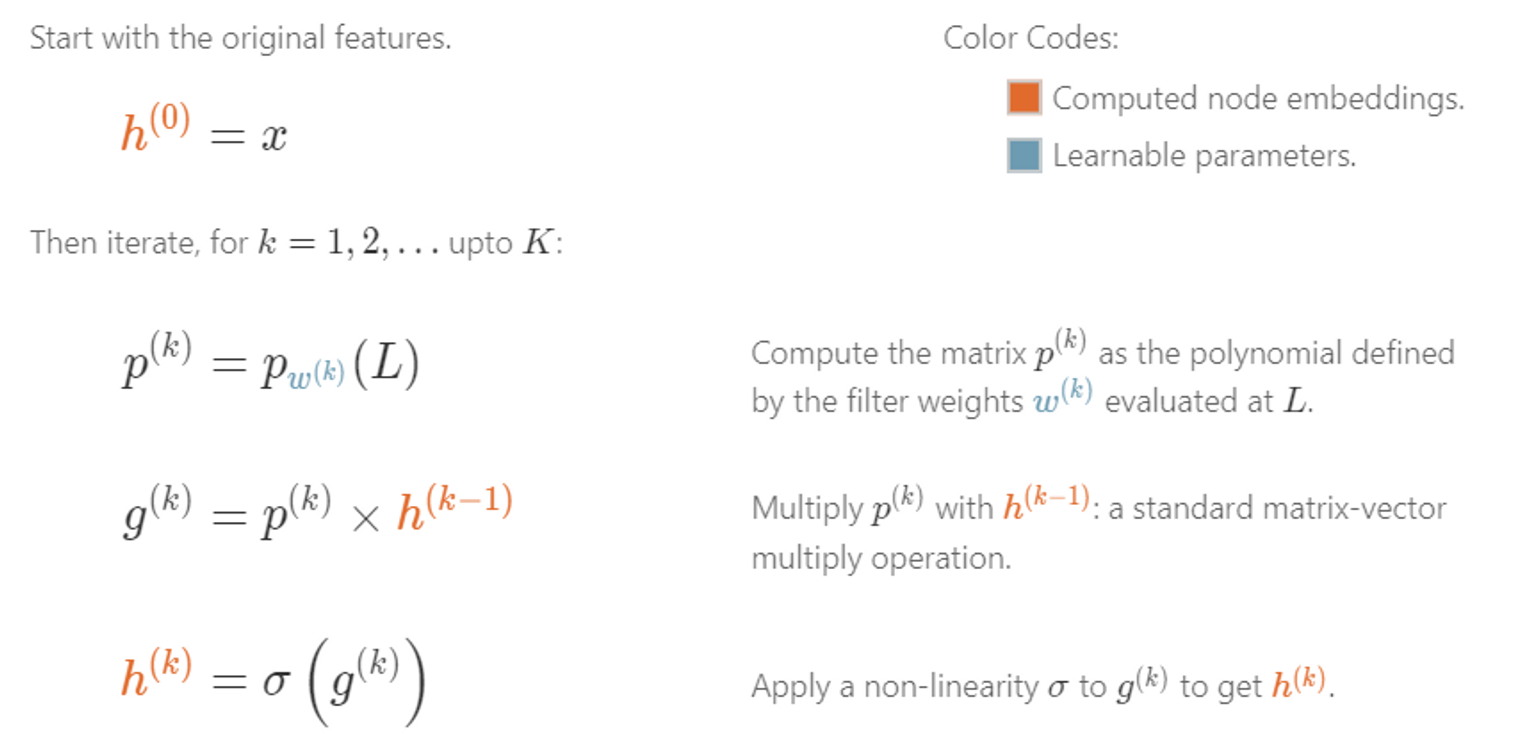
- w(k)의 weight를 가진 polynomial filter를 L에 적용하여 matrix p(k)를 계산
- p(k)에 h(k−1)를 곱해 g(k)를 계산
- g(k)에 non-linearity σ를 적용하여 h(k)를 계산
- CNN에서의 weight-sharing(동일한 필터를 여러 grid에 적용)과 마찬가지로, 다른 노드들에 같은 weight를 가진 filter를 적용
Modern Graph Neural Networks
pw(L)=w0In+w1L+w2L2+⋯+wdLd
- w1=1, 나머지 wi들은 모두 0
- pw(L)=L
- pw의 차원 d ⇒ 얼마나 localized된 필터를 사용할 것인가
xv′=Lvx=u∈G∑Lvuxu=u∈G∑(Dvu−Avu)xu=Dvxv−u∈N(v)∑xu
- Aggregation : 바로 이웃하는 노드 feature xu들을 aggregate
- Combination : 자기자신의 feature xv를 합침
💡 Polynomial filter를 사용해 가능한 것과 별개로 다른 종류의 “Aggregation”, “Combination” 를 사용하면 어떨까?
- Aggregation이 node-order equivariant하다면, convolution 전체가 node-order equivariant하다.
- 바로 이웃하는 노드들 사이의 “message-passing”과정이라고 이해할 수 있다.
- 1-hop localized convolution을 K번 반복하면, K-hop만큼 멀리에 있는 노드들까지 receptive field를 늘릴 수 있다.
Embedding Computation
Message-passing을 backbone으로 하는 많은 GNN Architecture들
-
Graph Convolutional Networks (GCN)
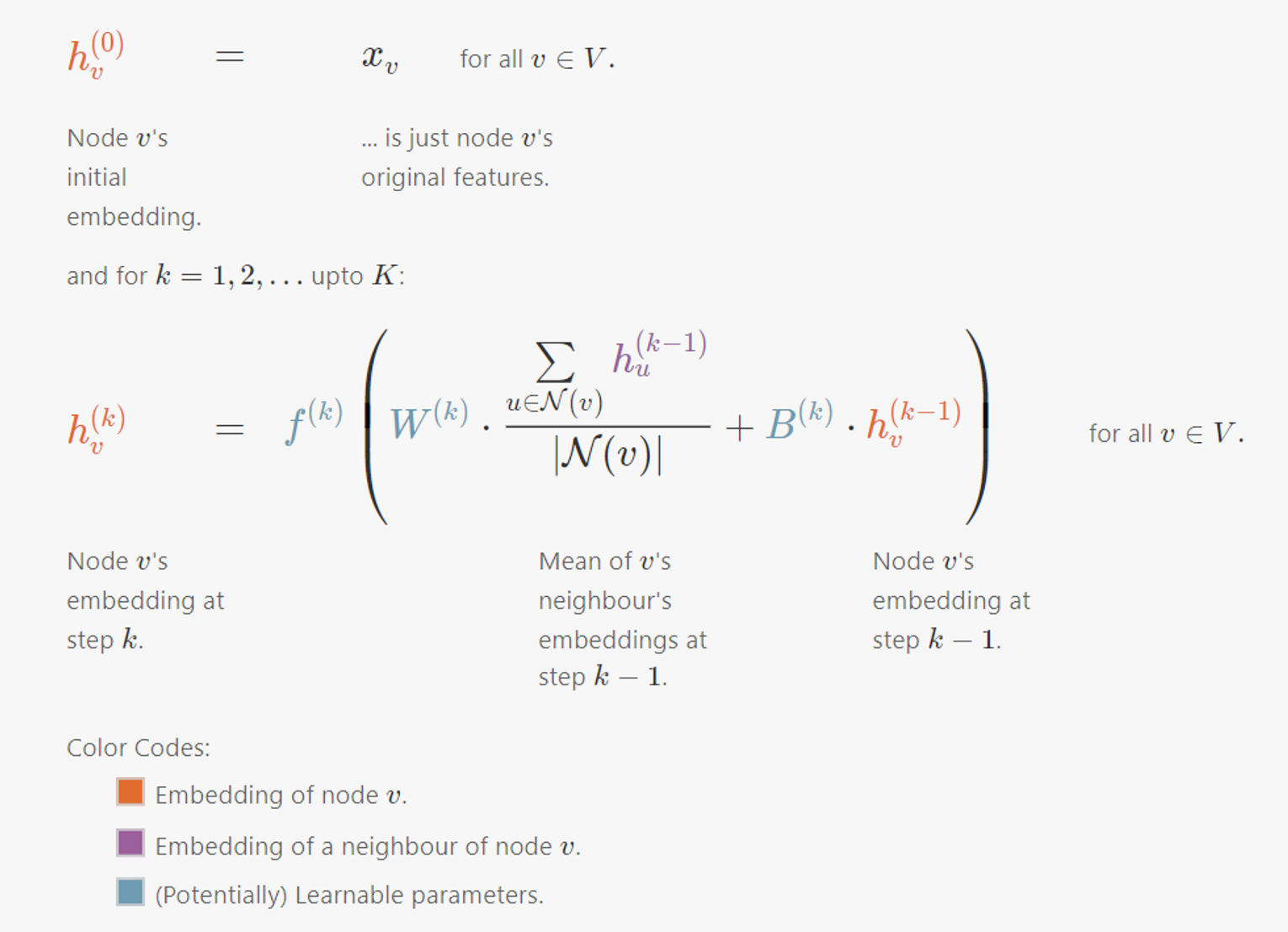
- 각 k 단계별로 학습가능한 function f(k), matrics W(k), B(k)는 모든 노드들에 걸쳐 공유됨
-
Graph Attention Networks (GAT)
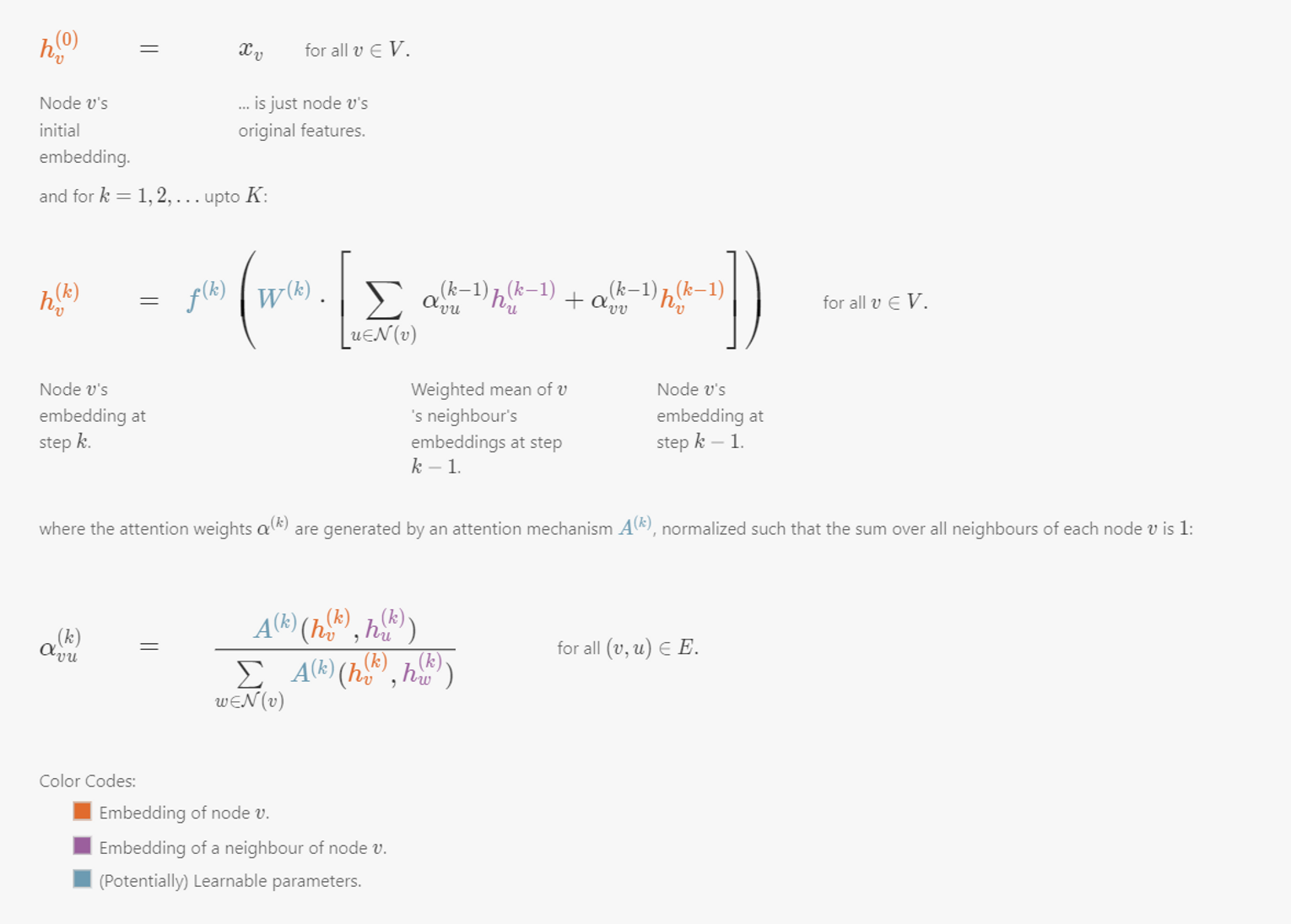
- 각 k 단계별로 학습가능한 function f(k), matrics W(k), B(k), Attention A(k)는 모든 노드들에 걸쳐 공유됨
- multi-head attention
-
Graph Sample and Aggregate (GraphSAGE)
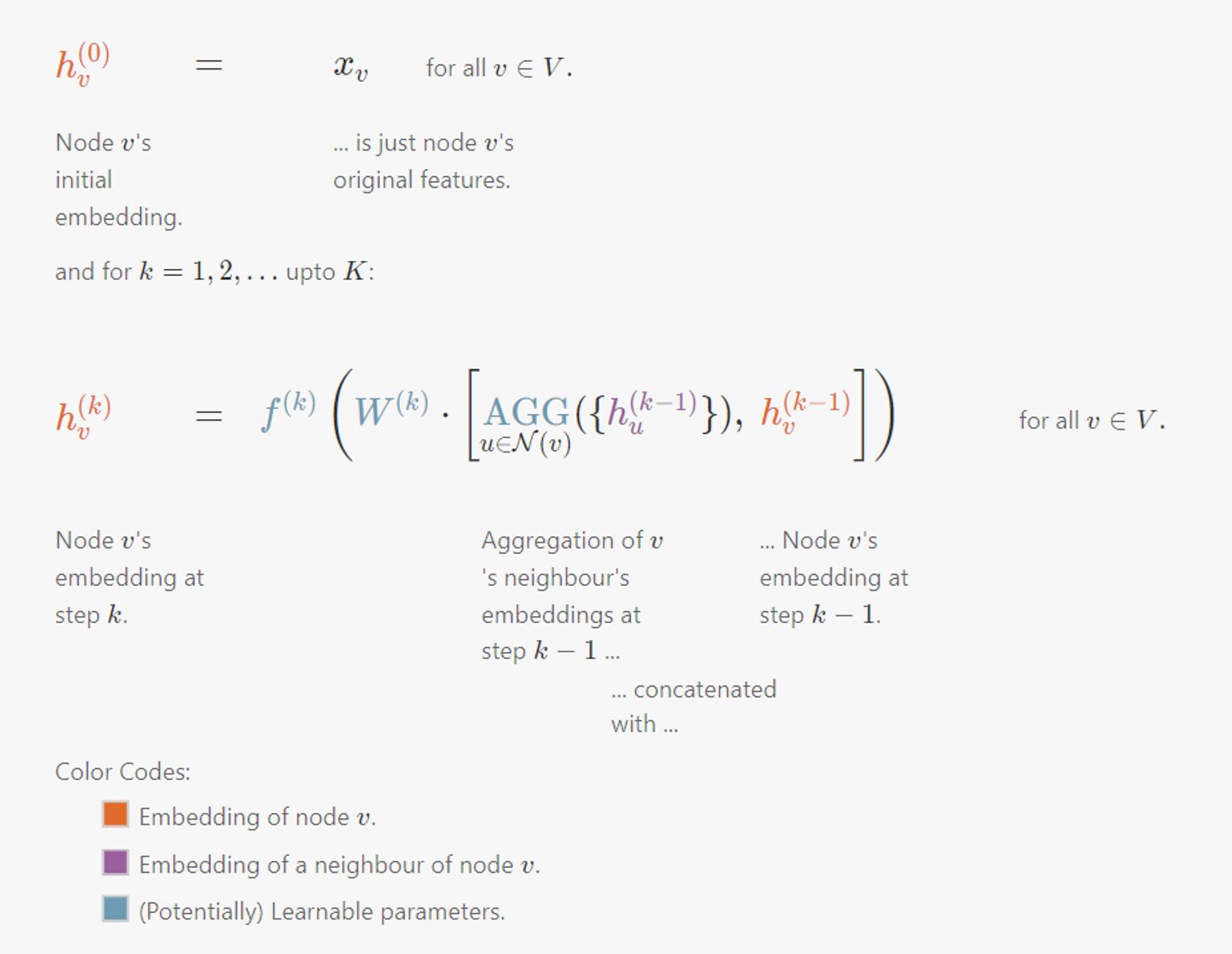
-
각 k 단계별로 학습가능한 function f(k), matrics W(k), AGG는 모든 노드들에 걸쳐 공유됨
-
AGG의 선택
- Mean(GCN과 유사)
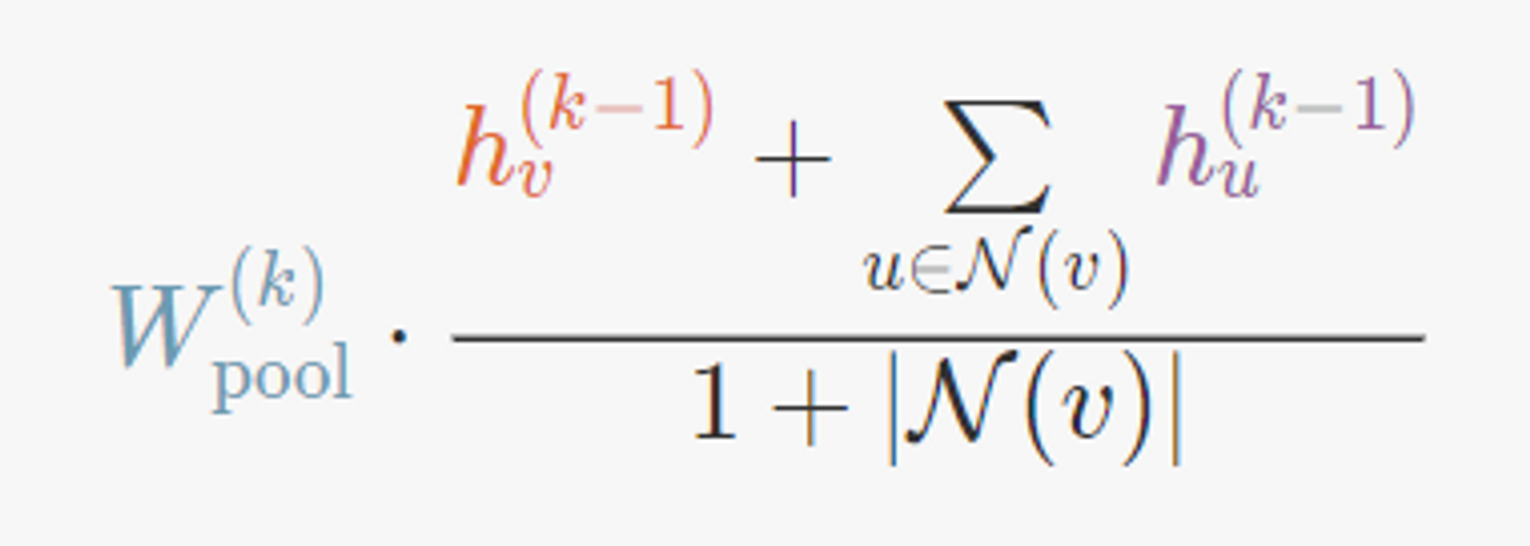
- Dimension-wise Maximum
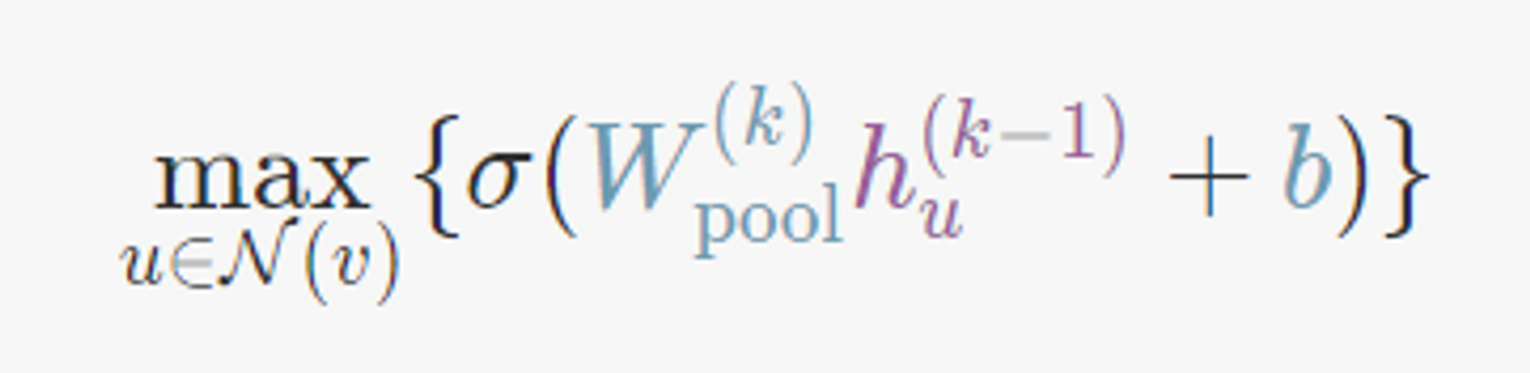
- LSTM (after ordering the sequence of neighbours)
-
neighbourhood sampling : 노드의 이웃노드 수에 관계 없이 고정된 수의 노드들을 random sampling하여 GraphSAGE가 매우 큰 그래프에도 적용될 수 있게됨, Node embedding의 variance는 증가
-
Graph Isomorphism Network (GIN)
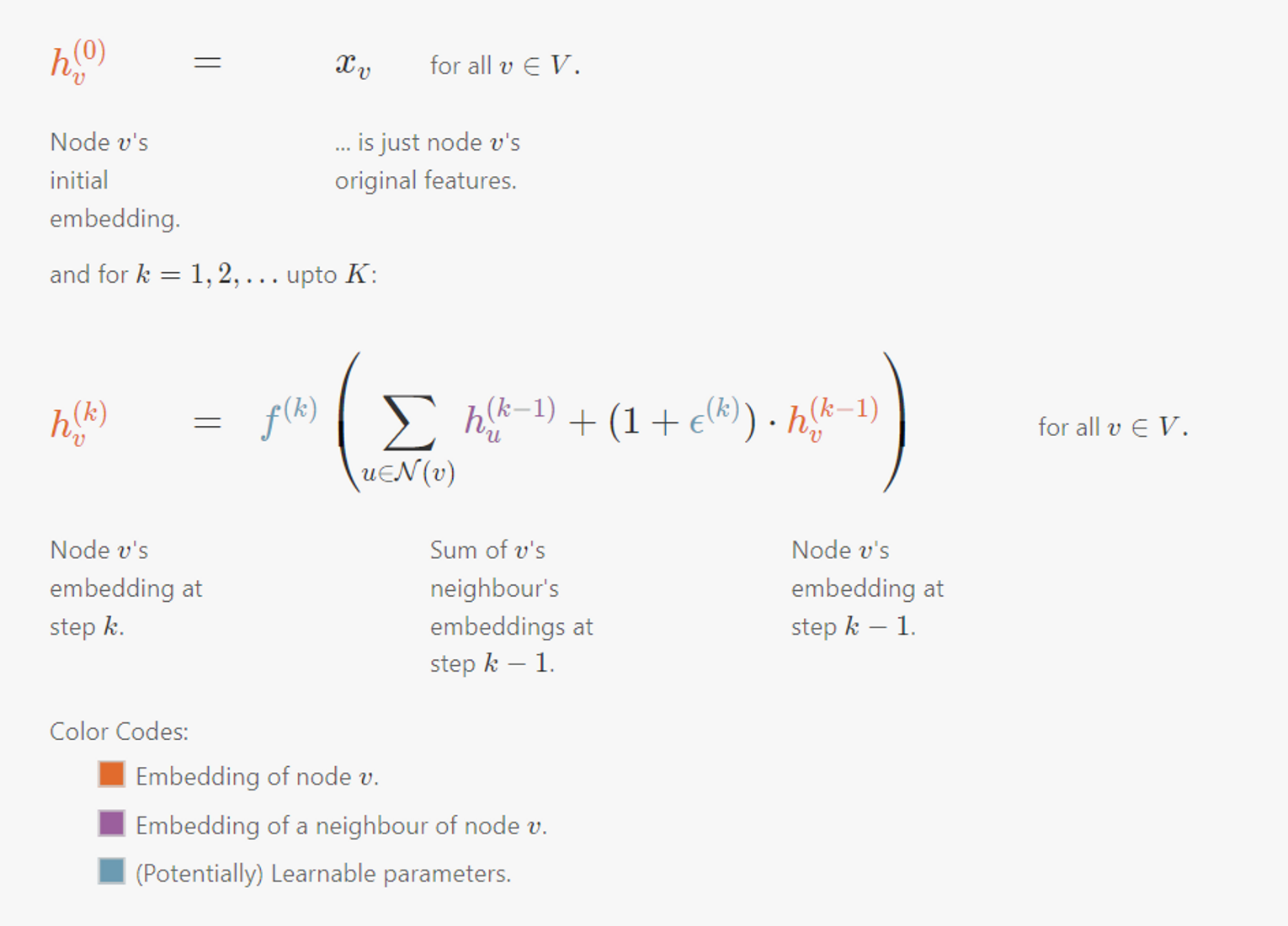
- 각 k 단계별로 학습가능한 function f(k), real-valued parameter ϵ(k)는 모든 노드들에 걸쳐 공유됨
Prediction
- 최종적으로 계산된 embedding을 사용해 각 노드에 대한 prediction을 만들어내는 방법은 다음과 같다.
yv^=PREDICT(hv(K))
- PREDICT : 또다른 Neural Net, 각 GNN을 학습할 때 같이 학습
From Local to Global Convolutions
message passing을 반복적으로 수행하여 그래프 전체에서 한 노드로 정보가 모일 수 있지만, 직접적으로 global convolution을 수행하는 방법이 있음
Spectral Convolutions
💡 feature vector x에 대해, Laplacian L을 사용해 G에 관해 x가 얼마나 smooth한지 quantify할 수 있음
∑i=1nxi2=1을 만족하는, 즉, normalize된 x에 대해
Rayleigh quotient를 정의하면 다음과 같다.
RL(x)=xTxxTLx=∑ixi2∑(i,j)∈E(xi−xj)2=(i,j)∈E∑(xi−xj)2
- Laplacian matrix의 value가 0이면 두 노드는 인접하지 않음.
- L=D−A
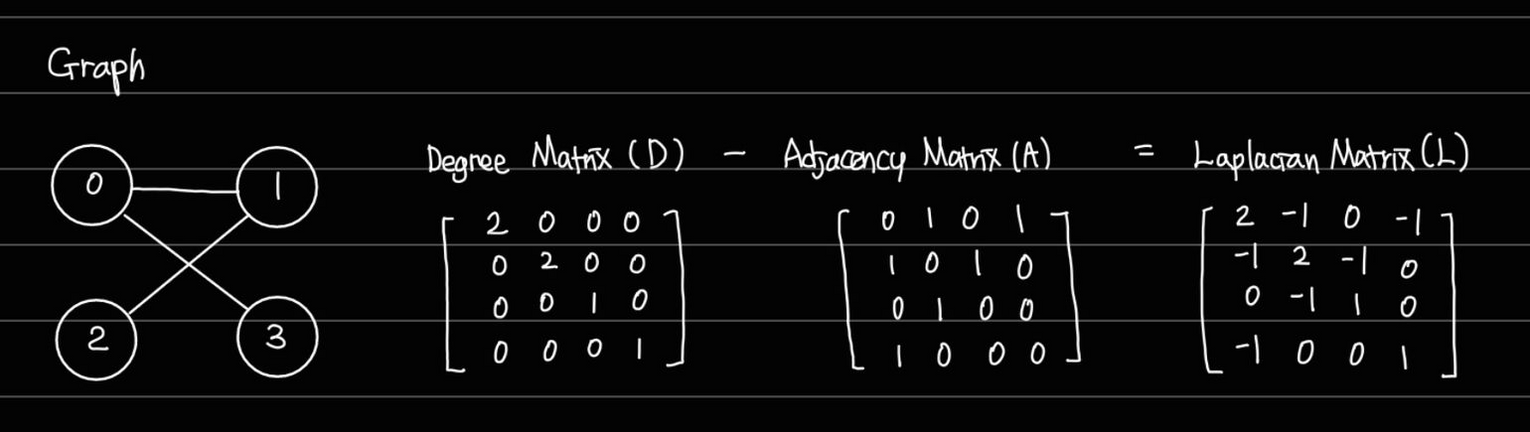
- 인접한 노드 feature의 차이의 제곱으로 정리할 수 있음
- = Smoothness
- 인접한 노드 feature가 유사하면 RL(x) 값이 작아짐
Laplacian matrix L
- Real, symmetric matrix
- eigenvalues : λ1≤⋯≤λn이
- 이에 대응하는 u1,…,un는 orthonormal
uk1Tuk2={10if k1=k2,if k1=k2.
argminx,x⊥{u1,…,ui−1}RL(x)=ui.
minx,x⊥{u1,…,ui−1}RL(x)=λi.
- L의 eigenvectors → successively less smooth
- L의 eigenvalue들을 “spectrum”이라고 할때, L의 spectral decomposition은
L=UΛUT
- Λ : 정렬된 eigenvalue들의 대각행렬 = ⎣⎢⎡λ1⋱λn⎦⎥⎤
- U : eigenvector들의 matrix, 정렬된 eigenvalue에 대응 = [u1…un]
eigenvector들은 orthonormality condition을 만족 : UTU=I
eigenvector들은 Rn의 basis로 다음과 같이 linear combination으로 feature vector x를 나타낼 수 있음
x=i=1∑nxi^ui=Ux^
- x^ : [x0…xn]의 coefficient, spectral representation of vector x
- orthonormality condition을 x=Ux^에 적용하면, natural representation x와 spectral representation x^ 사이 변환식으로 정리할 수 있음
x=Ux^ ⟺ UTx=x^
Embedding Computation
- k layers → each layers’ learnable parameter w^(k) = filter weights
- spectral representation을 계산하는데 필요한 eigenvector의 수 = m = 각 layer에 필요한 weight의 수
- spectral domain에서 convolution을 적용하면 direct convolution보다 더 적은 파라미터를 사용해도 됨
- Laplacian eigenvector들의 smoothness덕분에, spectral representation을 사용하면 자연스럽게 주변 노드들이 유사한 representation을 갖도록하는 inductive vias를 강화할 수 있음
- node feature가 1d라고 할 때, 각 layer의 output은 node representation h(k)의 벡터로, 각 행은 node의 representation을 의미
h(k)=⎣⎢⎢⎢⎡h1(k)⋮hn(k)⎦⎥⎥⎥⎤for each k=0,1,2,…up to K
Graph G
- adjacency matrix A
- degree matrix D
- Lapalcian L=D−A
- Λ : 정렬된 eigenvalue들의 대각행렬 = ⎣⎢⎡λ1⋱λn⎦⎥⎤
- U : eigenvector들의 matrix, 정렬된 eigenvalue에 대응 = [u1…un]
Computed node embeddingsLearnable parameters
- Start with original feature h(0)=x
- Iterate, for k=1,2,… upto K
-
Convert previous feature h(k−1) to its spectral representation h^(k−1)
: spectral representation으로 변환하기
h^(k−1)=UmTh(k−1)
-
Convolve with filter weights w^(k) in the spectral domain to get g^(k), ⊙ = element-wise multiplication
: weight가 w^(k)인 filter로 spectral domain에서의 convolution적용
g^(k)=w^(k)⊙h^(k−1)
-
Convert g^(k) back to its natural representation g(k)
: spectral → natural
g(k)=Umg^(k)
-
Apply a non-linearity σ to g(k) to get h(k)
h(k)=σ(g(k))
Spectral Convolutions are Node-Order Equivariant
Laplacian polynomial filter의 node equivariant 증명에서 보인 것과 유사한 접근으로 증명 가능
- proof. node order의 변경 : permutation matrix P( = 행 교환을 수행하는 행렬)와의 내적 그에 따라 다음과 같은 식의 변경 발생
xALUm→Px→PAPT→PLPT→PUm embedding computation에는 다음과 같은 변경x^w^g^g→(PUm)T(Px)=UmTx=x^→(PUm)T(Pw)=UmTw=w^→g^→(PUm)g^=P(Umg^)=Pg σ 가 elemnetwise 방향으로 적용되므로f(Px)=σ(Pg)=Pσ(g)=Pf(x) 와 같이 정리할 수 있다.
+) spectral의 x^,w^,g^는 node permutation이 되더라도 변하지 않는다.
Spectral convolution의 단점
- L로부터 eigenvector matrix Um을 계산해야 하는데, 아주 큰 그래프에 대해서 이것이 불가능해질 수도 있다.
- U_m 을 계산해내더라도, global convolution을 계산하는 것이 Um, UmT 을 반복적으로 곱해주어야해서 비효율적일 수 있다.
- 학습된 필터가 input그래프의 Laplacian L의 spectral decomposition에 관한것이기 때문에 그래프 specific해서 다른 구조를 가진 그래프에 transfer하여 적용하기 어렵다.
Global Propagation via Graph Embeddings
graph-level information을 node와 edge의 정보를 pooling함으로써 전체 그래프의 embedding을 계산하고, 그래프 embedding을 사용해 노드 embedding을 업데이트하여 전체 그래프의 embedding을 계산 → Relational inductive biases, deep learning, and graph networks
- spectral convolution이 잡아낼 수 있는 그래프의 topology를 무시하는 경향이 있음
Learning GNN Parameters
embedding computations → completely differentiable ⇒ end-to-end training이 가능
Task에 따른 Loss function L
Node Classification
L(yv,y^v)=−c∑yvclogy^vc
- y^vc : node v가 class c로 예측될 확률
- cross-entropy loss
- semi-supervised setting에서는 다음과 같이 정의할 수 있음
LG=∣Lab(G)∣∑v∈Lab(G)L(yv,y^v)
- Lab(G) : labelled nodes에 대해서만 loss계산
Graph Classification
node representation을 aggregate하여 전체 그래프에 대한 representation생성
- Pooling
hG=PREDICTG(AGGv∈G({hv}))
Link Prediction
adjacent, non-adjacent한 노드 쌍을 sampling하여 이 벡터 쌍을 연결 유무를 예측하기 위해 사용
L(yv,yu,evu)pvu=−evulog(pvu) − (1−evu)log(1−pvu)=σ(yvTyu)
- evu : node v와 u사이에 edge 가 있으면 1, otherwise 0
Others
NLP에서 ELMo나BERT에서 적용된 테크닉을 GNN에 적용해볼 수 있음
- local graph properties (eg. node degrees, clustering coefficient, masked node attributes)
- global graph properties (eg. pairwise distances, masked global attributes)
인접 노드가 비슷한 embedding을 갖도록 하기 위한 self-supervised technique 중 node2vec이나 DeepWalk등 random-walk와 유사한 접근을 하기도 함
LG=v∑u∈NR(v)∑log∑u′expzu′TzuexpzvTzu
- NR(v) : node v에서 시작하여 random-walk를 통해 방문한 node들의 multi-set
아주 큰 그래프에 대해서는 Noise Contrastive Estimation을 적용하기도 함