Out-of-distribution with Uncertainty
1. UNCERTAINTY MODELING FOR OUT-OF-DISTRIBUTION GENERALIZATION (ICLR 2022, 48회 인용) Code
- 기존 방법들은 potential domain shift에 의해 발생하는 uncertain statistics discrepancy를 고려하지 않음
- Domain shift에 따른 uncertainty를 모델링함으로써 네트워크 일반화 성능을 향상시킴
- model the feature statistics uncertainty under potential domain shifts and acts as a feature augmentation method for handling our-of-distribution generalization problem.
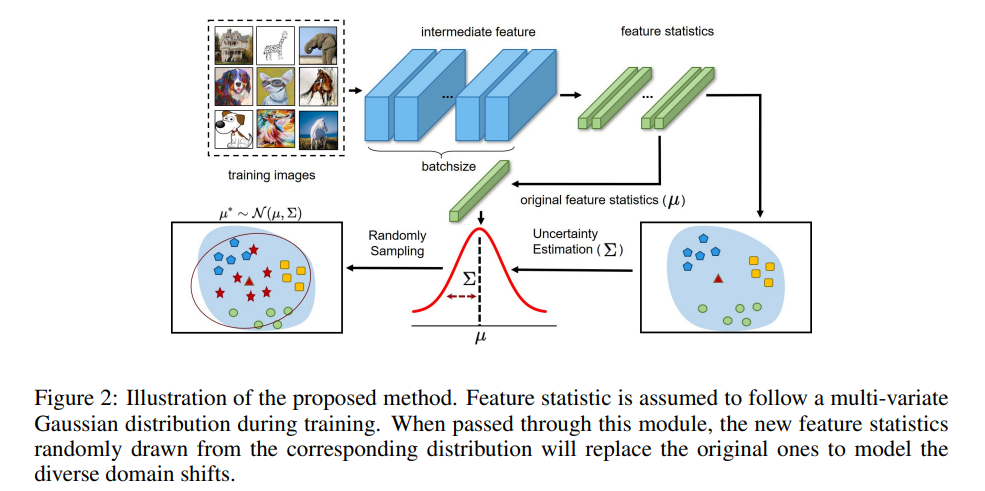
2. BaCOUn: Bayesian Classifers with Out-of-Distribution Uncertainty (ICML 2020,10회 인용)
- 신뢰성 있는 uncertainty estimates를 얻기 위해 Bayesian framework를 제안함
- plug-in "generator"로 학습 데이터 경계에 있는 포인트를 증강함
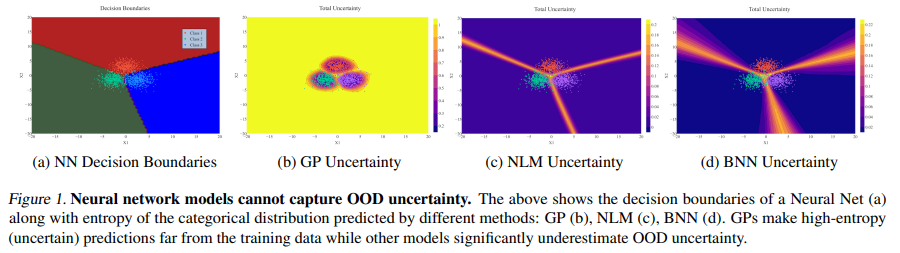
3. Can You Trust Your Model’s Uncertainty? Evaluating Predictive Uncertainty Under Dataset Shift (NeurIPS 2019, 1185회 인용)
- 입력 분포가 변동하는 실제 상황에서 uncertainty를 정량화하는 것은 중요함.
- Uncertainty 분야에서 기존에 Bayesian-and non Bayesian methods을 포함한 확률적 딥러닝 모델들이 등장했지만, Dataset shift에서의 정량적인 비교는 이루어지지 않음.
- 본 논문에서는 기존 최신 방법들로 정확도 및 calibration 측면으로 dataset shift의 효과를 조사함 (총 9가지 방법)
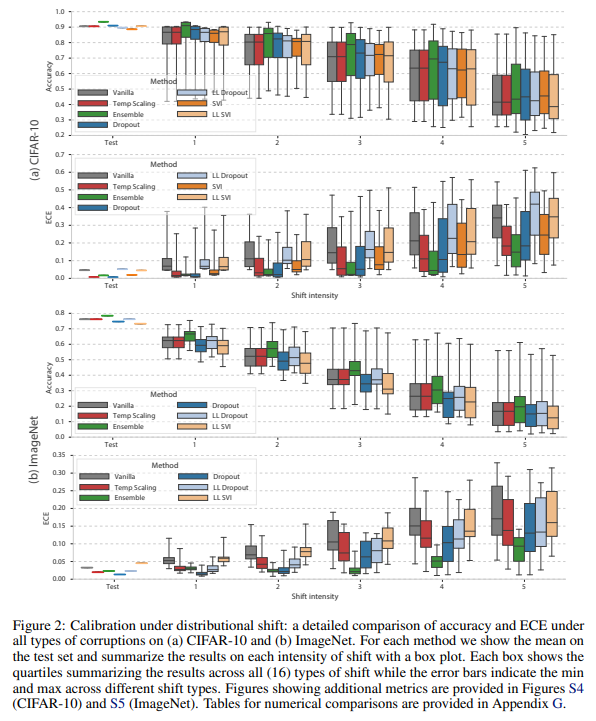