1. Introduction
- Interection이 오직 'click'으로 구성되는 것이 아닌 user과 관계있는 item들의 특징을 반영하여 내재된 정보를 파악한다.
- Knowledge Graph를 사용함으로써 추천에 대한 이유를 설명하고, Cold start 문제를 해결한다.
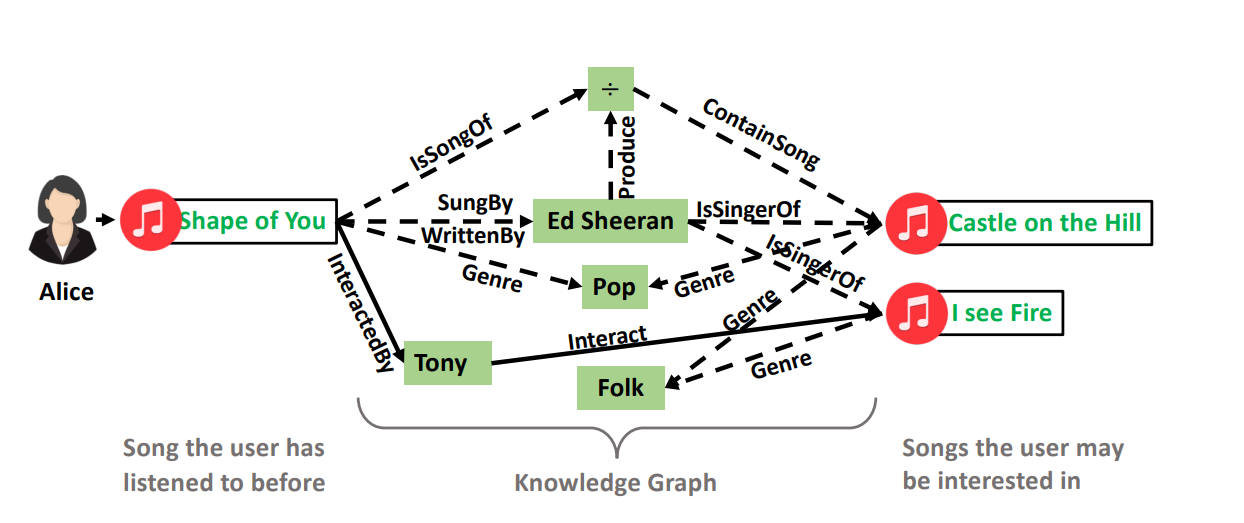
- 위의 그림에서 User는 'Alice'이고 이 유저가 'Castle on the Hill' 이라는 곡을 추천 받았을 때, 다음과 같은 경로를 볼 수 있다.
- (Alice, Interact, Shape of You) -> (Shape of You, IsSongOf, divide(÷)) -> (divide(÷), ContainSong, Castle on the Hill) ==> 수록곡을 통한 Path
- (Alice, Interact, Shape of You) -> (Shape of You, SungBy, Ed Sheeran) -> (Ed Sheeran, IsSingerOf, Castle on the Hill) ==> 가수(Ed Sheeran)를 통한 Path
- (Alice, Interact, Shape of You) -> (Shape of You, Interacted By, Tony) -> (Tony, Interact, Castle on the Hill) ==> 유저를 통한 Path
- 이러한 연결들은 경로에서 정보를 종합하여 보이지 않는 user-item interaction을 추론한다.
KPRN
- 본 논문의 저자들은 모델 이름을 KPRN(Knowledge-aware Path Recurrent Network'라고 지었고, 지식그래프의 path에 RNN 모델을 적용하였다.
- 기존 아이템 간의 관계형 데이터베이스 정보에 User-Item interaction 정보까지 고려하여 Knowledge Graph를 형성하고, 이는 정형데이터 관계와 Sequence 관계를 모두 포함하게 된다.
- 또한, 사용자에게 특정 아이템을 추천할 때, 다양한 관계 중 어떤 것에 중점을 두는지 학습하기 위하여 Weight Pooling을 사용한다.
- 위의 경우에서 가수(Sung by)때문에 Castle on the Hill을 듣는 다는 것에 대한 설명이 가능하도록, Sungby의 관계에 높은 가중치를 두고 학습한다.
2. Modeling
Embedding Layer
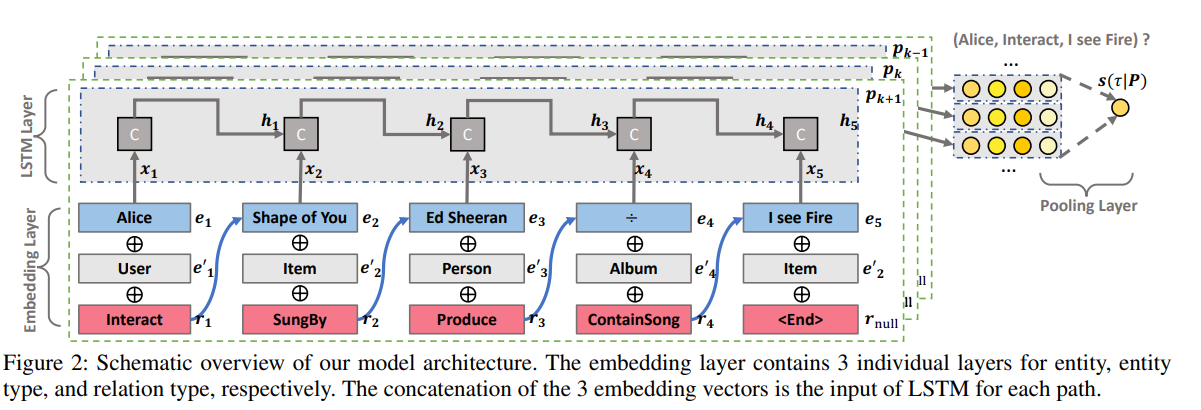
- Embedding을 할 때는, 동일한 entity-entity 쌍이 다른 의미(relation)을 가질 수 있고, 이러한 경우 다른 경로로써 포함한다.
LSTM Layer
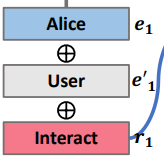
- Input : x (entity, entity type, relation type)
- LSTM(Long Short Term Memory) : 장/단기 기억이 가능하게 설계 된 신경망 구조이며 1과 0으로 나타나는 Label을 기준으로 학습한다.
- Pos Path와 Neg Path를 학습하며 Neg Path는 label이 0인 즉 상호작용이 없는 Path이다.
- LSTM에 대한 설명 참고 : http://www.incodom.kr/LSTM
Weighted Pooling Layer
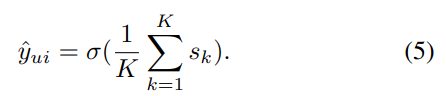



- (5)의 식은 path score들의 평균을 sigmoid 함수에 넣어준 값이 된다. 즉, path가 세개이고, 각각의 score가 [0.3, 0.6, 0.9]일 때, sigmoid(0.6)으로 약 0.64의 값이다.
- (6)의 식에서는 path score을 exp(sk/γ) 로 사용하면, γ(가중치)가 작은 수 일수록 결과값이 커진다. 여기서 y^ui값이 크다는 의미는 score가 강한 path가 더 큰 영향력을 가진다는 max pooling을 의미한다.
- (6)의 식에서 γ가 큰 수 일수록 y^ui값이 작아지며 avg pooling에 가까워진다.
3. Experiments
3-1. DataSet
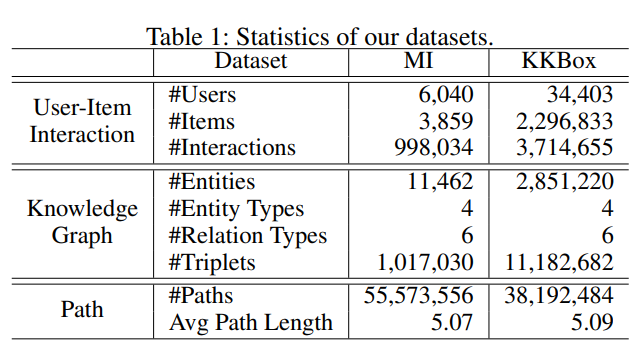
- IMDb의 knowledge graph data + MovieLens의 User-Item Interection인 MI(영화) 데이터와 음악 데이터 KKBox를 이용한다.
- noisy해지는 것을 방지하여 Path의 length는 최대 6으로 설정되었고, First Node가 User이고 Last Node가 Item인 Path들을 추출하여 사용한다.
3-2. Learning
- Positive pair를 설정한 후 Pos Path를 추출한다.
- Interaction이 없는 pair를 4개 설정한 후 Neg Path를 추출한다.
- 1과 2로 나온 데이터를 80%는 train data로, 20%는 test 데이터로 사용하여 학습한다. 이때의 목적함수는 다음과 같다.
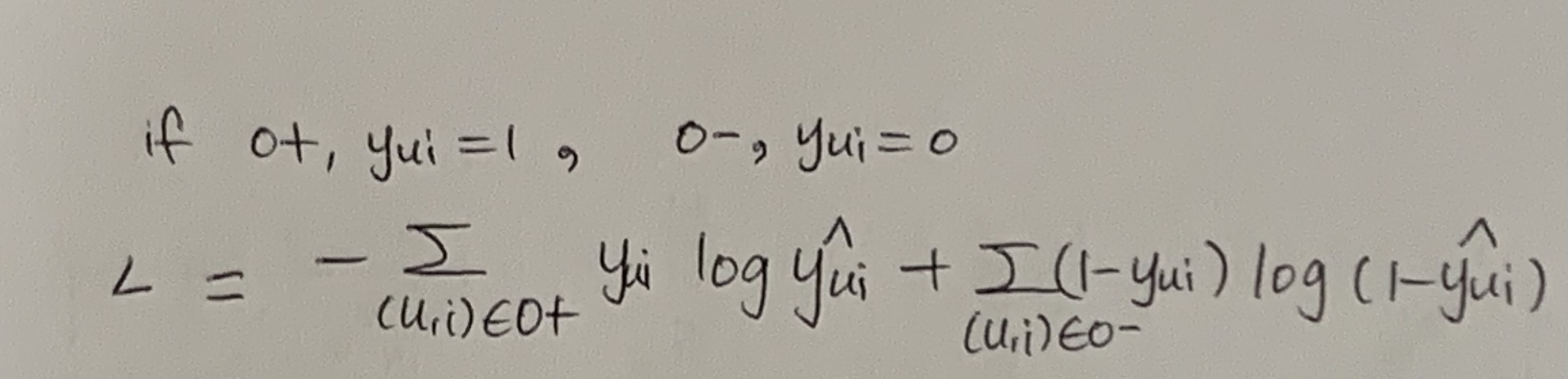

- Test는 1개의 test set pair과 random 한 100개의 쌍을 사용하고, 이 101개의 score로 평가를 한다. 이때, 평가지표는 Hit ratio@K, ndcg@K이다.
RQ1
Compared with the state-of-the-art KG-enhanced methods, how does our method perform?
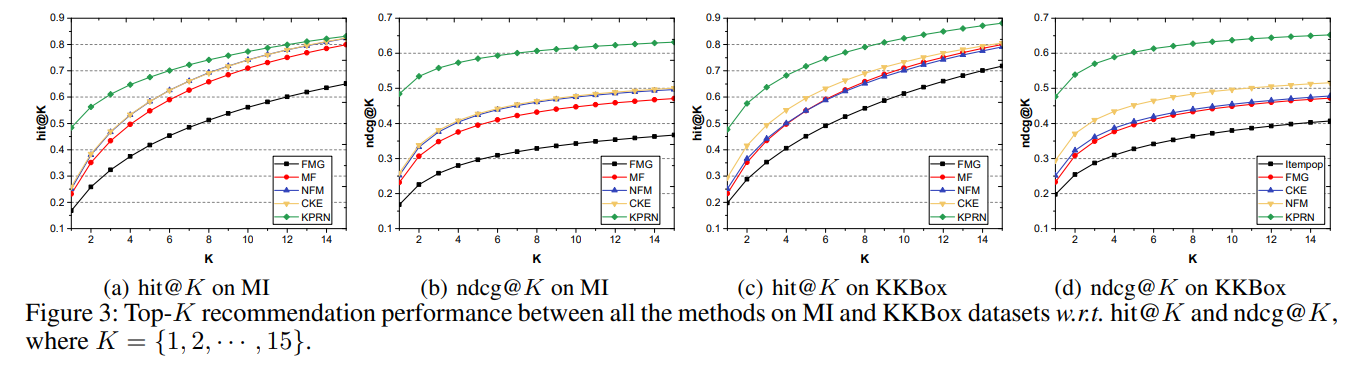
- MI와 KKBox 데이터에서 모두 가장 좋은 성능을 내는 것을 볼 수 있다.
RQ2
How does the multi-step path modeling (e.g., the incorporation of both entity and relation types) affect KPRN?

-
KPRN-r은 KPRN에서 relation을 고려하지 않은 모델이다.
-
MI 데이터 셋에서 relation을 고려하지 않았을 때 성능이 낮아지는 것을 미루어보아 relation을 고려해서 본 모델이 좋은 성능을 낸다고 말할 수 있다.
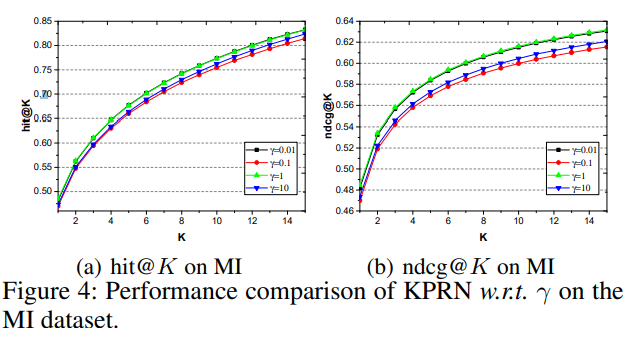
-
하이퍼파라미터가 크게 유의미하다고 보이지 않는다.
RQ3
Can our proposed method reason on paths to infer user preferences towards items?
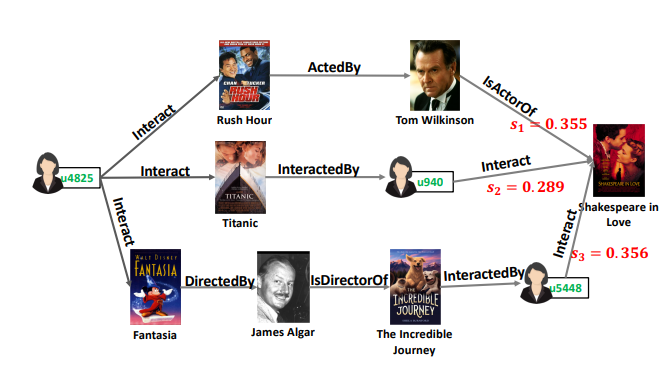
- 이 논문의 가장 큰 Contribution은 Reasoning이다.
- 본 모델은 Knowledge Graph를 따라서 사용자의 관심을 확장할 수 있을 뿐만 아니라 사용자에게 Item이 왜 추천되었는지에 대한 적합한 설명을 제공 가능하다.
참고
http://dsba.korea.ac.kr/seminar/?mod=document&uid=387
유익한 정보를 얻을 수 있어서 기쁩니다.