Natural language processing
Low-level parsing
토큰화, stemming(어간 추출)
의미 단위의 low level task
Word and phrase level (단어, 구)
part-of-speech(POS) tagging
Named entity recognition(NER)
- e.g., New York Times를 3개의 단어로 쪼개지 않고 하나 자체로 인식
Sentence level
Sentiment analysis(감정 분석)
machine translation(기계 번역)
Multi-sentence and paragraph
Question Answering (질의응답)
dialog systems (대화)
summarization (요약)
Entailment prediction
Text mining
social science와 매우 밀접한 관계
Document clustering
정보 검색
추천 시스템으로 발전
Trends of NLP
Word Embedding
RNN 계열 모델
Transformer models
Self-supervised training
- 자가지도학습
- 대규모 text 데이터를 통해 별도의 label이 필요없는 학습
- 문장에서 단어 일부를 가리고 맞히게 하는 형식
Bag-of-Words
1. Unique words를 포함하는 Vocabulary 구축
- Sentences : “John really really loves this movie“, “Jane really likes this song”
- Vocabulary: {“John“, “really“, “loves“, “this“, “movie“, “Jane“, “likes“, “song”}
- 중복 제거
2. Unique words → one-hot vectors
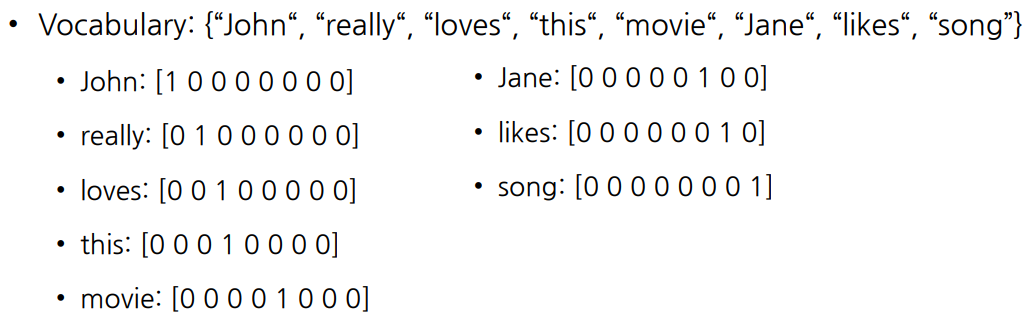
어떤 두 단어를 선택하든, distance는 2
어떤 두 단어를 선택하든, cosine similarity는 0
3. sentence / documents를 one-hot vectors로 표현 가능
- bag-of-words 벡터라고 칭함
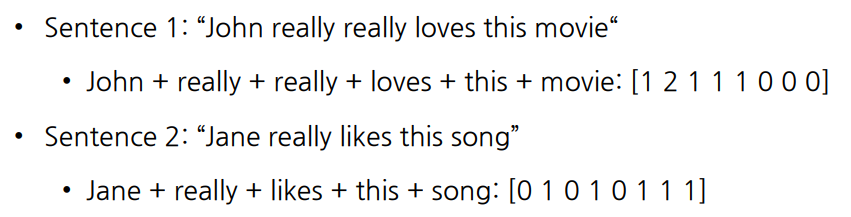
NaiveBayes Classifier
⇒ bag-of-words 벡터로 나타낸 document를 정해진 category 또는 class 중 하나로 분류
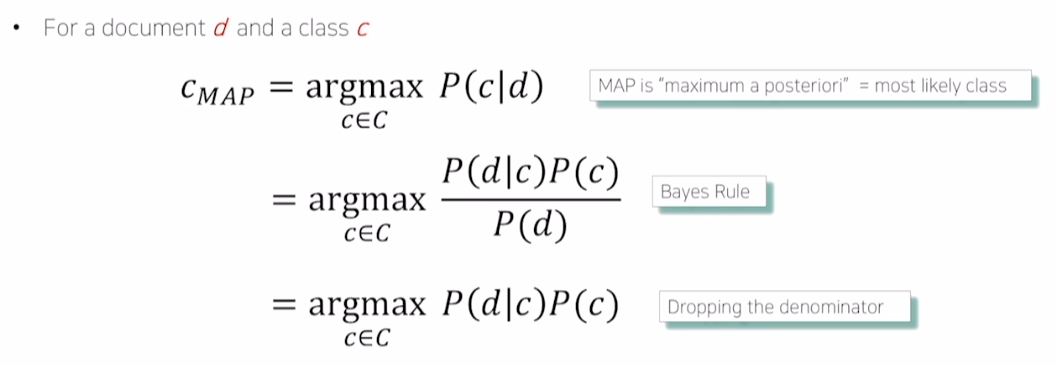
- d는 document, c는 class
- 문서가 주어졌을 때 가장 높은 확률로 분류될 class를 계산
- 베이지안 정리에 의해 아래와 같은 식으로 정리 가능

- w는 document에 속하는 words
- class를 고정시키고 해당 class에서 각 단어가 나타날 확률을 계산
- 각 단어가 독립이라면, 곱으로 나타낼 수 있음
Example
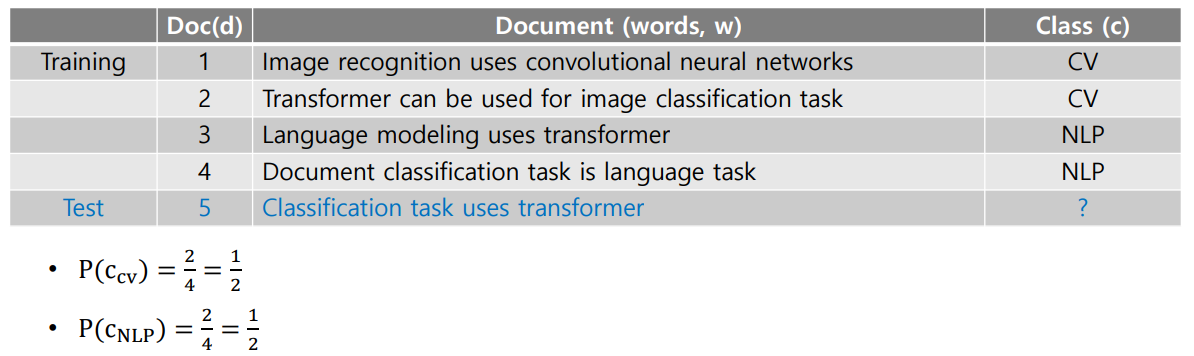
- class는 CV, NLP 2개이며 각 class가 나타날 확률은 1/2
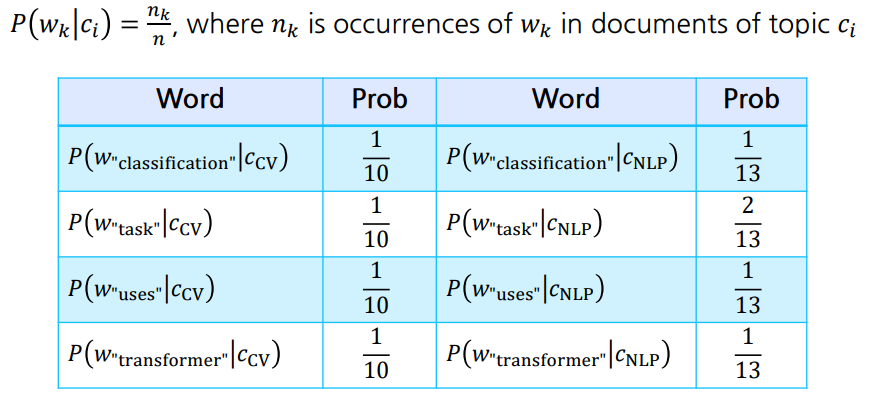
- 왼쪽은 class를 CV로 고정하고, CV 내 모든 document에서 각 단어가 나올 확률을 계산
- e.g., ‘task’는 CV class인 doc1, doc2에서 한 번 등장한다.
- ‘task’는 NLP class인 doc3, doc4에선 두 번 등장한다.
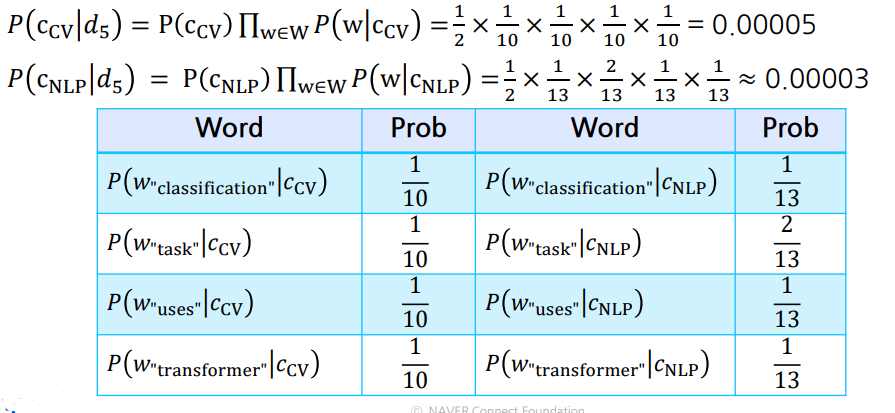
- 확률을 모두 곱해 더 높은 class인 CV로 분류
※ 모든 이미지 및 코드 출처는 네이버 커넥트재단 부스트캠프 AI Tech 5기입니다. ※