최근 Out-of-distribution in Image/NLP
1. Delving into Out-of-Distribution Detection with Vision-Language Representations (NeurIPS 2022, 12회 인용)
- single-modal이 아닌 multi-modal regime 다룸.
- training free, generalizable to many tasks, scalable to hundreds of classes, and does not require any prior information on OOD inputs 라는 장점을 가짐.
- in-distribution에 대한 text 정보를 주고 in-distribution인 image와 out-of-distribution인 image 구별하기
- in-distribution인 text prototype과 가까운 정도를 OOD uncertainty라고 정의함
- 가까운 정도를 측정하는 거리로는 cosine_similarity 활용
- 모든 text feature vector에 대해서 softmax 형태로 계산한 것 중 가장 큰 값을 선정하고, threshold 지정해서 OOD 구분
- MCM (multi-modal vision-language features) 활용
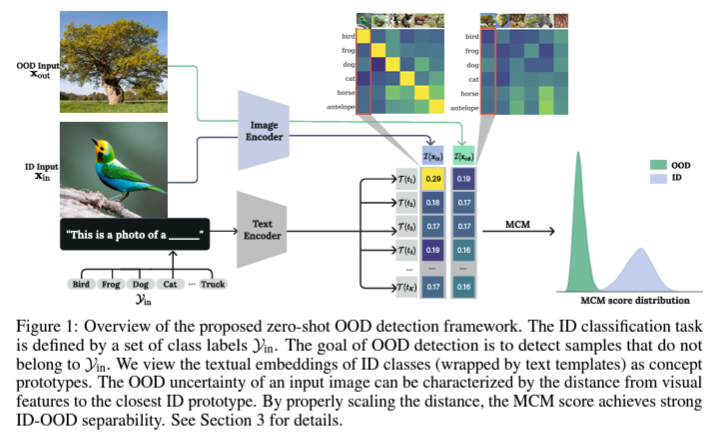
- OOD의 핵심은 ID representation을 잘 학습하는 것임
- recognition-based method가 아닌 reconstruction-based method에서 좋은 성능을 보임을 확인함
- OOD Detection model을 위해 Masked Image Modeling을 pretext task로 사용함
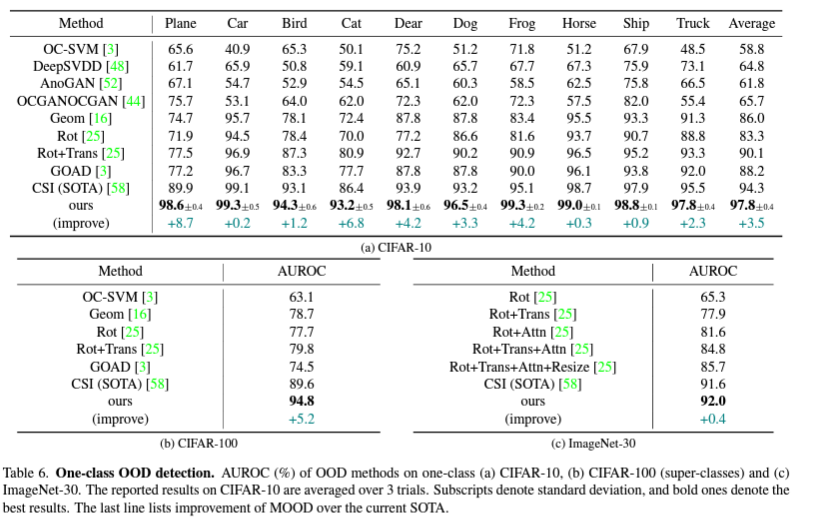
3. SIREN: Shaping Representations for Detecting Out-of-Distribution Objects (NeurIPS 2022, 7회 인용)
- 현장에서 OOD objects를 탐지하는 것은 매우 중요함
- object-level OOD detection은 아직 연구가 필요한 영역임
- 이를 위해 model-agnostic representation space와 서로 다른 신경망에 대해 generality한 것에 의존함
- Mixture of von Mises-Fisher (vMF) distributions를 사용함
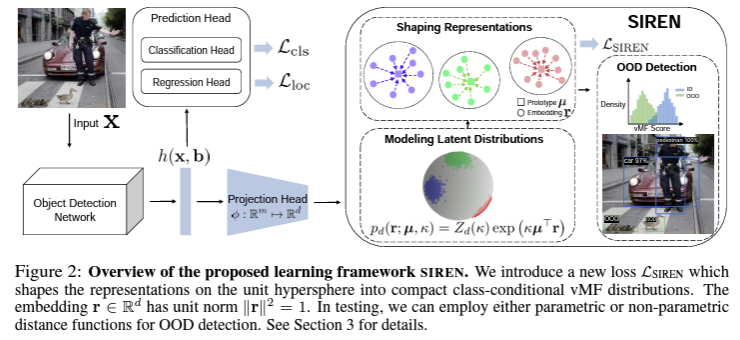
- 학습시 OOD sample에 대한 정보 없이도 가능한 모델 (unsupervised OOD detection model)
- Spectral discrepancy로 Uncertainty 구할 수 있음.
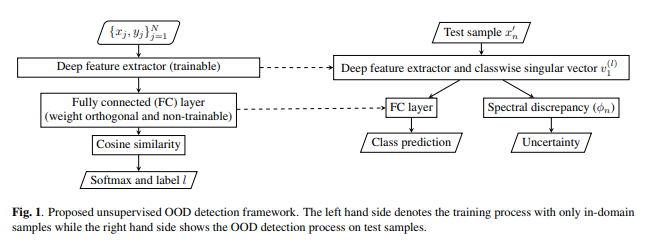
5. BARLE: Background-Aware Representation Learning for Background Shift Out-of-Distribution Detection (EMNLP 2022, 1회 인용 |impact factor: 23.10)
- 머신러닝은 OOD의 영향으로 성능 하락을 겪음
- 기존 OOD detection은 semantic-shift OOD samples에 초점이 맞춰져 있지만, background-shift OOD detection은 여전히 연구가 필요함
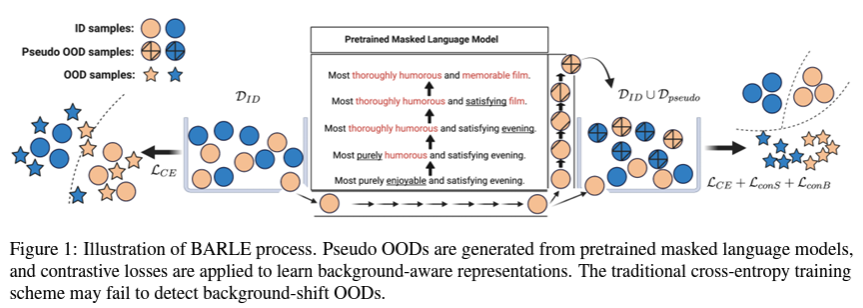
6. Hierarchical VAEs Know What They Don’t Know (PMLR 2021, 35회 인용)
- generative model이 생성은 잘 하지만, OOD에 high likelihood를 부여하는 문제가 존재함
- low-level features들로는 in-distribution과 out-distribution을 구분하기 어려움 (실험적 증명)
→ high-level features 기준으로 봐야 서로 다르다고 판단 가능함
7. Modeling Discriminative Representations for Out-of-Domain Detection with Supervised Contrastive Learning (ACL 2021, 37회 인용)
- OOD에 Contrastive Learning 적용한 근본 논문
- 기존 cross entropy만 사용하는 것은 카테고리 간의 margin을 명확하게 구별하지 못함
→ supervised contrastive loss를 추가하여 intra-class variance를 최소화하고, inter-class variance를 최대화함
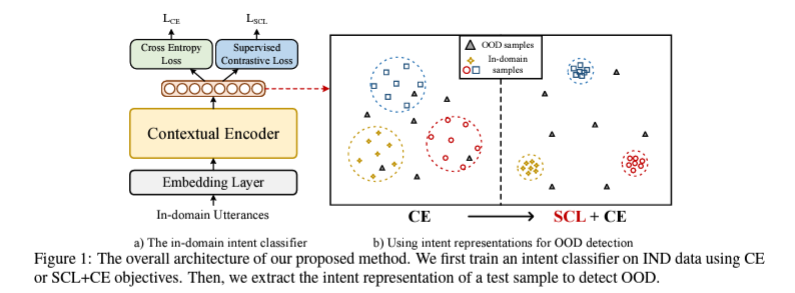
8. Towards Maximizing the Representation Gap between In-Domain & Out-of-Distribution Examples (NeurIPS 2020, 37회 인용)
- Dirichlet Prior Network (DPN)은 uncertainty type을 예측하는 모델임
- 하지만, DPN은 uncertainty가 높은 in-domain examples을 OOD와 구분하지 못하는 한계점 존재함
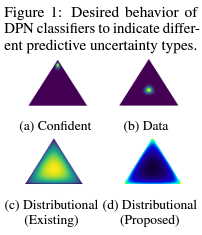
9. STEP: Out-of-Distribution Detection in the Presence of Limited In-Distribution Labeled Data (NeurIPS 2021, 14회 인용)
- 대부분의 SSL(semi-supervised learning) 방법들은 unlabeled data가 labeled data와 같은 분포에서 나왔다고 가정함
- 그러나 실제 상황에서는, OOD distribution에서 나온 샘플도 구분할 수 있어야 함
- Structure-Keep Unzipping 활용함
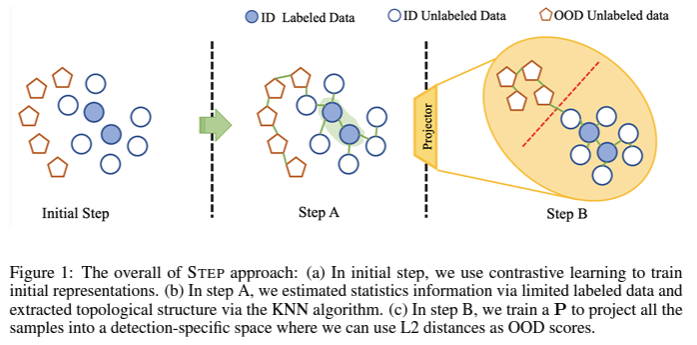