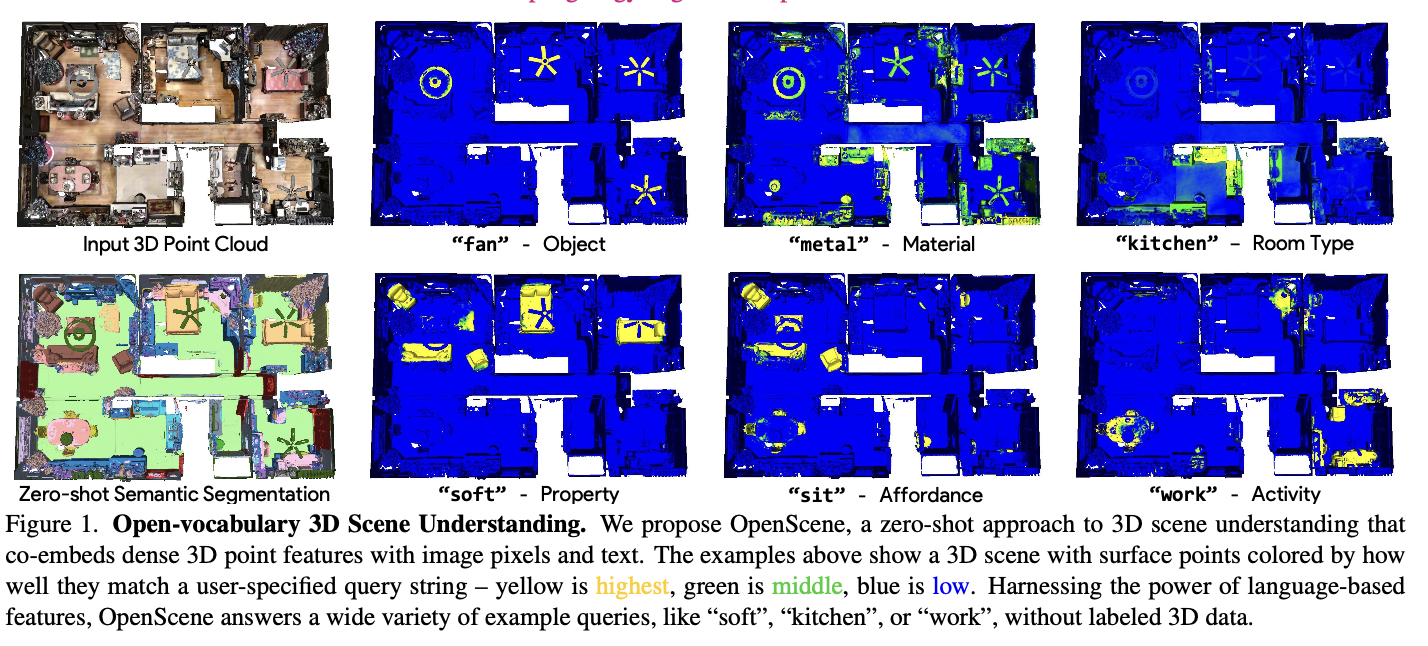
Overview
Pretrained CLIP을 이용하여 3D scene understanding을 하고자 한다. 이를 위해 3D point cloud와 multi-view 2D images를 입력으로 받아, 각 3D point에 해당하는 특징과 pretrained 2D encoder로부터 얻은 해당 3차원 점이 투영되는 픽셀들의 특징이 align되도록 distilation 기법을 적용한다.
Problem
- 기존 3D Scene understanding 기법들은 3D ground truth가 필요한 supervsied learning 방식 위주였다
- 기존 방법들은 single task마다 학습을 달리 해주어야 했다
Solution from the paper
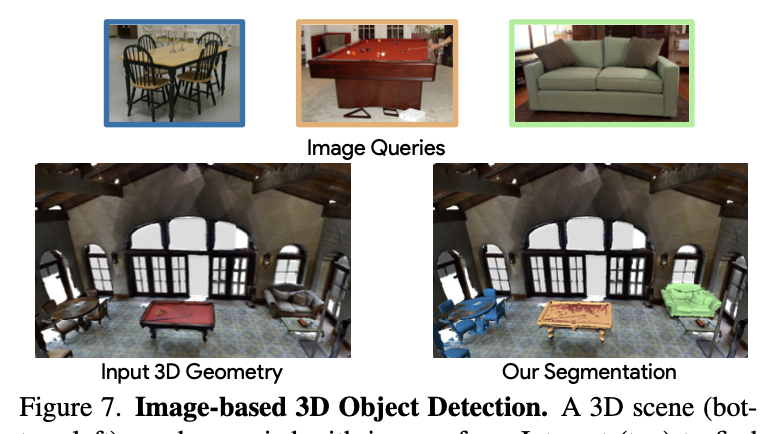
- 3D point enocoder 학습: Distilation from pretrained 2D encoder
- 3D point - 2D pixel distilation을 효과적으로 하기 위해, 2D pixel의 특징은 multi-view images에 대응되는 pixel들에서 얻은 f2Di=1,...k를 average pooling 하여 사용
- 2D feauture f2D와 3D feature f3D 중, text query feature ftext와 cos_similarity가 높은 feautre를 선택하는 2D-3D Ensemble 기법을 도입하여 성능을 boost
- Large-scale model의 knowledge를 활용하여, 여러 task를 해결
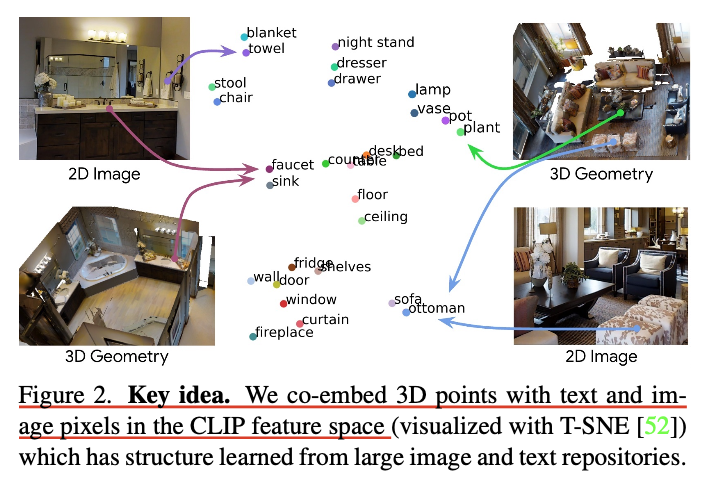
- 3D point feature f3D를 CLIP embedding space로 mapping하도록 distillation을 적용
- 3D semantic segmenation task의 zero-shot performace가 SOTA이다.
- 이 외에도 다양한 task(Image-based 3D object detection, 3D scene explanation, 3D search)를 추가적인 학습 없이 할 수 있음
- 3D scene explantaion 예시
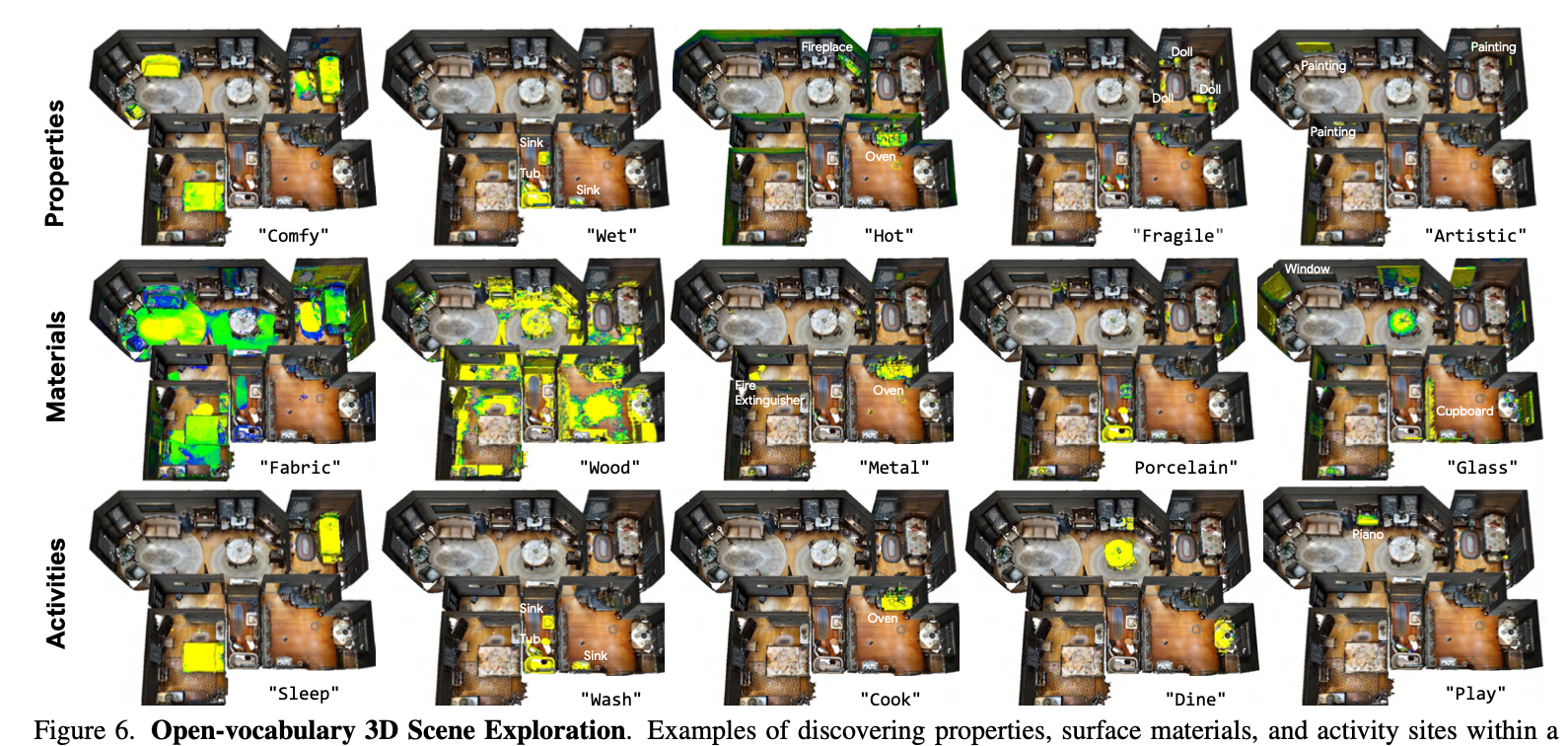