Generative Model Family
🔗 VAE
Posterior Q(Z|X)라는 likelihood function이 있어 sample이 얼마나 가능성 있는지 estimate하는 데에 사용할 수 있다.
🔗 Autogressive Model
- Pixel-CNN
- 이미지를 생성할 때 한 번에 한 pixel를 생성한다.
- WaveNet
- 오디오를 생성할 때 한 번에 한 frame를 생성한다.
- GPT-3
- Text를 생성할 때 한 번에 한 word를 생성한다.
- DaLL-E 1
- 한 번에 한 visual "code"를 생성한다.
- VQ-VAE(Vector Quantization VAE)
- Discretizing your latent variables
- 주어진 image에 대해 visual code를 얻을 수 있다.
- Image가 sequence of code/ sequence of feature maps으로 변환된다. 이것으로 autoregressively하게 어떤 image도 generate할 수 있다.
- 하나의 feature map이 하나의 code로 mapping되기 때문에 latent space에 512개의 feature map이 있으면 512개의 code sequence가 생성된다. 즉, 이미지가 더 이상 pixel이 아니라 512개짜리 code의 배열로 표현될 수 있다.
- 이런 autoencoder로 압축시켜놓고 압축된 space를 이산화시키는 과정은 이미지 뿐만 아니라 audio, time series signal등 아무 데이터에나 적용될 수 있다.
- 후속 model : VQ-GAN v2, Parti
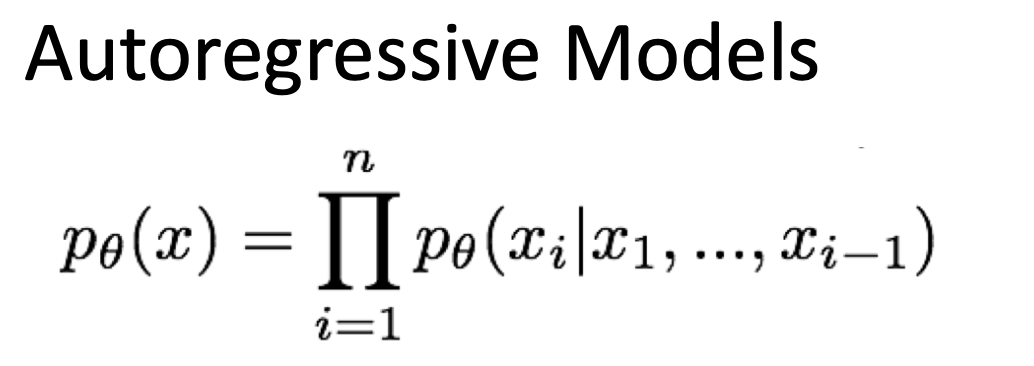
- Autoregressive model에서도 likelihood function을 factorize form으로 분해할 수 있다. 그래서 sample을 generate하고 이 sample이 얼마나 가능성 있는지 likelihood를 estimate할 수 있다.
❓ Data distribution(likelihood)를 신경쓰지 않고 좋은 synthetic sample들만 오직 원한다면?
이런 경우에 GAN을 쓸 수 있다.
🔗 GAN
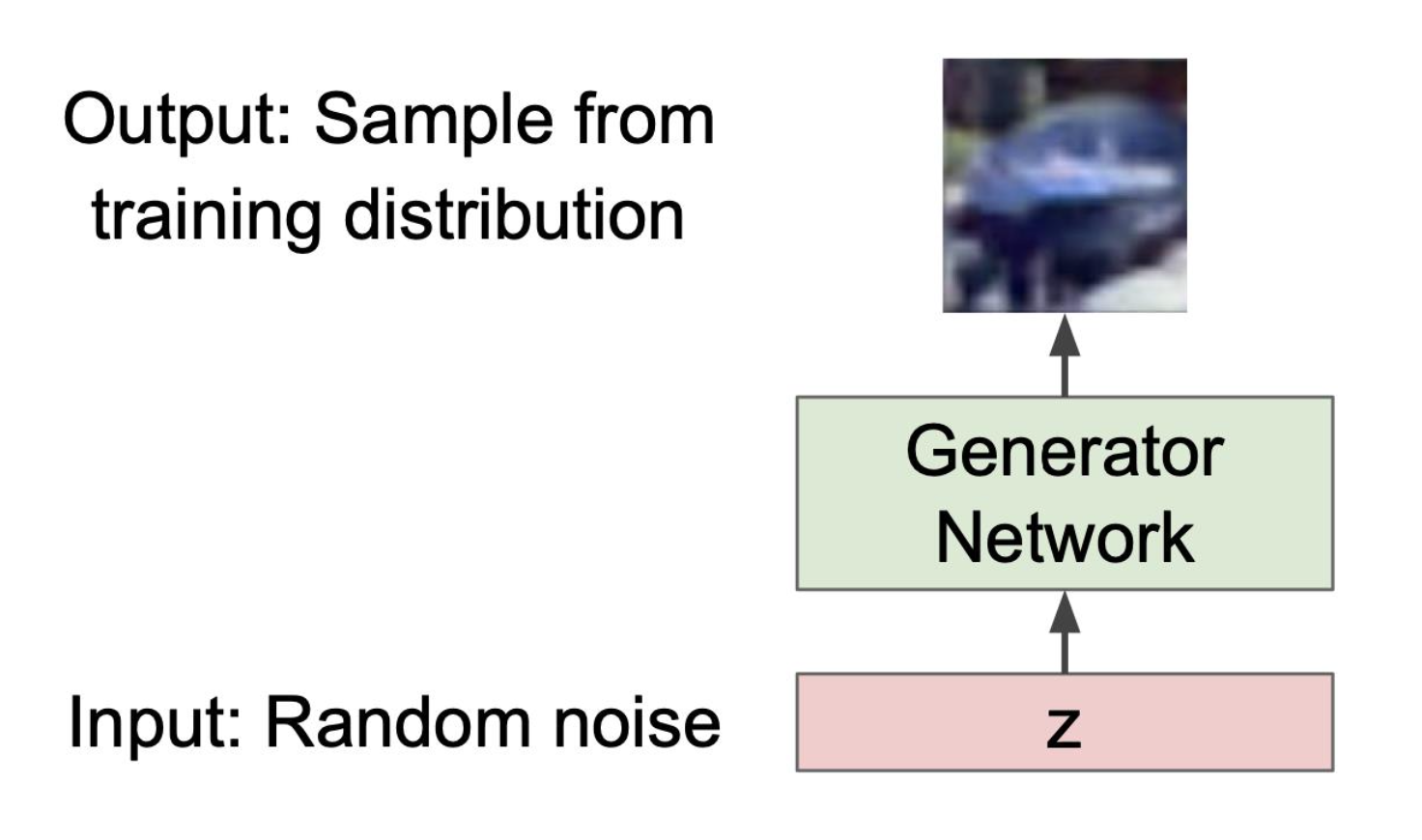
- image의 distribution을 approximate하거나 variational inference를 하지 않는다.
🔗 Diffusion
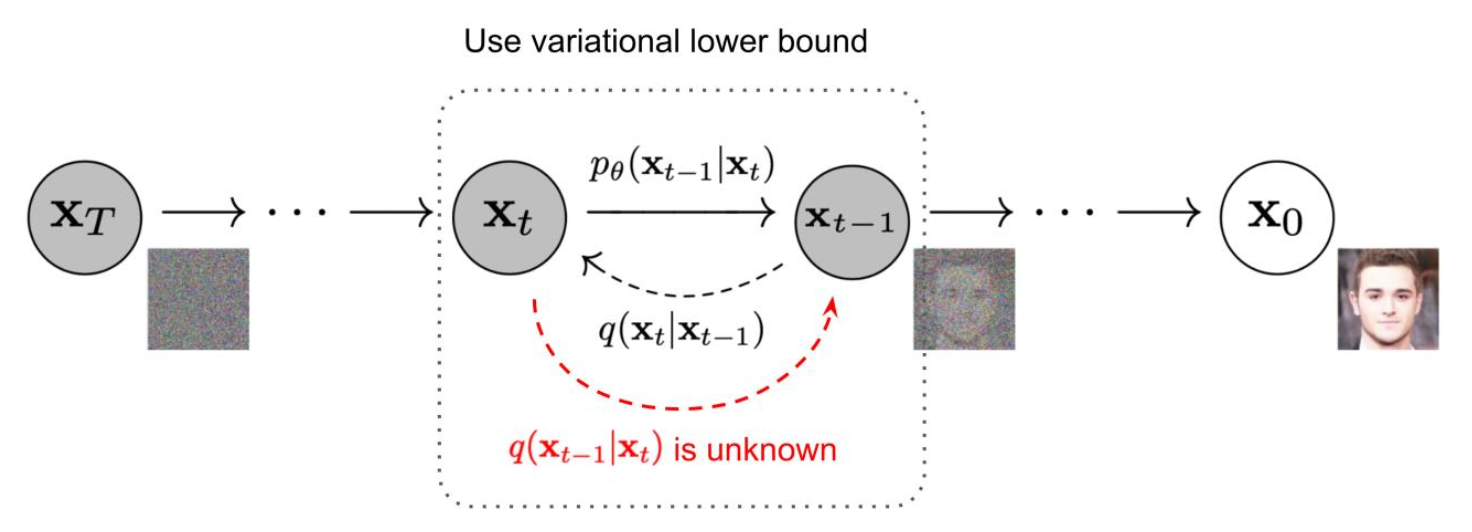
Generative Adversarial Network
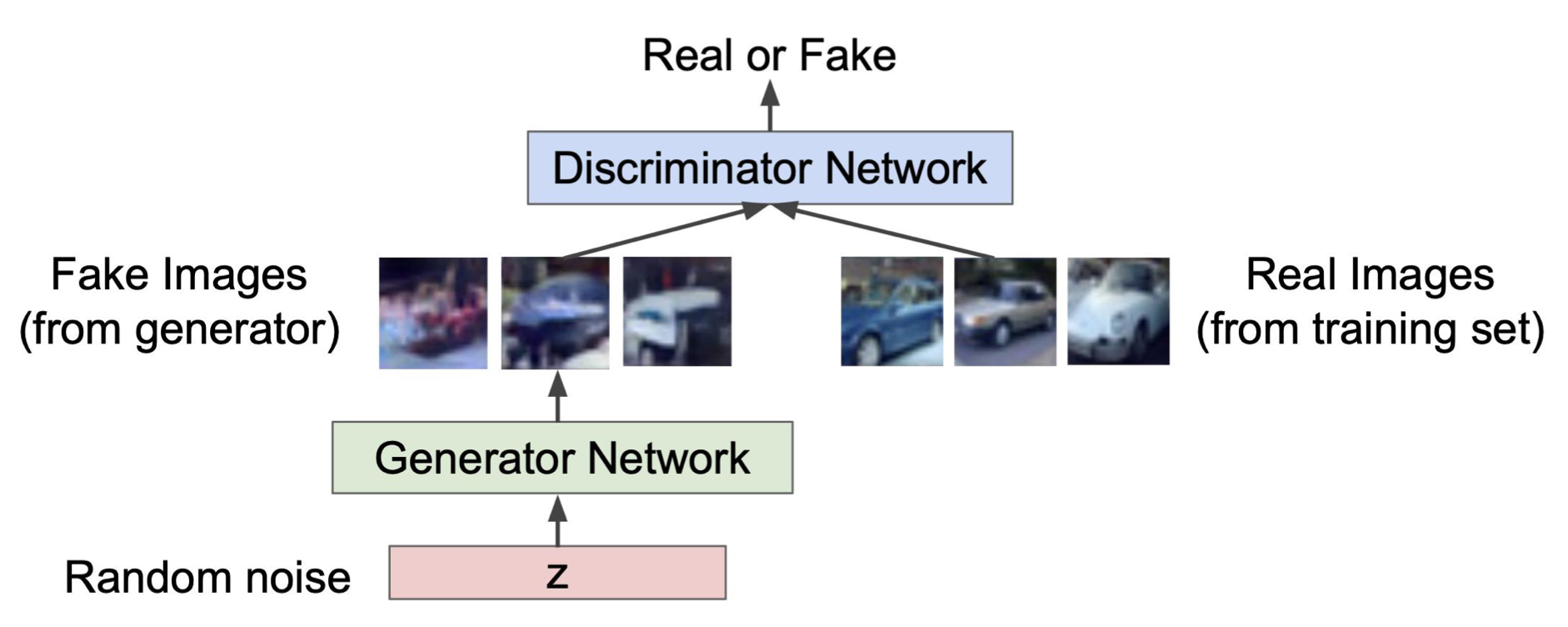
🔗 Binary classification
- Discriminator가 하는 것이 binary classification이다.
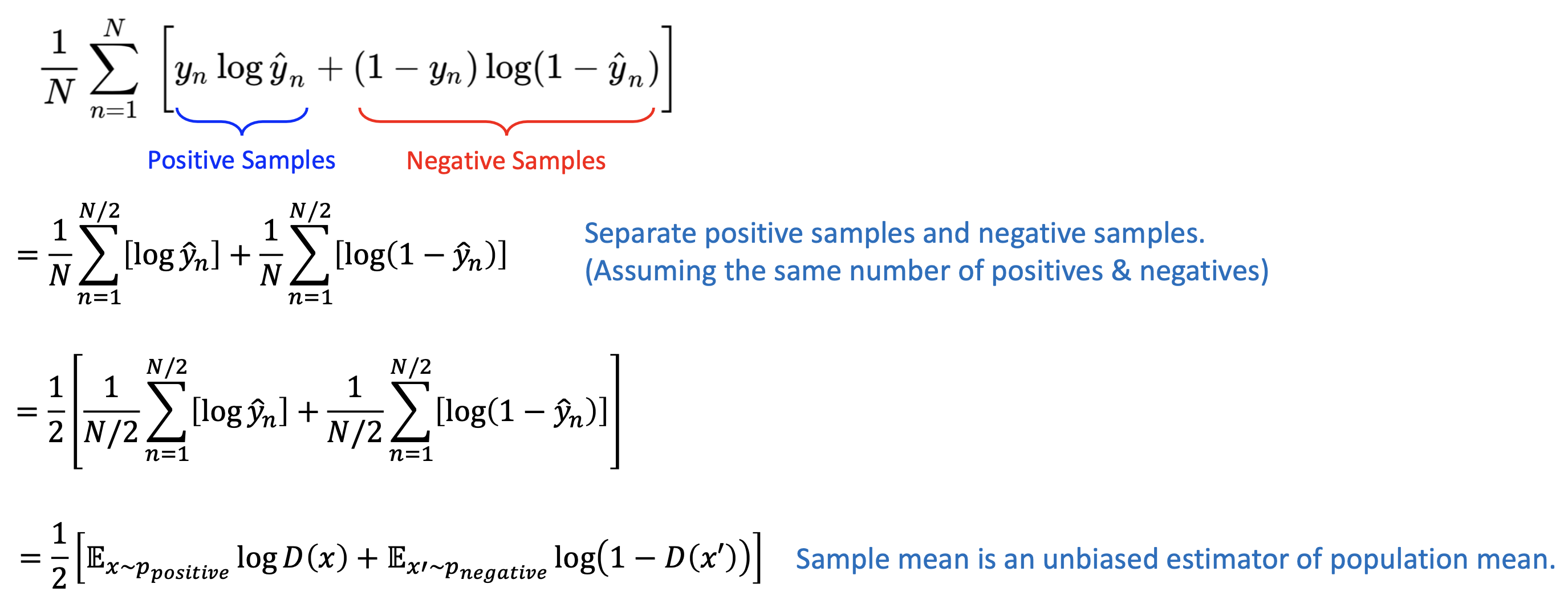
- Data의 절반이 positive sample이고, 절반이 negative sample이라고 가정했다.
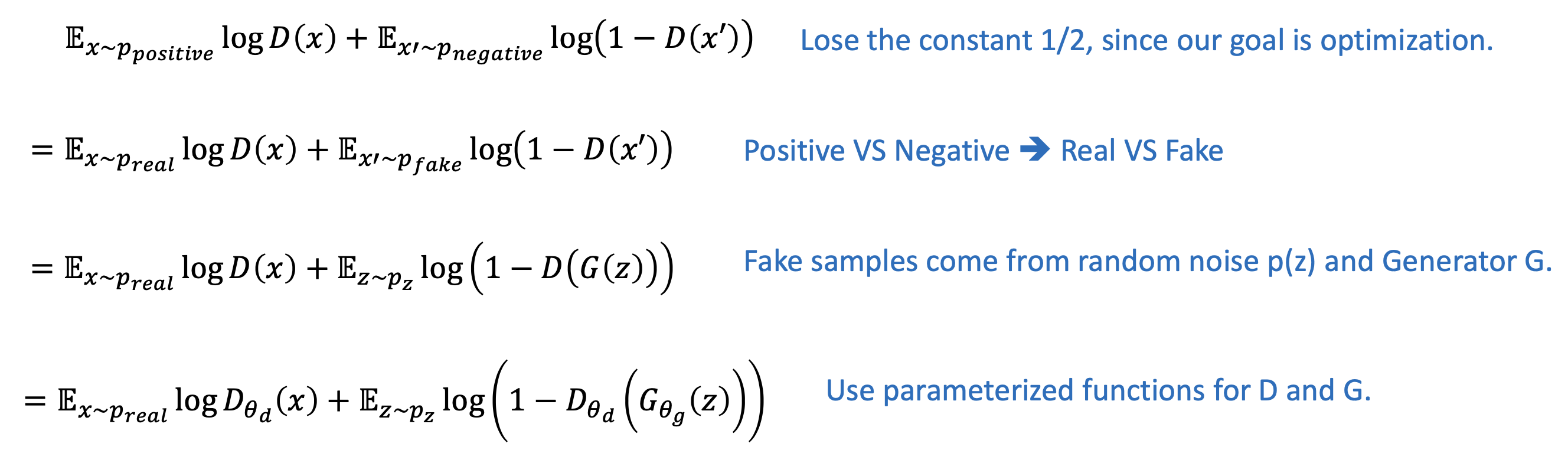
- 마지막 이 식이 GAN의 objective이다.
🔗 Two different goals
두 개의 objective가 있다!
🔗 MinMax Game

-
GAN은 D와 G 사이의 MinMax game이다.
-
이론적으로 D&G 가 Nash Equilibrium에 도달하면, D의 정확도는 50%(random guess)이고, G(z)의 distribution은 p(x)의 distribution과 같다.
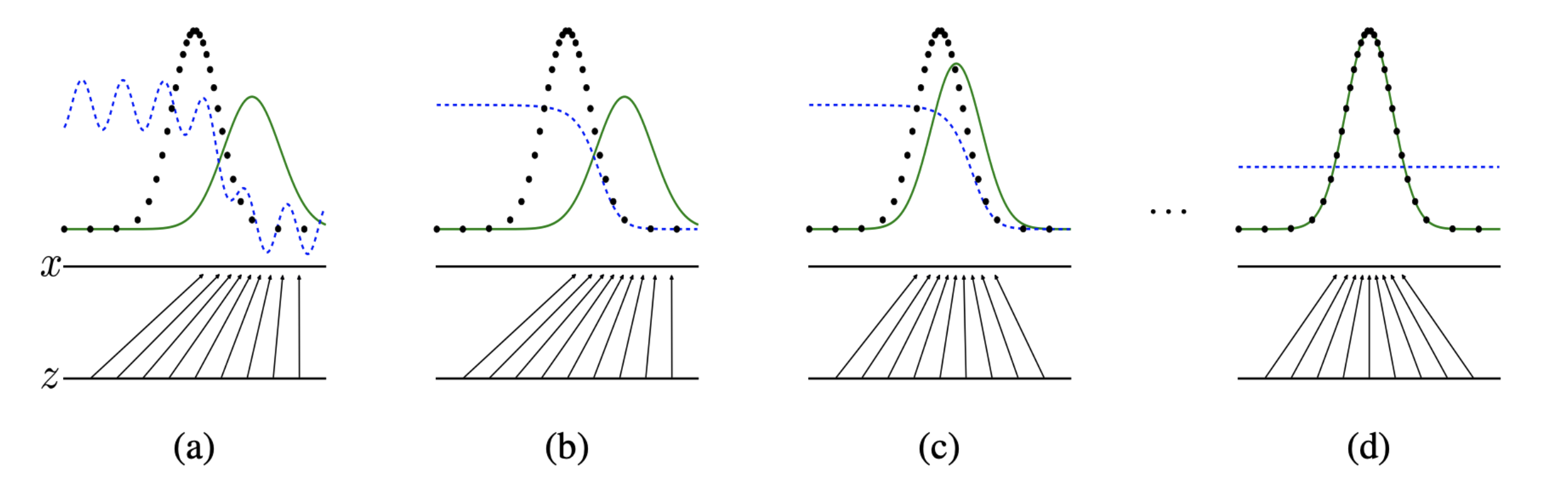
- 이것이 GAN을 train하여 이루고 싶은 것이지만 실제로 일어나진 않는다. 실제로 일어나려면 D와 G가 동시에 훈련되어야하는데 D 훈련하고 G 훈련하고 D하고 G하고 이런식으로 하기 때문이다. 그래서 보장할 수 없다.
🔗 Modified MinMax Game
1. D와 G를 동시에 훈련하지 않고 D하고 G하는 것을 반복한다.
2. MaxMax game을 한다.
-
이유: G가 잘 훈련되지 않는다.
- 초기 단계에서는, D가 G를 쉽게 제압하기 때문에 D(G(z))가 거의 항상 0이다. 그런데 log(1-D(G(z)))를 보면, 초기 단계에서는 안타깝게도 gradient가 거의 0이고 D(G(Z))가 1과 가까울때만 gradient가 유의미하게 생긴다. 이상적으로는 훈련 마지막에 gradient가 0에 가까워야 하는데, 여기서는 상황이 반대로 일어난다.
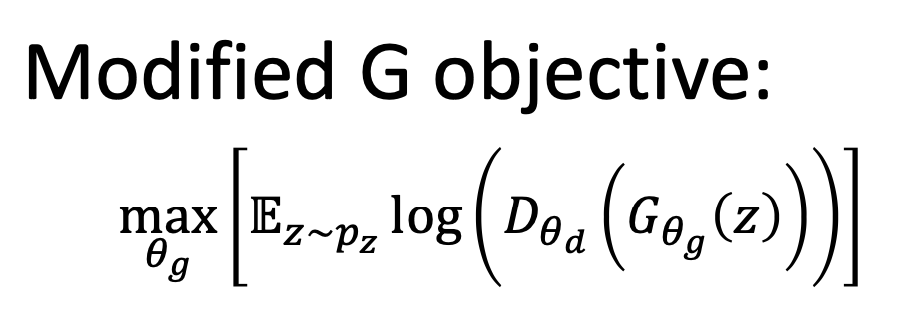
-
Discriminator가 맞출 likelihood를 minimizing하는 대신, 틀릴 likelihood를 maximizing한다.
- 이제 initial phase인 D(G(z))가 0일 때도 gradient signal이 높다.
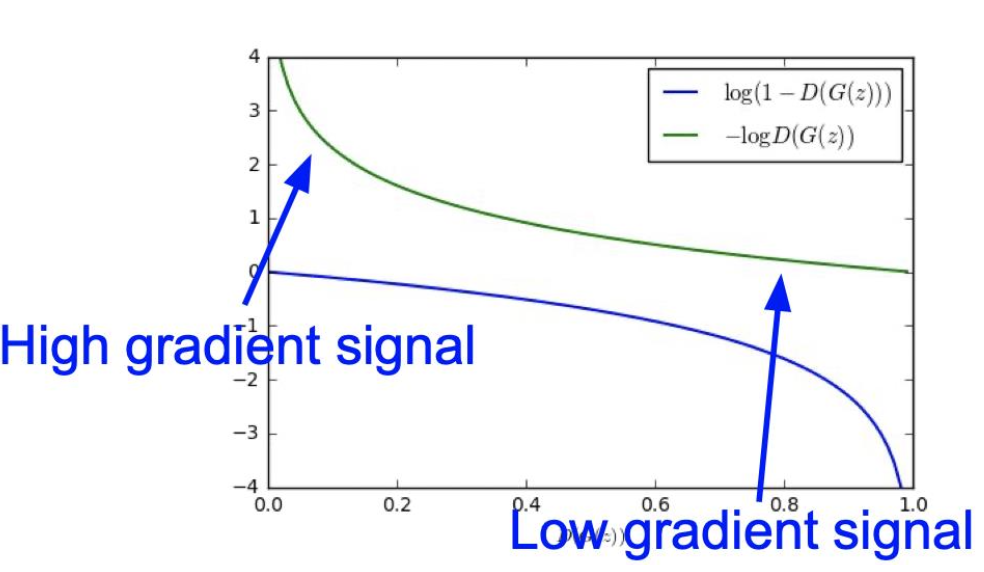
-
이제 Nash Equilibrium은 없다. 더이상 MinMax game이 아니다.
Training GAN
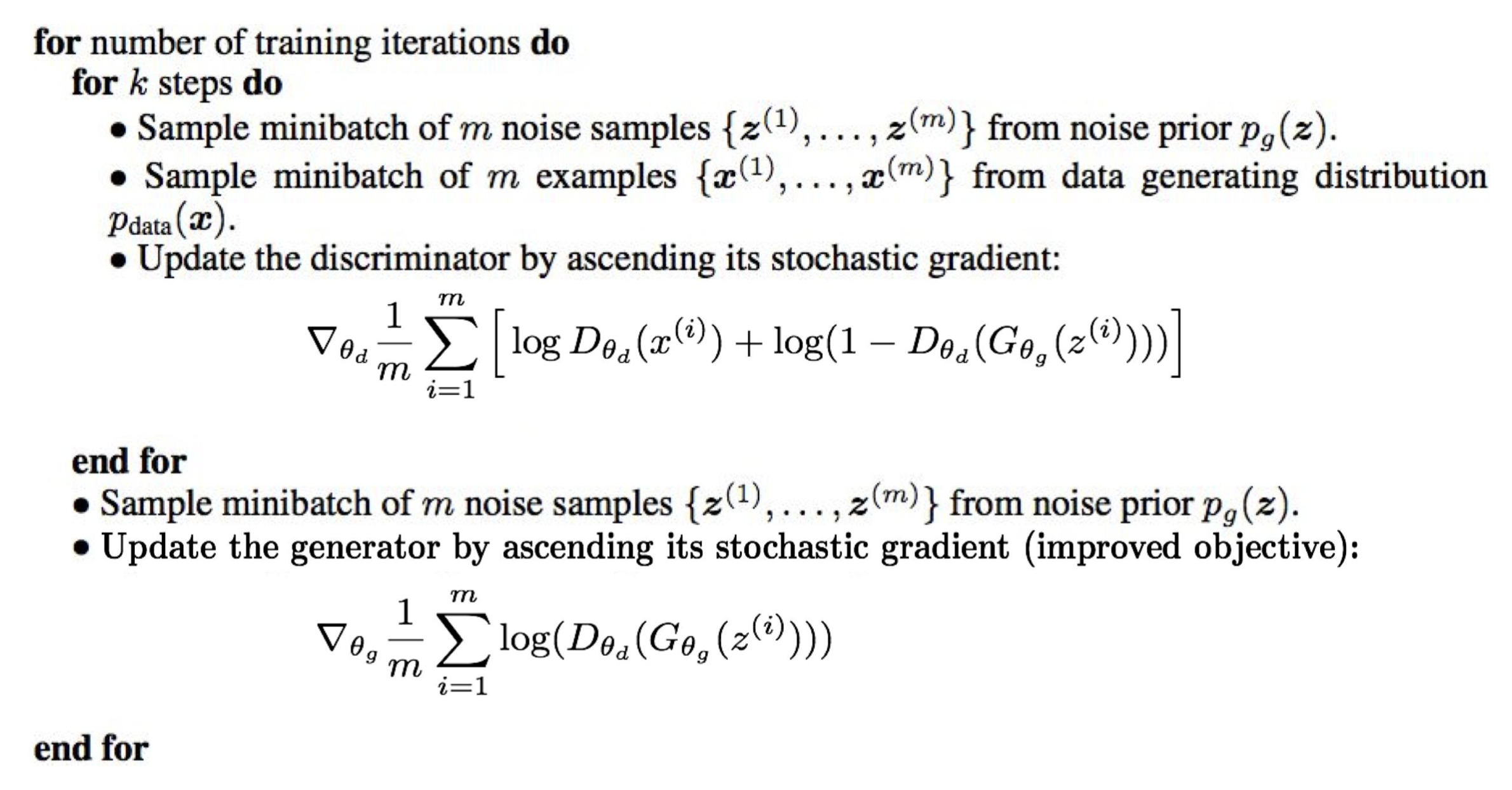
- k step동안 discriminator를 update하고, 1 step동안 generator를 update한다.
- 이유 : Discriminator가 좋은 decision boundary를 갖길 바란다. 그래서 G가 D를 잘 속이길 바란다.
- Discriminator - teacher
- Generator - student
- k는 hyperparameter이다. 가장 좋은 k값은 어떤 문제이냐에 따라 달려있다.
🔗 When to Stop Training
- D와 G가 oscillate하여 G가 언제 특정 기준을 만족하는지 알 수가 없다.
- Typical way
- 중간중간 fake samples를 생성해보고 visual improvement가 없으면 training을 멈춘다.
- Evaluation metric(FID score or Inception score)을 사용한다.
- GAN variants를 사용한다.
- Wasserstein GAN은 더 indicative한 G loss를 제공한다. 그래서 G loss가 얼마나 감소하는지 보고 만족하면 종료하면 된다.
Generating Fake Samples
- Uniform/Gaussian distribution에서 z를 sample하여 generator에 넣어 fake sample x'을 얻는다.
⭐️ Mode Collapse
-
G가 오직 하나의 매우매우 realistic해보이는 같은 fake sample만 generate한다면 D(G(z))는 항상 1이다. 그럼 G가 이긴다. 이것이 G가 Mode Collapse에 빠지는 것이다. 모든 가능한 image G(z) 중에서 오직 하나의 specific한 sample만 선택하기 때문에 모든 z가 random distribution의 특정 영역에서만 집중적으로 나오게 된다.
-
우리는 generator가 가능한 한 다양한 realistic 이미지를 만들기를 원하는데 이렇게 되면 G가 limited diversity를 가지는 sample을 generate하게 된다.
-
이것을 G가 bad local minimum에 빠졌다고 하며, Mode Collapse에 빠진 것이다.
-
Remedy
- Tune hyperparameters
- Use GAN variants (Wasserstein GAN, Unrolled GAN)
VAE vs Autoregressive vs GAN
🔗 VAE
-
Generation
- N(0,1)에서 sample하여 decoder에 투입
-
Measuring performance
- x와 x' 사이의 reconstruction loss를 사용하거나 posterior likelihood Q(z|x)를 사용
-
Image quality
- Vanilla VAE는 blurry한 이미지 생성
- Mean Squared Error loss를 사용하기 때문
- 현대 VAEs는 더 좋아지긴 했는데 요즘 VAE는 많이 사용하지 않음
🔗 PixelCNN
-
Generation
- Initial pixel들이 주어지면, 나머지 pixel들을 생성
- 상대적으로 generation process가 느림
-
Measuring performance
- Sample의 likelihood를 사용할 수 있다. Directly하게 이 form을 이용해서 할 수 있어 easy하다.
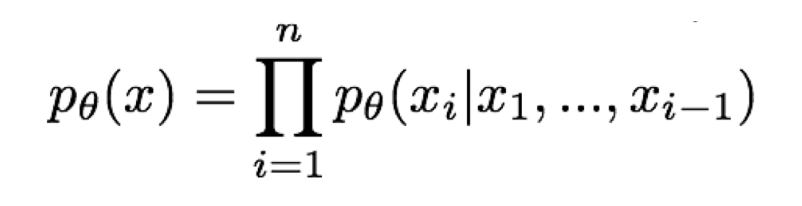
-
Image quality
- Better than vanilla VAE
- Slow inference
🔗 GAN
- Generation
- Uniform 혹은 Gaussian에서 sample해서 Generator에 투입
- Image quality
- Sharpest quality
- StyleGAN은 1024*1024 해상도의 image를 매우 높은 quality로 성공적으로 generate했다.
- Mode collapse가 잠복해 있다.
✔️ Cutting-edge Progress
- Diffusion VS Autoregressive
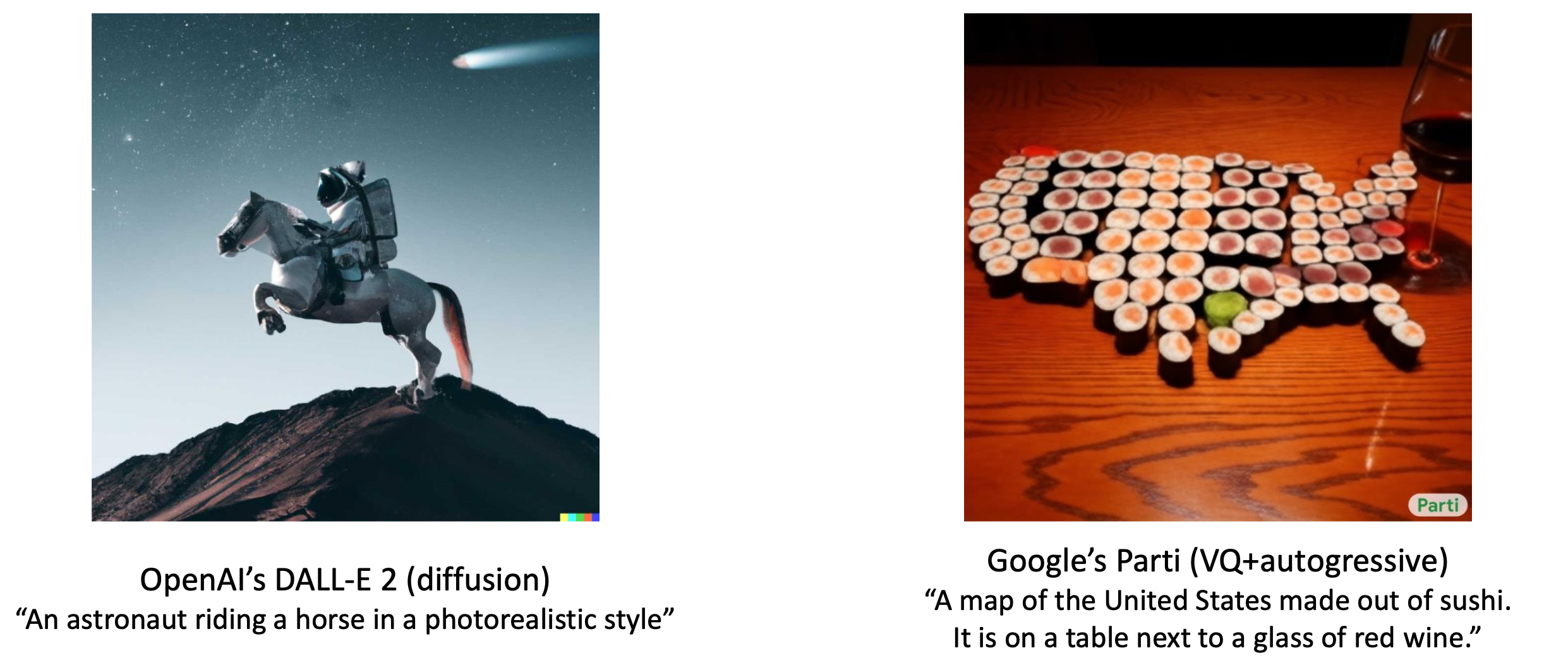
- Autoregressive : discrete code sequence로 변환한다는 것이, text(word sequence)도 discrete code이기 때문에 호환성이 더 좋을 것이다. 그리고 이를 거대한 transformer에 넣으면 더 적합할 것이다.
- Text prompt가 Diffusion model보다 Autoregressive model보다 길다.
- Detail quality는 diffusion model이 더 낫다.
Evaluating GAN
- VAE 혹은 PixelCNN은 explicit distribution을 사용하는 것과 달리 GAN은 implicit distribution을 사용한다.
- Implicit distribution is embedded into the generator. Random z를 realistic sample에 map하기 때문에 generator안에 distribution이 있다.
- Fake sample의 quality를 측정하는 것이 어렵다.
- Two popular metrics
- Inception Score
- Frechet Inception Distance(FID) Score
Inception Score
- Pre-trained model인 Inception v3 model을 사용한다.
- Inception v3 model이 마지막에 1000 dimensional softmax scores를 가지는데, 이것을 이용해 image quality가 어떤지 evaluate한다.
🔗 Two criteria
- Image quality
- Do images look like a specific object (i.e. class)? Is it realistic?
- A single image should have a focused p(y|x)
- Single image를 inception v3 model에 넣어, 1000 dimensional softmax를 얻으면, 이 softmax가 1개의 특정 class에 가능한 한 많이 집중하길 바란다.
- p(y|x) of low entropy ➔ High image quality
- Image diversity
- Is a wide range of objects generated?
- Marginal probability p(y)는 1000 class에 골고루 분포하길 바란다.
- p(y) of high entropy ➔ Diverse images
- Large KL( p(y|x) || p(y) ) ➔ Inception score is high
🔗 Inception Score
- Worst score: 1.0
- Best score: N
- Pre-trained model의 output class의 수와 같다.
Frechet Inception Distance (FID) Score
-
이것도 또한 Inception v3 model을 사용한다.
-
Softmax(output probabilities) 바로 직전의 마지막 pooling layer(2048d vector representation of image)를 사용한다.
-
Real과 fake 사이의 feature distribution을 비교한다.
- 여러 real sample을 넣어 bunch of 2048d vector(real)를 얻고, 여러 fake sample을 넣어 bunch of 2046d vector(fake)를 얻는다.
- 각각(image features)이 gaussian distribution을 형성한다고 가정한다.
- Real Gaussian distribution과 fake Gaussian distribution 사이의 distance를 계산한다.
🔗 FID Score
Applications of GAN
- Text-to-Image GAN
- Cycle GAN
- Style GAN
Reference
- AI504: Programming for AI Lecture at KAIST AI