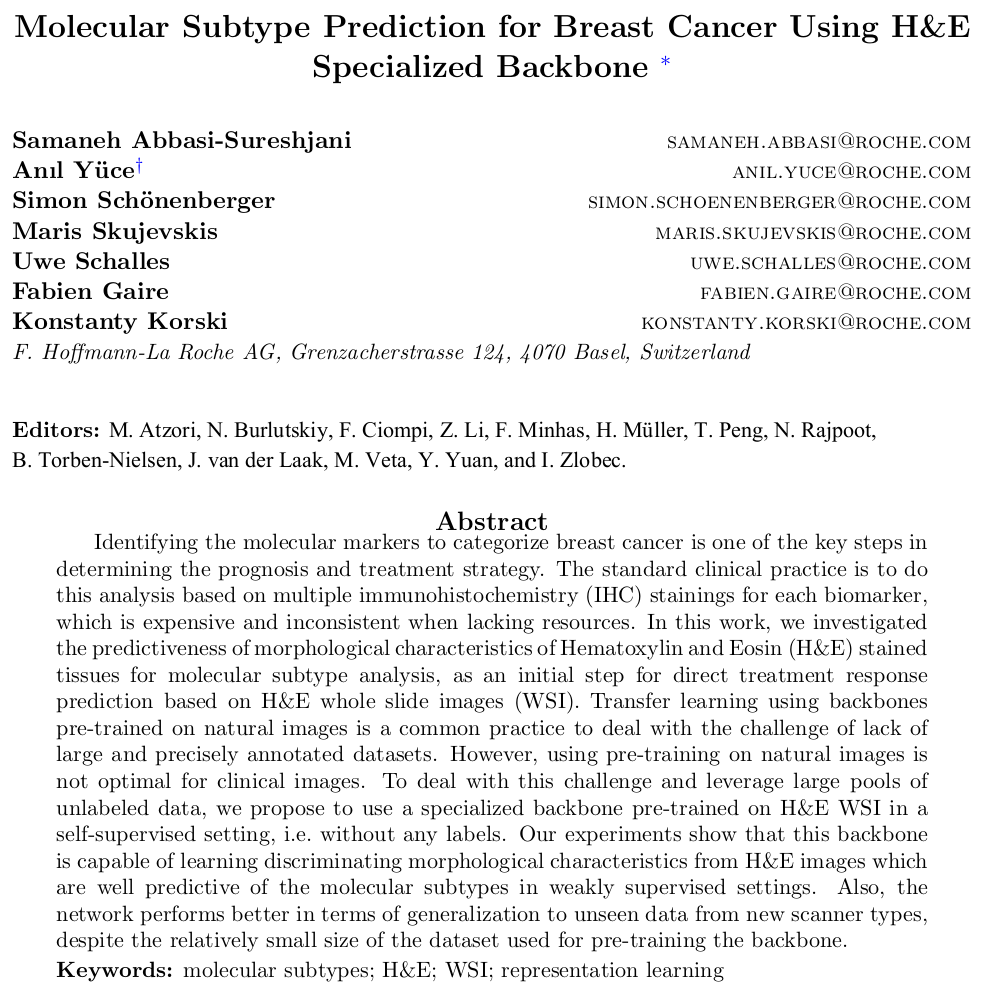
Molecular Subtype Prediction for Breast Cancer Using H&E Specialized Backbone
Attention-based aggregation, weakly supervised learning with self-supervised learning과 관련된 내용을 담고 있다.
Introduction
Breast cancer의 molecular marker를 통해 subtype을 파악하는 것은 prognosis와 treatment를 위한 첫 단계이다. 이를 수행하기 위한 immunohistochemistry (IHC) staining 방식은 비용이 크기에, H&E stained whole slide image (WSI) 만으로 breast cancer의 subtype을 예측하는 연구가 발전되어 왔다.
Annotation이 부족하고 크기가 크다는 데이터 특성으로, natural image를 통해 모델을 pre-training하는 방식이 사용되어 왔으나 optimal하지 않았다. 그렇기에 label이 없는 H&E WSI를 self-supervised setting으로 pre-training하여 feature extraction을 진행하는 방식이 고안되었다.
또한 본 논문의 저자는 natural image와 WSI로 pre-train한 모델을 unseen data에서 test하였고 self-supervised learning setting에서 generalization 측면에서 더 좋은 성능을 보인다는 것을 발견하였다.
Method
Representation learning
Large unlabeled set으로부터 visual feature를 뽑아내기 위해 self-supervised learning, 그 중에서도 BYOL (Beyond your own latent) 모델을 적용하였다.
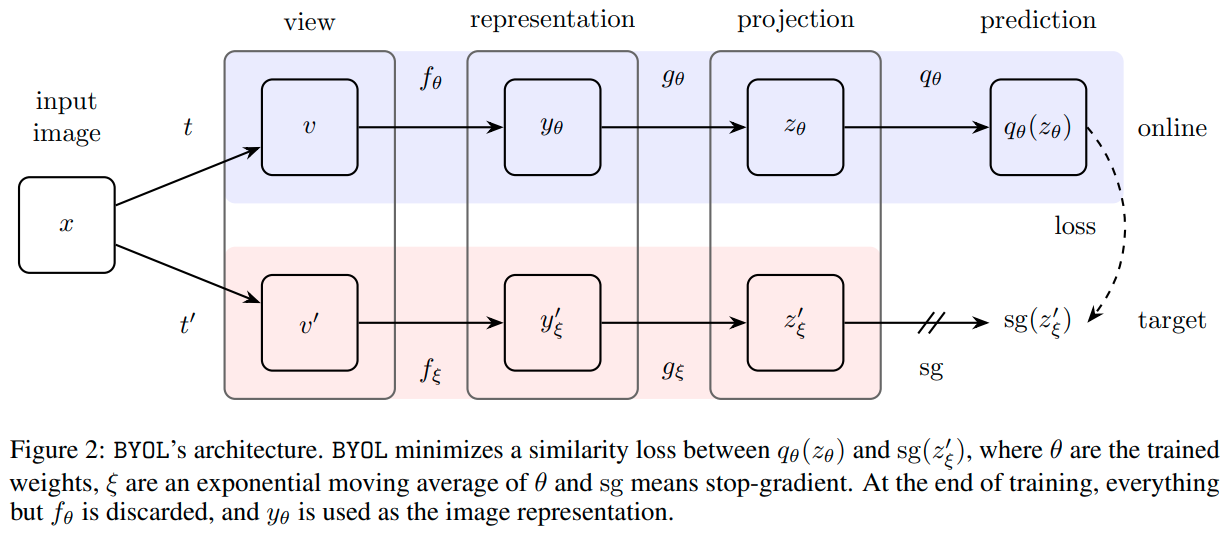
BYOL 모델은 기존 SSL의 contrastive learning 방식과 달리 negative pair를 사용하지 않고, online과 target 두가지의 network를 사용한다. 같은 input image에 대해 서로 다른 t, t′ augmentation을 거친 prediction 결과 qθ(zθ)와 sg(zξ′) 사이의 loss로 back propagation을 통해 online network를 학습한다. 또한 target network도 online network를 통해 학습하긴 하지만 back propagation이 아닌 slow-moving average 방식으로 학습한다. 이는 trivial한 solution으로 모델이 collapse하는 것을 막기 위함이다. 자세한 내용은 BYOL 논문을 참고하도록 하자.
BYOL training에 쓰인 데이터는 다음과 같다.
- TCGA-BRCA, TCGA-LUAD, TCGA-THCA, TCGA-DLBC
- three private cohorts provided by commercial vendors
- total 1045378 tiles are used, 10% is selected as validation set
Molecular subtyping using an attention-based MIL framework
H&E image로부터 feature extraction 후에 attention-based deep MIL framework를 적용하여 slide-level의 final score를 계산하였다. Embedding은 다음과 같은 두가지 방식으로 생성되었다.
- pre-trained on H&E images with BYOL
- pre-trained on ImageNet dataset
이후 bag classifier에게 세 가지 종류의 classification을 수행하도록 하였다. non-TNBC sample 사이에서 confusion이 있었기에, 따로 separate한 experiment 2가 설계됨.
- Two-class classification [Her2+, ER−] vs. [Her2+, ER+]
- Three-class classification [Her2−, ER+] vs. [Her2−, ER−, PR−] vs. [Her2+, ER+] and [Her2+, ER−] combined
- Four-class classification [Her2−, ER+] vs. [Her2+, ER−] vs. [Her2+, ER+] vs. [Her2−, ER−, PR−]
Whole framework training에는 다음과 같은 dataset이 사용되었다.
- TCGA-BRCA, TCGA-LUAD, TCGA-THCA, TCGA-DLBC (different from pre-training)
- TCGA-BRCA (Leica Aperio scanner)
- six private cohorts provided by Commercial Vendors (CVD)
- two Internal Datasets called ID1, ID2
Generalization 성능 측정을 위해, train과 test 과정에서 다른 cohort dataset을 사용하였다.
- Test1 80% slides from each cohort training, 20% for test
- Test2 80% slides from the cohorts TCGa, ID1, ID2 training and 20% for test, while all slides from the CVD are used for testing
다음은 per cohort, per category for each of these settings의 slide number overview이다. Test2는 다양한 스캐너와 staining protocol로 만들어진 independent cohort를 포함하여, real-world와 좀 더 가까운 데이터로 볼 수 있다.
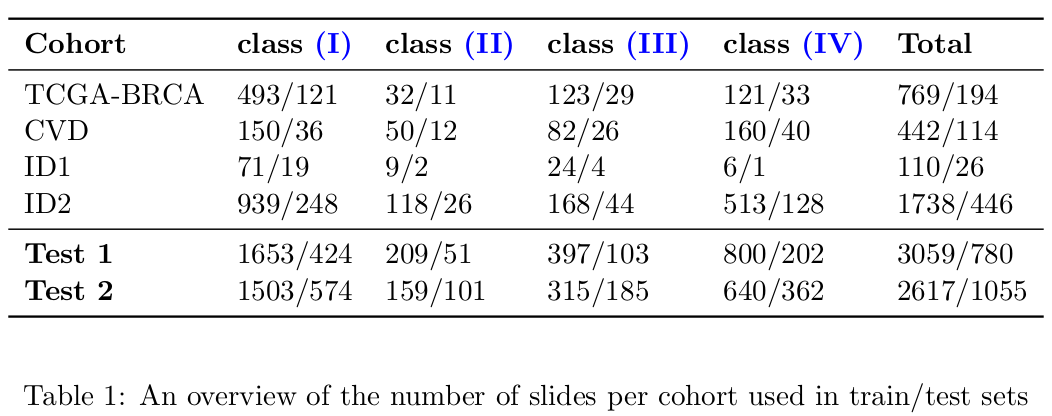
Results and Discussion
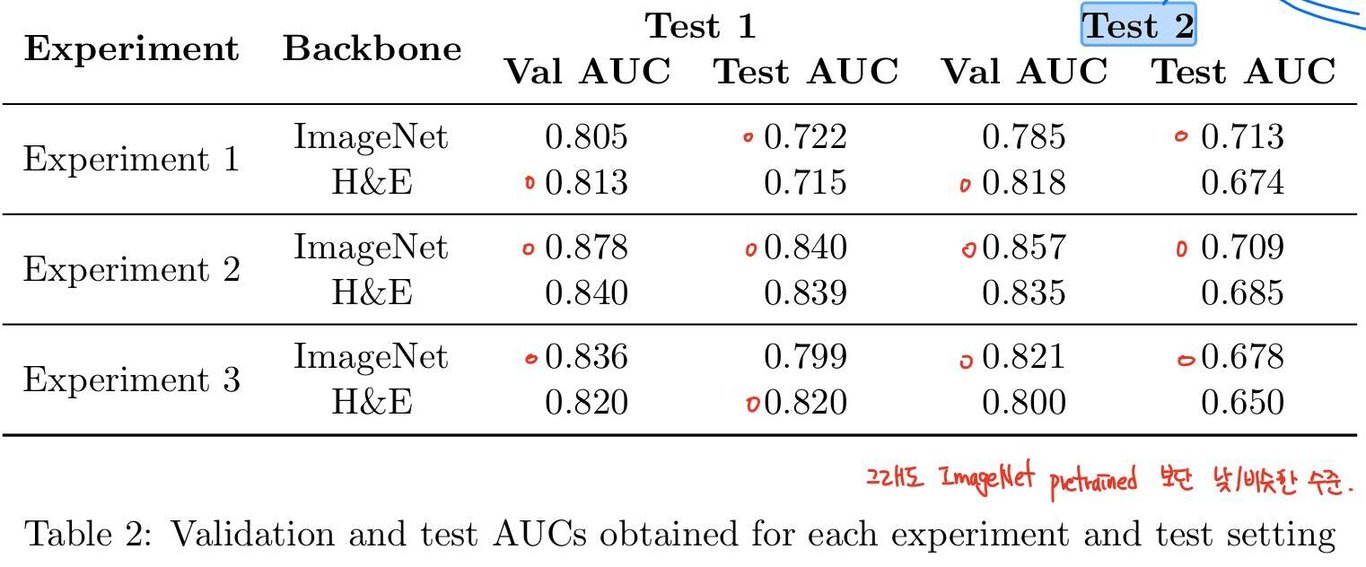
- Exp1보다 Exp2,3이 sample 수가 더 많고 finer categorized 되었기에 성능이 높다 해석한다.
- pretrain-data set의 크기 차이와 unlabeled 특성에도 불구하고 성능이 비슷했기에, this shows the great potential of the self-supervised learning이라 해석함.
- non-TNBC 사이의 confusion이 있어 이를 분리한 experiment 2가 설계되었고, experiment 3의 결과가 experment 2보다 잘 나오지 않은 결과로 confusion이 재확인됨.
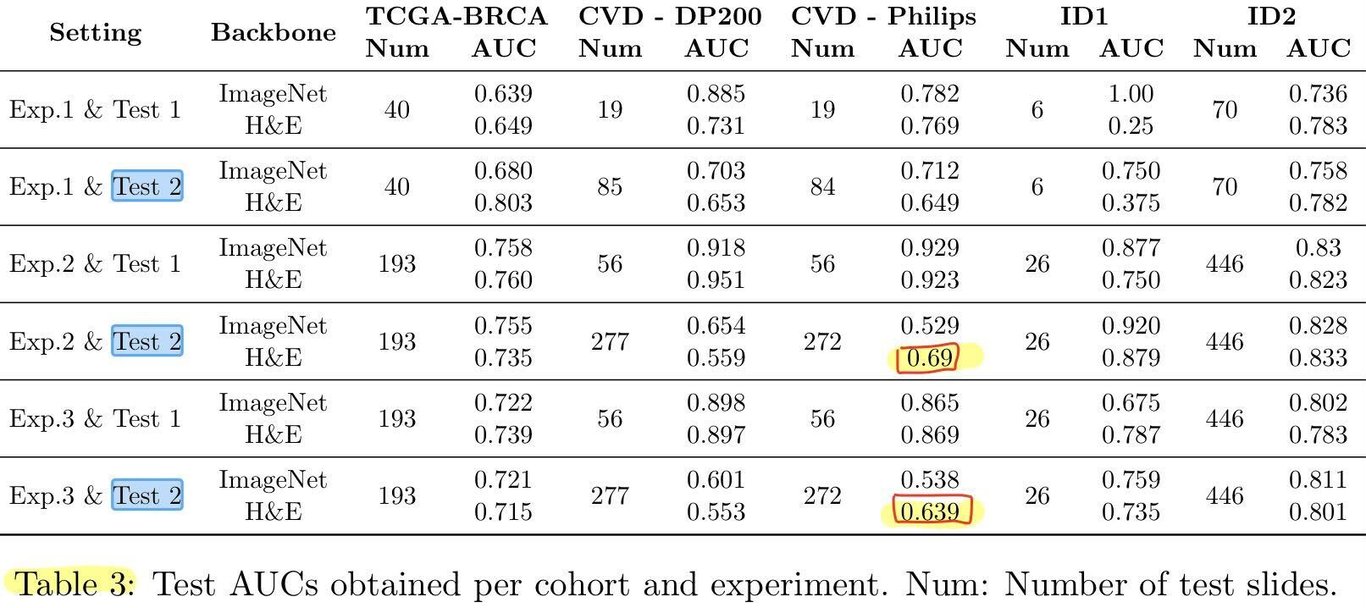
- Generalization to new unseen data 성능을 더 상세히 분석하기 위해, cohort를 분리하여 AUC를 계산함.
- highlight 된 값을 토대로, generalize better to an unseen scanner type이라 해석함.
Conclusion
- attention-based aggregation: attention을 활용한 deep MIL framework를 활용함.
- weakly supervised learning with self-supervised learning: BYOL 모델을 적용하여 large, unannotated H&E WSI (patient-level annotated) 으로 embedding backbone을 pre-train함.
Attention을 활용한 tile aggregation framework를 사용하였다. Attention 자체에 대해 겁을 먹고 있었는데 조금씩 익숙해지면서 논문에 등장해도 담담히 받아들이게 되었다. (따로 attention 글 쓰진 않을 것 같다는 뜻) Unannotated dataset으로 contrastive learning 시킨 backbone 활용해도 AUC가 ImageNet의 그것과 비슷하게 나오는 것을 확인했고, generalization 측면에서의 성능도 확인하였다. 하지만 성능을 확신하기엔 다른 연구들이 필요하지 않을까 하는 생각이 들었다. 그리고 역시.. SSL은 데이터가 많이 필요한 듯 싶다.
궁금했던 점
- Experiment 2, 3의 나눠서 진행한 것의 차이를 잘 모르겠다.. 논문에 따르면 어짜피 나중에 Experiment 2의 4C3 개 결과를 다시 안에서 평균을 내었다고 하는데, non-TNBC를 분리한 것과 왜 차이가 있는 건지 잘 모르겠다.
- BYOL 모델이 RL 모델과 참 닮은 것 같다.
참고:
https://arxiv.org/abs/2006.07733