OOD Detection
[목적]
: OOD dataset에서는 분류 결과를 산출하는 것이 무의미하기 때문에 분류 결과를 산출하지 않도록 사전에 탐지하여야 함.
-> ID sample과 OOD sample을 구분할 수 있는 OOD score를 계산하는 것이 방법론의 핵심임
[Partial and Asymmetric Contrastive Learning for Out-of-Distribution Detection in Long-Tailed Recognition]
OOD의 2가지 목적
(1) in-distibution sample로부터 OOD samples을 구분하는 것 (i.e. AD)
(2) in-distribution sample classification에서 높은 성능을 보이는 것
[Baseline method]
1. Maximal Softmax Prediction (MSP)
: 모델의 분류를 위한 output인 Softmax Prediction 값을 Confidence Score로 활용
2. Mahalanobis Distance
: 딥러닝 모델의 Final Layer 직전의 embedding vector를 통해 mahalnobis distance를 구함
- Mahalnobis Distance를 통한 Mahalnobis Score인 M(x)를 계산함
1) ODIN의 input pre-processing technic: ODIN과 동일하게 FGSM을 활용
2) Feature Ensemble: neural network의 모든 layer로부터 mahalabis score를 계산하여 weighted averaging하여 최종 score로 사용함
https://www.youtube.com/watch?v=vAfT77U5Zjk
3. Outlier Exposure
: 활용 가능한 OOD Sample이 존재한다면, OOD Detection의 성능을 향상시킬 수 있음
- OE dataset에 대한 정답 label을 Uniform 분포로 하여 Classifier를 학습함
[Advanced method]
1. ODIN: Enhancing the Reliability of Out-of-distribution Image Detection in Neural Networks (ICLR 2018, 1393회 인용)
- re-training을 사용하지 않는다는 장점이 있음
- temperature scaling과 input에 대한 perturbation을 이용함
- 이는 in과 out의 softmax score 차이를 더 크게 만들어줌.
- log-softmax score가 in-distribution에서 더 큰 gradient를 갖는다는 성질을 활용함
2. Training Confidence-calibrated Classifiers for Detecting Out-of-distribution Samples (ICLR 2018, 731회 인용)
- 기존에는 주어진 pre-trained neural classifier를 기반으로 threshold-based detectors를 사용함.
- 이러한 방법은 inference procedures를 향상시키는데 초점이 맞춰져 있음.
- 본 논문에서는 inference algorithm이 더 잘 작동할 수 있는 방법을 제안함.
- Confidence loss와 GAN loss 사용함
- In-distribution(학습 데이터)주변의 Out-of-distribution을 사용해야 좁은 class boundary 형성할 수 있음.
- GAN으로 생성한 데이터를 OOD sample로 정의하고 Generator, Discriminator, Classifier(←OOD Detection용) 학습
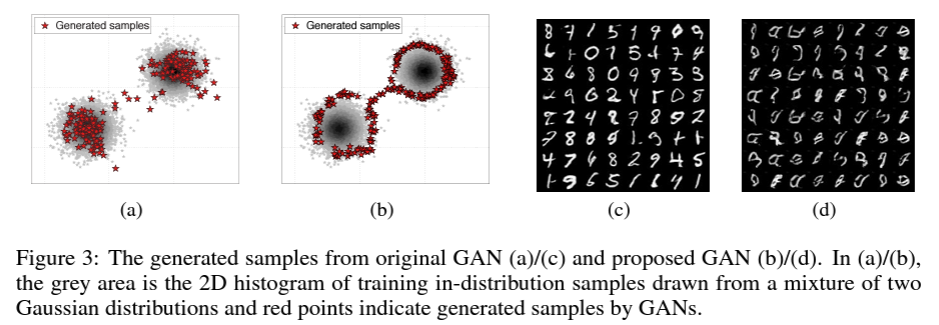
3. Deep Anomaly Detection with Outlier Exposure (ICLR 2019, 1060회 인용)
- OOD에 해당하는 데이터를 학습에 사용하여 각 OOD Detection 방법론에서의 성능을 높임
- MSP에서는, OOD 데이터의 정답 label을 uniform 분포로 하여 classifier 학습함
- Confidence Branch에서는, OOD 데이터의 confidence score가 0으로 가게 학습함
- 어떤 OOD 데이터를 학습에 사용해야 좋은지?
- 다양한 OOD 데이터
- 학습시킨 OOD 데이터와 테스트에 활용한 OOD 데이터의 유사성은 상관이 없지만, In-distribution 데이터와 학습시킨 OOD 데이터는 유사성이 있는 경우 성능이 좋음 (유사성이 높으면 구분이 어렵기 때문)
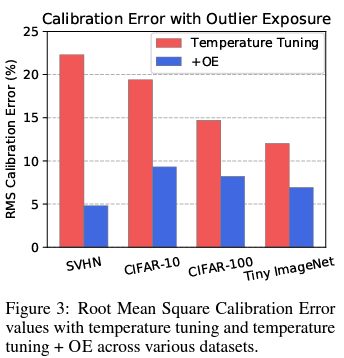
4. Unsupervised Out-of-Distribution Detection by Maximum Classifier Discrepancy (ICCV 2019, 119회 인용)
- 모델의 Feature Extractor에서, 두개의 Classifier Network로 나눈 뒤에 이 두 Classifier의 Softmax값을 비교함으로써 문제를 해결함.
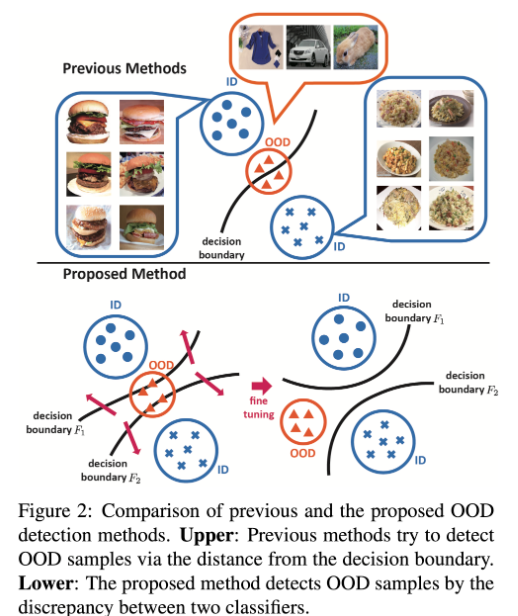
5. CSI: Novelty Detection via Contrastive Learning on Distributionally Shifted Instances (NeurlPS 2020, 355회 인용)
- OOD detection score: 가장 가까운 sample의 코사인 유사도 * Representation vector의 Norm
- 각도 뿐만 아니라 거리도 고려하기 위함 (코사인 유사도를 최대화하는 데는 한계가 있기 때문에 사용함)
- Contrastive learning과 Classification을 동시에 학습함.
- OOD-ness가 높은 transformation은 negative pair를 만드는데 활용함
- 추가적으로, 회전한 각도를 Classification하는 작업도 수행함.
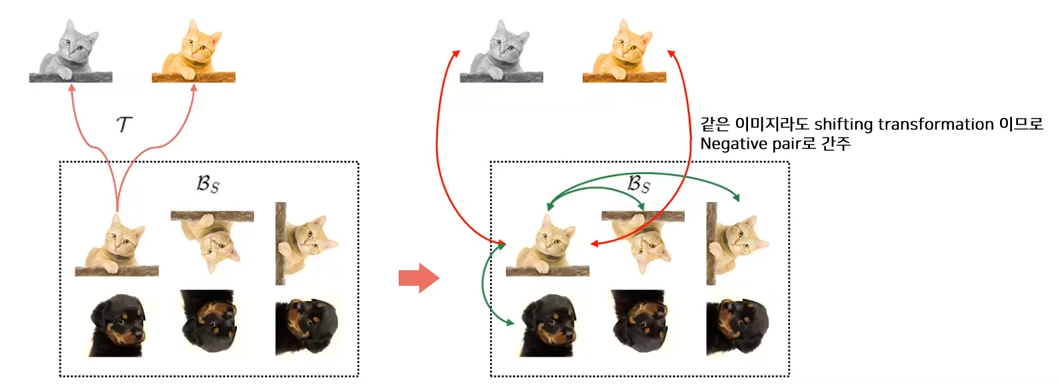
7. Outlier Exposure with Confidence Control for Out-of-Distribution Detection (Neurocomputing 2021, 69회 인용)
- 알고 있는 Class(D_in)를 예측한 confident score를 training accuracy와 근접하도록
- 모르는 class의 sample에 대해서는 DNN이 uncertain하게끔(output으로 uniform 분포 생성하게끔)
- (softmax를 거치면 각 클래스에 속할 확률이 나오는데 binary에서는 이를 accuracy로 해석할 수 있지 않나?)
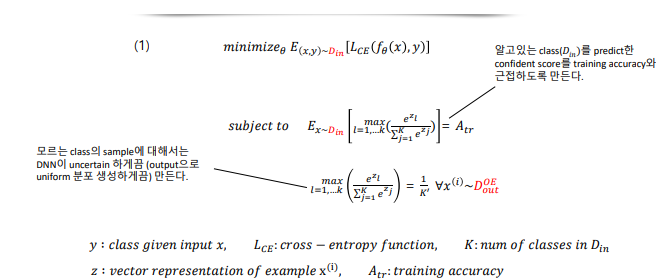
[추가 설명]
-
Novelty Detection은 지금까지 등장하지 않았지만 충분히 등장할 수 있는 sample을 찾아내는 연구, 즉 데이터가 오염이 되지 않은 상황을 가정하는 연구와 관련된 용어라고 할 수 있고, Outlier Detection은 등장할 가능성이 거의 없는, 데이터에 오염이 발생했을 가능성이 있는 sample을 찾아 내는 연구와 관련된 용어 정도로 구분하여 정리할 수 있습니다.
-
정상 sample이 multi-class인 경우, 정상 sample이라는 표현 대신 In-distribution sample이라는 표현을 사용함.
-
시계열로 보면, Novelty Detection은 정상이지만 분포만 변한 경우를 의미하고, Outlier Detection은 이상을 의미한다고 해석하면 될라나?
https://hoya012.github.io/blog/anomaly-detection-overview-1/
[OOD Scoring Functions]
Zhou, W., Liu, F., & Chen, M. (2021). Contrastive out-of-distribution detection for pretrained transformers. arXiv preprint arXiv:2104.08812.
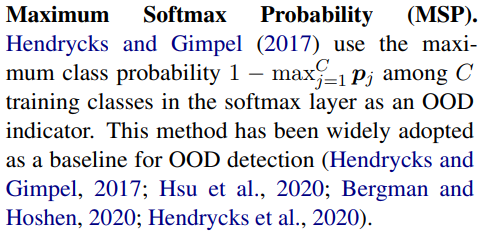
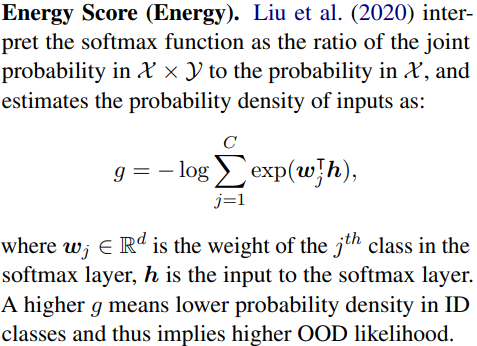
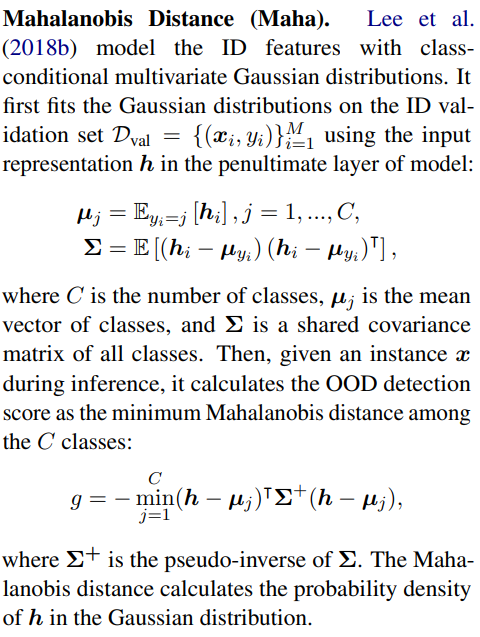
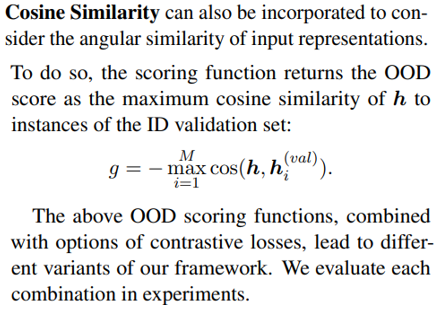