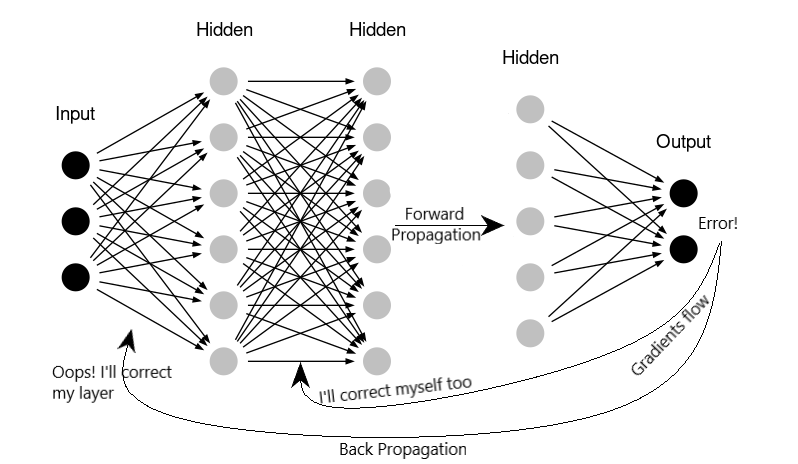
- 효과
1. 학습 속도 개선
2. 가중치 초기값(Initial weights)의 의존도 감소
3. 과적합 방지
4. 기울기 손실(Gradient vanishing) 문제 해결
- 계층 정규화(Layer normalization) : 주로 순환 신경망에서 배치 정규화와 비슷한 교화가 있으며 빠르게 수렴하게 도와준다.
- 계층 정규화는 네트워크 각 층의 출력 분포를 정규화하는 것이다.
class LayerNorm(nn.Module):
def __init__(self, hidden_size, eps=1e-5):
"""Construct a layernorm module in the TF style (epsilon inside the square root).
"""
super(LayerNorm, self).__init__()
self.weight = nn.Parameter(torch.ones(hidden_size))
self.bias = nn.Parameter(torch.zeros(hidden_size))
self.variance_epsilon = eps
self.init_weights()
def init_weights(self):
self.weight.data.fill_(1.0)
self.bias.data.zero_()
def forward(self, x):
u = x.mean(-1, keepdim=True)
s = (x - u).pow(2).mean(-1, keepdim=True)
x = (x - u) / torch.sqrt(s + self.variance_epsilon)
return self.weight * x + self.bias