0. Intro
성적표 주셈.
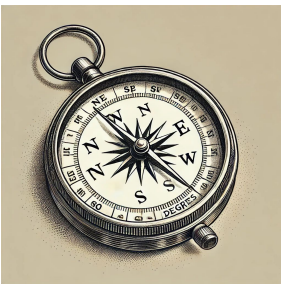
잘하고 있는지 나침반 같은 것.
1. Classification Metric
1.1 Metric
지표(Metric)이란, 모델이 올바르게 학습되고 있는지 그 수치를 정량적으로 나타낸것.
1.2 Classification Metric
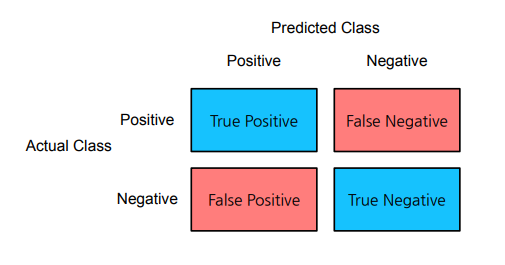
- Precision=TP+FPTP : 예측한 것들 중에서 얼마나 잘 맞추었냐
- Recall=TP+FNTP : 실제 true것들 중에서 얼마나 true 인지
- F1score=2∗Precision+RecallPrecision∗Recall
🤔 왜 굳이 metric을 구별해야 하는 걸까?
👉 무엇을 더 중요하게 생각하는지에 따라 비용이 달라질 수 있기 때문에.
1.3 Trade-off Single Model
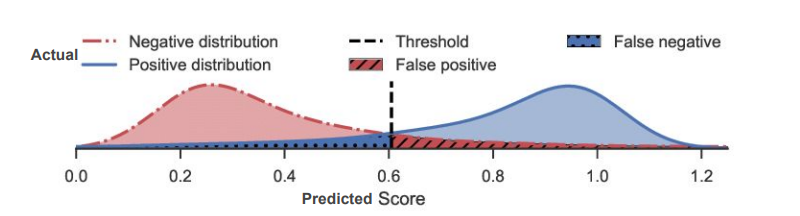
Threshold 위체에 따라 Precision, Recall이 반대로 변한다.서로 trade-off함
- 따라서 우리는 비용에 따라 Threshold를 통해 Precision , Recall 을 조정해 볼 수 있다.
1.4 Multi-Class 문제
Macro vs Weighted vs Micro
Macro
- 각 클래스별 Metric을 구해 동일한 가중치로 계산
- 모든 클래스를 "균형있게"평가
Weighted
- Macro metric을 구할 때 클래스별 샘플 수에 비례한 가중치로 평균
Micro
- 모든 클래스의 TP,FP,FN을 합산해 metric을 구하고 이에 대해 Metric을 계산
1.5 Top-k Accuracty
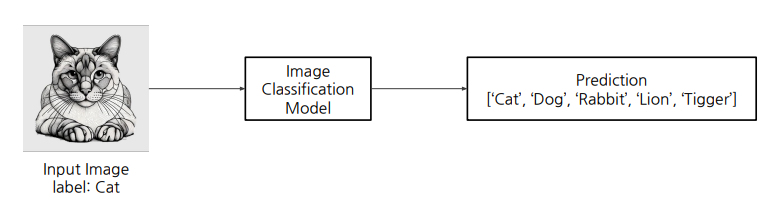
위의 이미지처럼 그럴 듯한 정답이 여러개 있으면, N개 까지의 모두 출력.
2. Ensemble
2.1 Ensemble
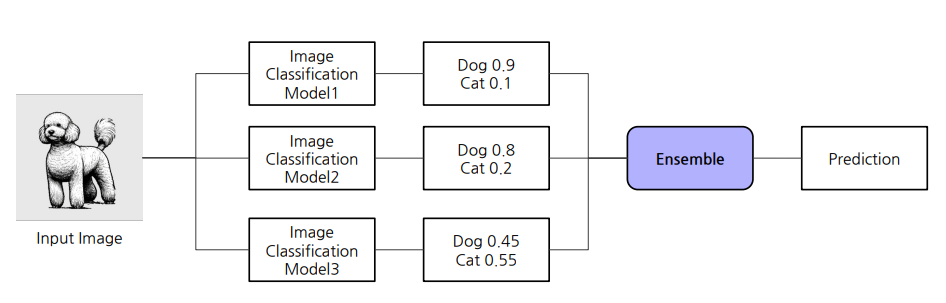
동일한 태스크의 여러가지 모델의 결과를 혼합하여 성능을 높인다.
2.2 Voting
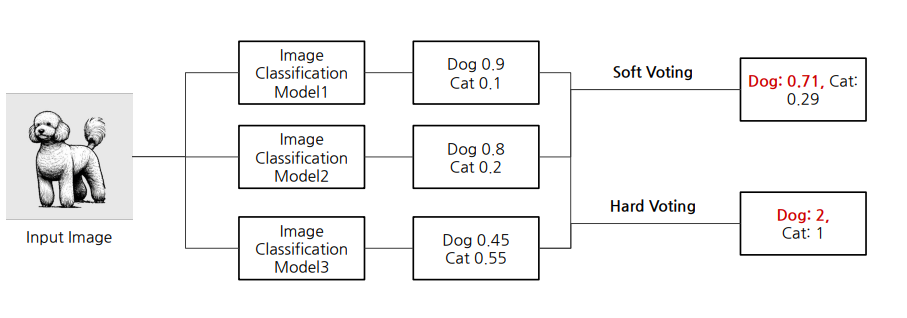
Soft Voting : 확률값을 그래도 평균해서 값 제출
Hard Voting : 각 모델의 결과를 0과 1로 치환해서 투표값 합쳐서 산출.
2.3 Weighted Ensemble
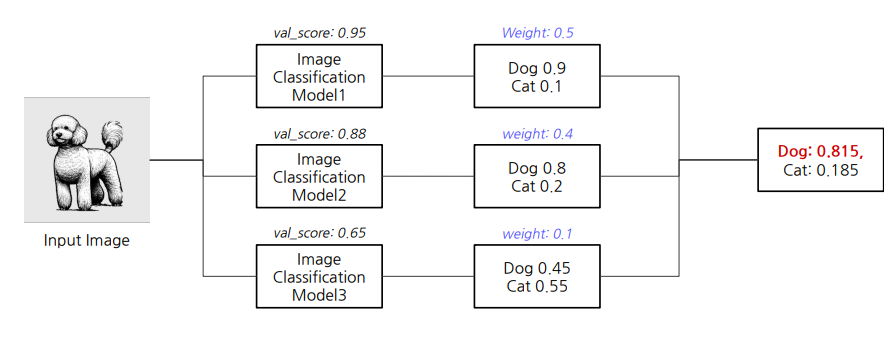
성능에 좋은 모델에 더 가중치를 두어서 앙상블.
2.4 Cross-Validation
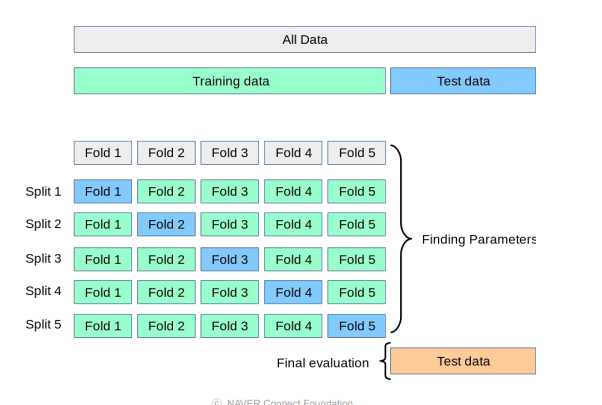
훈련데이터를 N개로 나누어서 N-1개는 train 시키고 1개는 validate하는것.
2.5 Test-Time Augmentation(TTA)
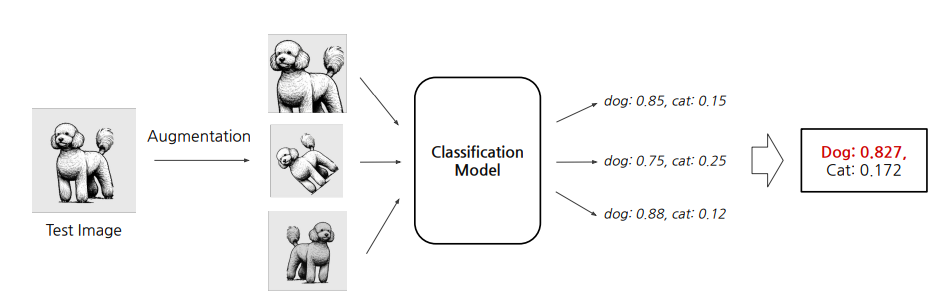
테스트 데이터의 이미지를 N번 Augmentation후 그 N개의 결과를 앙상블.
- 변환을 거친 여러 케이스의 결과를 앙상블하므로 이전보다 예측 안정성 증가.
3. 참고자료