K-Nearest Neighbor (kNN)
from sklearn.datasets import make_blobs
import numpy as np
import matplotlib.pyplot as plt
from matplotlib import colors
%matplotlib inline
from sklearn.model_selection import train_test_split
from sklearn.metrics import euclidean_distances
from sklearn.neighbors import KNeighborsClassifier
데이터 분할 및 KNeighborsClassifier 훈련
from sklearn.model_selection import train_test_split
X_train, X_test, y_train, y_test = train_test_split(X, y)
from sklearn.neighbors import KNeighborsClassifier
knn = KNeighborsClassifier(n_neighbors=1)
knn.fit(X_train, y_train)
print(f"accuracy: {knn.score(X_test, y_test):.2f}")
neighbors = range(1, 30, 2)
training_scores = []
test_scores = []
X_train, X_test, y_train, y_test = train_test_split(X, y, random_state=13)
for n_neighbors in neighbors:
knn = KNeighborsClassifier(n_neighbors=n_neighbors).fit(X_train, y_train)
training_scores.append(knn.score(X_train, y_train))
test_scores.append(knn.score(X_test, y_test))
시각화
plt.figure()
plt.plot(neighbors, training_scores, label="training scores")
plt.plot(neighbors, test_scores, label="test scores")
plt.ylabel("accuracy")
plt.xlabel("n_neighbors")
plt.legend()
plt.savefig("images/knn_model_complexity.png", bbox_inches='tight')
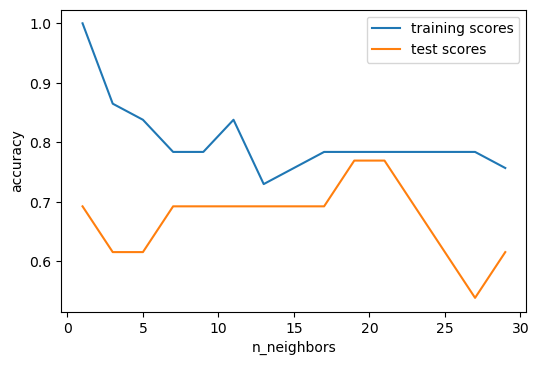
Overfitting the test set
line = np.linspace(0, 8, 100)
train = 1./(1 + np.exp(-line))
plt.plot(train, label='training accuracy')
gen_true = - (line/ 10) ** 2 + (line/10 - .5) ** 3 + 1
gen_true = train - (line/15) ** 2 - .2
plt.plot(gen_true, label="real_generalization")
plt.ylabel("Accuracy")
plt.xlabel("Hyper-parameter")
plt.legend()
plt.savefig("images/overfitting_validation_set_1.png")
rng = np.random.RandomState(0)
plt.plot(train, label='training accuracy')
plt.plot(gen_true, label="idealized generalization")
validation_set = gen_true + rng.normal(scale=.07, size=100)
plt.plot(validation_set, label="validation set")
plt.ylabel("Accuracy")
plt.xlabel("Hyper-parameter")
plt.legend()
plt.savefig("images/overfitting_validation_set_2.png")
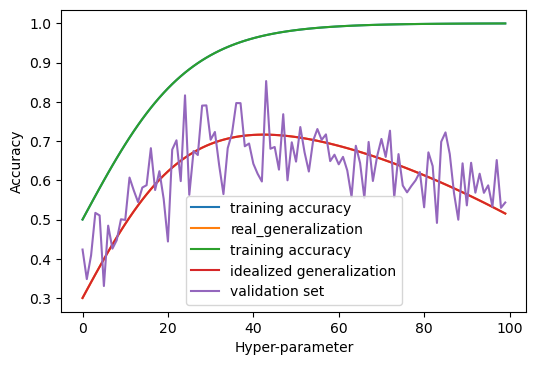
Threefold split
X_trainval, X_test, y_trainval, y_test = train_test_split(X, y)
X_train, X_val, y_train, y_val = train_test_split(X_trainval, y_trainval)
val_scores = []
neighbors = np.arange(1, 15, 2)
for i in neighbors:
knn = KNeighborsClassifier(n_neighbors=i)
knn.fit(X_train, y_train)
val_scores.append(knn.score(X_val, y_val))
print(f"best validation score: {np.max(val_scores):.3}")
best_n_neighbors = neighbors[np.argmax(val_scores)]
print("best n_neighbors:", best_n_neighbors)
knn = KNeighborsClassifier(n_neighbors=best_n_neighbors)
knn.fit(X_trainval, y_trainval)
print(f"test-set score: {knn.score(X_test, y_test):.3f}")
Cross validation
from sklearn.model_selection import cross_val_score
X_train, X_test, y_train, y_test = train_test_split(X, y)
cross_val_scores = []
for i in neighbors:
knn = KNeighborsClassifier(n_neighbors=i)
scores = cross_val_score(knn, X_train, y_train, cv=10)
cross_val_scores.append(np.mean(scores))
print(f"best cross-validation score: {np.max(cross_val_scores):.3}")
best_n_neighbors = neighbors[np.argmax(cross_val_scores)]
print(f"best n_neighbors: {best_n_neighbors}")
knn = KNeighborsClassifier(n_neighbors=best_n_neighbors)
knn.fit(X_train, y_train)
print(f"test-set score: {knn.score(X_test, y_test):.3f}")
from sklearn.model_selection import (KFold, ShuffleSplit, StratifiedKFold, StratifiedShuffleSplit)
import numpy as np
import matplotlib.pyplot as plt
from matplotlib.patches import Patch
np.random.seed(1338)
cmap_data = plt.cm.Paired
cmap_cv = plt.cm.coolwarm
n_splits = 4
n_points = 100
X = np.random.randn(100, 10)
percentiles_classes = [.1, .3, .6]
y = np.hstack([[ii] * int(100 * perc)
for ii, perc in enumerate(percentiles_classes)])
# Evenly spaced groups repeated once
rng = np.random.RandomState(42)
group_prior = rng.dirichlet([2]*10)
rng.multinomial(100, group_prior)
groups = np.repeat(np.arange(10), rng.multinomial(100, group_prior))
def visualize_groups(classes, groups, name):
# Visualize dataset groups
fig, ax = plt.subplots()
ax.scatter(range(len(groups)), [.5] * len(groups), c=groups, marker='_',
lw=50, cmap=cmap_data)
ax.scatter(range(len(groups)), [3.5] * len(groups), c=classes, marker='_',
lw=50, cmap=cmap_data)
ax.set(ylim=[-1, 5], yticks=[.5, 3.5],
yticklabels=['Data\ngroup', 'Data\nclass'], xlabel="Sample index")
visualize_groups(y, groups, 'no groups')
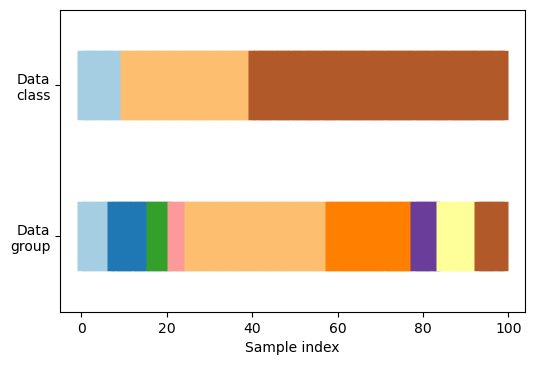
GridSearchCV
from sklearn.model_selection import GridSearchCV
X_train, X_test, y_train, y_test = train_test_split(X, y, stratify=y)
param_grid = {'n_neighbors': np.arange(1, 30, 2)}
grid = GridSearchCV(KNeighborsClassifier(), param_grid=param_grid, cv=10,
return_train_score=True)
grid.fit(X_train, y_train)
print(f"best parameters: {grid.best_params_}")
print(f"test-set score: {grid.score(X_test, y_test):.3f}")
results.plot('param_n_neighbors', 'mean_train_score')
results.plot('param_n_neighbors', 'mean_test_score', ax=plt.gca())
plt.fill_between(results.param_n_neighbors.astype(np.int),
results['mean_train_score'] + results['std_train_score'],
results['mean_train_score'] - results['std_train_score'], alpha=0.2)
plt.fill_between(results.param_n_neighbors.astype(np.int),
results['mean_test_score'] + results['std_test_score'],
results['mean_test_score'] - results['std_test_score'], alpha=0.2)
plt.legend()
plt.savefig("images/grid_search_n_neighbors.png", bbox_inches='tight')
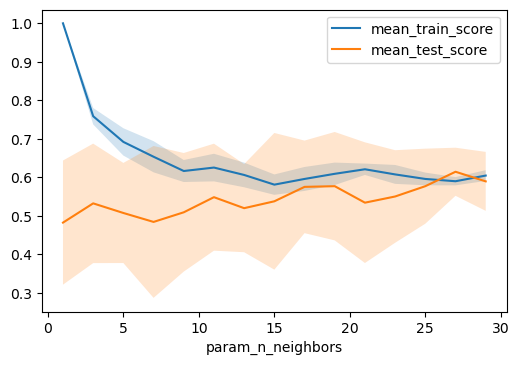
Machine learning is revolutionizing industries by enabling systems to learn from data and make decisions without human intervention. In particular, companies like heron intelligent equipment are leveraging machine learning to develop smart technologies that improve efficiency and productivity. By analyzing vast amounts of data, machine learning algorithms power automation, predictive maintenance, and optimization in various sectors, making processes faster, more accurate, and cost-effective.