화학 정보학 주요 분야 소개
화학정보학이란?
화학 정보학이란? : Field of information technology that uses computers and computer programs to facilitate the collection, storage, analysis and manipulation of large quantities of chemical data
Bioinformatics vs Cheminformatics
- Biological data : Bioinformatics (DNA, Protein sequence, etc)
- Chemical data : Cheminformation (molecular structure, bio-activity, etc)
(in Drug discovery pipeline,,)
- Bioinformatics : Target indentification, validation
- Structural biology : Target validation, Lead identification
생물학의 한 분야로, 분자 구조를 주로 연구하는 학문, 특히 단백질의 3차원 구조를 다룬다.
- Cheminformatics and systems biology : Lead identification, optimization, Preclinical tests
- System biology : 구성요소를 분해하여 개별적인 기능을 식별하는 방식이 아닌, 특정 현상을 정의하기 위해 수많은 변인들의 연결고리를 규명함으로써 현상에 대한 근원적인 이해를 하고자 하는 학문, 유전자나 분자, 세포 수준의 요소를 통해 그들 간의 관계를 찾고, 관계에 대한 정보들을 시스템 차원의 관점에서 통합하여 분석하는 학문
최근 computing power가 향상 됨에 따른 오믹스 생물학의 발전으로, 다수의 변인에 대해 동시 측정이 가능하게 되어 생명현상을 전체주의적인 관점에서 해석하고자 하는 시스템 생물학적 접근이 활발하게 시도되고 있다.
응용분야 : 신약개발, 독성학, ...
*interdisciplinary
CADD
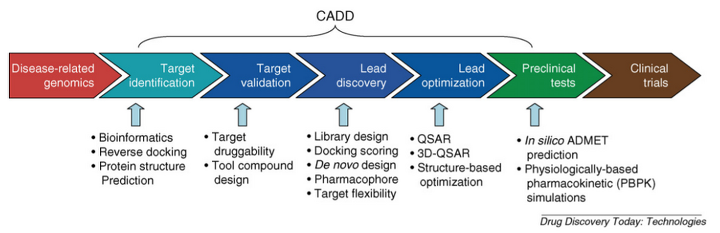
*reverse docking : target fishing
*De novo design: target structure가 정해진 상태에서 compound design 설계
*PBPK: 몸속에 혈류에 흡수되서 어떻게 우리 organ에 diliver가 되는지 (systems biology)
각 단계에서 활용되는 기술들
-
Target identification
- Target Prediction: bioactive compound의 molecular target 예측 (=Target Fishing)
천연물 혹은 phenotypics screening을 통해 발견된 화합물의 target 예측
* 방법: revese docking, revese QSAR
- Drug repositioning(이미 약효가 알려진 약물이 다른 질병에 약효가 있을 경우)에 활용
- Toxicity 예측 시 활용 (원하지 않는 target에 binding 하는 지를 예측하는것
- Protein structure prediction : 단백질 구조를 예측하는 것
*AlphaFold(DeepMind)
-
Target validation
- Target druggability: Likelihood of being able to modulate a target with a small molecule drug
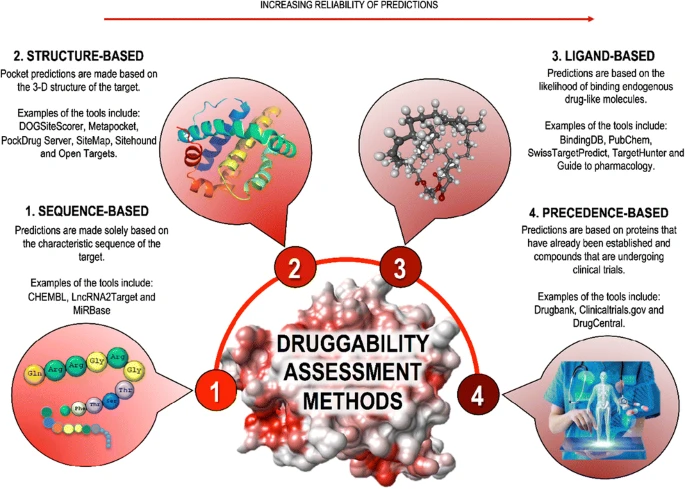
주로 사용되는 method = structure based method : binding pocket
-
Lead discovery & Optimization
- Compound library design : Hit compound identification시 사용, 어떤 compound가 디자인 되어야하는지..?
- Virtual screening : compound가 이미 있고, 그 중 가장 적합한게 뭔지 찾는거
*ligand based method : 이미 binding 한다고 알려진 ligand가 있을 경우, (단백질의 구조에 대한 정보가 없어도 된다.) Machine learning algorithm ⇒ QSAR 3D shape similar search ⇒ 3D QSAR 장점: structure-based보다 더 정확함 단점: active ligand가 있어야되어 완전 새로운 target에 대해서는 적용할 수 없다. (me-too drug를 만들 때 주로 사용되는 방법)
-
Docking
Binding pocket(receptor)에 가장 잘 붙는 ligand를 찾는것
scoring함 (highest energy, lowest energy)
-
Pharmacophore modeling (simplified docking)
Pharmacophore-based VS : A pharmacopher is an abstract description of molecular features that are necessary for molecular recognition of a ligand by biological macromolecule
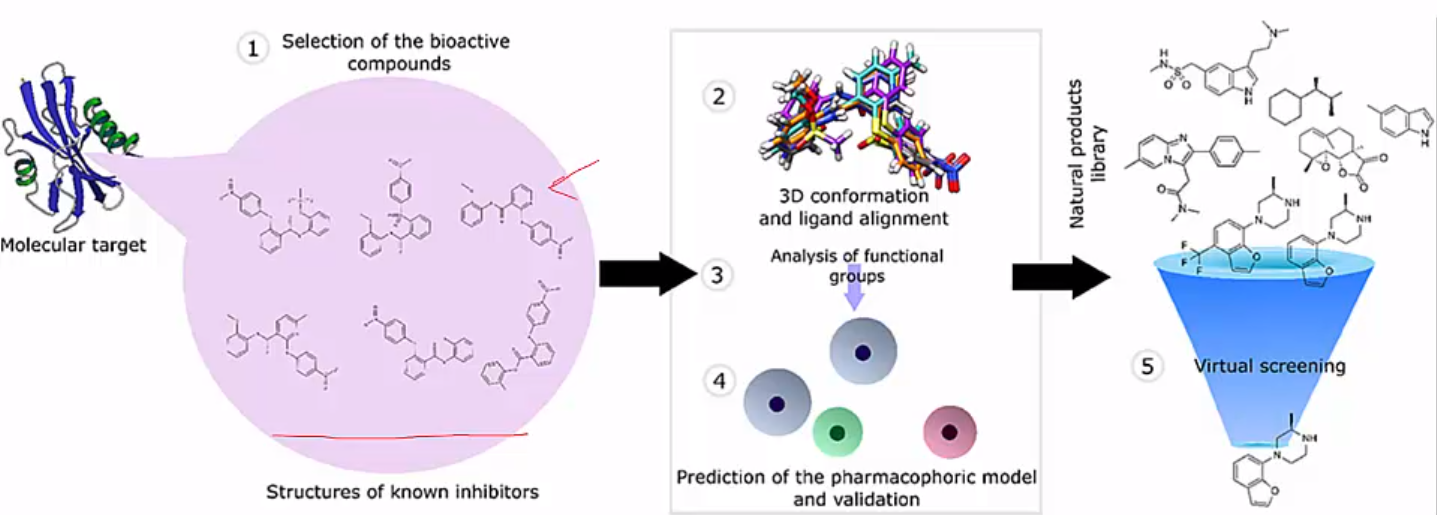
3. QSAR
- De novo design : 처음부터 적합한 compound를 디자인 하는거
Deep learning: Reinforcement learning
- Preclinical testing
- ADME/Tox prediction and optimization
- Physiologically-based Pharamcokinetics (PBPK) simulation
- 임산부, 어린아이 등 실험을 할 수없는 환자군에 대해서 ADME/Tox 예측하기 위해 사용
출처 : LAIDD 강의