Chi-Square GOF test
- Used to evaluate the distribution of one categorical variable with more than 2 levels.
- Evaluating by comparing the distribution of that categorical variable to a hypothetical distribution.
- Used to evaluate if the distribution of levels of a single categorical variable follows a hypothesized distribution.
Evaluating the hypotheses
- quantify how different the observed counts are from the expected counts.
- large deviations from what would be expected based on sampling variation(chance) alone provide strong evidence for the alternative hypotheis.
- called a goodness of fit test since we're evaluating how well the observed data fit the expected distribution.
Conditions for the Chi-square Test
- Independence: Sampled observations must be independent
- Random sample / assignment
- If sampling without replacement, n < 10% of population
- Each case only contributes to one cell in the table
- Sample size: Each particular scenario (i.e. cell) must have at least 5 expected counts
Anatomy of a Test Statistic
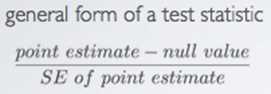
- Identifying the difference between a point estimate and an expected value if the null hypothesis were true.
- Standardizing that difference using the standard error of the point estimate.
Chi-Square Statistic

When dealing with counts and investigating how far the observed counts are from the expected counts, we use a new statistic called the chi-square(X2) statistic.
※ A cell is referred to a level of the categorical variable
Why Square?
- Want to get rid of negatives: positive standardized difference
- Not absolute, but square: highly unusual differences between observed and expected will appear even more unusual.
Degrees of Freedom

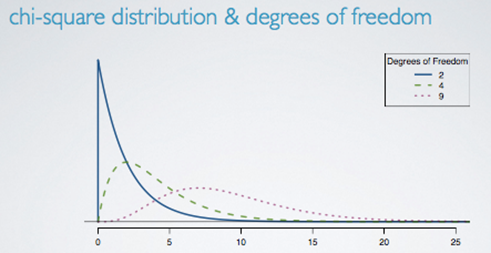
- Chi-square distribution has only one parameter: degrees of freedom: influences the shape, center and spread.
- To determine if the calculated X2 statistic is considered unusually high or not, we need to first describe its distribution.
P–value
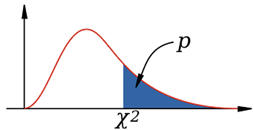
- P-value for a chi-square test is defined as the tail area above the calculated test statistic
- Because the test statistic is always positive, and a higher test statistic means a higher deviation from the null hypothesis
- You get p-value from X2 and d using the table.
INSTRUCTIONS
- Set the hypothesis
- H0: Actual and expected distributions follow the same distribution
- H1: Actual and expected distributions do not follow the same distribution
- Calculate the expected number
- Check conditions
- Draw sampling distribution, calculate test statistic, shade p-value
- Make a decision, and interpret it in context of the research question
(example)
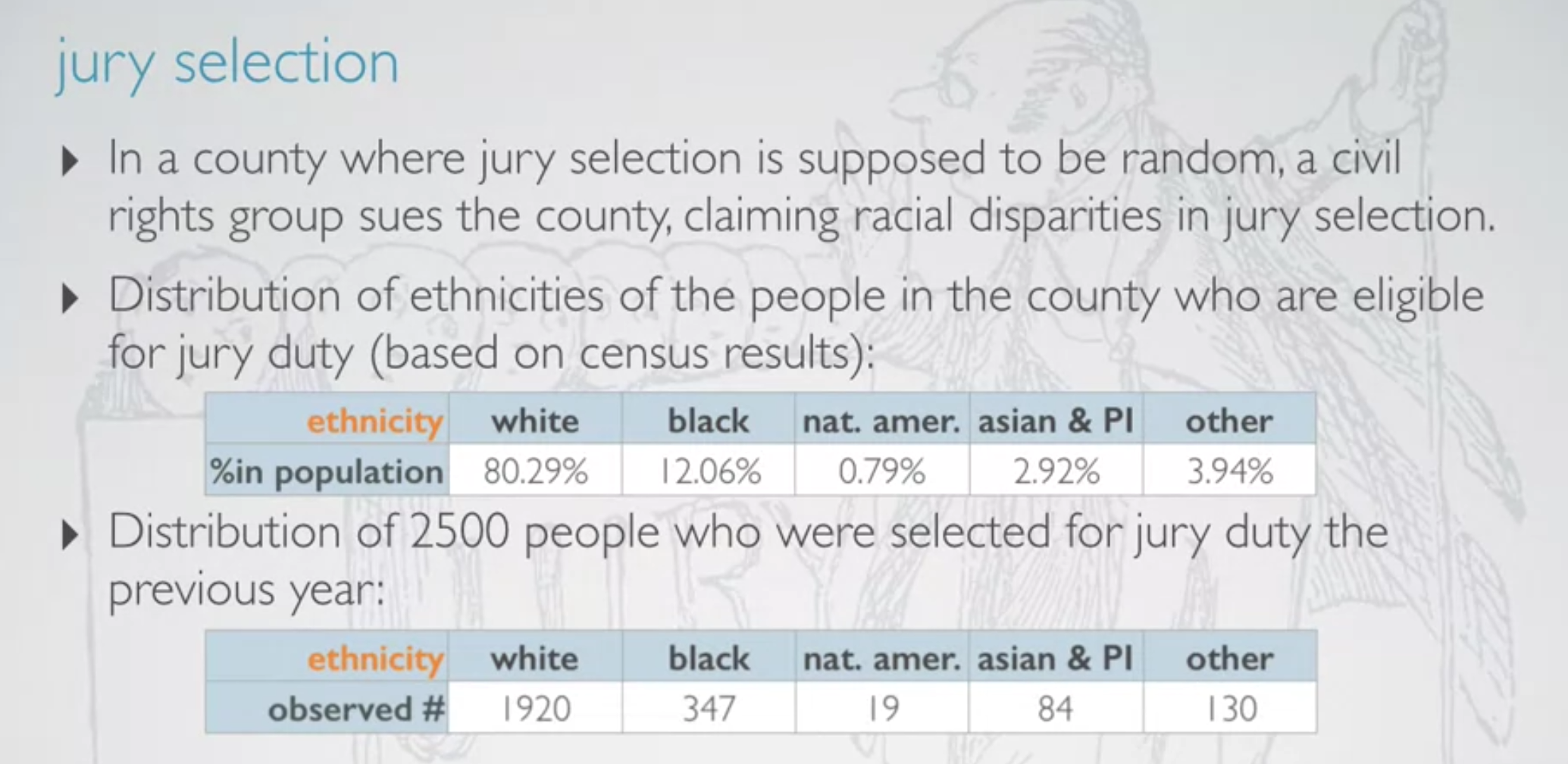
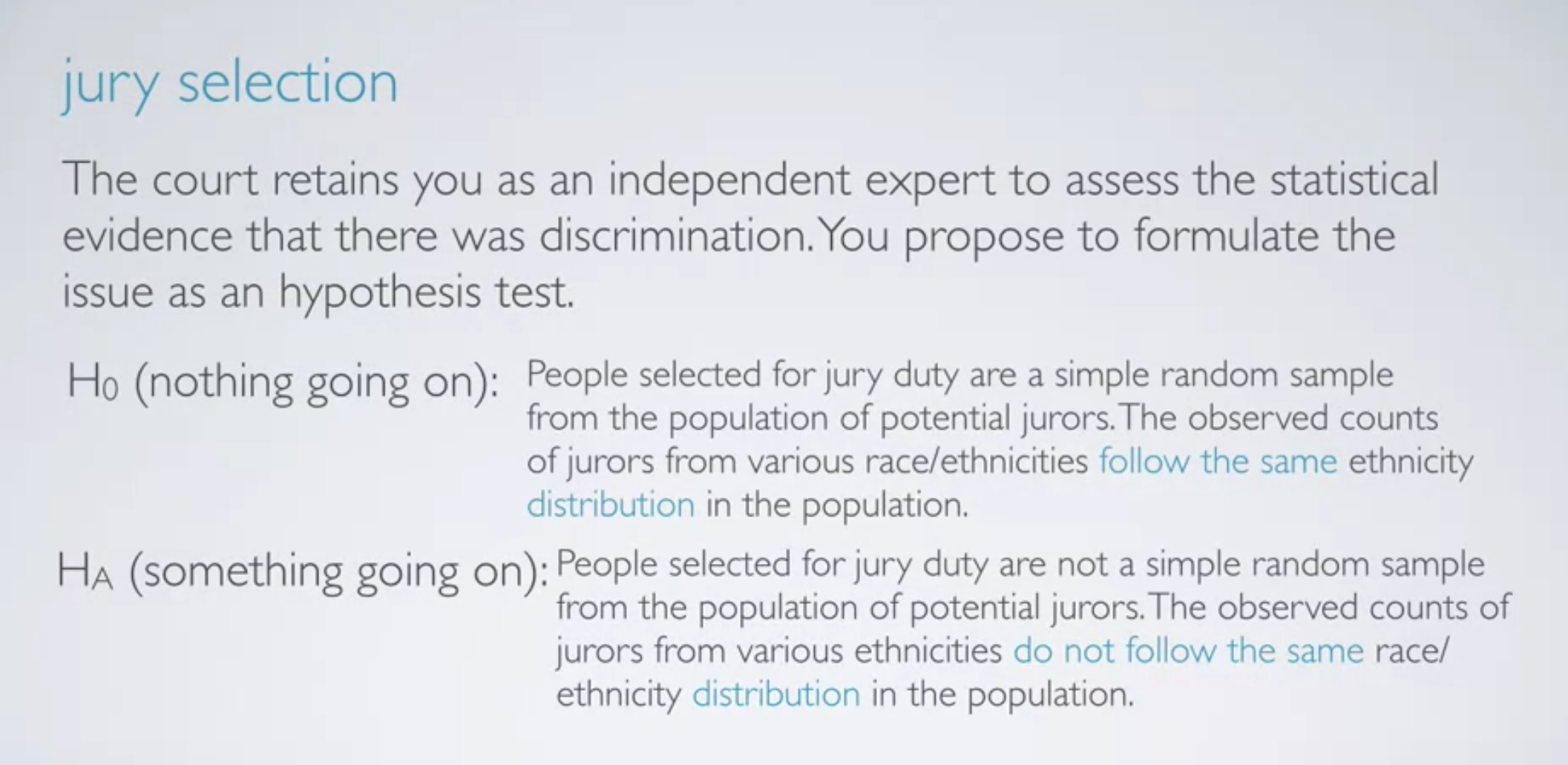
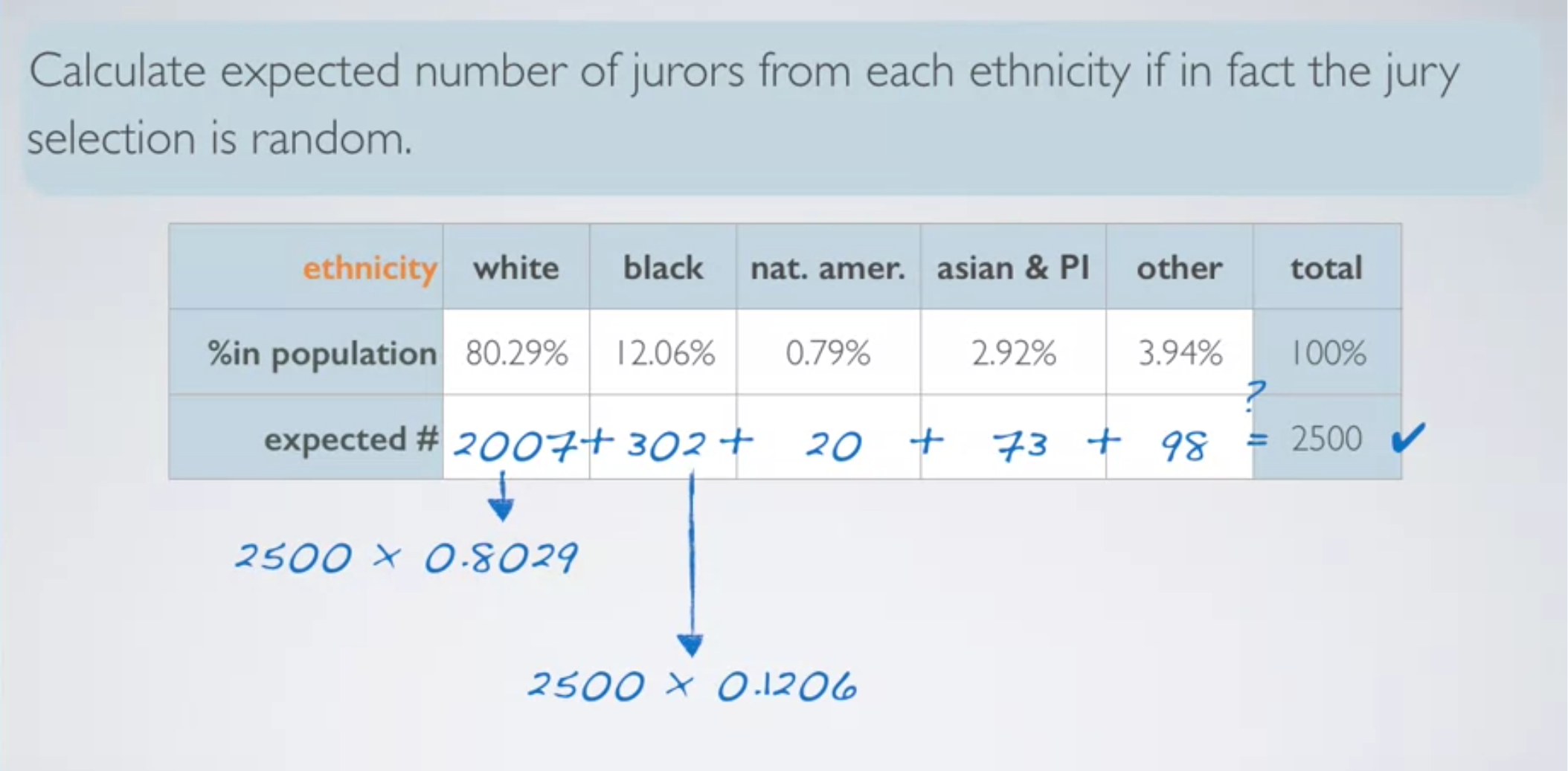
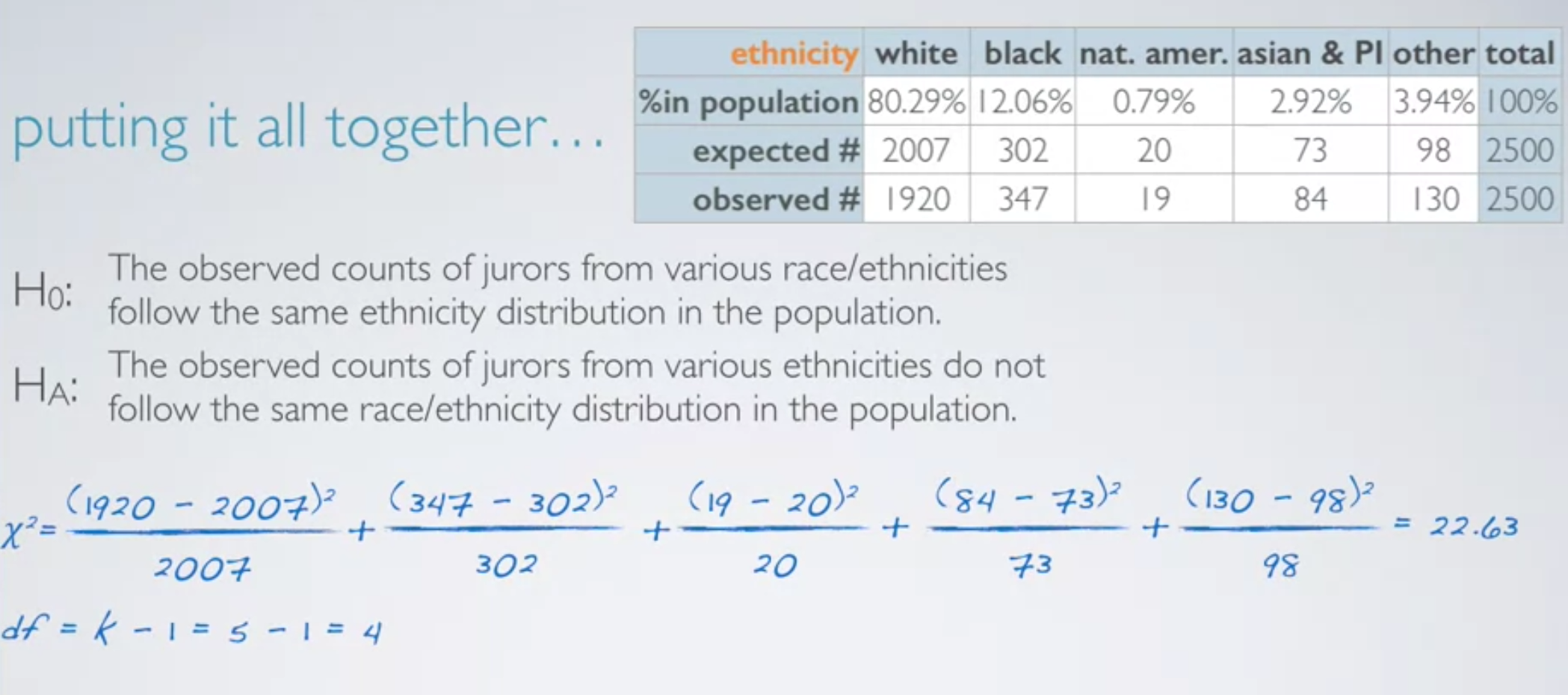
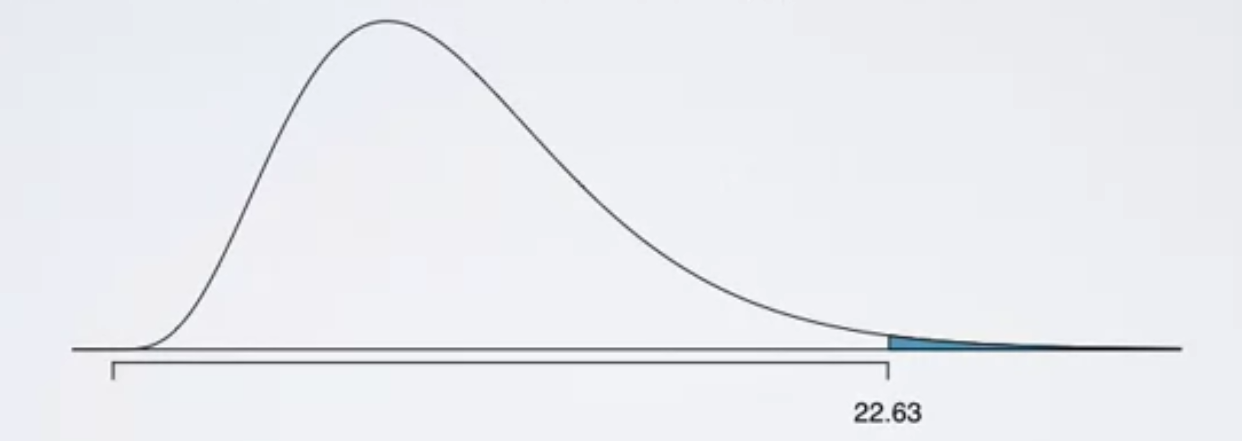