논문 : Context Embeddings for Efficient Answer Generation in RAG
Background
Problem state
-
Context가 길어지면서 응답 시간이 길어지게 됨
-
이를 해결하기 위한 이전 Compression 연구들은 Compression Model이 너무 크거나, 효율성이 낮거나 Fine-tuning을 하지 않고 사용하는 등의 문제가 존재
Contribution
-
효과적인 compression model (COCOM : COntext, COmpression Model)을 제안
-
Compression에 대한 Memory와 Time 측면에서 분석
-
높은 성능을 유지하면서 Inference time을 최대 5.69배, GFLOPs는 최대 22배 까지 줄임
- GFLOPs가 줄었다는 것은 계산량이 줄었다는 의미
-
Ablastion 실험을 통해 효율적인 generation을 위한 중요한 요소를 분석
Method
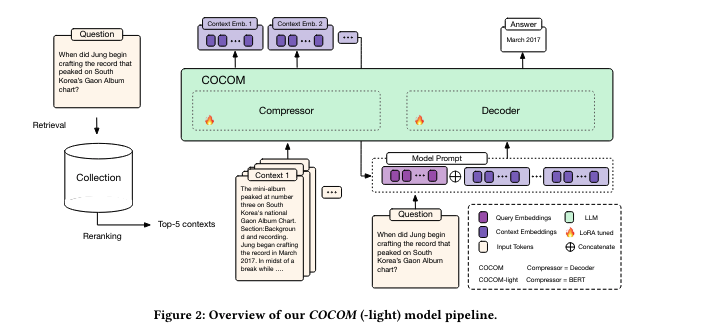
C=t1,t2,...,tnE=e1,e2,...,ekϕcomp:{t1,t2,...,tn}→{e1,e2,...,ek}∈RdC:ContextE:context embeddingk≪nei∈Rdd:LLM’s hidden dimension
- COCOM의 주요 아이디어는 LLM의 input으로 제공되는 context를 작은 context embedding으로 변환하는 것
- context embeddings과 user input을 기반으로 응답을 생성
θLLM:{E,x}→rx:input
ϕcomp=θLLM
-
compression task에서는 input에 spectial token(<AE>)을 prepend하고 ξ에 따라 시퀀스 끝의 다른 수의 embedding tokens (<CTX>)를 append 함
-
last hidden layer의 representations를 context embedding으로 사용하며, Decoder뿐만 아니라 BERT와 같은 encoder-only 모델을 compressor로 사용하여 비교
-
context embedding의 수(k=∣E∣)는 변경할 수 있으며 original context의 compression level을 제어할 수 있으며 본 논문에서는 아래와 같이 계산함
- 예를 들어, context length가 128이고 compression rate가 64라면, context embeddings는 2로 설정할 수 있고 input을 64배 줄임
ξ=⌊ξn⌋
n=∣C∣(length of the tokenized input)
-
Knowledge-intensive task에서 multiple passages 들은 concat되고 모델에 제공됨
-
COCOM도 multiple passages의 context embeddings를 LLM에 제공함
-
Context들은 독립적으로 압축되어지며, context embeddings 사이에 [SEP]을 삽입하여 각각을 구별하여 LLM에게 제공함
Pre-training Context Embeddings
L(θLLM)=−xt∈X∑logPθLLM(xt∣x1,x2,...,xt−1)
- auto-encoding : Context Embedding으로 부터 original input token으로 복원될 수 있도록 하기 위해 compressor와 LLM을 연결하여 학습함. 이 단계는 context embedding을 이용해 QA를 할 수 있도록 하는 최종 objective 전 단계이며 같은 입력에 대한 compress와 decompress를 학습하는데 목적이 있음.
E=ϕcomp(x1,x2,...,xT)L(θLLM,ϕcomp)=−xt∈X∑logPθLLM(xt∣E,x1,x2,...,xt−1)
- Language Modeling from context embeddings : 최종적으로 context embeddings을 기반으로 질문에 응답하기 위한 task를 위해, 아래와 같이 학습하며 이는 주어진 입력에 대한 compress를 학습할 뿐만 아니라 context embedding의 content를 활용하는 방법을 효율적으로 학습함
L(θLLM,ϕcomp)=−xt∈XB∑logPθLLM(xt∣ϕcomp(XA),x1,x2,...,xt−1)X={x1,x2,...,xT}XA={x1,x2,xj}XB={xj+1,...,xT}
- XA를 EA로 압축한 후, 이를 기반으로 XB를 생성하게 되고, 이는 auto-encoding과 상호보완적으로 사용하여 original input을 recovering하는 것과 context embeddings를 통해 content를 활용하는 방법까지 학습
Fine-tuning
- RAG application을 위해, question(q)과 retrieval system에 의해 추출 된 relevant context(s)를 압축한 E을 활용하여 fine-tuning 수행
L(θLLM,ϕcomp)=−rt∈R∑logPθLLM(rt∣IE,q,r1,r2,...,rt−1)IE,q:combined into an instructionR=(r1,r2,...,rT)
Results
-
COCOM : compressor과 generator가 Mistral-7B-Instruct-v0.2로 같음
-
COCOM-light : compressor로 bert-base-uncased 사용
-
compression rates (ξ) : 4, 16, 128
-
SPLADE-v3를 사용하여 top-50 reranking
-
DeBERTa-v3를 사용하여 top-50 retrieval
-
모든 실험은 top-5를 context로 사용
-
chunk size나 hyperparameters는 논문 참조
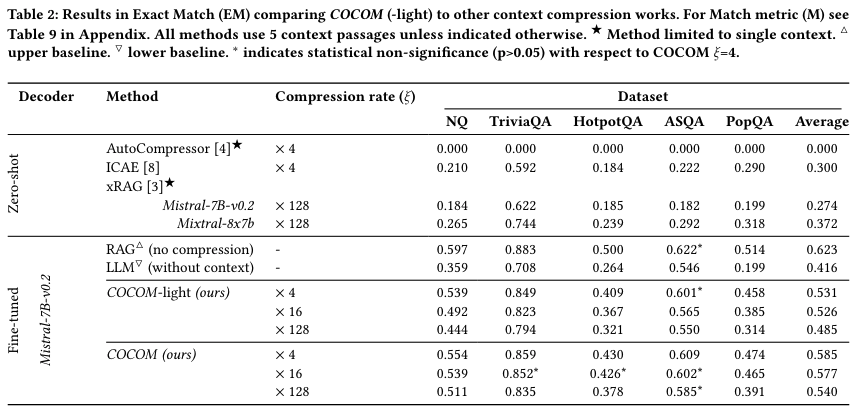
-
RAG, LLM은 각각 context를 전부 준 경우와 아예 안준 경우를 의미하고 upper bound, lower bound로 사용함
-
COCOM은 upper bound와 lower bound에 위치하며 다른 방법론 대비 높은 성능을 보여줌
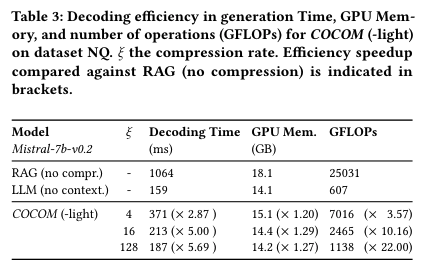
- compression rate가 높을 수록 빠른 속도로 generation 가능
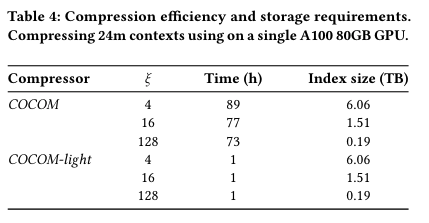
-
COCOM-light는 COCOM 보다 최대 89배 적은 계산을 필요로 함
-
Index 크기는 compression rate와 반대로 움직이는데, 압축률이 높을 수록 인덱스 저장 요구 사항이 줄어들지만 첫 번째 이미지에서 보듯 품질 저하 발생
Conclusion