새싹 인공지능 응용sw 개발자 양성 교육 프로그램 심선조 강사님 수업 정리 글입니다.
1. 깔끔한 데이터
-
넓은 데이터
데이터프레임의 열은 파이썬의 변수와 비슷한 역할을 한다.
데이터프레임의 열이 옆으로 길게 늘어선 형태가 넓은 데이터라고 한다.
-
melt메서드
지정한 열의 데이터를 모두 행으로 정리해 준다.
-
melt메서드 인자
id_vars : 위치를 그대로 유지할 열의 이름을 지정
value_vars : 행을 위치를 변경할 열의 이름을 지정
var_name: value_vars로 위치를 변경한 열의 이름을 지정
value_name: var_name으로 위치를 변경한 열의 데이터를 지정한 열의 이름을 지정
-
pd.melt(
frame: 'DataFrame',
id_vars=None,
value_vars=None,
var_name=None,
value_name='value',
col_level=None,
ignore_index: 'bool' = True, #기존의 index을 무시하고 새로 부여하겠다.
) -> 'DataFrame'
import pandas as pd
df = pd.read_csv('data/pew.csv')
df.head(2)
|
religion |
<$10k |
$10-20k |
$20-30k |
$30-40k |
$40-50k |
$50-75k |
$75-100k |
$100-150k |
>150k |
Don't know/refused |
0 |
Agnostic |
27 |
34 |
60 |
81 |
76 |
137 |
122 |
109 |
84 |
96 |
1 |
Atheist |
12 |
27 |
37 |
52 |
35 |
70 |
73 |
59 |
74 |
76 |
data=pd.melt(df,id_vars='religion')
data
|
religion |
variable |
value |
0 |
Agnostic |
<$10k |
27 |
1 |
Atheist |
<$10k |
12 |
2 |
Buddhist |
<$10k |
27 |
3 |
Catholic |
<$10k |
418 |
4 |
Don’t know/refused |
<$10k |
15 |
... |
... |
... |
... |
175 |
Orthodox |
Don't know/refused |
73 |
176 |
Other Christian |
Don't know/refused |
18 |
177 |
Other Faiths |
Don't know/refused |
71 |
178 |
Other World Religions |
Don't know/refused |
8 |
179 |
Unaffiliated |
Don't know/refused |
597 |
180 rows × 3 columns
data.columns = ['religion', 'income', 'count']
data.sample()
|
religion |
income |
count |
61 |
Historically Black Prot |
$30-40k |
238 |
data=pd.melt(df,id_vars='religion',var_name='income',value_name='count')
data.sample()
|
religion |
income |
count |
69 |
Other Faiths |
$30-40k |
46 |
data.rename(columns={"income":"소득영역","count":"갯수"})
|
religion |
소득영역 |
갯수 |
0 |
Agnostic |
<$10k |
27 |
1 |
Atheist |
<$10k |
12 |
2 |
Buddhist |
<$10k |
27 |
3 |
Catholic |
<$10k |
418 |
4 |
Don’t know/refused |
<$10k |
15 |
... |
... |
... |
... |
175 |
Orthodox |
Don't know/refused |
73 |
176 |
Other Christian |
Don't know/refused |
18 |
177 |
Other Faiths |
Don't know/refused |
71 |
178 |
Other World Religions |
Don't know/refused |
8 |
179 |
Unaffiliated |
Don't know/refused |
597 |
180 rows × 3 columns
df=pd.read_csv('data/billboard.csv')
df.head(1)
|
year |
artist |
track |
time |
date.entered |
wk1 |
wk2 |
wk3 |
wk4 |
wk5 |
... |
wk67 |
wk68 |
wk69 |
wk70 |
wk71 |
wk72 |
wk73 |
wk74 |
wk75 |
wk76 |
0 |
2000 |
2 Pac |
Baby Don't Cry (Keep... |
4:22 |
2000-02-26 |
87 |
82.0 |
72.0 |
77.0 |
87.0 |
... |
NaN |
NaN |
NaN |
NaN |
NaN |
NaN |
NaN |
NaN |
NaN |
NaN |
1 rows × 81 columns
df.columns
Index(['year', 'artist', 'track', 'time', 'date.entered', 'wk1', 'wk2', 'wk3',
'wk4', 'wk5', 'wk6', 'wk7', 'wk8', 'wk9', 'wk10', 'wk11', 'wk12',
'wk13', 'wk14', 'wk15', 'wk16', 'wk17', 'wk18', 'wk19', 'wk20', 'wk21',
'wk22', 'wk23', 'wk24', 'wk25', 'wk26', 'wk27', 'wk28', 'wk29', 'wk30',
'wk31', 'wk32', 'wk33', 'wk34', 'wk35', 'wk36', 'wk37', 'wk38', 'wk39',
'wk40', 'wk41', 'wk42', 'wk43', 'wk44', 'wk45', 'wk46', 'wk47', 'wk48',
'wk49', 'wk50', 'wk51', 'wk52', 'wk53', 'wk54', 'wk55', 'wk56', 'wk57',
'wk58', 'wk59', 'wk60', 'wk61', 'wk62', 'wk63', 'wk64', 'wk65', 'wk66',
'wk67', 'wk68', 'wk69', 'wk70', 'wk71', 'wk72', 'wk73', 'wk74', 'wk75',
'wk76'],
dtype='object')
df_melt = pd.melt(df,
id_vars=['year', 'artist', 'track', 'time', 'date.entered'],
var_name='week',
value_name='rating')
df.shape
(317, 81)
df_melt.shape
(24092, 7)
하나의 열이 여러 의미를 가지고 있는 경우
예를 들어 ebola데이터 집합에 Deaths_Guinea는 '사망자 수'와 '나라 이름'을 합쳐 만든 이름이다.
df=pd.read_csv('data/country_timeseries.csv')
df.sample()
|
Date |
Day |
Cases_Guinea |
Cases_Liberia |
Cases_SierraLeone |
Cases_Nigeria |
Cases_Senegal |
Cases_UnitedStates |
Cases_Spain |
Cases_Mali |
Deaths_Guinea |
Deaths_Liberia |
Deaths_SierraLeone |
Deaths_Nigeria |
Deaths_Senegal |
Deaths_UnitedStates |
Deaths_Spain |
Deaths_Mali |
19 |
11/18/2014 |
241 |
2047.0 |
7082.0 |
6190.0 |
20.0 |
1.0 |
4.0 |
1.0 |
6.0 |
1214.0 |
2963.0 |
1267.0 |
8.0 |
0.0 |
1.0 |
0.0 |
6.0 |
df_melt=pd.melt(df,id_vars=['Date','Day'])
df_melt.variable
0 Cases_Guinea
1 Cases_Guinea
2 Cases_Guinea
3 Cases_Guinea
4 Cases_Guinea
...
1947 Deaths_Mali
1948 Deaths_Mali
1949 Deaths_Mali
1950 Deaths_Mali
1951 Deaths_Mali
Name: variable, Length: 1952, dtype: object
df_melt['variable']
0 Cases_Guinea
1 Cases_Guinea
2 Cases_Guinea
3 Cases_Guinea
4 Cases_Guinea
...
1947 Deaths_Mali
1948 Deaths_Mali
1949 Deaths_Mali
1950 Deaths_Mali
1951 Deaths_Mali
Name: variable, Length: 1952, dtype: object
df_melt.variable.str.split('_')
0 [Cases, Guinea]
1 [Cases, Guinea]
2 [Cases, Guinea]
3 [Cases, Guinea]
4 [Cases, Guinea]
...
1947 [Deaths, Mali]
1948 [Deaths, Mali]
1949 [Deaths, Mali]
1950 [Deaths, Mali]
1951 [Deaths, Mali]
Name: variable, Length: 1952, dtype: object
df_melt['status'] = df_melt.variable.str.split('_').str.get(0)
df_melt['country'] = df_melt.variable.str.split('_').str.get(1)
df_melt.sample(3)
|
Date |
Day |
variable |
value |
status |
country |
360 |
3/28/2014 |
6 |
Cases_SierraLeone |
2.0 |
Cases |
SierraLeone |
190 |
8/1/2014 |
132 |
Cases_Liberia |
468.0 |
Cases |
Liberia |
804 |
7/20/2014 |
120 |
Cases_Spain |
NaN |
Cases |
Spain |
여러 열을 하나로 정리하기
df = pd.read_csv('data/weather.csv')
df.head(1)
|
id |
year |
month |
element |
d1 |
d2 |
d3 |
d4 |
d5 |
d6 |
... |
d22 |
d23 |
d24 |
d25 |
d26 |
d27 |
d28 |
d29 |
d30 |
d31 |
0 |
MX17004 |
2010 |
1 |
tmax |
NaN |
NaN |
NaN |
NaN |
NaN |
NaN |
... |
NaN |
NaN |
NaN |
NaN |
NaN |
NaN |
NaN |
NaN |
27.8 |
NaN |
1 rows × 35 columns
df_melt = pd.melt(df,
id_vars=['id','year','month','element'],
var_name='day',
value_name='temp')
df_pivot = df_melt.pivot_table(index=['id','year','month','day'],
columns='element',
values='temp')
df_pivot.index
MultiIndex([('MX17004', 2010, 1, 'd30'),
('MX17004', 2010, 2, 'd11'),
('MX17004', 2010, 2, 'd2'),
('MX17004', 2010, 2, 'd23'),
('MX17004', 2010, 2, 'd3'),
('MX17004', 2010, 3, 'd10'),
('MX17004', 2010, 3, 'd16'),
('MX17004', 2010, 3, 'd5'),
('MX17004', 2010, 4, 'd27'),
('MX17004', 2010, 5, 'd27'),
('MX17004', 2010, 6, 'd17'),
('MX17004', 2010, 6, 'd29'),
('MX17004', 2010, 7, 'd3'),
('MX17004', 2010, 7, 'd14'),
('MX17004', 2010, 8, 'd23'),
('MX17004', 2010, 8, 'd5'),
('MX17004', 2010, 8, 'd29'),
('MX17004', 2010, 8, 'd13'),
('MX17004', 2010, 8, 'd25'),
('MX17004', 2010, 8, 'd31'),
('MX17004', 2010, 8, 'd8'),
('MX17004', 2010, 10, 'd5'),
('MX17004', 2010, 10, 'd14'),
('MX17004', 2010, 10, 'd15'),
('MX17004', 2010, 10, 'd28'),
('MX17004', 2010, 10, 'd7'),
('MX17004', 2010, 11, 'd2'),
('MX17004', 2010, 11, 'd5'),
('MX17004', 2010, 11, 'd27'),
('MX17004', 2010, 11, 'd26'),
('MX17004', 2010, 11, 'd4'),
('MX17004', 2010, 12, 'd1'),
('MX17004', 2010, 12, 'd6')],
names=['id', 'year', 'month', 'day'])
df_pivot.columns
Index(['tmax', 'tmin'], dtype='object', name='element')
df_pivot.values
array([[27.8, 14.5],
[29.7, 13.4],
[27.3, 14.4],
[29.9, 10.7],
[24.1, 14.4],
[34.5, 16.8],
[31.1, 17.6],
[32.1, 14.2],
[36.3, 16.7],
[33.2, 18.2],
[28. , 17.5],
[30.1, 18. ],
[28.6, 17.5],
[29.9, 16.5],
[26.4, 15. ],
[29.6, 15.8],
[28. , 15.3],
[29.8, 16.5],
[29.7, 15.6],
[25.4, 15.4],
[29. , 17.3],
[27. , 14. ],
[29.5, 13. ],
[28.7, 10.5],
[31.2, 15. ],
[28.1, 12.9],
[31.3, 16.3],
[26.3, 7.9],
[27.7, 14.2],
[28.1, 12.1],
[27.2, 12. ],
[29.9, 13.8],
[27.8, 10.5]])
df_pivot.reset_index(inplace=True)
df_pivot.index
RangeIndex(start=0, stop=33, step=1)
df_pivot.values
array([[0, 0, 'MX17004', 2010, 1, 'd30', 27.8, 14.5],
[1, 1, 'MX17004', 2010, 2, 'd11', 29.7, 13.4],
[2, 2, 'MX17004', 2010, 2, 'd2', 27.3, 14.4],
[3, 3, 'MX17004', 2010, 2, 'd23', 29.9, 10.7],
[4, 4, 'MX17004', 2010, 2, 'd3', 24.1, 14.4],
[5, 5, 'MX17004', 2010, 3, 'd10', 34.5, 16.8],
[6, 6, 'MX17004', 2010, 3, 'd16', 31.1, 17.6],
[7, 7, 'MX17004', 2010, 3, 'd5', 32.1, 14.2],
[8, 8, 'MX17004', 2010, 4, 'd27', 36.3, 16.7],
[9, 9, 'MX17004', 2010, 5, 'd27', 33.2, 18.2],
[10, 10, 'MX17004', 2010, 6, 'd17', 28.0, 17.5],
[11, 11, 'MX17004', 2010, 6, 'd29', 30.1, 18.0],
[12, 12, 'MX17004', 2010, 7, 'd3', 28.6, 17.5],
[13, 13, 'MX17004', 2010, 7, 'd14', 29.9, 16.5],
[14, 14, 'MX17004', 2010, 8, 'd23', 26.4, 15.0],
[15, 15, 'MX17004', 2010, 8, 'd5', 29.6, 15.8],
[16, 16, 'MX17004', 2010, 8, 'd29', 28.0, 15.3],
[17, 17, 'MX17004', 2010, 8, 'd13', 29.8, 16.5],
[18, 18, 'MX17004', 2010, 8, 'd25', 29.7, 15.6],
[19, 19, 'MX17004', 2010, 8, 'd31', 25.4, 15.4],
[20, 20, 'MX17004', 2010, 8, 'd8', 29.0, 17.3],
[21, 21, 'MX17004', 2010, 10, 'd5', 27.0, 14.0],
[22, 22, 'MX17004', 2010, 10, 'd14', 29.5, 13.0],
[23, 23, 'MX17004', 2010, 10, 'd15', 28.7, 10.5],
[24, 24, 'MX17004', 2010, 10, 'd28', 31.2, 15.0],
[25, 25, 'MX17004', 2010, 10, 'd7', 28.1, 12.9],
[26, 26, 'MX17004', 2010, 11, 'd2', 31.3, 16.3],
[27, 27, 'MX17004', 2010, 11, 'd5', 26.3, 7.9],
[28, 28, 'MX17004', 2010, 11, 'd27', 27.7, 14.2],
[29, 29, 'MX17004', 2010, 11, 'd26', 28.1, 12.1],
[30, 30, 'MX17004', 2010, 11, 'd4', 27.2, 12.0],
[31, 31, 'MX17004', 2010, 12, 'd1', 29.9, 13.8],
[32, 32, 'MX17004', 2010, 12, 'd6', 27.8, 10.5]], dtype=object)
df = pd.read_csv('data/billboard.csv')
df
|
year |
artist |
track |
time |
date.entered |
wk1 |
wk2 |
wk3 |
wk4 |
wk5 |
... |
wk67 |
wk68 |
wk69 |
wk70 |
wk71 |
wk72 |
wk73 |
wk74 |
wk75 |
wk76 |
0 |
2000 |
2 Pac |
Baby Don't Cry (Keep... |
4:22 |
2000-02-26 |
87 |
82.0 |
72.0 |
77.0 |
87.0 |
... |
NaN |
NaN |
NaN |
NaN |
NaN |
NaN |
NaN |
NaN |
NaN |
NaN |
1 |
2000 |
2Ge+her |
The Hardest Part Of ... |
3:15 |
2000-09-02 |
91 |
87.0 |
92.0 |
NaN |
NaN |
... |
NaN |
NaN |
NaN |
NaN |
NaN |
NaN |
NaN |
NaN |
NaN |
NaN |
2 |
2000 |
3 Doors Down |
Kryptonite |
3:53 |
2000-04-08 |
81 |
70.0 |
68.0 |
67.0 |
66.0 |
... |
NaN |
NaN |
NaN |
NaN |
NaN |
NaN |
NaN |
NaN |
NaN |
NaN |
3 |
2000 |
3 Doors Down |
Loser |
4:24 |
2000-10-21 |
76 |
76.0 |
72.0 |
69.0 |
67.0 |
... |
NaN |
NaN |
NaN |
NaN |
NaN |
NaN |
NaN |
NaN |
NaN |
NaN |
4 |
2000 |
504 Boyz |
Wobble Wobble |
3:35 |
2000-04-15 |
57 |
34.0 |
25.0 |
17.0 |
17.0 |
... |
NaN |
NaN |
NaN |
NaN |
NaN |
NaN |
NaN |
NaN |
NaN |
NaN |
... |
... |
... |
... |
... |
... |
... |
... |
... |
... |
... |
... |
... |
... |
... |
... |
... |
... |
... |
... |
... |
... |
312 |
2000 |
Yankee Grey |
Another Nine Minutes |
3:10 |
2000-04-29 |
86 |
83.0 |
77.0 |
74.0 |
83.0 |
... |
NaN |
NaN |
NaN |
NaN |
NaN |
NaN |
NaN |
NaN |
NaN |
NaN |
313 |
2000 |
Yearwood, Trisha |
Real Live Woman |
3:55 |
2000-04-01 |
85 |
83.0 |
83.0 |
82.0 |
81.0 |
... |
NaN |
NaN |
NaN |
NaN |
NaN |
NaN |
NaN |
NaN |
NaN |
NaN |
314 |
2000 |
Ying Yang Twins |
Whistle While You Tw... |
4:19 |
2000-03-18 |
95 |
94.0 |
91.0 |
85.0 |
84.0 |
... |
NaN |
NaN |
NaN |
NaN |
NaN |
NaN |
NaN |
NaN |
NaN |
NaN |
315 |
2000 |
Zombie Nation |
Kernkraft 400 |
3:30 |
2000-09-02 |
99 |
99.0 |
NaN |
NaN |
NaN |
... |
NaN |
NaN |
NaN |
NaN |
NaN |
NaN |
NaN |
NaN |
NaN |
NaN |
316 |
2000 |
matchbox twenty |
Bent |
4:12 |
2000-04-29 |
60 |
37.0 |
29.0 |
24.0 |
22.0 |
... |
NaN |
NaN |
NaN |
NaN |
NaN |
NaN |
NaN |
NaN |
NaN |
NaN |
317 rows × 81 columns
df_melt = pd.melt(df,
id_vars=['year','artist','track','time','date.entered'],
var_name='week',
value_name='rating')
df_melt
|
year |
artist |
track |
time |
date.entered |
week |
rating |
0 |
2000 |
2 Pac |
Baby Don't Cry (Keep... |
4:22 |
2000-02-26 |
wk1 |
87.0 |
1 |
2000 |
2Ge+her |
The Hardest Part Of ... |
3:15 |
2000-09-02 |
wk1 |
91.0 |
2 |
2000 |
3 Doors Down |
Kryptonite |
3:53 |
2000-04-08 |
wk1 |
81.0 |
3 |
2000 |
3 Doors Down |
Loser |
4:24 |
2000-10-21 |
wk1 |
76.0 |
4 |
2000 |
504 Boyz |
Wobble Wobble |
3:35 |
2000-04-15 |
wk1 |
57.0 |
... |
... |
... |
... |
... |
... |
... |
... |
24087 |
2000 |
Yankee Grey |
Another Nine Minutes |
3:10 |
2000-04-29 |
wk76 |
NaN |
24088 |
2000 |
Yearwood, Trisha |
Real Live Woman |
3:55 |
2000-04-01 |
wk76 |
NaN |
24089 |
2000 |
Ying Yang Twins |
Whistle While You Tw... |
4:19 |
2000-03-18 |
wk76 |
NaN |
24090 |
2000 |
Zombie Nation |
Kernkraft 400 |
3:30 |
2000-09-02 |
wk76 |
NaN |
24091 |
2000 |
matchbox twenty |
Bent |
4:12 |
2000-04-29 |
wk76 |
NaN |
24092 rows × 7 columns
df.shape, df_melt.shape
((317, 81), (24092, 7))
df_melt[df_melt.track == 'Loser']
|
year |
artist |
track |
time |
date.entered |
week |
rating |
3 |
2000 |
3 Doors Down |
Loser |
4:24 |
2000-10-21 |
wk1 |
76.0 |
320 |
2000 |
3 Doors Down |
Loser |
4:24 |
2000-10-21 |
wk2 |
76.0 |
637 |
2000 |
3 Doors Down |
Loser |
4:24 |
2000-10-21 |
wk3 |
72.0 |
954 |
2000 |
3 Doors Down |
Loser |
4:24 |
2000-10-21 |
wk4 |
69.0 |
1271 |
2000 |
3 Doors Down |
Loser |
4:24 |
2000-10-21 |
wk5 |
67.0 |
... |
... |
... |
... |
... |
... |
... |
... |
22510 |
2000 |
3 Doors Down |
Loser |
4:24 |
2000-10-21 |
wk72 |
NaN |
22827 |
2000 |
3 Doors Down |
Loser |
4:24 |
2000-10-21 |
wk73 |
NaN |
23144 |
2000 |
3 Doors Down |
Loser |
4:24 |
2000-10-21 |
wk74 |
NaN |
23461 |
2000 |
3 Doors Down |
Loser |
4:24 |
2000-10-21 |
wk75 |
NaN |
23778 |
2000 |
3 Doors Down |
Loser |
4:24 |
2000-10-21 |
wk76 |
NaN |
76 rows × 7 columns
df_songs = df_melt[['year','artist','track','time']]
df_songs
|
year |
artist |
track |
time |
0 |
2000 |
2 Pac |
Baby Don't Cry (Keep... |
4:22 |
1 |
2000 |
2Ge+her |
The Hardest Part Of ... |
3:15 |
2 |
2000 |
3 Doors Down |
Kryptonite |
3:53 |
3 |
2000 |
3 Doors Down |
Loser |
4:24 |
4 |
2000 |
504 Boyz |
Wobble Wobble |
3:35 |
... |
... |
... |
... |
... |
24087 |
2000 |
Yankee Grey |
Another Nine Minutes |
3:10 |
24088 |
2000 |
Yearwood, Trisha |
Real Live Woman |
3:55 |
24089 |
2000 |
Ying Yang Twins |
Whistle While You Tw... |
4:19 |
24090 |
2000 |
Zombie Nation |
Kernkraft 400 |
3:30 |
24091 |
2000 |
matchbox twenty |
Bent |
4:12 |
24092 rows × 4 columns
df_songs.drop_duplicates(inplace=True)
df_songs.shape
C:\Users\user\AppData\Local\Temp\ipykernel_6124\2197431707.py:1: SettingWithCopyWarning:
A value is trying to be set on a copy of a slice from a DataFrame
See the caveats in the documentation: https://pandas.pydata.org/pandas-docs/stable/user_guide/indexing.html#returning-a-view-versus-a-copy
df_songs.drop_duplicates(inplace=True) #drop_duplicates = 중복된 값 빼내기, (inplace=True)= 원본에도 저장
(317, 4)
df_songs['id'] = range(len(df_songs))
df_songs.head()
C:\Users\user\AppData\Local\Temp\ipykernel_6124\1941119944.py:1: SettingWithCopyWarning:
A value is trying to be set on a copy of a slice from a DataFrame.
Try using .loc[row_indexer,col_indexer] = value instead
See the caveats in the documentation: https://pandas.pydata.org/pandas-docs/stable/user_guide/indexing.html#returning-a-view-versus-a-copy
df_songs['id'] = range(len(df_songs))
|
year |
artist |
track |
time |
id |
0 |
2000 |
2 Pac |
Baby Don't Cry (Keep... |
4:22 |
0 |
1 |
2000 |
2Ge+her |
The Hardest Part Of ... |
3:15 |
1 |
2 |
2000 |
3 Doors Down |
Kryptonite |
3:53 |
2 |
3 |
2000 |
3 Doors Down |
Loser |
4:24 |
3 |
4 |
2000 |
504 Boyz |
Wobble Wobble |
3:35 |
4 |
df_ratings = df_melt.merge(df_songs,on=['year','artist','track','time'])
df_ratings
|
year |
artist |
track |
time |
date.entered |
week |
rating |
id |
0 |
2000 |
2 Pac |
Baby Don't Cry (Keep... |
4:22 |
2000-02-26 |
wk1 |
87.0 |
0 |
1 |
2000 |
2 Pac |
Baby Don't Cry (Keep... |
4:22 |
2000-02-26 |
wk2 |
82.0 |
0 |
2 |
2000 |
2 Pac |
Baby Don't Cry (Keep... |
4:22 |
2000-02-26 |
wk3 |
72.0 |
0 |
3 |
2000 |
2 Pac |
Baby Don't Cry (Keep... |
4:22 |
2000-02-26 |
wk4 |
77.0 |
0 |
4 |
2000 |
2 Pac |
Baby Don't Cry (Keep... |
4:22 |
2000-02-26 |
wk5 |
87.0 |
0 |
... |
... |
... |
... |
... |
... |
... |
... |
... |
24087 |
2000 |
matchbox twenty |
Bent |
4:12 |
2000-04-29 |
wk72 |
NaN |
316 |
24088 |
2000 |
matchbox twenty |
Bent |
4:12 |
2000-04-29 |
wk73 |
NaN |
316 |
24089 |
2000 |
matchbox twenty |
Bent |
4:12 |
2000-04-29 |
wk74 |
NaN |
316 |
24090 |
2000 |
matchbox twenty |
Bent |
4:12 |
2000-04-29 |
wk75 |
NaN |
316 |
24091 |
2000 |
matchbox twenty |
Bent |
4:12 |
2000-04-29 |
wk76 |
NaN |
316 |
24092 rows × 8 columns
df_ratings = df_ratings[['id', 'date.entered', 'week', 'rating']]
df_ratings
|
id |
date.entered |
week |
rating |
0 |
0 |
2000-02-26 |
wk1 |
87.0 |
1 |
0 |
2000-02-26 |
wk2 |
82.0 |
2 |
0 |
2000-02-26 |
wk3 |
72.0 |
3 |
0 |
2000-02-26 |
wk4 |
77.0 |
4 |
0 |
2000-02-26 |
wk5 |
87.0 |
... |
... |
... |
... |
... |
24087 |
316 |
2000-04-29 |
wk72 |
NaN |
24088 |
316 |
2000-04-29 |
wk73 |
NaN |
24089 |
316 |
2000-04-29 |
wk74 |
NaN |
24090 |
316 |
2000-04-29 |
wk75 |
NaN |
24091 |
316 |
2000-04-29 |
wk76 |
NaN |
24092 rows × 4 columns
df_songs
|
year |
artist |
track |
time |
id |
0 |
2000 |
2 Pac |
Baby Don't Cry (Keep... |
4:22 |
0 |
1 |
2000 |
2Ge+her |
The Hardest Part Of ... |
3:15 |
1 |
2 |
2000 |
3 Doors Down |
Kryptonite |
3:53 |
2 |
3 |
2000 |
3 Doors Down |
Loser |
4:24 |
3 |
4 |
2000 |
504 Boyz |
Wobble Wobble |
3:35 |
4 |
... |
... |
... |
... |
... |
... |
312 |
2000 |
Yankee Grey |
Another Nine Minutes |
3:10 |
312 |
313 |
2000 |
Yearwood, Trisha |
Real Live Woman |
3:55 |
313 |
314 |
2000 |
Ying Yang Twins |
Whistle While You Tw... |
4:19 |
314 |
315 |
2000 |
Zombie Nation |
Kernkraft 400 |
3:30 |
315 |
316 |
2000 |
matchbox twenty |
Bent |
4:12 |
316 |
317 rows × 5 columns
import numpy as np
plt.rcParams['axes.unicode_minus'] = False
import warnings
warnings.filterwarnings('ignore')
data = np.random.randint(-100,100,50).cumsum()
data
array([ 6, 20, 101, 128, 187, 205, 152, 123, 146, 100, 47,
46, -19, -27, 55, -43, -113, -168, -112, -38, -87, -64,
-128, -163, -209, -198, -148, -173, -125, -115, -55, -45, -55,
-123, -78, 2, -85, 14, -6, -80, -29, 54, 42, -41,
3, 93, 65, 104, 9, -56], dtype=int32)
import matplotlib.pyplot as plt
plt.plot(range(50),data,'r')
plt.title('시간별 가격 추이',fontproperties=fontprop)
plt.ylabel('주식가격',fontproperties=fontprop)
plt.xlabel('시간(분)',fontproperties=fontprop)
Text(0.5, 0, '시간(분)')
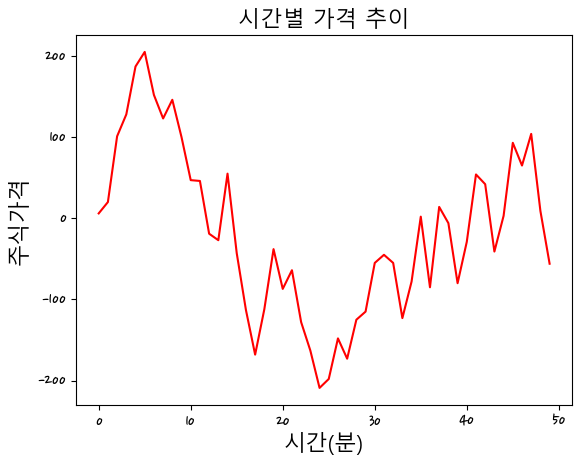
import matplotlib.font_manager as fm
path = 'malgun.ttf'
fontprop = fm.FontProperties(fname=path,size=16)
plt.plot(range(50),data,'r')
plt.title('시간별 가격 추이',fontproperties=fontprop)
plt.ylabel('주식가격',fontproperties=fontprop)
plt.xlabel('시간(분)',fontproperties=fontprop)
Text(0.5, 0, '시간(분)')
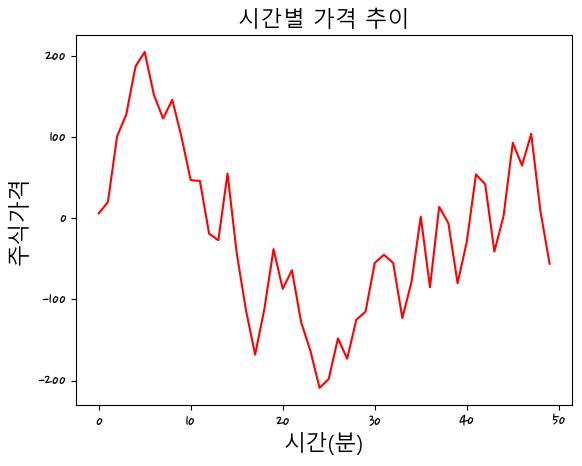
plt.rcParams['font.size']
14.0
plt.rcParams['font.family']
['Nanum Pen Script']
plt.rcParams['font.family'] = 'Nanum Pen Script'
plt.plot(range(50),data,'r')
plt.title('시간별 가격 추이')
plt.ylabel('주식가격')
plt.xlabel('시간(분)')
Text(0.5, 0, '시간(분)')
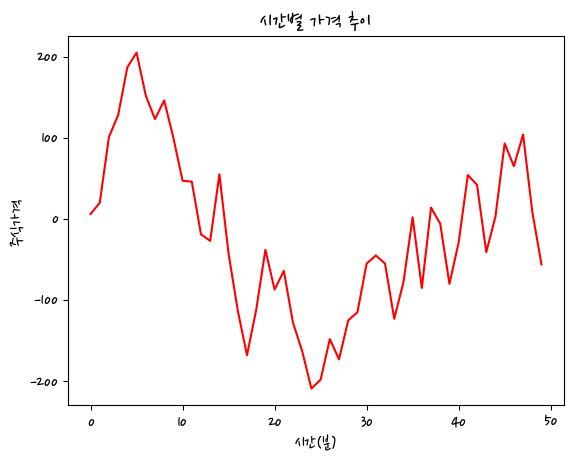
import matplotlib
matplotlib.matplotlib_fname()
'C:\\anaconda\\lib\\site-packages\\matplotlib\\mpl-data\\matplotlibrc'
fm.findSystemFonts()
['C:\\Windows\\Fonts\\H2MKPB.TTF',
'C:\\Windows\\Fonts\\ROCK.TTF',
'C:\\Windows\\Fonts\\FREESCPT.TTF',
'C:\\Windows\\Fonts\\JUICE___.TTF',
'C:\\Windows\\Fonts\\YuGothL.ttc',
'C:\\Windows\\Fonts\\comici.ttf',
'C:\\Windows\\Fonts\\ITCBLKAD.TTF',
'C:\\Windows\\Fonts\\DUBAI-REGULAR.TTF',
'C:\\Windows\\Fonts\\batang.ttc',
'C:\\Windows\\Fonts\\BOOKOSI.TTF',
'C:\\Windows\\Fonts\\HMKMMAG.TTF',
'C:\\Windows\\Fonts\\STZHONGS.TTF',
'C:\\Windows\\Fonts\\trebuc.ttf',
'C:\\Windows\\Fonts\\STCAIYUN.TTF',
'C:\\Windows\\Fonts\\consolaz.ttf',
'C:\\Windows\\Fonts\\FRABK.TTF',
'C:\\Windows\\Fonts\\marlett.ttf',
'C:\\Windows\\Fonts\\corbel.ttf',
'C:\\Windows\\Fonts\\cambriaz.ttf',
'C:\\Windows\\Fonts\\segoeuisl.ttf',
'C:\\Windows\\Fonts\\FRADM.TTF',
'C:\\Windows\\Fonts\\msyhbd.ttc',
'C:\\Windows\\Fonts\\constan.ttf',
'C:\\Windows\\Fonts\\TCM_____.TTF',
'C:\\Windows\\Fonts\\tahoma.ttf',
'C:\\Windows\\Fonts\\segoesc.ttf',
'C:\\Windows\\Fonts\\POORICH.TTF',
'C:\\Windows\\Fonts\\TCCM____.TTF',
'C:\\Windows\\Fonts\\LeelUIsl.ttf',
'C:\\Windows\\Fonts\\ITCKRIST.TTF',
'C:\\Windows\\Fonts\\ARIALN.TTF',
'C:\\Windows\\Fonts\\Candaral.ttf',
'C:\\Users\\user\\AppData\\Local\\Microsoft\\Windows\\Fonts\\NanumBrush.ttf',
'C:\\Windows\\Fonts\\MT.TTF',
'C:\\Windows\\Fonts\\micross.ttf',
'C:\\Windows\\Fonts\\MTEXTRA.TTF',
'C:\\Windows\\Fonts\\BELL.TTF',
'C:\\Windows\\Fonts\\framdit.ttf',
'C:\\Windows\\Fonts\\H2SA1M.TTF',
'C:\\Windows\\Fonts\\LTYPE.TTF',
'C:\\Windows\\Fonts\\BRLNSDB.TTF',
'C:\\Windows\\Fonts\\georgiaz.ttf',
'C:\\Windows\\Fonts\\LSANSI.TTF',
'C:\\Windows\\Fonts\\LTYPEO.TTF',
'C:\\Windows\\Fonts\\FRADMIT.TTF',
'C:\\Windows\\Fonts\\ROCC____.TTF',
'C:\\Windows\\Fonts\\seguihis.ttf',
'C:\\Windows\\Fonts\\BELLB.TTF',
'C:\\Windows\\Fonts\\TCMI____.TTF',
'C:\\Windows\\Fonts\\HTOWERT.TTF',
'C:\\Windows\\Fonts\\STLITI.TTF',
'C:\\Windows\\Fonts\\Sitka.ttc',
'C:\\Windows\\Fonts\\corbelb.ttf',
'C:\\Windows\\Fonts\\HARLOWSI.TTF',
'C:\\Windows\\Fonts\\ELEPHNT.TTF',
'C:\\Windows\\Fonts\\BOD_B.TTF',
'C:\\Windows\\Fonts\\georgiab.ttf',
'C:\\Windows\\Fonts\\LFAXI.TTF',
'C:\\Windows\\Fonts\\malgunsl.ttf',
'C:\\Windows\\Fonts\\l_10646.ttf',
'C:\\Windows\\Fonts\\MSUIGHUR.TTF',
'C:\\Windows\\Fonts\\segoepr.ttf',
'C:\\Windows\\Fonts\\timesbi.ttf',
'C:\\Windows\\Fonts\\simsunb.ttf',
'C:\\Windows\\Fonts\\TCCB____.TTF',
'C:\\Windows\\Fonts\\WINGDNG3.TTF',
'C:\\Windows\\Fonts\\MATURASC.TTF',
'C:\\Windows\\Fonts\\BOOKOSB.TTF',
'C:\\Windows\\Fonts\\PAPYRUS.TTF',
'C:\\Windows\\Fonts\\LEELAWAD.TTF',
'C:\\Windows\\Fonts\\PERTILI.TTF',
'C:\\Windows\\Fonts\\LSANSDI.TTF',
'C:\\Windows\\Fonts\\BSSYM7.TTF',
'C:\\Windows\\Fonts\\segoeuil.ttf',
'C:\\Windows\\Fonts\\PARCHM.TTF',
'C:\\Windows\\Fonts\\ROCKEB.TTF',
'C:\\Windows\\Fonts\\CURLZ___.TTF',
'C:\\Windows\\Fonts\\framd.ttf',
'C:\\Windows\\Fonts\\CHILLER.TTF',
'C:\\Windows\\Fonts\\HANBatangB.ttf',
'C:\\Windows\\Fonts\\ANTQUAB.TTF',
'C:\\Windows\\Fonts\\calibri.ttf',
'C:\\Windows\\Fonts\\gadugi.ttf',
'C:\\Windows\\Fonts\\SitkaI.ttc',
'C:\\Windows\\Fonts\\BERNHC.TTF',
'C:\\Windows\\Fonts\\verdanai.ttf',
'C:\\Windows\\Fonts\\FRAMDCN.TTF',
'C:\\Windows\\Fonts\\WINGDNG2.TTF',
'C:\\Windows\\Fonts\\MG.TTF',
'C:\\Windows\\Fonts\\ARIALNBI.TTF',
'C:\\Windows\\Fonts\\mvboli.ttf',
'C:\\Windows\\Fonts\\FZYTK.TTF',
'C:\\Windows\\Fonts\\SIMLI.TTF',
'C:\\Windows\\Fonts\\PERB____.TTF',
'C:\\Windows\\Fonts\\bahnschrift.ttf',
'C:\\Windows\\Fonts\\Candaraz.ttf',
'C:\\Windows\\Fonts\\georgiai.ttf',
'C:\\Windows\\Fonts\\SCRIPTBL.TTF',
'C:\\Windows\\Fonts\\YuGothR.ttc',
'C:\\Windows\\Fonts\\PERTIBD.TTF',
'C:\\Windows\\Fonts\\HANDotumExt.ttf',
'C:\\Windows\\Fonts\\HMKMRHD.TTF',
'C:\\Windows\\Fonts\\BOD_PSTC.TTF',
'C:\\Windows\\Fonts\\GOUDOS.TTF',
'C:\\Windows\\Fonts\\VINERITC.TTF',
'C:\\Windows\\Fonts\\H2PORL.TTF',
'C:\\Windows\\Fonts\\H2GTRE.TTF',
'C:\\Windows\\Fonts\\ERASBD.TTF',
'C:\\Windows\\Fonts\\arial.ttf',
'C:\\Windows\\Fonts\\ROCKBI.TTF',
'C:\\Windows\\Fonts\\OLDENGL.TTF',
'C:\\Windows\\Fonts\\BOD_CI.TTF',
'C:\\Windows\\Fonts\\cour.ttf',
'C:\\Windows\\Fonts\\calibrili.ttf',
'C:\\Windows\\Fonts\\BAUHS93.TTF',
'C:\\Windows\\Fonts\\HMFMMUEX.TTC',
'C:\\Windows\\Fonts\\calibriz.ttf',
'C:\\Windows\\Fonts\\MOD20.TTF',
'C:\\Windows\\Fonts\\GIGI.TTF',
'C:\\Windows\\Fonts\\segmdl2.ttf',
'C:\\Windows\\Fonts\\H2GTRM.TTF',
'C:\\Windows\\Fonts\\OUTLOOK.TTF',
'C:\\Windows\\Fonts\\SCHLBKB.TTF',
'C:\\Windows\\Fonts\\segoeuiz.ttf',
'C:\\Windows\\Fonts\\segoeui.ttf',
'C:\\Windows\\Fonts\\constanz.ttf',
'C:\\Windows\\Fonts\\simsun.ttc',
'C:\\Windows\\Fonts\\ANTQUAI.TTF',
'C:\\Windows\\Fonts\\HARNGTON.TTF',
'C:\\Windows\\Fonts\\NGULIM.TTF',
'C:\\Windows\\Fonts\\georgia.ttf',
'C:\\Windows\\Fonts\\TEMPSITC.TTF',
'C:\\Windows\\Fonts\\ebrima.ttf',
'C:\\Windows\\Fonts\\CASTELAR.TTF',
'C:\\Windows\\Fonts\\GOTHIC.TTF',
'C:\\Windows\\Fonts\\COPRGTL.TTF',
'C:\\Windows\\Fonts\\ENGR.TTF',
'C:\\Windows\\Fonts\\CALISTB.TTF',
'C:\\Windows\\Fonts\\BKANT.TTF',
'C:\\Windows\\Fonts\\GILI____.TTF',
'C:\\Windows\\Fonts\\NirmalaS.ttf',
'C:\\Windows\\Fonts\\GOTHICBI.TTF',
'C:\\Windows\\Fonts\\MAIAN.TTF',
'C:\\Windows\\Fonts\\LFAX.TTF',
'C:\\Windows\\Fonts\\msyh.ttc',
'C:\\Windows\\Fonts\\consolab.ttf',
'C:\\Windows\\Fonts\\H2MJRE.TTF',
'C:\\Windows\\Fonts\\msyhl.ttc',
'C:\\Windows\\Fonts\\GILBI___.TTF',
'C:\\Windows\\Fonts\\courbi.ttf',
'C:\\Windows\\Fonts\\DUBAI-BOLD.TTF',
'C:\\Windows\\Fonts\\SNAP____.TTF',
'C:\\Windows\\Fonts\\GOTHICI.TTF',
'C:\\Windows\\Fonts\\PRISTINA.TTF',
'C:\\Windows\\Fonts\\seguibl.ttf',
'C:\\Windows\\Fonts\\LBRITED.TTF',
'C:\\Windows\\Fonts\\BOD_BLAI.TTF',
'C:\\Windows\\Fonts\\STFANGSO.TTF',
'C:\\Windows\\Fonts\\OCRAEXT.TTF',
'C:\\Windows\\Fonts\\GILB____.TTF',
'C:\\Windows\\Fonts\\seguiemj.ttf',
'C:\\Windows\\Fonts\\malgunbd.ttf',
'C:\\Windows\\Fonts\\COLONNA.TTF',
'C:\\Windows\\Fonts\\PER_____.TTF',
'C:\\Windows\\Fonts\\ebrimabd.ttf',
'C:\\Windows\\Fonts\\sylfaen.ttf',
'C:\\Windows\\Fonts\\STENCIL.TTF',
'C:\\Windows\\Fonts\\wingding.ttf',
'C:\\Windows\\Fonts\\CALISTI.TTF',
'C:\\Windows\\Fonts\\Candarab.ttf',
'C:\\Windows\\Fonts\\gulim.ttc',
'C:\\Windows\\Fonts\\BRADHITC.TTF',
'C:\\Windows\\Fonts\\corbelli.ttf',
'C:\\Windows\\Fonts\\palab.ttf',
'C:\\Windows\\Fonts\\phagspab.ttf',
'C:\\Windows\\Fonts\\TCCEB.TTF',
'C:\\Windows\\Fonts\\MM.TTF',
'C:\\Windows\\Fonts\\Nirmala.ttf',
'C:\\Windows\\Fonts\\courbd.ttf',
'C:\\Windows\\Fonts\\seguibli.ttf',
'C:\\Windows\\Fonts\\BOD_BLAR.TTF',
'C:\\Windows\\Fonts\\calibrii.ttf',
'C:\\Windows\\Fonts\\arialbi.ttf',
'C:\\Windows\\Fonts\\RAVIE.TTF',
'C:\\Windows\\Fonts\\msyi.ttf',
'C:\\Windows\\Fonts\\corbell.ttf',
'C:\\Windows\\Fonts\\BRUSHSCI.TTF',
'C:\\Windows\\Fonts\\NirmalaB.ttf',
'C:\\Windows\\Fonts\\couri.ttf',
'C:\\Windows\\Fonts\\HATTEN.TTF',
'C:\\Windows\\Fonts\\ERASDEMI.TTF',
'C:\\Windows\\Fonts\\timesbd.ttf',
'C:\\Windows\\Fonts\\FELIXTI.TTF',
'C:\\Windows\\Fonts\\FRABKIT.TTF',
'C:\\Windows\\Fonts\\msjh.ttc',
'C:\\Windows\\Fonts\\ROCKI.TTF',
'C:\\Windows\\Fonts\\FTLTLT.TTF',
'C:\\Windows\\Fonts\\webdings.ttf',
'C:\\Windows\\Fonts\\corbeli.ttf',
'C:\\Windows\\Fonts\\GOUDOSB.TTF',
'C:\\Windows\\Fonts\\CENSCBK.TTF',
'C:\\Windows\\Fonts\\NIAGSOL.TTF',
'C:\\Windows\\Fonts\\CALIFR.TTF',
'C:\\Windows\\Fonts\\LTYPEB.TTF',
'C:\\Windows\\Fonts\\ntailu.ttf',
'C:\\Windows\\Fonts\\constani.ttf',
'C:\\Windows\\Fonts\\LSANSD.TTF',
'C:\\Windows\\Fonts\\ARIALNB.TTF',
'C:\\Windows\\Fonts\\PLAYBILL.TTF',
'C:\\Windows\\Fonts\\HTOWERTI.TTF',
'C:\\Windows\\Fonts\\BOD_CR.TTF',
'C:\\Windows\\Fonts\\HYHWPEQ.TTF',
'C:\\Windows\\Fonts\\comicz.ttf',
'C:\\Windows\\Fonts\\LHANDW.TTF',
'C:\\Windows\\Fonts\\BROADW.TTF',
'C:\\Users\\user\\AppData\\Local\\Microsoft\\Windows\\Fonts\\NanumBarunGothic-YetHangul.ttf',
'C:\\Windows\\Fonts\\ERASMD.TTF',
'C:\\Windows\\Fonts\\SCHLBKBI.TTF',
'C:\\Windows\\Fonts\\SIMYOU.TTF',
'C:\\Windows\\Fonts\\calibrib.ttf',
'C:\\Windows\\Fonts\\MK.TTF',
'C:\\Windows\\Fonts\\ROCKB.TTF',
'C:\\Windows\\Fonts\\BRITANIC.TTF',
'C:\\Windows\\Fonts\\MAGNETOB.TTF',
'C:\\Windows\\Fonts\\GLECB.TTF',
'C:\\Windows\\Fonts\\GLSNECB.TTF',
'C:\\Windows\\Fonts\\ARIALNI.TTF',
'C:\\Windows\\Fonts\\BOOKOS.TTF',
'C:\\Windows\\Fonts\\ROCCB___.TTF',
'C:\\Windows\\Fonts\\MB.TTF',
'C:\\Windows\\Fonts\\times.ttf',
'C:\\Windows\\Fonts\\LBRITEDI.TTF',
'C:\\Windows\\Fonts\\HMKMAMI.TTF',
'C:\\Windows\\Fonts\\RAGE.TTF',
'C:\\Windows\\Fonts\\STXINWEI.TTF',
'C:\\Windows\\Fonts\\COOPBL.TTF',
'C:\\Windows\\Fonts\\STXIHEI.TTF',
'C:\\Windows\\Fonts\\BOOKOSBI.TTF',
'C:\\Windows\\Fonts\\NIAGENG.TTF',
'C:\\Windows\\Fonts\\LBRITEI.TTF',
'C:\\Windows\\Fonts\\\x7f\x7f\x7f\x7fBOLD.TTF',
'C:\\Windows\\Fonts\\trebucbi.ttf',
'C:\\Users\\user\\AppData\\Local\\Microsoft\\Windows\\Fonts\\NanumPen.ttf',
'C:\\Windows\\Fonts\\seguisb.ttf',
'C:\\Windows\\Fonts\\CENTURY.TTF',
'C:\\Windows\\Fonts\\MP.TTF',
'C:\\Windows\\Fonts\\GOTHICB.TTF',
'C:\\Windows\\Fonts\\LATINWD.TTF',
'C:\\Windows\\Fonts\\BRLNSR.TTF',
'C:\\Windows\\Fonts\\HMFMOLD.TTF',
'C:\\Windows\\Fonts\\ntailub.ttf',
'C:\\Windows\\Fonts\\FZSTK.TTF',
'C:\\Windows\\Fonts\\corbelz.ttf',
'C:\\Windows\\Fonts\\STKAITI.TTF',
'C:\\Windows\\Fonts\\lucon.ttf',
'C:\\Windows\\Fonts\\PERBI___.TTF',
'C:\\Windows\\Fonts\\mmrtextb.ttf',
'C:\\Windows\\Fonts\\LeelaUIb.ttf',
'C:\\Windows\\Fonts\\GILSANUB.TTF',
'C:\\Windows\\Fonts\\palabi.ttf',
'C:\\Windows\\Fonts\\BOD_R.TTF',
'C:\\Windows\\Fonts\\FRSCRIPT.TTF',
'C:\\Windows\\Fonts\\seguisli.ttf',
'C:\\Windows\\Fonts\\segoeprb.ttf',
'C:\\Windows\\Fonts\\\x7f\x7f\x7f\x7f.TTF',
'C:\\Windows\\Fonts\\comic.ttf',
'C:\\Windows\\Fonts\\segoescb.ttf',
'C:\\Windows\\Fonts\\GARABD.TTF',
'C:\\Windows\\Fonts\\comicbd.ttf',
'C:\\Windows\\Fonts\\arialbd.ttf',
'C:\\Windows\\Fonts\\SitkaZ.ttc',
'C:\\Windows\\Fonts\\TCB_____.TTF',
'C:\\Windows\\Fonts\\MISTRAL.TTF',
'C:\\Windows\\Fonts\\consola.ttf',
'C:\\Windows\\Fonts\\GOUDYSTO.TTF',
'C:\\Windows\\Fonts\\cambriab.ttf',
'C:\\Windows\\Fonts\\H2GPRM.TTF',
'C:\\Windows\\Fonts\\\x7f\x7f\x7f\x7fEXTRABOLD.TTF',
'C:\\Windows\\Fonts\\DUBAI-LIGHT.TTF',
'C:\\Windows\\Fonts\\AGENCYB.TTF',
'C:\\Windows\\Fonts\\STSONG.TTF',
'C:\\Windows\\Fonts\\HANDotum.ttf',
'C:\\Windows\\Fonts\\trebucbd.ttf',
'C:\\Windows\\Fonts\\FRADMCN.TTF',
'C:\\Windows\\Fonts\\malgun.ttf',
'C:\\Windows\\Fonts\\H2GSRB.TTF',
'C:\\Windows\\Fonts\\ELEPHNTI.TTF',
'C:\\Windows\\Fonts\\COPRGTB.TTF',
'C:\\Windows\\Fonts\\BASKVILL.TTF',
'C:\\Windows\\Fonts\\CALIFI.TTF',
'C:\\Windows\\Fonts\\HMFMPYUN.TTF',
'C:\\Windows\\Fonts\\BOD_CBI.TTF',
'C:\\Windows\\Fonts\\CALIFB.TTF',
'C:\\Windows\\Fonts\\BRLNSB.TTF',
'C:\\Windows\\Fonts\\cambriai.ttf',
'C:\\Windows\\Fonts\\CENTAUR.TTF',
'C:\\Windows\\Fonts\\timesi.ttf',
'C:\\Windows\\Fonts\\seguisym.ttf',
'C:\\Windows\\Fonts\\VIVALDII.TTF',
'C:\\Windows\\Fonts\\H2PORM.TTF',
'C:\\Windows\\Fonts\\JUMJA.TTF',
'C:\\Windows\\Fonts\\monbaiti.ttf',
'C:\\Windows\\Fonts\\HANBatang.ttf',
'C:\\Windows\\Fonts\\cambria.ttc',
'C:\\Windows\\Fonts\\KUNSTLER.TTF',
'C:\\Windows\\Fonts\\AGENCYR.TTF',
'C:\\Windows\\Fonts\\mingliub.ttc',
'C:\\Windows\\Fonts\\palai.ttf',
'C:\\Windows\\Fonts\\LBRITE.TTF',
'C:\\Windows\\Fonts\\ERASLGHT.TTF',
'C:\\Windows\\Fonts\\STHUPO.TTF',
'C:\\Windows\\Fonts\\Gabriola.ttf',
'C:\\Windows\\Fonts\\IMPRISHA.TTF',
'C:\\Windows\\Fonts\\tahomabd.ttf',
'C:\\Windows\\Fonts\\REFSAN.TTF',
'C:\\Windows\\Fonts\\ALGER.TTF',
'C:\\Windows\\Fonts\\TSPECIAL1.TTF',
'C:\\Windows\\Fonts\\segoeuii.ttf',
'C:\\Windows\\Fonts\\LFAXD.TTF',
'C:\\Windows\\Fonts\\seguisbi.ttf',
'C:\\Windows\\Fonts\\holomdl2.ttf',
'C:\\Windows\\Fonts\\HANBatangExt.ttf',
'C:\\Windows\\Fonts\\SHOWG.TTF',
'C:\\Windows\\Fonts\\VLADIMIR.TTF',
'C:\\Windows\\Fonts\\constanb.ttf',
'C:\\Windows\\Fonts\\msjhl.ttc',
'C:\\Windows\\Fonts\\impact.ttf',
'C:\\Windows\\Fonts\\TCBI____.TTF',
'C:\\Windows\\Fonts\\BOD_I.TTF',
'C:\\Windows\\Fonts\\CALIST.TTF',
'C:\\Windows\\Fonts\\seguili.ttf',
'C:\\Windows\\Fonts\\YuGothB.ttc',
'C:\\Windows\\Fonts\\msgothic.ttc',
'C:\\Windows\\Fonts\\ariali.ttf',
'C:\\Windows\\Fonts\\MJ.TTF',
'C:\\Windows\\Fonts\\Candarai.ttf',
'C:\\Windows\\Fonts\\segoeuib.ttf',
'C:\\Windows\\Fonts\\calibril.ttf',
'C:\\Windows\\Fonts\\trebucit.ttf',
'C:\\Windows\\Fonts\\STXINGKA.TTF',
'C:\\Windows\\Fonts\\FRAHVIT.TTF',
'C:\\Windows\\Fonts\\REFSPCL.TTF',
'C:\\Windows\\Fonts\\ITCEDSCR.TTF',
'C:\\Windows\\Fonts\\taileb.ttf',
'C:\\Windows\\Fonts\\LTYPEBO.TTF',
'C:\\Windows\\Fonts\\GIL_____.TTF',
'C:\\Windows\\Fonts\\gadugib.ttf',
'C:\\Windows\\Fonts\\LFAXDI.TTF',
'C:\\Windows\\Fonts\\CALISTBI.TTF',
'C:\\Windows\\Fonts\\verdanaz.ttf',
'C:\\Windows\\Fonts\\MN.TTF',
'C:\\Windows\\Fonts\\GARA.TTF',
'C:\\Windows\\Fonts\\SitkaB.ttc',
'C:\\Windows\\Fonts\\HANBatangExtBB.ttf',
'C:\\Windows\\Fonts\\msjhbd.ttc',
'C:\\Windows\\Fonts\\MSUIGHUB.TTF',
'C:\\Windows\\Fonts\\DUBAI-MEDIUM.TTF',
'C:\\Windows\\Fonts\\ANTQUABI.TTF',
'C:\\Windows\\Fonts\\pala.ttf',
'C:\\Windows\\Fonts\\INFROMAN.TTF',
'C:\\Windows\\Fonts\\GOUDOSI.TTF',
'C:\\Windows\\Fonts\\HANBatangExtB.ttf',
'C:\\Windows\\Fonts\\ariblk.ttf',
'C:\\Windows\\Fonts\\BELLI.TTF',
'C:\\Windows\\Fonts\\SCHLBKI.TTF',
'C:\\Windows\\Fonts\\Candarali.ttf',
'C:\\Windows\\Fonts\\PALSCRI.TTF',
'C:\\Windows\\Fonts\\LeelawUI.ttf',
'C:\\Windows\\Fonts\\symbol.ttf',
'C:\\Windows\\Fonts\\phagspa.ttf',
'C:\\Windows\\Fonts\\GARAIT.TTF',
'C:\\Windows\\Fonts\\LSANS.TTF',
'C:\\Windows\\Fonts\\JOKERMAN.TTF',
'C:\\Windows\\Fonts\\BOD_BI.TTF',
'C:\\Windows\\Fonts\\verdanab.ttf',
'C:\\Windows\\Fonts\\HANDotumB.ttf',
'C:\\Windows\\Fonts\\LEELAWDB.TTF',
'C:\\Windows\\Fonts\\ONYX.TTF',
'C:\\Windows\\Fonts\\Candara.ttf',
'C:\\Windows\\Fonts\\MH.TTF',
'C:\\Windows\\Fonts\\FORTE.TTF',
'C:\\Windows\\Fonts\\H2HDRM.TTF',
'C:\\Windows\\Fonts\\LCALLIG.TTF',
'C:\\Windows\\Fonts\\Inkfree.ttf',
'C:\\Windows\\Fonts\\consolai.ttf',
'C:\\Windows\\Fonts\\MTCORSVA.TTF',
'C:\\Windows\\Fonts\\FRAHV.TTF',
'C:\\Windows\\Fonts\\ARLRDBD.TTF',
'C:\\Windows\\Fonts\\PERI____.TTF',
'C:\\Windows\\Fonts\\H2MJSM.TTF',
'C:\\Windows\\Fonts\\javatext.ttf',
'C:\\Windows\\Fonts\\mmrtext.ttf',
'C:\\Windows\\Fonts\\YuGothM.ttc',
'C:\\Windows\\Fonts\\verdana.ttf',
'C:\\Windows\\Fonts\\GILLUBCD.TTF',
'C:\\Windows\\Fonts\\taile.ttf',
'C:\\Windows\\Fonts\\GILC____.TTF',
'C:\\Windows\\Fonts\\BOD_CB.TTF',
'C:\\Windows\\Fonts\\himalaya.ttf']
[(f.fname, f.name) for f in fm.fontManager.ttflist if 'Malgun' in f.name]
[('C:\\Windows\\Fonts\\malgunbd.ttf', 'Malgun Gothic'),
('C:\\Windows\\Fonts\\malgun.ttf', 'Malgun Gothic'),
('C:\\Windows\\Fonts\\malgunsl.ttf', 'Malgun Gothic')]
[(f.fname, f.name) for f in fm.fontManager.ttflist if 'Nanum' in f.name]
[('C:\\Users\\user\\AppData\\Local\\Microsoft\\Windows\\Fonts\\NanumPen.ttf',
'Nanum Pen Script'),
('C:\\Users\\user\\AppData\\Local\\Microsoft\\Windows\\Fonts\\NanumBarunGothic-YetHangul.ttf',
'NanumBarunGothic YetHangul'),
('C:\\Windows\\Fonts\\\x7f\x7f\x7f\x7fBOLD.TTF', 'NanumGothic'),
('C:\\Users\\user\\AppData\\Local\\Microsoft\\Windows\\Fonts\\NanumBrush.ttf',
'Nanum Brush Script'),
('C:\\Windows\\Fonts\\\x7f\x7f\x7f\x7fEXTRABOLD.TTF', 'NanumGothic'),
('C:\\Windows\\Fonts\\\x7f\x7f\x7f\x7f.TTF', 'NanumGothic')]
matplotlib.matplotlib_fname()
'C:\\anaconda\\lib\\site-packages\\matplotlib\\mpl-data\\matplotlibrc'
import pandas as pd
first_df = pd.read_csv('전국_평균_분양가격(2013년_9월부터_2015년_8월까지).csv',encoding='cp949')
first_df.head(2)
|
지역 |
2013년12월 |
2014년1월 |
2014년2월 |
2014년3월 |
2014년4월 |
2014년5월 |
2014년6월 |
2014년7월 |
2014년8월 |
... |
2014년11월 |
2014년12월 |
2015년1월 |
2015년2월 |
2015년3월 |
2015년4월 |
2015년5월 |
2015년6월 |
2015년7월 |
2015년8월 |
0 |
서울 |
18189 |
17925 |
17925 |
18016 |
18098 |
19446 |
18867 |
18742 |
19274 |
... |
20242 |
20269 |
20670 |
20670 |
19415 |
18842 |
18367 |
18374 |
18152 |
18443 |
1 |
부산 |
8111 |
8111 |
9078 |
8965 |
9402 |
9501 |
9453 |
9457 |
9411 |
... |
9208 |
9208 |
9204 |
9235 |
9279 |
9327 |
9345 |
9515 |
9559 |
9581 |
2 rows × 22 columns
first_df.info()
<class 'pandas.core.frame.DataFrame'>
RangeIndex: 17 entries, 0 to 16
Data columns (total 22 columns):
# Column Non-Null Count Dtype
--- ------ -------------- -----
0 지역 17 non-null object
1 2013년12월 17 non-null int64
2 2014년1월 17 non-null int64
3 2014년2월 17 non-null int64
4 2014년3월 17 non-null int64
5 2014년4월 17 non-null int64
6 2014년5월 17 non-null int64
7 2014년6월 17 non-null int64
8 2014년7월 17 non-null int64
9 2014년8월 17 non-null int64
10 2014년9월 17 non-null int64
11 2014년10월 17 non-null int64
12 2014년11월 17 non-null int64
13 2014년12월 17 non-null int64
14 2015년1월 17 non-null int64
15 2015년2월 17 non-null int64
16 2015년3월 17 non-null int64
17 2015년4월 17 non-null int64
18 2015년5월 17 non-null int64
19 2015년6월 17 non-null int64
20 2015년7월 17 non-null int64
21 2015년8월 17 non-null int64
dtypes: int64(21), object(1)
memory usage: 3.0+ KB
last_df = pd.read_csv('주택도시보증공사_전국_평균_분양가격(2019년_12월).csv', encoding='cp949')
last_df.head(2)
|
지역명 |
규모구분 |
연도 |
월 |
분양가격(㎡) |
0 |
서울 |
전체 |
2015 |
10 |
5841 |
1 |
서울 |
전용면적 60㎡이하 |
2015 |
10 |
5652 |
last_df.info()
<class 'pandas.core.frame.DataFrame'>
RangeIndex: 4335 entries, 0 to 4334
Data columns (total 5 columns):
# Column Non-Null Count Dtype
--- ------ -------------- -----
0 지역명 4335 non-null object
1 규모구분 4335 non-null object
2 연도 4335 non-null int64
3 월 4335 non-null int64
4 분양가격(㎡) 4058 non-null object
dtypes: int64(2), object(3)
memory usage: 169.5+ KB
last_df.isna().sum()
지역명 0
규모구분 0
연도 0
월 0
분양가격(㎡) 277
dtype: int64
last_df['분양가격']=pd.to_numeric(last_df['분양가격(㎡)'],errors='coerce')
from numpy import nan
type(nan)
float
last_df.info()
<class 'pandas.core.frame.DataFrame'>
RangeIndex: 4335 entries, 0 to 4334
Data columns (total 8 columns):
# Column Non-Null Count Dtype
--- ------ -------------- -----
0 지역명 4335 non-null object
1 규모구분 4335 non-null object
2 연도 4335 non-null int64
3 월 4335 non-null int64
4 분양가격(㎡) 4058 non-null object
5 분양가격 3957 non-null float64
6 평당분양가격 3957 non-null float64
7 전용면적 4335 non-null object
dtypes: float64(2), int64(2), object(4)
memory usage: 271.1+ KB
last_df['평당분양가격']=last_df['분양가격']*3.3
last_df['평당분양가격'].describe()
count 3957.000000
mean 10685.824488
std 4172.222780
min 6164.400000
25% 8055.300000
50% 9484.200000
75% 11751.300000
max 42002.400000
Name: 평당분양가격, dtype: float64
last_df
|
지역명 |
규모구분 |
연도 |
월 |
분양가격(㎡) |
분양가격 |
평당분양가격 |
전용면적 |
0 |
서울 |
전체 |
2015 |
10 |
5841 |
5841.0 |
19275.3 |
전체 |
1 |
서울 |
전용면적 60㎡이하 |
2015 |
10 |
5652 |
5652.0 |
18651.6 |
60㎡~ |
2 |
서울 |
전용면적 60㎡초과 85㎡이하 |
2015 |
10 |
5882 |
5882.0 |
19410.6 |
60㎡~85㎡~ |
3 |
서울 |
전용면적 85㎡초과 102㎡이하 |
2015 |
10 |
5721 |
5721.0 |
18879.3 |
85㎡~102㎡~ |
4 |
서울 |
전용면적 102㎡초과 |
2015 |
10 |
5879 |
5879.0 |
19400.7 |
102㎡~ |
... |
... |
... |
... |
... |
... |
... |
... |
... |
4330 |
제주 |
전체 |
2019 |
12 |
3882 |
3882.0 |
12810.6 |
전체 |
4331 |
제주 |
전용면적 60㎡이하 |
2019 |
12 |
NaN |
NaN |
NaN |
60㎡~ |
4332 |
제주 |
전용면적 60㎡초과 85㎡이하 |
2019 |
12 |
3898 |
3898.0 |
12863.4 |
60㎡~85㎡~ |
4333 |
제주 |
전용면적 85㎡초과 102㎡이하 |
2019 |
12 |
NaN |
NaN |
NaN |
85㎡~102㎡~ |
4334 |
제주 |
전용면적 102㎡초과 |
2019 |
12 |
3601 |
3601.0 |
11883.3 |
102㎡~ |
4335 rows × 8 columns
last_df.규모구분.unique()
array(['전체', '전용면적 60㎡이하', '전용면적 60㎡초과 85㎡이하', '전용면적 85㎡초과 102㎡이하',
'전용면적 102㎡초과'], dtype=object)
last_df.전용면적 = last_df.규모구분.str.replace('전용면적', '')
last_df
|
지역명 |
규모구분 |
연도 |
월 |
분양가격(㎡) |
분양가격 |
평당분양가격 |
전용면적 |
0 |
서울 |
전체 |
2015 |
10 |
5841 |
5841.0 |
19275.3 |
전체 |
1 |
서울 |
전용면적 60㎡이하 |
2015 |
10 |
5652 |
5652.0 |
18651.6 |
60㎡~ |
2 |
서울 |
전용면적 60㎡초과 85㎡이하 |
2015 |
10 |
5882 |
5882.0 |
19410.6 |
60㎡~85㎡~ |
3 |
서울 |
전용면적 85㎡초과 102㎡이하 |
2015 |
10 |
5721 |
5721.0 |
18879.3 |
85㎡~102㎡~ |
4 |
서울 |
전용면적 102㎡초과 |
2015 |
10 |
5879 |
5879.0 |
19400.7 |
102㎡~ |
... |
... |
... |
... |
... |
... |
... |
... |
... |
4330 |
제주 |
전체 |
2019 |
12 |
3882 |
3882.0 |
12810.6 |
전체 |
4331 |
제주 |
전용면적 60㎡이하 |
2019 |
12 |
NaN |
NaN |
NaN |
60㎡~ |
4332 |
제주 |
전용면적 60㎡초과 85㎡이하 |
2019 |
12 |
3898 |
3898.0 |
12863.4 |
60㎡~85㎡~ |
4333 |
제주 |
전용면적 85㎡초과 102㎡이하 |
2019 |
12 |
NaN |
NaN |
NaN |
85㎡~102㎡~ |
4334 |
제주 |
전용면적 102㎡초과 |
2019 |
12 |
3601 |
3601.0 |
11883.3 |
102㎡~ |
4335 rows × 8 columns
last_df['전용면적'] = last_df.['전용면적'].str.replace('초과','~')
last_df
File "C:\Users\user\AppData\Local\Temp\ipykernel_14356\3079234100.py", line 1
last_df['전용면적'] = last_df.['전용면적'].str.replace('초과','~') #last_df.전용면적 = 제대로 안 들어감
^
SyntaxError: invalid syntax
last_df['전용면적'] = last_df['전용면적'].str.replace('이하','~')
last_df
|
지역명 |
규모구분 |
연도 |
월 |
분양가격(㎡) |
분양가격 |
평당분양가격 |
전용면적 |
0 |
서울 |
전체 |
2015 |
10 |
5841 |
5841.0 |
19275.3 |
전체 |
1 |
서울 |
전용면적 60㎡이하 |
2015 |
10 |
5652 |
5652.0 |
18651.6 |
60㎡~ |
2 |
서울 |
전용면적 60㎡초과 85㎡이하 |
2015 |
10 |
5882 |
5882.0 |
19410.6 |
60㎡~85㎡~ |
3 |
서울 |
전용면적 85㎡초과 102㎡이하 |
2015 |
10 |
5721 |
5721.0 |
18879.3 |
85㎡~102㎡~ |
4 |
서울 |
전용면적 102㎡초과 |
2015 |
10 |
5879 |
5879.0 |
19400.7 |
102㎡~ |
... |
... |
... |
... |
... |
... |
... |
... |
... |
4330 |
제주 |
전체 |
2019 |
12 |
3882 |
3882.0 |
12810.6 |
전체 |
4331 |
제주 |
전용면적 60㎡이하 |
2019 |
12 |
NaN |
NaN |
NaN |
60㎡~ |
4332 |
제주 |
전용면적 60㎡초과 85㎡이하 |
2019 |
12 |
3898 |
3898.0 |
12863.4 |
60㎡~85㎡~ |
4333 |
제주 |
전용면적 85㎡초과 102㎡이하 |
2019 |
12 |
NaN |
NaN |
NaN |
85㎡~102㎡~ |
4334 |
제주 |
전용면적 102㎡초과 |
2019 |
12 |
3601 |
3601.0 |
11883.3 |
102㎡~ |
4335 rows × 8 columns
last_df['전용면적'] = last_df['전용면적'].str.replace(' ','').str.strip()
last_df
|
지역명 |
규모구분 |
연도 |
월 |
분양가격(㎡) |
분양가격 |
평당분양가격 |
전용면적 |
0 |
서울 |
전체 |
2015 |
10 |
5841 |
5841.0 |
19275.3 |
전체 |
1 |
서울 |
전용면적 60㎡이하 |
2015 |
10 |
5652 |
5652.0 |
18651.6 |
60㎡~ |
2 |
서울 |
전용면적 60㎡초과 85㎡이하 |
2015 |
10 |
5882 |
5882.0 |
19410.6 |
60㎡~85㎡~ |
3 |
서울 |
전용면적 85㎡초과 102㎡이하 |
2015 |
10 |
5721 |
5721.0 |
18879.3 |
85㎡~102㎡~ |
4 |
서울 |
전용면적 102㎡초과 |
2015 |
10 |
5879 |
5879.0 |
19400.7 |
102㎡~ |
... |
... |
... |
... |
... |
... |
... |
... |
... |
4330 |
제주 |
전체 |
2019 |
12 |
3882 |
3882.0 |
12810.6 |
전체 |
4331 |
제주 |
전용면적 60㎡이하 |
2019 |
12 |
NaN |
NaN |
NaN |
60㎡~ |
4332 |
제주 |
전용면적 60㎡초과 85㎡이하 |
2019 |
12 |
3898 |
3898.0 |
12863.4 |
60㎡~85㎡~ |
4333 |
제주 |
전용면적 85㎡초과 102㎡이하 |
2019 |
12 |
NaN |
NaN |
NaN |
85㎡~102㎡~ |
4334 |
제주 |
전용면적 102㎡초과 |
2019 |
12 |
3601 |
3601.0 |
11883.3 |
102㎡~ |
4335 rows × 8 columns
last_df.전용면적.unique()
array(['전체', ' 60㎡이하', ' 60㎡초과 85㎡이하', ' 85㎡초과 102㎡이하', ' 102㎡초과'],
dtype=object)
last_df.columns
Index(['지역명', '규모구분', '연도', '월', '분양가격(㎡)', '분양가격', '평당분양가격', '전용면적'], dtype='object')
last_df.drop(columns=['규모구분','분양가격(㎡)'],inplace=True)
last_df.columns
Index(['지역명', '연도', '월', '분양가격', '평당분양가격', '전용면적'], dtype='object')
last_df.groupby(['지역명'])['평당분양가격'].mean()
지역명
강원 7890.750000
경기 13356.895200
경남 9268.778138
경북 8376.536515
광주 9951.535821
대구 11980.895455
대전 10253.333333
부산 12087.121200
서울 23599.976400
세종 9796.516456
울산 10014.902013
인천 11915.320732
전남 7565.316532
전북 7724.235484
제주 11241.276712
충남 8233.651883
충북 7634.655600
Name: 평당분양가격, dtype: float64
last_df.groupby(['지역명'])['평당분양가격'].mean().plot(kind='bar')
<AxesSubplot:xlabel='지역명'>
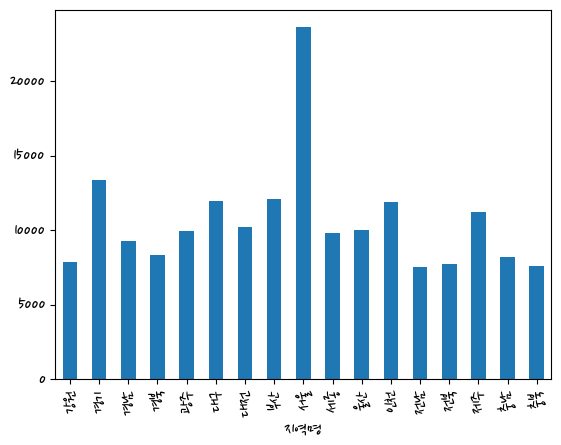
last_df.groupby(['지역명'])['평당분양가격'].mean().plot(kind='barh')
<AxesSubplot:ylabel='지역명'>
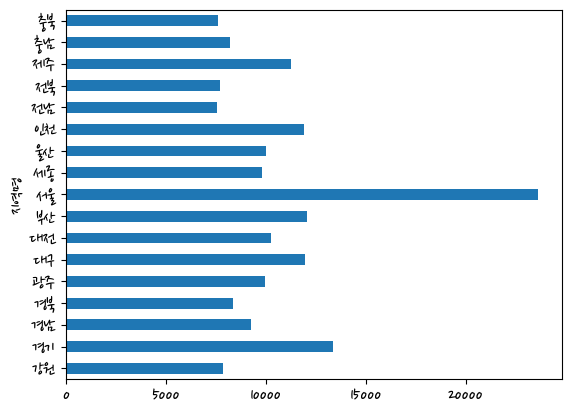
data=last_df.groupby(['지역명'])['평당분양가격'].mean().reset_index()
data.round(-1)
|
지역명 |
평당분양가격 |
0 |
강원 |
7890.0 |
1 |
경기 |
13360.0 |
2 |
경남 |
9270.0 |
3 |
경북 |
8380.0 |
4 |
광주 |
9950.0 |
5 |
대구 |
11980.0 |
6 |
대전 |
10250.0 |
7 |
부산 |
12090.0 |
8 |
서울 |
23600.0 |
9 |
세종 |
9800.0 |
10 |
울산 |
10010.0 |
11 |
인천 |
11920.0 |
12 |
전남 |
7570.0 |
13 |
전북 |
7720.0 |
14 |
제주 |
11240.0 |
15 |
충남 |
8230.0 |
16 |
충북 |
7630.0 |
pd.pivot_table(last_df,index=['전용면적','지역명'],values=['평당분양가격']).reset_index().round()
|
전용면적 |
지역명 |
평당분양가격 |
0 |
102㎡~ |
강원 |
8311.0 |
1 |
102㎡~ |
경기 |
14772.0 |
2 |
102㎡~ |
경남 |
10358.0 |
3 |
102㎡~ |
경북 |
9157.0 |
4 |
102㎡~ |
광주 |
11042.0 |
... |
... |
... |
... |
80 |
전체 |
전남 |
7284.0 |
81 |
전체 |
전북 |
7293.0 |
82 |
전체 |
제주 |
10785.0 |
83 |
전체 |
충남 |
7815.0 |
84 |
전체 |
충북 |
7219.0 |
85 rows × 3 columns
data = last_df.groupby(['지역명'])['평당분양가격'].mean()
data.sort_values(ascending=False).plot(kind='barh')
<AxesSubplot:xlabel='전용면적'>
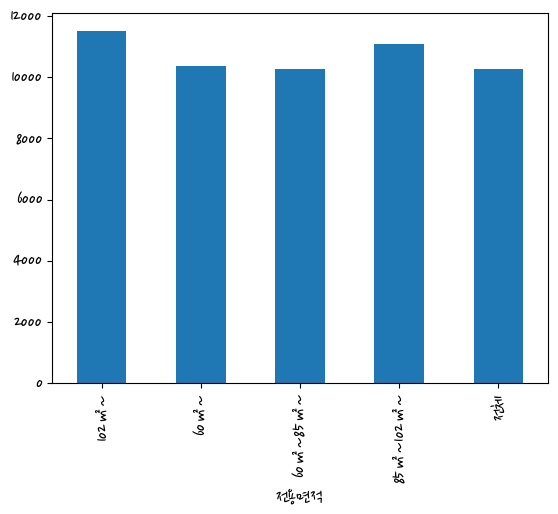
last_df.groupby(['전용면적'])['평당분양가격'].mean().sort_index().plot(kind='bar')
<AxesSubplot:xlabel='전용면적'>
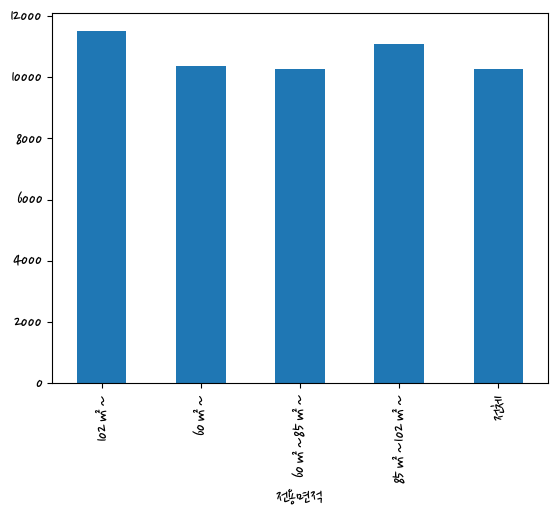
data = last_df.groupby(['연도'])['평당분양가격'].mean().plot(kind='bar')
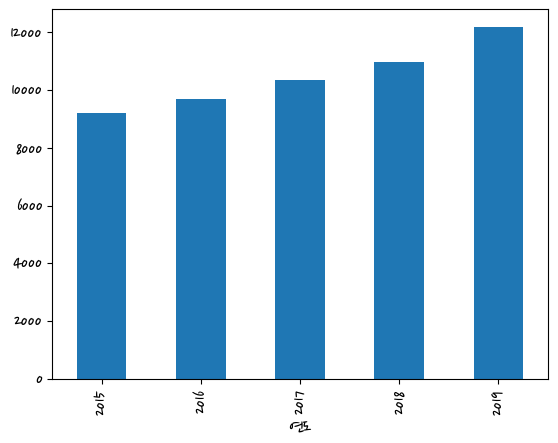
data = last_df.groupby(['연도'])['평당분양가격'].mean()
data
연도
2015 9202.735802
2016 9683.025000
2017 10360.487653
2018 10978.938411
2019 12188.293092
Name: 평당분양가격, dtype: float64
data = last_df.pivot_table(index='월',columns='연도',values='평당분양가격')
data.plot(kind='box')
<AxesSubplot:>
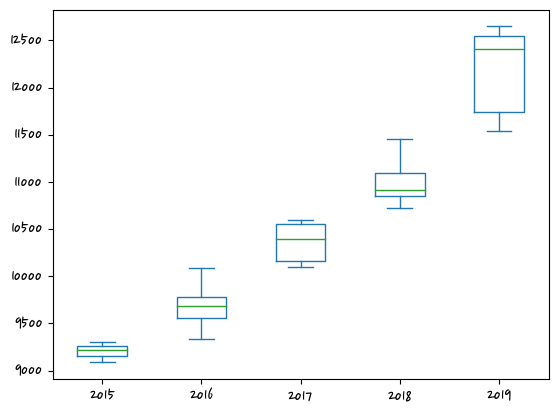
import seaborn as sns
sns.boxplot(data=last_df,x='연도',y='평당분양가격',hue='전용면적')
<AxesSubplot:xlabel='연도', ylabel='평당분양가격'>
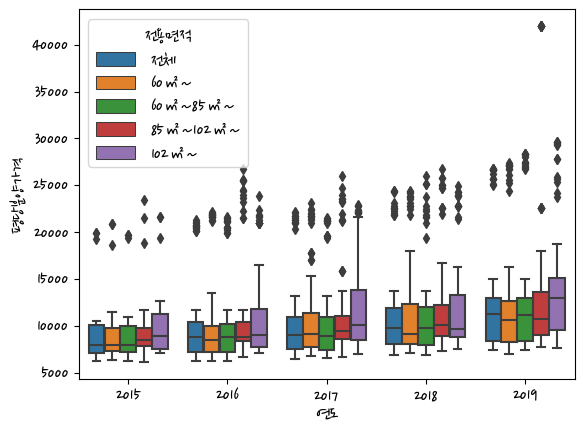
sns.violinplot(data=last_df,x='연도',y='평당분양가격')
<AxesSubplot:xlabel='연도', ylabel='평당분양가격'>
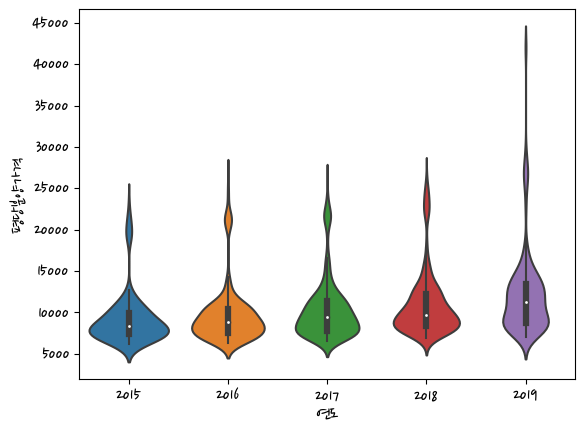
first_df
|
지역 |
2013년12월 |
2014년1월 |
2014년2월 |
2014년3월 |
2014년4월 |
2014년5월 |
2014년6월 |
2014년7월 |
2014년8월 |
... |
2014년11월 |
2014년12월 |
2015년1월 |
2015년2월 |
2015년3월 |
2015년4월 |
2015년5월 |
2015년6월 |
2015년7월 |
2015년8월 |
0 |
서울 |
18189 |
17925 |
17925 |
18016 |
18098 |
19446 |
18867 |
18742 |
19274 |
... |
20242 |
20269 |
20670 |
20670 |
19415 |
18842 |
18367 |
18374 |
18152 |
18443 |
1 |
부산 |
8111 |
8111 |
9078 |
8965 |
9402 |
9501 |
9453 |
9457 |
9411 |
... |
9208 |
9208 |
9204 |
9235 |
9279 |
9327 |
9345 |
9515 |
9559 |
9581 |
2 |
대구 |
8080 |
8080 |
8077 |
8101 |
8267 |
8274 |
8360 |
8360 |
8370 |
... |
8439 |
8253 |
8327 |
8416 |
8441 |
8446 |
8568 |
8542 |
8542 |
8795 |
3 |
인천 |
10204 |
10204 |
10408 |
10408 |
10000 |
9844 |
10058 |
9974 |
9973 |
... |
10020 |
10020 |
10017 |
9876 |
9876 |
9938 |
10551 |
10443 |
10443 |
10449 |
4 |
광주 |
6098 |
7326 |
7611 |
7346 |
7346 |
7523 |
7659 |
7612 |
7622 |
... |
7752 |
7748 |
7752 |
7756 |
7861 |
7914 |
7877 |
7881 |
8089 |
8231 |
5 |
대전 |
8321 |
8321 |
8321 |
8341 |
8341 |
8341 |
8333 |
8333 |
8333 |
... |
8067 |
8067 |
8067 |
8067 |
8067 |
8145 |
8272 |
8079 |
8079 |
8079 |
6 |
울산 |
8090 |
8090 |
8090 |
8153 |
8153 |
8153 |
8153 |
8153 |
8493 |
... |
8891 |
8891 |
8526 |
8526 |
8629 |
9380 |
9192 |
9190 |
9190 |
9215 |
7 |
경기 |
10855 |
10855 |
10791 |
10784 |
10876 |
10646 |
10266 |
10124 |
10134 |
... |
10356 |
10379 |
10391 |
10355 |
10469 |
10684 |
10685 |
10573 |
10518 |
10573 |
8 |
세종 |
7601 |
7600 |
7532 |
7814 |
7908 |
7934 |
8067 |
8067 |
8141 |
... |
8592 |
8560 |
8560 |
8560 |
8555 |
8546 |
8546 |
8671 |
8669 |
8695 |
9 |
강원 |
6230 |
6230 |
6230 |
6141 |
6373 |
6350 |
6350 |
6268 |
6268 |
... |
6365 |
6365 |
6348 |
6350 |
6182 |
6924 |
6846 |
6986 |
7019 |
7008 |
10 |
충북 |
6589 |
6589 |
6611 |
6625 |
6678 |
6598 |
6587 |
6586 |
6586 |
... |
6724 |
6743 |
6749 |
6747 |
6783 |
6790 |
6805 |
6682 |
6601 |
6603 |
11 |
충남 |
6365 |
6365 |
6379 |
6287 |
6552 |
6591 |
6644 |
6805 |
6914 |
... |
6940 |
6989 |
6976 |
6980 |
7161 |
7017 |
6975 |
6939 |
6935 |
6942 |
12 |
전북 |
6282 |
6281 |
5946 |
5966 |
6277 |
6306 |
6351 |
6319 |
6436 |
... |
6583 |
6583 |
6583 |
6583 |
6542 |
6551 |
6556 |
6601 |
6750 |
6580 |
13 |
전남 |
5678 |
5678 |
5678 |
5696 |
5736 |
5656 |
5609 |
5780 |
5685 |
... |
5768 |
5784 |
5784 |
5833 |
5825 |
5940 |
6050 |
6243 |
6286 |
6289 |
14 |
경북 |
6168 |
6168 |
6234 |
6317 |
6412 |
6409 |
6554 |
6556 |
6563 |
... |
6881 |
6989 |
6992 |
6953 |
6997 |
7006 |
6966 |
6887 |
7035 |
7037 |
15 |
경남 |
6473 |
6485 |
6502 |
6610 |
6599 |
6610 |
6615 |
6613 |
6606 |
... |
7125 |
7332 |
7592 |
7588 |
7668 |
7683 |
7717 |
7715 |
7723 |
7665 |
16 |
제주 |
7674 |
7900 |
7900 |
7900 |
7900 |
7900 |
7914 |
7914 |
7914 |
... |
7724 |
7739 |
7739 |
7739 |
7826 |
7285 |
7285 |
7343 |
7343 |
7343 |
17 rows × 22 columns
first_df_melt = first_df.melt(id_vars='지역',var_name='기간', value_name='평당분양가격')
first_df_melt.head(2)
|
지역 |
기간 |
평당분양가격 |
0 |
서울 |
2013년12월 |
18189 |
1 |
부산 |
2013년12월 |
8111 |
last_df.columns
Index(['지역명', '연도', '월', '분양가격', '평당분양가격', '전용면적'], dtype='object')
last_df.info()
<class 'pandas.core.frame.DataFrame'>
RangeIndex: 4335 entries, 0 to 4334
Data columns (total 6 columns):
# Column Non-Null Count Dtype
--- ------ -------------- -----
0 지역명 4335 non-null object
1 연도 4335 non-null int64
2 월 4335 non-null int64
3 분양가격 3957 non-null float64
4 평당분양가격 3957 non-null float64
5 전용면적 4335 non-null object
dtypes: float64(2), int64(2), object(2)
memory usage: 203.3+ KB
first_df_melt.columns
Index(['지역', '기간', '평당분양가격'], dtype='object')
first_df_melt['연도'] = first_df_melt['기간'].str.split('년').str.get(0).astype('int')
first_df_melt['월'] = first_df_melt['기간'].str.split('년').str.get(1).str.replace('월','').astype('int')
first_df_melt.columns = ['지역명', '기간', '평당분양가격','연도','월']
cols = ['지역명', '연도', '월', '평당분양가격']
data_last =last_df.loc[last_df['전용면적']=='전체',cols]
data_first = first_df_melt[cols]
result=pd.concat([data_first,data_last])
result
|
지역명 |
연도 |
월 |
평당분양가격 |
0 |
서울 |
2013 |
12 |
18189.0 |
1 |
부산 |
2013 |
12 |
8111.0 |
2 |
대구 |
2013 |
12 |
8080.0 |
3 |
인천 |
2013 |
12 |
10204.0 |
4 |
광주 |
2013 |
12 |
6098.0 |
... |
... |
... |
... |
... |
4310 |
전북 |
2019 |
12 |
8144.4 |
4315 |
전남 |
2019 |
12 |
8091.6 |
4320 |
경북 |
2019 |
12 |
9616.2 |
4325 |
경남 |
2019 |
12 |
10107.9 |
4330 |
제주 |
2019 |
12 |
12810.6 |
1224 rows × 4 columns