https://www.bradyneal.com/causal-inference-course
Introduction to Causal Inference라는 강의를 듣고 정리했습니다.
3. The Flow of Association and Causation in Graphs
3-1. Local Markov assumption
- non-descendant에 대하여 independent
- 아래 상황에서 P(x4∣x3,x2,x1)=P(x4∣x3)
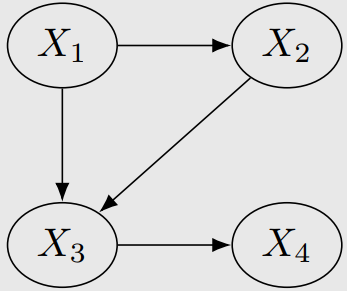
3-2. Bayesian network factorization
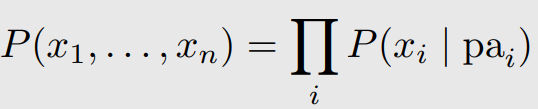
- parent만 child에게 영향을 준다는 의미
- local markov assumption = bayesian network factorization
3-3. Minimality assumption
- local markov assumption : non-descendant에 대하여 independent
- adjacent nodes는 dependent
3-4. Causal edges assumption
- every parent가 모든 children의 direct cause가 된다.

3-4. Chain and Fork
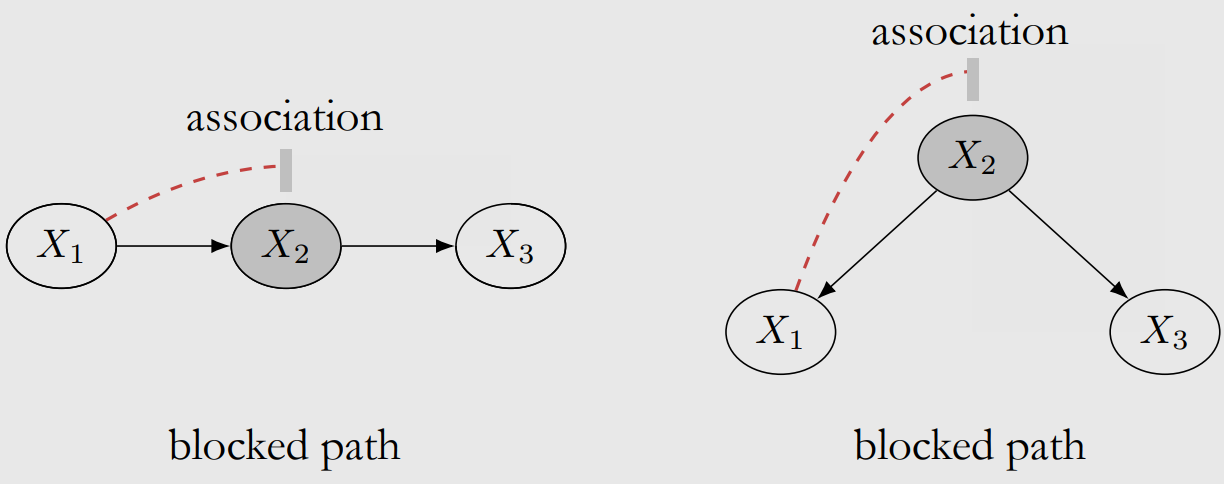
- 증명
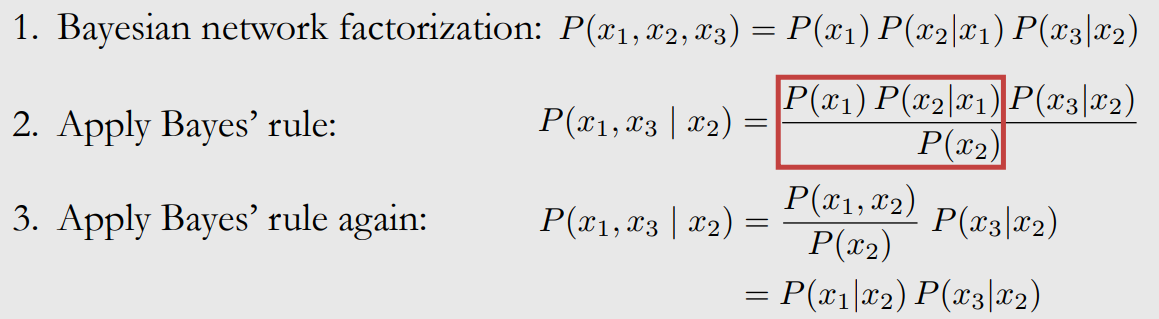
3-4. Immoralities(Collider)
- collider의 parents의 independence 증명
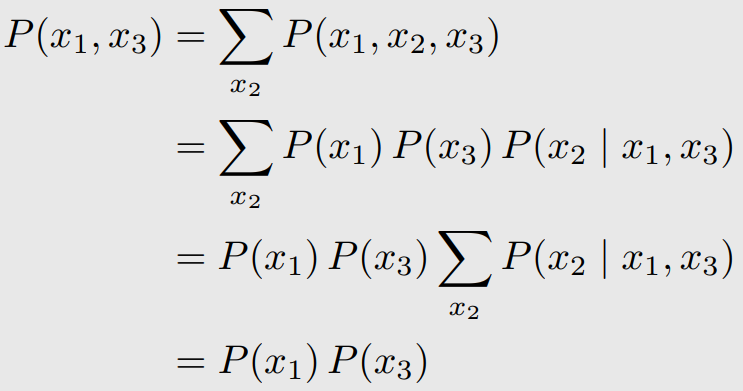
- conditioning on descendants of collider
- collider의 parent의 independence가 깨진다.
3-5. Blocked path definition
- conditioning set에
- Confounder는 모두 포함
- Collider와 Collider의 desendants는 모두 포함되지 않음
3-6. d-separation
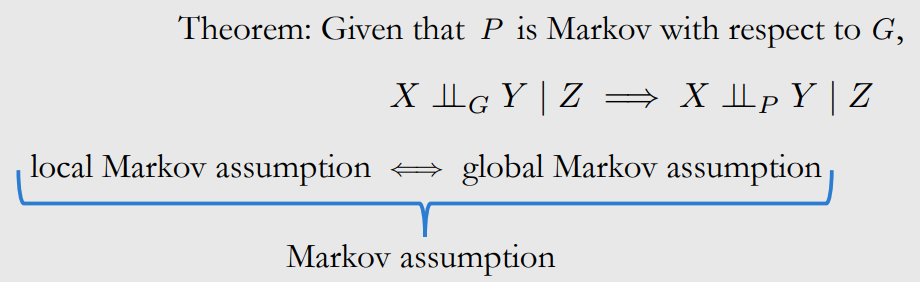
이해안됨.