논문 제목: NNrepair: Constraint-based Repair of Neural Network Classifiers
📕 Summary
Abstract
- NNrepair is a technique for repairing neural network classifiers by identifying faulty network parameters and applying small modifications to fix them.
- It improves the overall accuracy of models, fixes security vulnerabilities caused by poisoned training data, and enhances the network's robustness against adversarial attacks
Introduction
-
Neural networks are widely used in various applications, but they can have defects that need repair, such as low accuracy, vulnerability to adversarial attacks, or poisoning of training data .
-
Traditional methods like retraining can be difficult and expensive, resulting in a network that is different from the original one
-
The paper introduces NNrepair, a novel constraint-solving based approach for repairing neural networks in three scenarios: improving overall accuracy, fixing security vulnerabilities caused by poisoned data, and enhancing robustness against adversarial attacks
-
NNrepair uses fault localization to identify potentially faulty network parameters and applies constraint solving to make small modifications to remedy the defects
-
The focus is on repairing the learnable parameters of the neural network model, specifically the weights on the edges connecting neurons
-
The evaluation on MNIST and CIFAR-10 models shows that NNrepair significantly improves accuracy on poisoned and adversarial data, as well as providing small improvements in overall accuracy without requiring new data or re-training
📕 Solution
- NNrepair is a constraint-based technique that uses fault localization to identify potentially faulty network parameters and applies constraint solving to make small modifications to remedy the defects.
- The technique incorporates strategies such as inferring correctness specifications for intermediate layer repair and generating experts for each class.
- NNrepair is implemented in the NNrepair tool pipeline, which is based on NeuroSPF. It translates a trained Keras model into Java, uses Symbolic PathFinder (SPF) for concolic execution, and z3 for constraint solving.
- The evaluation of NNrepair is conducted on MNIST and CIFAR-10 models, using two architectures for MNIST and a 15-layer CNN for CIFAR-10. The results are presented in Table 2, showing the improvement in accuracy obtained over the original models for different repair scenarios.
Algorithm
Overview of the approach
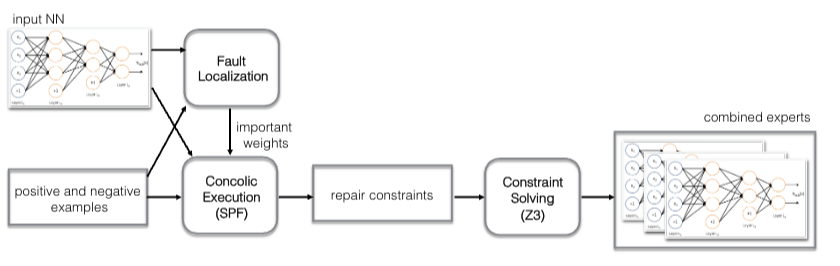
two types of NN-REPAIR
1. Intermediate-layer repair
2. Last-layter repair
4.1. Intermediate-layer repair

4.2. Last-layter repair
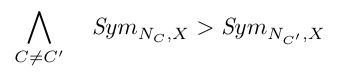
three scenarios: improving the overall accuracy, fixing security vulnerabilities caused by data poisoning and improving the adversarial robustness of the networks
Inference time comparison (Naive Combination Strategy)
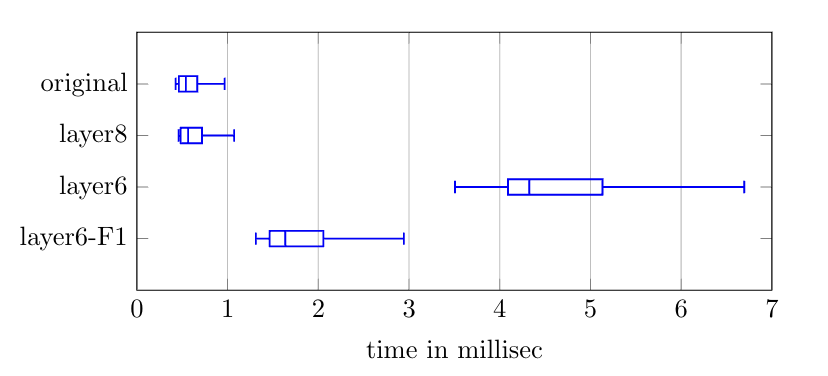
📕 Conclusion
Summary of NN-REPAIR performance on all models
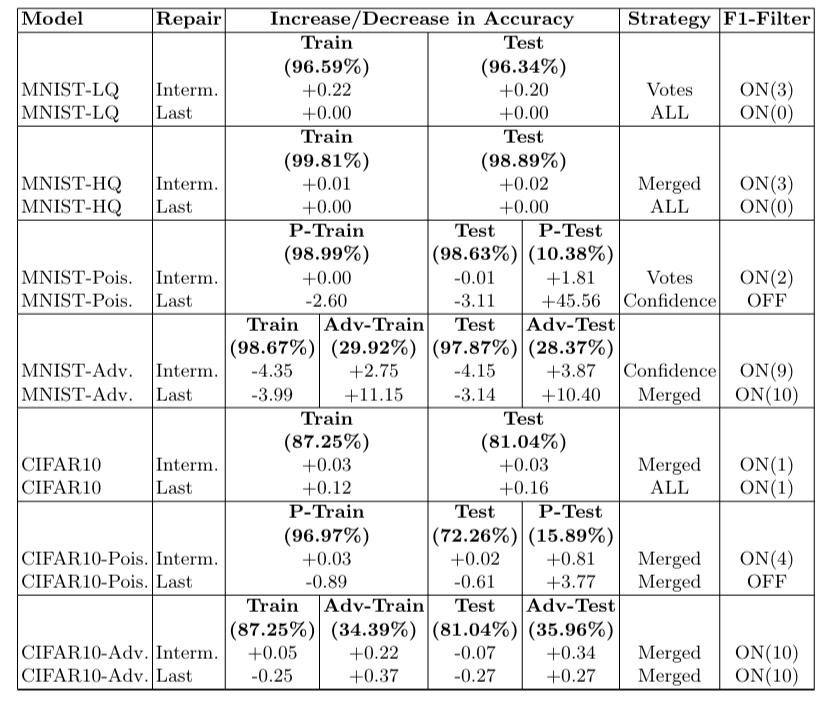
Contribution
- The paper introduces NNrepair, a constraint-based technique for repairing neural network classifiers, addressing three scenarios: improving overall accuracy, fixing security vulnerabilities caused by poisoned data, and enhancing robustness against adversarial attacks.
- NNrepair uses fault localization to identify potentially faulty network parameters and applies constraint solving to make small modifications to remedy the defects.
- The technique incorporates novel strategies such as inferring correctness specifications for intermediate layer repair and generating experts for each class.
- Evaluation on MNIST and CIFAR-10 models demonstrates that NNrepair significantly improves accuracy on poisoned and adversarial data, as well as providing small improvements in overall accuracy without requiring new data or re-training.
- NNrepair contributes to the field of neural network repair by providing a precise yet efficient approach to fixing network logic and enhancing the performance and security of neural network classifiers.