๐ ์ด๋ฒ ํฌ์คํ
์ ๊ณ ๋ ค๋ํ๊ต ๊น์ฑ๋ฒ ๊ต์๋์ ๊ฐ์์์์ ์ฐธ๊ณ ํ์ฌ ๋ฐ๋๊ธฐ๋ฐ ์ด์์น ํ์ง ์๊ณ ๋ฆฌ์ฆ ์ค LOF์ ๋ํด ๋ค๋ฃจ๊ณ ์ ํฉ๋๋ค.
์ฐ์ Anomaly Detection์ด ๋ฌด์์ธ์ง์ ๋ํด ์์๋ด
์๋ค.
Anomaly Detection (์ด์์น ํ์ง)
Notion
- ์ฝ๊ฒ ๋งํด, ๋ฐ์ดํฐ ์์์ Normal(์ ์) sample๊ณผ Abnormal(๋น์ ์, ์ด์์น, ํน์ด์น) sample์ ๊ตฌ๋ณํด๋ด๋ ๋ฌธ์ ๋ฅผ ์๋ฏธํฉ๋๋ค. ์ฆ, ๋งค์ฐ ๋ง์ ์ ์ ๋ฐ์ดํฐ์์ ๊ทน์์์ ๋น์ ์ ๋ฐ์ดํฐ๋ฅผ ๊ตฌ๋ณํ๋ ๊ฒ์ด ๋ชฉ์ ์
๋๋ค.
- ์ฃผ๋ก ์ด๋ฒคํธ ์ฌ๊ฑด์ด ์ ์ ๋ฐ์ดํฐ์ ๋นํด ์ ์ ๋ฐ์ดํฐ๋ฅผ ๋ค๋ฃจ๋ ๋ถ์ผ์์ ๋ง์ด ์ฌ์ฉํฉ๋๋ค.
- Anomaly Detection์ ํ์ต ๋ฐ์ดํฐ ์
์ ๋น์ ์์ ์ธ sample์ด ํฌํจ๋๋์ง, ๊ฐ sample์ label์ด ์กด์ฌํ๋์ง, ๋น์ ์์ ์ธ sample์ ์ฑ๊ฒฉ์ด ์ ์ sample๊ณผ ์ด๋ป๊ฒ ๋ค๋ฅธ์ง, ์ ์ sample์ class๊ฐ ๋จ์ผ class ์ธ์ง Multi-class ์ธ์ง ๋ฑ์ ๋ฐ๋ผ ๋ค๋ฅธ ์ฉ์ด๋ฅผ ์ฌ์ฉํฉ๋๋ค.
์๋ฅผ ๋ค์ด, Novelty Detection๋ผ๋ ์ฉ์ด๋ ์กด์ฌํ๋ ์ด๋ ์ง๊ธ๊น์ง ๋ฑ์ฅํ์ง ์์์ง๋ง ์ถฉ๋ถํ ๋ฑ์ฅํ ์ ์๋ sample์ ์ฐพ์๋ด๋ ์ฐ๊ตฌ, ์ฆ ๋ฐ์ดํฐ๊ฐ ์ค์ผ์ด ๋์ง ์์ ์ํฉ์ ๊ฐ์ ํ๋ ์ฐ๊ตฌ์ ๊ด๋ จ๋ ์ฉ์ด๋ผ๊ณ ํ ์ ์๊ณ , Outlier Detection์ ๋ฑ์ฅํ ๊ฐ๋ฅ์ฑ์ด ๊ฑฐ์ ์๋, ๋ฐ์ดํฐ์ ์ค์ผ์ด ๋ฐ์ํ์ ๊ฐ๋ฅ์ฑ์ด ์๋ sample์ ์ฐพ์ ๋ด๋ ์ฐ๊ตฌ์ ๊ด๋ จ๋ ์ฉ์ด ์ ๋๋ก ๊ตฌ๋ถํ์ฌ ์ ๋ฆฌํ ์ ์์ต๋๋ค.
Local Outlier Factor (LOF)
Notion
- ๊ฐ๊ฐ์ ๊ด์ธก์น๊ฐ ๋ฐ์ดํฐ ์์์ ์ผ๋ง๋ ๋ฒ์ด๋ ์๋๊ฐ์ ๋ํ ์ ๋(์ด์์น ์ ๋)๋ฅผ ๋ํ๋
๋๋ค. LOF์ ๊ฐ์ฅ ์ค์ํ ํน์ง์ ๋ชจ๋ ๋ฐ์ดํฐ๋ฅผ ์ ์ฒด์ ์ผ๋ก ๊ณ ๋ คํ๋ ๊ฒ์ด ์๋๋ผ, ํด๋น ๊ด์ธก์น์ ์ฃผ๋ณ ๋ฐ์ดํฐ(neighbor)๋ฅผ ์ด์ฉํ์ฌ ๊ตญ์์ (local) ๊ด์ ์ผ๋ก ์ด์์น ์ ๋๋ฅผ ํ์
ํ๋ ๊ฒ์
๋๋ค.
- ์ ์๊ฐ์ฒด๋ ์ฃผ๋ก ์ฃผ๋ณ์ ๋ฐ์ดํฐ๊ฐ ๋ง์ด ์กด์ฌํ๋ฉฐ, ๋ถ๋ ๊ฐ์ฒด๋ ์ฃผ๋ก ๋จ๋
์ผ๋ก ์กด์ฌํจ์ ๊ฐ์ ํฉ๋๋ค.
- ์ฃผ๋ณ ๋ฐ์ดํฐ๋ฅผ ๋ช๊ฐ๊น์ง ๊ณ ๋ คํ ๊ฒ์ธ๊ฐ๋ฅผ ๋ํ๋ด๋ k๋ผ๋ ํ์ดํผ-ํ๋ผ๋ฏธํฐ(hyper-parameter)๋ง ๊ฒฐ์ ํ๋ฉด ๋๋ ์ฅ์ ์ด ์์ต๋๋ค.
- ๋ฐ์ดํฐ๊ฐ ๋ง์์ง์๋ก ๊ณ์ฐ๋ณต์ก๋๊ฐ ์ฌ๋ผ๊ฐ๋ ์๊ณ ๋ฆฌ์ฆ์
๋๋ค.
- ๋ฐ์ดํฐ๊ฐ ๊ณ ์ฐจ์์ธ ๊ฒฝ์ฐ, ์ ์ฐจ์์ผ๋ก ์ฐจ์ ์ถ์ํ์ฌ ์ํํ๋ ๊ฒ์ด ์ข์ต๋๋ค.
Motivation
- ์ง๋จ C1 ๊ณผ ์ง๋จ C2์ density๊ฐ ๋ค๋ฅด๋ฉฐ, ๋ฐ์ดํฐ o1์ ๋์ ๋๊ฒ ๋ค๋ฅธ ๋ฐ์ดํฐ์์ ๊ฑฐ๋ฆฌ๊ฐ ๋ฉ๊ธฐ์ ๊ฑธ๋ฌ๋ด๊ธฐ ์ฝ์ง๋ง ๋ฐ์ดํฐ o2๋ ๊ฑธ๋ฌ๋ด๊ธฐ๊ฐ ์ด๋ ต์ต๋๋ค.
์ผ์ ๊ฑฐ๋ฆฌ๋ก ๊ธฐ์ค์ ์ผ์ ๊ฒฝ์ฐ, C1ํน์ C2์๋ง ํนํ๋ outlier detction์ ํ๊ฒ ๋ฉ๋๋ค.
์ด๋ฌํ ๋ฌธ์ ์์์์, local์ ์๋์ ์ธ dense๋ฅผ ๋น๊ตํ์ฌ outlier๋ฅผ ์ ํ์๋ lof๊ฐ ๋ฑ์ฅํ์ต๋๋ค.
ํฐ ํ์, neighbor๋ค์ dense๋ฅผ ๊ณ ๋ คํ์ฌ ๋น๊ตํ๋ค๋ ๊ฒ์
๋๋ค.
Algorithm
1. K-distance of object p
-
์๊ธฐ ์์ (p)๋ฅผ ์ ์ธํ๊ณ k๋ฒ์งธ๋ก ๊ฐ๊น์ด ์ด์๊ณผ์ ๊ฑฐ๋ฆฌ
-
์์ : 4-distance of object p : ์๊ธฐ ์์ ์ ์ ์ธํ 4๋ฒ์งธ๋ก ๊ฐ๊น์ด ์ด์๊ณผ์ ๊ฑฐ๋ฆฌ = 2.0
-
์ฃผ์์ฌํญ : distance๊ฐ continuous๋ผ๋ฉด 3-distance๋ด์ ์ ํํ 3๊ฐ์ neighbor๊ฐ ๋ค์ด์๊ฒ ์ง๋ง, ๊ฑฐ๋ฆฌ๊ฐ 1,2,3,3,3,3๊ฐ์ด discreteํด์ ๊ฒน์น๋ ๊ฒฝ์ฐ๋ผ๋ฉด 3-distance๋ด์ 5๊ฐ๋ 10๊ฐ๋ neighbor๋ก ๋ค์ด์์ ์๋ ์์ต๋๋ค. ์ด๋ฅผ ๋ฐ๋ก ๋ํ๋ด์ฃผ๊ธฐ ์ํด k-distance(p)์์ ๋ค์ด์จ ๋ฐ์ดํฐ๊ฐฏ์๋ฅผ Nkโ,(p)๋ผ๊ณ ๋ถ๋ฅธ๋ค.
2. K-distance neighborhood of object p ((Nkโ,(p)))
- k๋ฒ์งธ๋ก ๊ฐ๊น์ด ์ด์๊ณผ์ ๊ฑฐ๋ฆฌ๋ฅผ ์์ผ๋ก ํํํ ๋, ์ ์์ ํฌํจ๋๋ ๋ชจ๋ ๊ฐ์ฒด๋ค์ ๊ฐ์
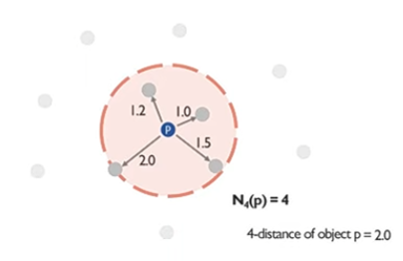
- ์์ : k = 4์ผ ๋, N4โ(p) = 4๊ฐ ํฌํจ
3. Reachabillity Distance (Reachabillitydistancekโ(p,o))
- ์ฃผ๋ณ ๋ฐ์ดํฐ o๋ฅผ ๊ธฐ์ค์ผ๋ก k๋ฒ์งธ ๊ฐ๊น์ด ์ด์๊ณผ์ ๊ฑฐ๋ฆฌ(k-distance of o)์ o์ p์ฌ์ด ๊ฑฐ๋ฆฌ ๊ฐ์ ์ต๋๊ฐ
- Reachabillitydistancekโ(p,o)=max(kโdistanceofo,d(p,o))
- ์์ : k = 5์ผ ๋, k-distance of o๋ 1.2, d(p,o)๋ 2.0์ด๋ค.
Reachabillitydistance5โ(p,o)=max(1.2,2.0)=2.0
4. Local reachabillity density of object p (lrdkโ(p))
- ์๊ธฐ ์์ (p) ์ฃผ๋ณ์ ๋ฐ๋๊ฐ ๋์ ๊ฒฝ์ฐ, ์ ์์ ๋ถ๋ชจ๊ฐ ์์์ง๊ฒ ๋์ด lrdkโ(p)๊ฐ์ด ์ปค์ง๊ฒ ๋ฉ๋๋ค. = ์ ์
- ๋ฐ๋๋ก ์๊ธฐ ์์ (p) ์ฃผ๋ณ์ ๋ฐ๋๊ฐ ๋ฎ์ ๊ฒฝ์ฐ, ์ ์์ ๋ถ๋ชจ๊ฐ ์ปค์ง๊ฒ ๋์ด lrdkโ(p)๊ฐ์ด ์์์ง๊ฒ ๋ฉ๋๋ค. = ๋น์ ์
5. Local Outlier Factor of object p (LOFkโ(p))
- ์๊ธฐ ์์ (p) ์ฃผ๋ณ์ ๋ฐ๋๊ฐ ๋ฎ์ ๊ฒฝ์ฐ, ํฐ lrdkโ(o), ์์ lrdkโ(p) = 1๋ณด๋ค ํฐ LOFkโ(p)๊ฐ์ ๊ฐ์ต๋๋ค.
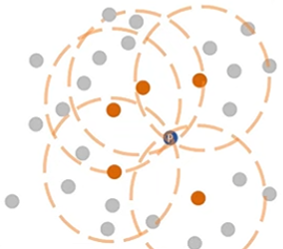
- ํ์ง๋ง ์๊ธฐ ์์ (p) ์ฃผ๋ณ์ ๋ฐ๋๊ฐ ๋ฎ์ง๋ง ์ ์ฒด์ ์ผ๋ก ๋ฐ๋๊ฐ ๋ฎ์ ๊ฒฝ์ฐ, ์์ lrdkโ(o), ์์ lrdkโ(p) = 1๊ทผ๋ฐฉ์ LOFkโ(p)๊ฐ์ ๊ฐ๊ฒ๋ฉ๋๋ค.
- ๊ฒฐ๋ก ์ ์ผ๋ก, 1๊ทผ๋ฐฉ์ ๊ฐ์ ๊ฐ์ง๋ค๋ฉด, ์ค๋ฐ์กฐ๋ฐํ ๊ตฐ์ง์ด๋ ํฌ๊ฒ ํผ์ณ์ง ๊ตฐ์ง์ด๋ ๋ฐ์ดํฐ๊ฐ ๊ทธ ์์ ํฌํจ๋์ด ์๋ค๊ณ ๊ฐ์ฃผํ์ฌ ์ ์์ด๋ผ๊ณ ํ ์ ์๋ค.
Code
import numpy as np
import matplotlib.pyplot as plt
from sklearn.neighbors import LocalOutlierFactor
from matplotlib.pyplot import figure
figure(num=None, figsize=(8, 6), dpi=80, facecolor='w', edgecolor='k')
np.random.seed(123)
X_inliers = 0.3 * np.random.randn(100, 2)
X_inliers = np.r_[X_inliers + 2, 2*X_inliers - 2]
X_outliers = np.random.uniform(low=-4, high=4, size=(20, 2))
X = np.r_[X_inliers, X_outliers]
n_outliers = len(X_outliers)
ground_truth = np.ones(len(X), dtype=int)
ground_truth[-n_outliers:] = -1
clf = LocalOutlierFactor(n_neighbors=20, contamination=0.1)
y_pred = clf.fit_predict(X)
n_errors = (y_pred != ground_truth).sum()
X_scores = clf.negative_outlier_factor_
plt.title("Local Outlier Factor (LOF)")
plt.scatter(X[:, 0], X[:, 1], color='black', s=3., label='Data points')
radius = (X_scores.max() - X_scores) / (X_scores.max() - X_scores.min())
plt.scatter(X[:, 0], X[:, 1], s=500 * radius, edgecolors='g',
facecolors='none', label='Outlier scores')
n = np.copy(X_scores)
n[n>-2] = np.nan
n = np.round(n,2)
n = -1 * n
for i, txt in enumerate(n):
if np.isnan(txt):continue
plt.annotate(txt, (X[i,0], X[i,1]))
legend = plt.legend(loc='upper left')
plt.show()
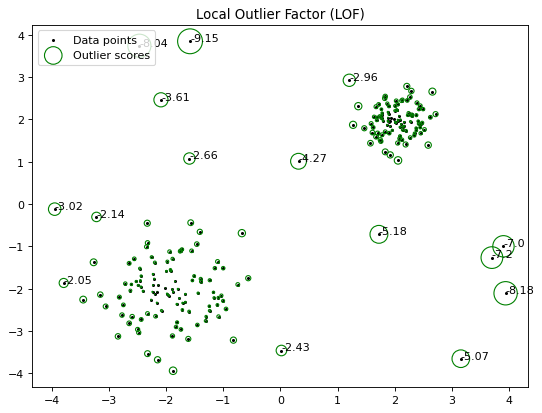