💡 NeRF가 novel view synthesis 잘하던데 NeRF랑 GAN으로 3D-Aware generation 해보자
Generation Pipeline : GAN
Representation : NeRF
✍️ Abstract
기본적으로 Generator와 Discriminator가 존재하는 GAN 아키텍처를 사용하는데, generator에서 2D 이미지를 생성하기 위해서 Radiance Fields를 사용한다. 이때 다음과 같은 점에서 NeRF setting과 조금 다르다.
- 그냥 vanila NeRF는 한 scene (Lego, fern etc)을 MLP 에 fitting하는 용도이지만, GRAF는 MLP에서 다양한 scene이 나오길 원하기 때문에 input으로 random성을 줄 noise가 필요하다. 이게 Shape code와 Apperance code이고, 이런 code들에 condition되어 있기 때문에 GRAF에서 사용하는 Radiance fields를 Conditional Radiance Field하고 부른다.
- Patch 도입. training시 전체 이미지를 만들어 내면서 하면 computation이 너무 비싸다. 그래서 이미지의 한 부분인 patch를 생성하고 이를 discriminate함. 어떤 patch를 쓸지는 random 으로 결정함. inference할 땐 whole image가 patch라고 생각하고 생성하면 됨.
📌 Main Method
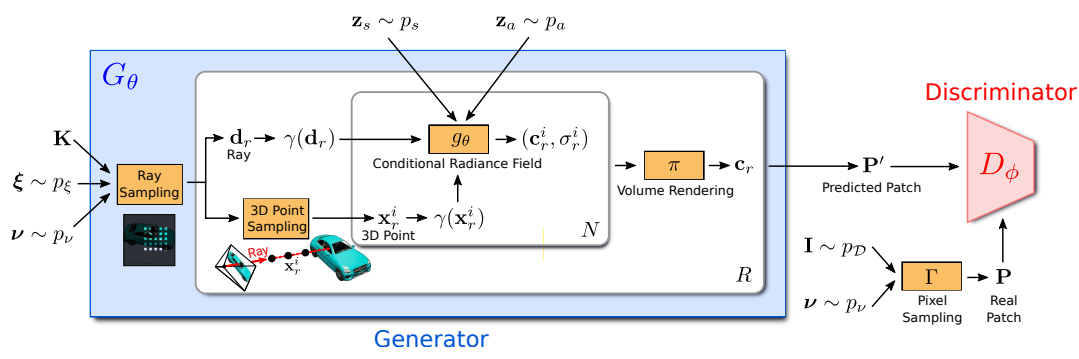
Generator
The Generator Gθ takes
- Camera matrix K
- 카메라의 intrinsic parameter들을 나타내는 matrix이며 카메라가 어떻게 이미지를 capture할꺼냐에 관한 parameter이다 (focal length etc)
- Camera Pose ξ ∼pξ(uniform distribution)
- Extrinsic parameter에 해당하는 값들 인데 그냥 upper hemisphere에 카메라가 오도록 해놓고 uniform distribution에서 sample!
- 2D sampling pattern ν ∼pν
- Image를 하나 다 생성하는 것보다 더 효율적으로 학습하기 위해서 Patch라는 걸 도입함! 이 Patch의 크기, patch의 문양?등을 결정하는 parameter라고 보면됨.
- Shape codes zs∈Rm
- Shape을 결정할 noise. From 가우시안
- Appearance codes za∈Rn
- Appearance를 결정할 noise. From 가우시안
as input, and outputs an image patch P′ which is discriminated by Dϕ
Generator에서 2D patch(image)를 생성해내는 과정은 다음과 같다.
1. Ray sampling
카메라 matrix, 카메라 pose, sampling pattern을 사용하여 R개의 RAY를 sampling한다
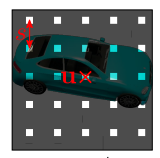
2. Point Sampling
R개의 ray에서 각각 N개의 point를 sampling한다!
3. Conditional Radiance Field gθ
각각의 point당 gθ에 query하여 density와 RGB값을 구함. 이때 apperance code와 shape code도 오른쪽 그림과 같이 input 됨. 결국 다음과 같은 함수임! 그저 3D좌표와 direction, code를 받으면 RGB랑 density를 output함.
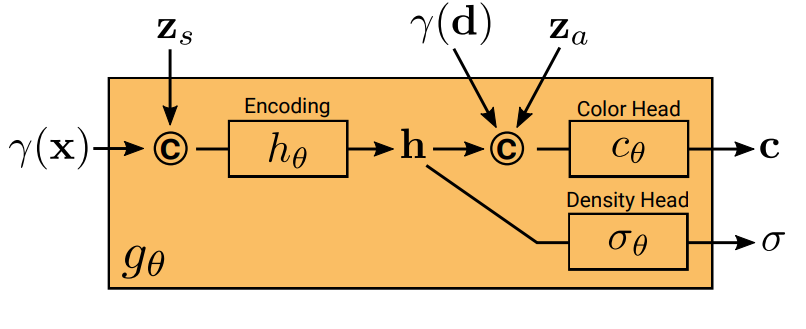
(γ(x),γ(d),zs,za)→(c,σ)
4. Volume Rendering
너프랑 똑같음. 너프를 완벽하게 이해하자!
Discriminator Dϕ
The discriminator Dθ compares the predicted patch P′ and the real patch P from real Image I drawn from data distribution pD
그냥 2D convolution discriminator. 단지 training할 땐 전체 이미지가 아닌 Patch를 구별!
💪Training, Inference
Training할 때랑 inference할 때를 한번 쭉 다시 살펴보자. Dataset은 cars나 Celeb 같은 그냥 2D 이미지들이다!
Training
- Camera matrix, pose, patch pattern을 하나씩 랜덤으로 뽑음.
- 1의 parameter들로 R개의 ray를 sampling
- 각각의 RAY당 N개의 point를 sampling
- 각각의 Point좌표랑 가우시안에서 뽑아온 zs∈Rm, za∈Rn 를 사용하여 해당 점의 밀도와 RGB값 구함
- 각 RAY에 대하여 모든 point들의 밀도와 RGB를 이용하여 한 pixel로 volume rendering.
- 모든 RAY에 대하여 하면 patch(image)임!
- patch를 discriminator에 넣어서 real인지 아닌지 판별!
Inference
- 특정 Camera matrix, pose를 잡고 patch pattern는 이미지 전체로 고정!
- Generator로 이미지 생성!
- 2번에서 사용한 zs∈Rm, za∈Rn 를 바꾸면 다양한 이미지 생성가능!
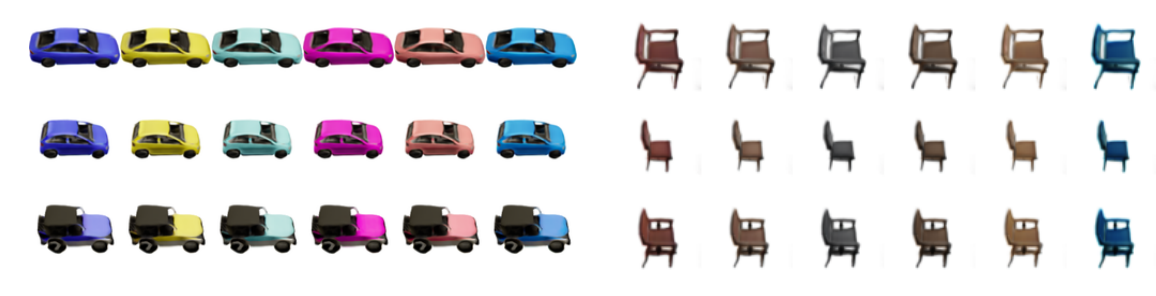
- 1번에서 사용한 Camera matrix, pose를 바꾸고 zs∈Rm, za∈Rn 를 고정하면 한 scene에 대한 여러 방면의 이미지를 볼수 있음!
