📖 Gradient Descent Methods
- Stochastic gradient descent
- Update with the gradient computed from a single sample.
- Mini-batch gradient descent
- Update with the gradient computed from a subset of data.
- Batch gradient descent
- Update with the gradient computed from the whole data.
📖 Batch-size Matters
- "It has been observed in practice that when using a larger batch there is a degradation in the quality of the model, as measured by its ability to generalize."
- "We ... present numerical evidence that supports the view that large batch methods tend to converge to sharp minimizers of the training and testing functions. In contrast, small-batch methods consistently converge to flat minimizers... this is due to the inherent noise in the gradient estimation."
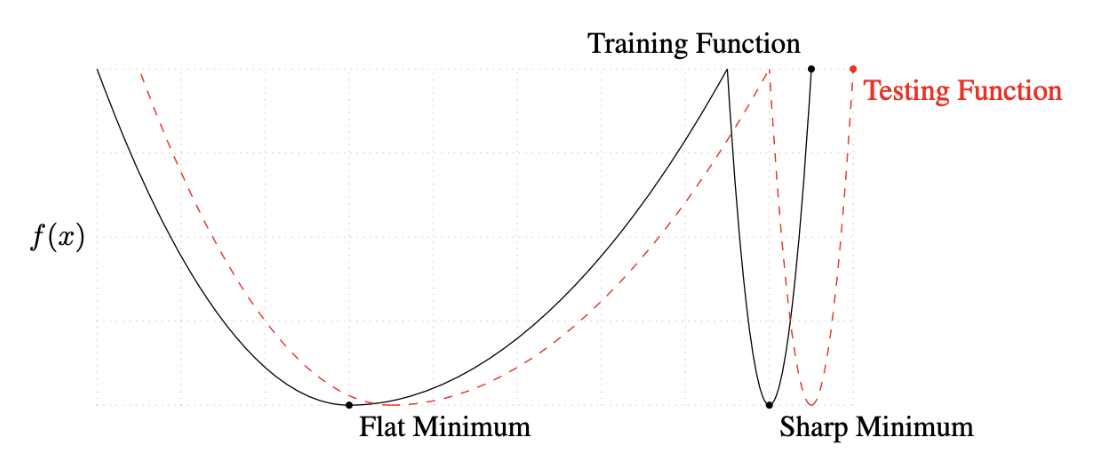
📖 Gradient Descent Methods
- Stochastic gradient descent
- Momentum
- Nesterov accelerated gradient
- Adagrad
- Adadelta
- RMSprop
- Adam
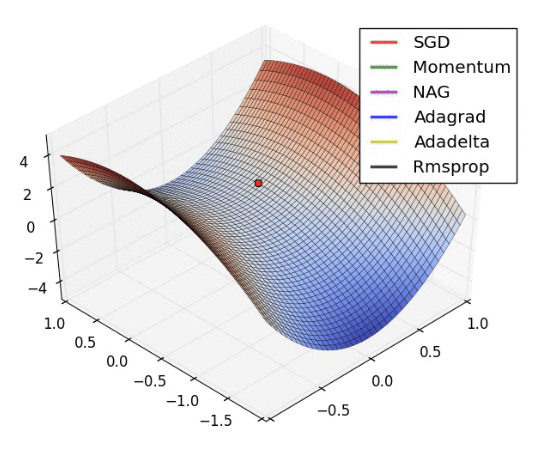
📖 Gradient Descent

📖 Momentum
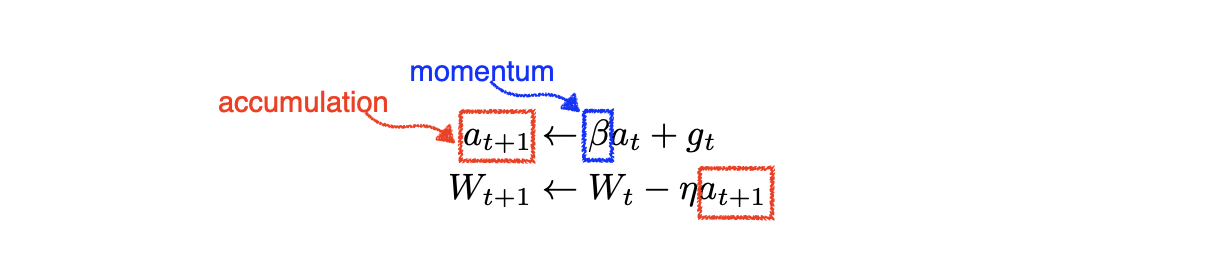
📖 Nesterov Accelerated Gradient

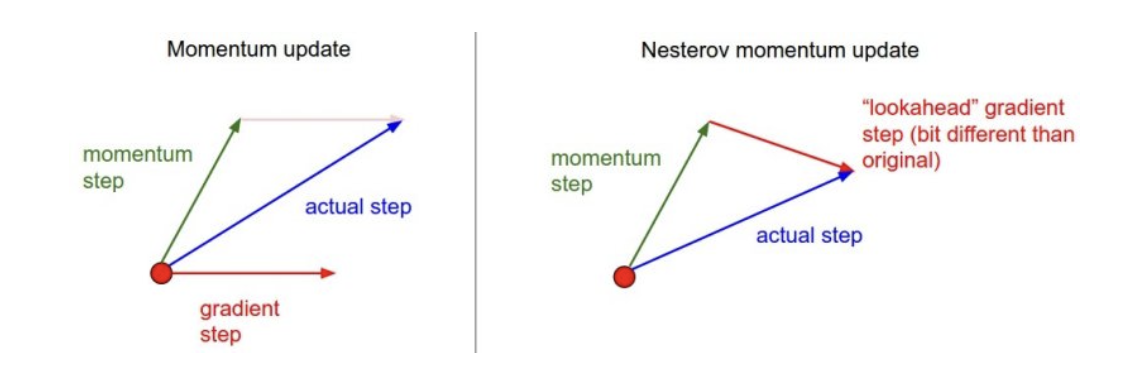
📖 Adagrad
- Adagrad adapts the learning rate, performing larger updates for infrequent and smaller updates for frequent parameters.
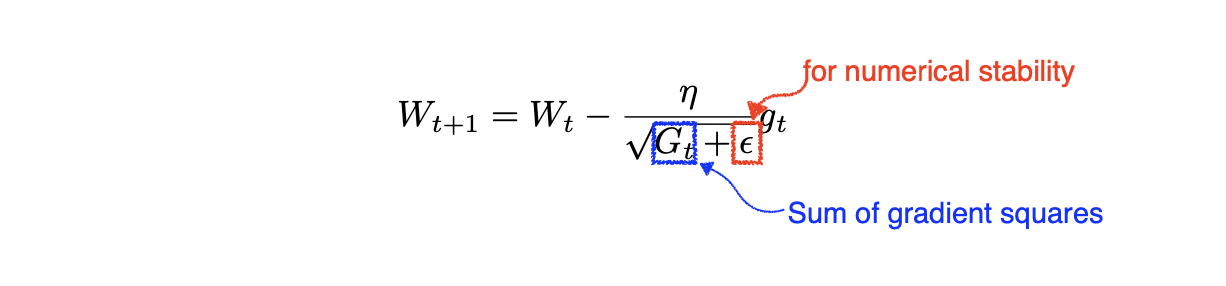
📖 Adadelta
- Adadelta extends Adagrad to reduce its monotonically decreasing the learning rate by restricting the accumulation window.
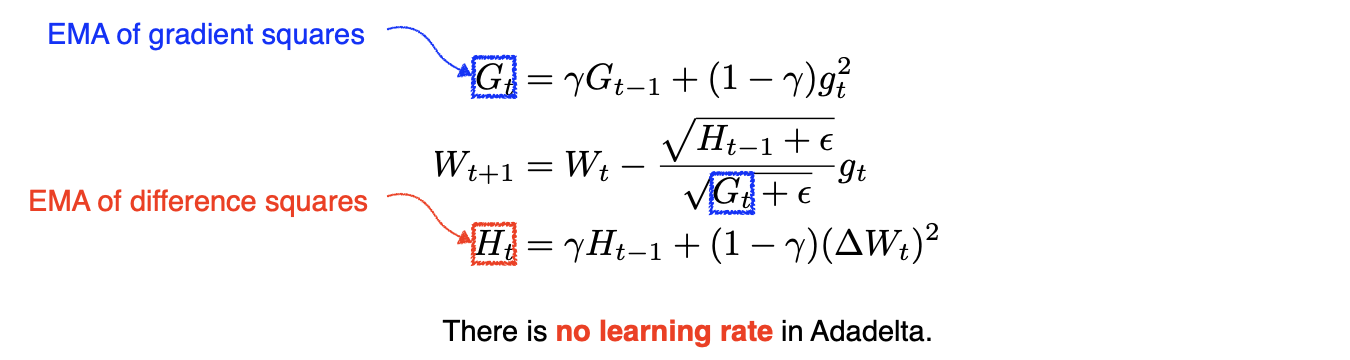
📖 RMSprop
- RMSprop is an unpublished, adaptive learning rate method proposed by Geoff Hinton in his lecture.

📖 Adam
- Adaptive Moment Estimation (Adam) leverages both past gradients and squared gradients.
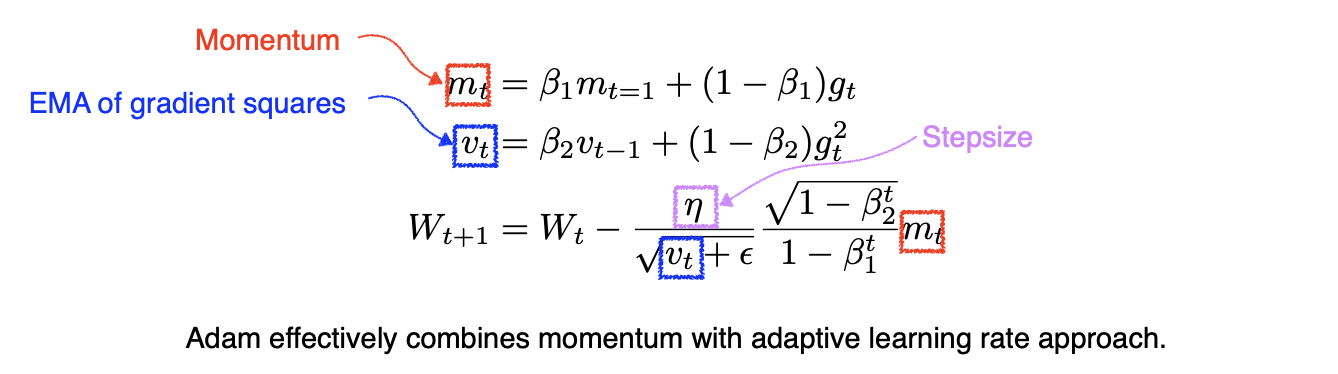
<이 게시물은 최성준 교수님의 'Gradient Descent Methods' 강의 자료를 참고하여 작성되었습니다.>
본 포스트의 학습 내용은 [부스트캠프 AI Tech 5기] Pre-Course 강의 내용을 바탕으로 작성되었습니다.
부스트캠프 AI Tech 5기 Pre-Course는 일정 기간 동안에만 운영되는 강의이며,
AI 관련 강의를 학습하고자 하시는 분들은 부스트코스 AI 강좌에서 기간 제한 없이 학습하실 수 있습니다.
(https://www.boostcourse.org/)