small-batch methods consistently converge to flat minimizers, and our experiments support a commonly held view that this is due to the
inherent noise in the gradient estimation.
- small batch 는 minima에 수렴하기 쉽고, generalization 성능이 좋음 (= training error와 validation error의 차이가 적음)
- large batch 는 성능이 떨어지는 경향이 있음
- 이러한 이유의 이론적 배경을 설명하는 논문
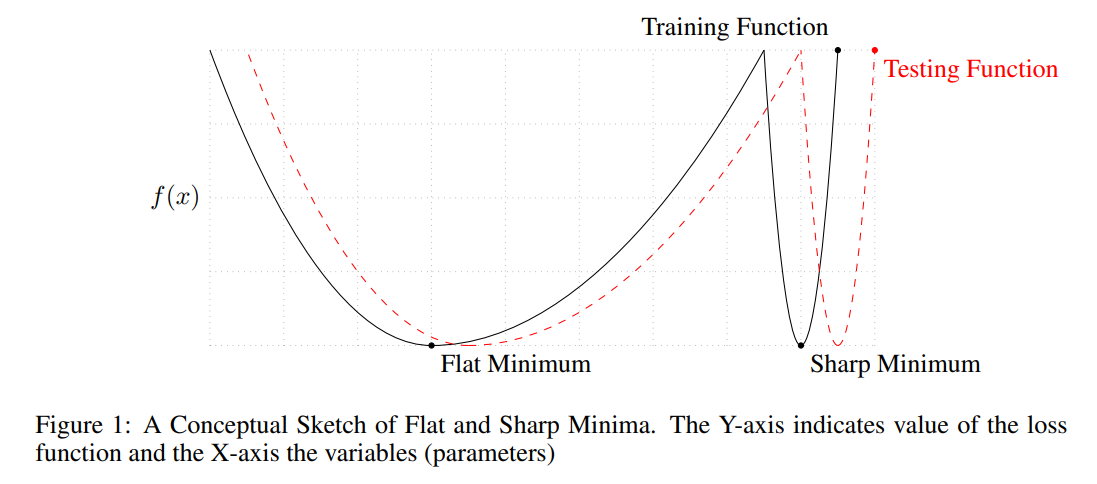
- large batch는 sharp minimum으로 수렴하고 samll batch 는 flat minimum으로 수렴함
- testing function (test loss)또한 sharp 한 형태이기 때문에 testing accuacy와 trainig accuracy의 차이가 발생함
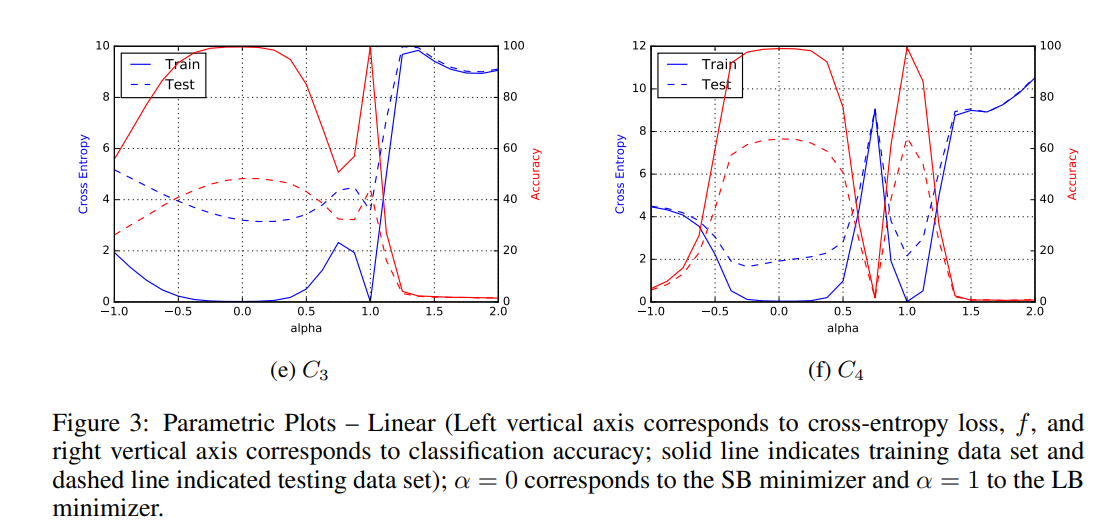
- LB로 이동할수록 minimum 이 sharp 형태임을 알 수 있음
- 결론, LB일수록 test loss, train loss 차이가 큰 optimal에 수렴할 가능성이 높다
질문: LB일수록 전체 데이터셋을 대표하기 때문에 더 generalization 성능이 높아야할것같은데, ?