Sequential Model
몇 개의 데이터가 입력되든 동작할 수 있어야 함
- Markov Model
- 현재는 바로 전 과거에만 dependent하다고 가정
- Latent autoregressive model
- 하나의 과거가 이전의 모든 과거를 요약하고 있는 Hidden state
RNN
→ 먼 과거의 정보가 현재 정보에 영향을 적게 미침 (Short-term dependencies)
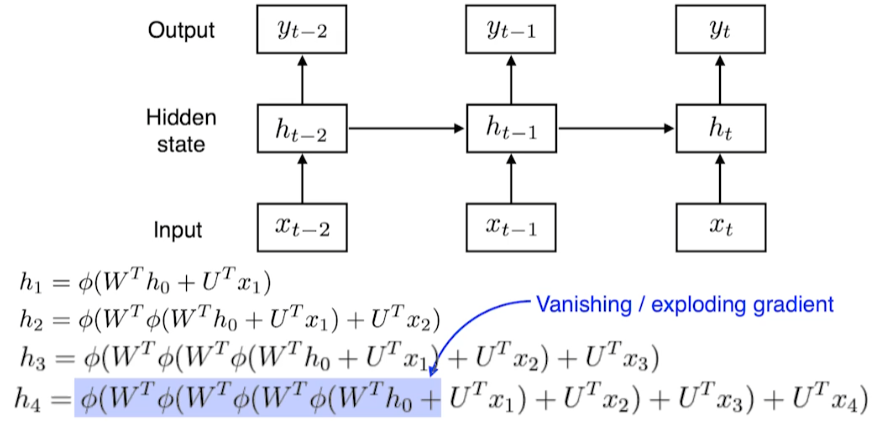
-
만약 activation function이 sigmoid라면, sigmoid 함수는 값을 0 ~ 1 사이로 mapping
- 중첩될수록 gradient 값은 계속 작아짐 (Vanishing)
-
activation function이 ReLU라면, gradient가 계속 양수면 그 값을 계속 곱함
- 중첩될수록 gradient 값이 매우 커짐 (exploding)
LSTM
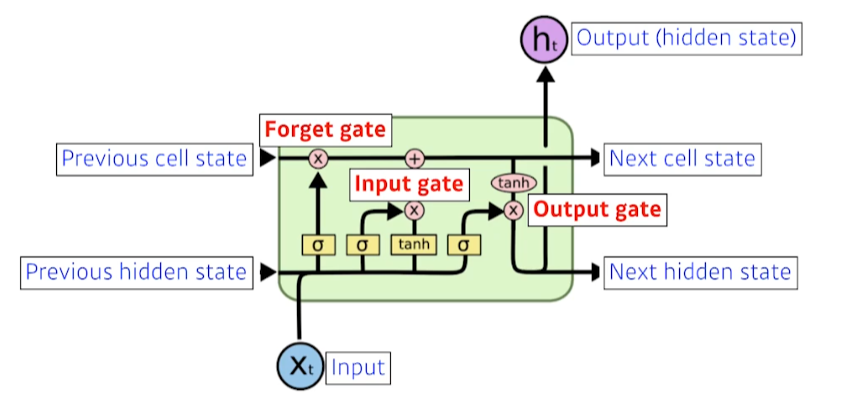
Cell State
- time step t까지의 모든 정보를 요약하고 있는 state
- 컨베이어 벨트처럼 물건을 올리고, 내리는 조작 기능 (gate)
Forget Gate
- 이전 cell state에서 어떤 정보를 버릴지 결정
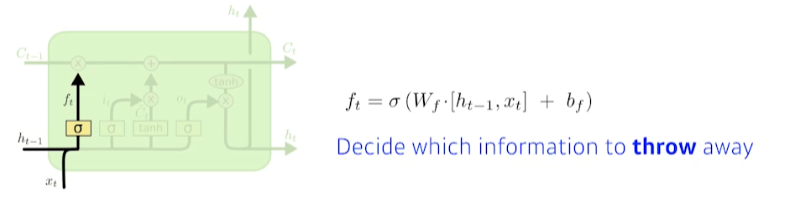
- previous hidden state(output)와 현재 입력을 가지고 sigmoid를 통과시켜 이전 cell state에서 넘어오는 정보 중 버릴 것을 결정
- 현재 cell state에 어떤 정보를 올릴지 결정
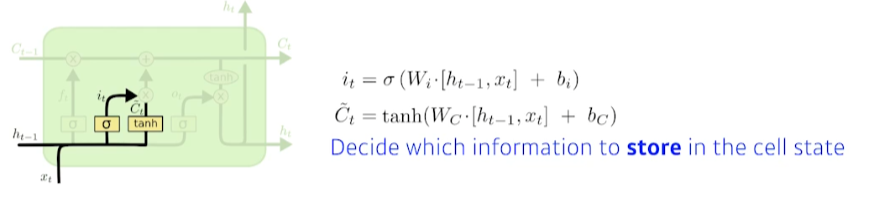
- input gate는 previous hidden state(output)와 현재 입력을 가지고 sigmoid를 통과시켜 만듦
- Ct~는 previous hidden state와 현재 입력에 tanh를 적용시켜 만들어진 cell state candidate
Update cell
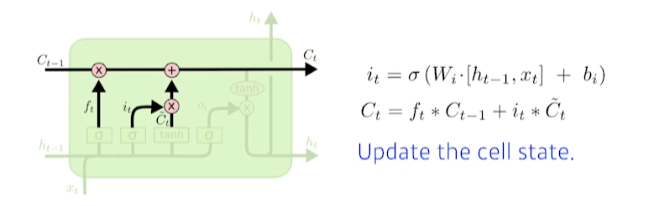
- 이전까지 summarize된 cell state와 현재 정보와 이전 output으로 얻어진 cell state candidate를 잘 조합해 새로운 cell state에 update
- 이전 cell state는 forget gate를, 현재 cell state candidate 에는 input gate를 통과시켜 현재 cell state Ct 를 만듦
Output Gate
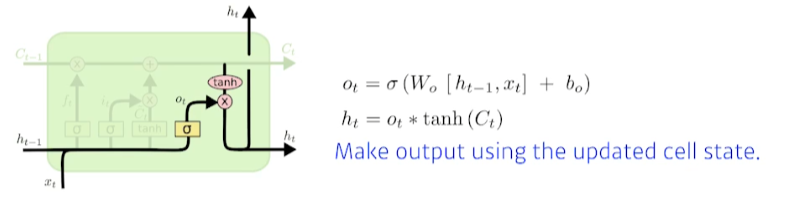
- 현재 cell state에서 어떤 값을 밖으로 빼낼지 결정
- next cell state와 next hidden state가 만들어짐
GRU (Gated Recurrent Unit)
- reset gate , update gate 두 개의 gate
- cell state가 없고 hidden state만 존재
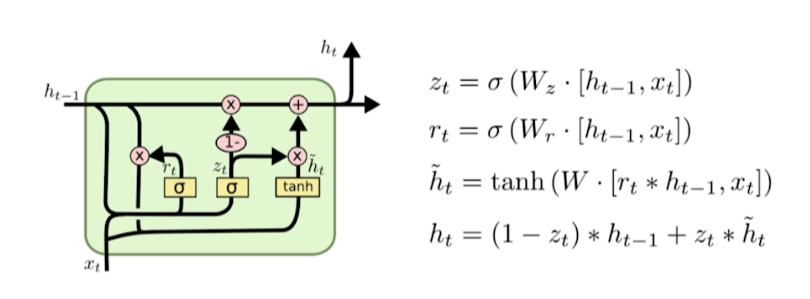
※ 모든 이미지의 출처는 네이버 커넥트재단 부스트캠프 AI Tech 5기입니다. ※