소개
- 이 글은 논문을 읽고 정리하기 위한 글입니다.
- 내용에 오류가 있는 부분이 있다면 조언 및 지적 언제든 환영입니다!
DOVER
: A method for combining diarization output
[1] 2019, ASRU Workshop 에 올라온 논문입니다. (paper)
DOVER-LAP
: A method for combining overlap-aware diarization outputs
[2] 2021, SLP Workshop 에 올라온 논문입니다. (Paper, github, ppt)
Citation
[1]
@misc{stolcke2020dover,
title={DOVER: A Method for Combining Diarization Outputs},
author={Andreas Stolcke and Takuya Yoshioka},
year={2020},
eprint={1909.08090},
archivePrefix={arXiv},
primaryClass={cs.CL}
}
[2]
@misc{raj2020doverlap,
title={DOVER-Lap: A Method for Combining Overlap-aware Diarization Outputs},
author={Desh Raj and Leibny Paola Garcia-Perera and Zili Huang and Shinji Watanabe and Daniel Povey and Andreas Stolcke and Sanjeev Khudanpur},
year={2020},
eprint={2011.01997},
archivePrefix={arXiv},
primaryClass={eess.AS}
}
참조
Diarization Output Voting Error Reduction (DOVER)
Introduction
Goals
- 다양한 Speaker Diarization 모델들의 결과를 효과적으로 앙상블 하기위한 기술
- 기존 ASR 에서의 ROVER와 같은 ensemble 에서 motivated 됨
Problems
- Systems outputs may have different number of speaker estimate
- System outputs are usually in different label space
- 훈련된 dataset이 달라서 speaker target이 다를 수 있다.
- 즉, 각 모델이 같은 화자에 대해서 각자 다른 label 값으로 출력할 수 있다.
- Systems outputs may have different number of speaker estimate
- 각 시스템은 각기 다른 화자 수를 예측할 수 있다.
Main Proposal
- Label mapping
- Maximal matching algorithm based on a global cost tensor
- Label voting
- Weighted majority voting considers speaker count in region
Proposed Method
- 각각 다른 Clustering 방식 및 후처리를 적용한 3가지 SD 결과가 있다고 하자.
Label Mapping
-
각기 다른 모델에서 나온 SD 결과에 대한 대표 Speaker label를 만들어내는 과정
-
Pair-wise incremental label mapping
- Incremental pair-wise
+ 전제를 한번에 합치는 것이 아닌 2개의 결과 씩 합쳐 나가는 방식
- label mapping algorithm (Hungarian method)
+ 2개의 결과를 합치는 알고리즘으로 Hungarian method 를 사용함
+ SD 결과들을 Graph
로 해석하였고, Vertex
는 하나의 segment, Edge
값은 두 Segment 간에 overlap duration 으로 구성함
+ 이후 2개의 Vertex
간에 Edge weight 합이 최소가 되도록 하는 이분 매칭(Bi-partite graph matching)을 찾아냄
+ 매칭 이후 두 Vertex가 연결된 경우 같은 label
로 간주
- Incremental pair-wise
+ 2개 합친 결과와 나머지 결과간에 label mapping algorithm 진행
-
How to select Hypothesis pair
- 1) Method 1 (centroid selection)
- Rank all the hypothesis based on average DER to all other hypothesis. Choose the top-ranked as anchor
- 가장 낮은 DER를 가지는 Hypothesis를 선택하여 시작
- 2) Method 2 (Random sampling)
- Run N times, once with each hypothesis as anchor and finally average all
- N 번 돌린 결과에 대한 평균을 사용
Label voting
- Label Mapping 이후에 global speaker label space가 아래와 같다고 하자.
- 첫번째 region에 대한 Label voting 결과는 아래와 같다.
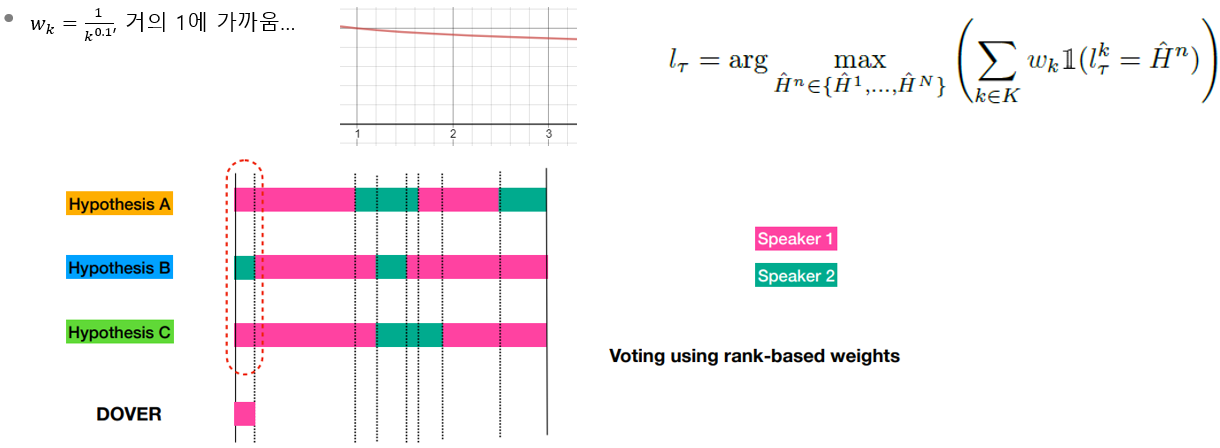
- 모든 region 에 label voting 이루어 진 DOVER 결과는 아래와 같다.
- Experiment results는 DOVER-lap과 같이 설명하도록 하겠다.
DOVER-lap (DOVER
-Over
lap)
Introduction
Limitation
- 1) non optimal label mapping
- Hypothesis 를 합치는 순서에 따라 결과가 달라지기 때문에, optimal pair-wise cost를 보장하지 못한다.
- 즉, 모든 hypothesis를 동시에 볼 수 없다.
- 2) overlap speech
- DOVER의 Voting method는 overlapping speaker segment를 다루지 못한다.
Main Proposal
- 1) Revised Label matching
- 이분 매칭을 K-partite matching 형태의 3D matching 으로 근사하여 모든 pair-wise edge weights를 동시에 다룰 수 있게 되었다.
- 2) Revised label voting
- Overlap speaker 상황에 대한 voting 방법을 추가하였다.
Proposal Method
Label matching
- 기존의 2개의 가정을 선택하여 incremental 하게 합쳐나가는 DOVER label matching 방식이 아닌 한번에 모든 가정을 합쳐나가는 방식을 사용했다.
- 여기서 각 가정들을 조합하여 만들 수 있는 하나의 조합을
tuple
로 정의하였다.
- 아래 그림과 같이 3개의 가정들에 대해서 DOVER와 같이 Graph로 변경하여 생각한다.
- 1) Compute Global Cost Tensor
- 3개의 가정들에서 각 segment인
Vertex
를 하나 씩 선택하여 3명 간에 overlap 정도를 cost로 계산한다.
- Cost(a3,b3,c1)=−(Ma3,b3+Ma3,c1+Mb3,c1)
- 위 Cost 값은 아래 오른쪽 그림에 빨간 박스와 같이 3차원 좌표의 값으로 생각할 수 있다.
- 다른 vertex 조합에 대해서도 cost를 계산해간다.
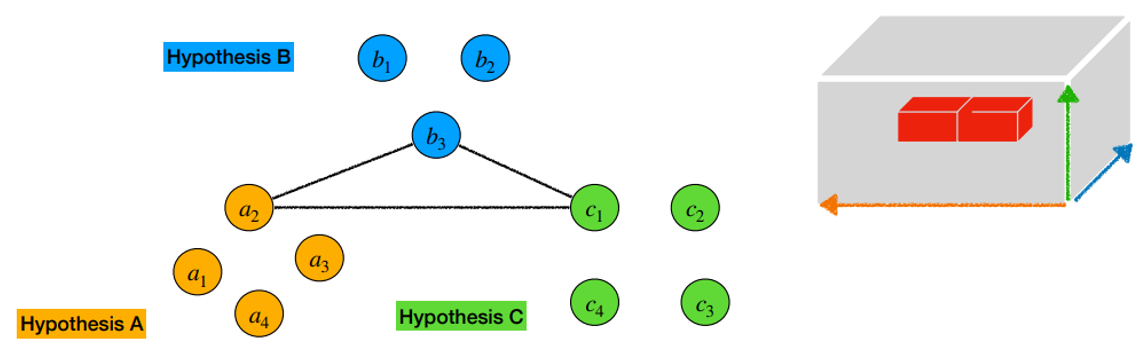
- 모든 경우의 tuple cost 값을 계산하고, 이 cost 값을 가진 tensor 를
global cost tensor
라고 지칭하자.
- 2) Label Matching Algorithm
- 2.1) Pick tuple with lowest cost
+ 가장 낮은 cost 값을 가지는 tuple 를 선택하여 speaker label를 할당한다.
- 2.2) Discard all tuples containing these labels
+ Assign 된 좌표에 대해서는 모두 discard 한다.
- 2.3) 위 2.1, 2.2 과정을 반복한다.
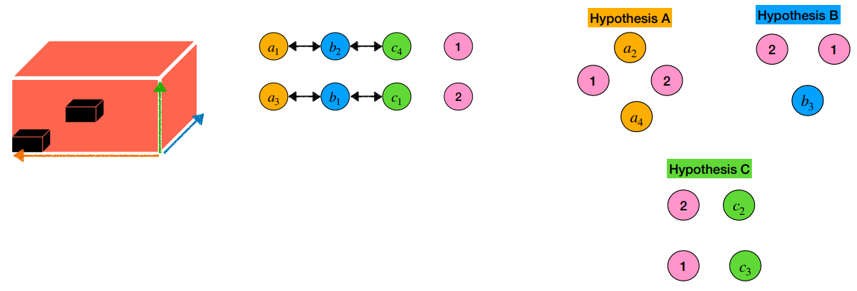
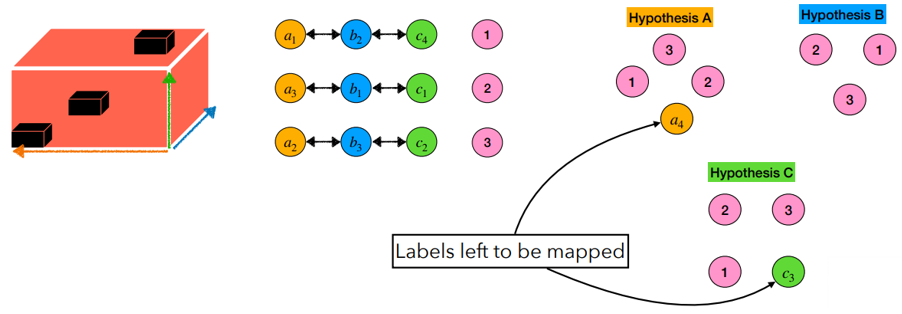
- 2.4) If no tuples remaining but labels left to be mapped, remove filled dimensions and repeat
+ tuple에 대해서 모두 mapping 되었는데도 남은 label이 존재하면, 2차원에 대해서 mapping 작업을 진행한다.
최종 label matching 출력은 아래와 같다.
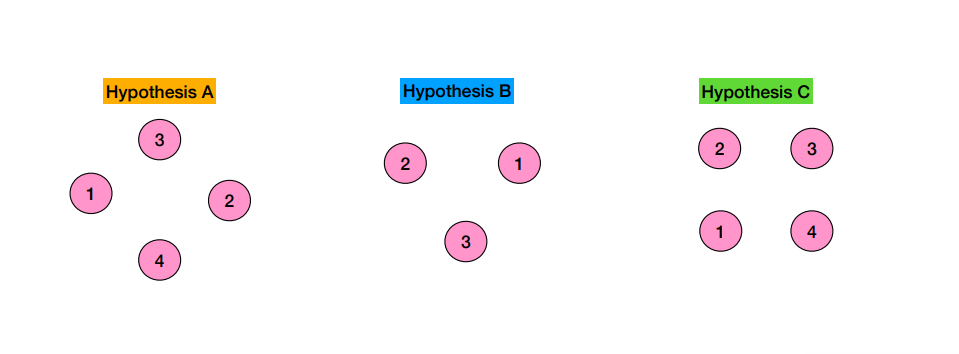
Label Voting
DOVER-lap은 기존 DOVER와 다르게 한 region에 대해서 multi-speaker 상황인 overlap을 다룰 수 있도록 하였다.
- 1) Divide into regions
- Dover과 동일하게 speaker의 시작과 끝 지점으로 region을 나눈다.
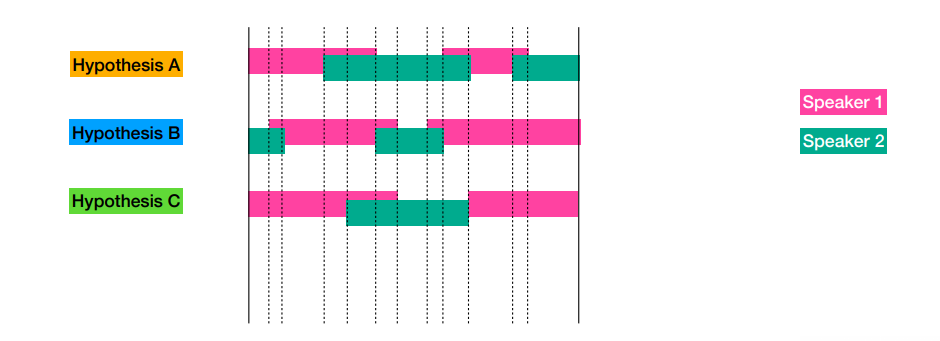
- 2) Estimate number of speakers in each region
- 각 Region에 존재하는 화자 수를 추정한다.
- 이때, Region에 대한 화자 수에 대한 majority voting이 이루어지는데, DOVER과 동일하게 rank-weighting 를 사용한다.
- 모든 region 에 대한 화자 수와 rank-weighting을 고려한 결과는 아래와 같다.
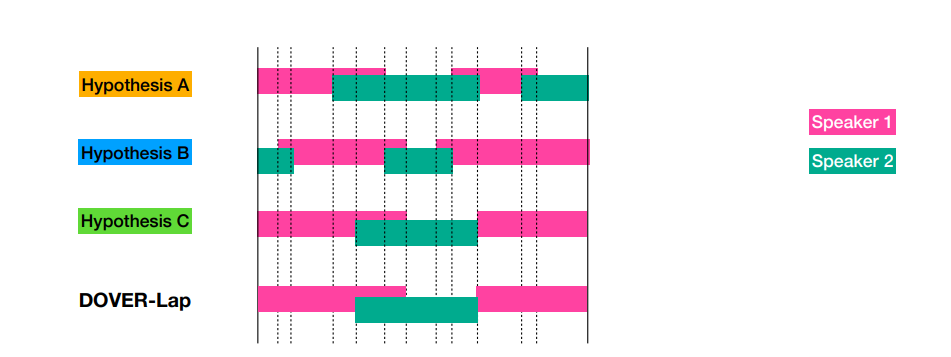
- 구체적인 알고리즘이 궁금하다면 아래 글을 참고하면 좋을 것 같다.
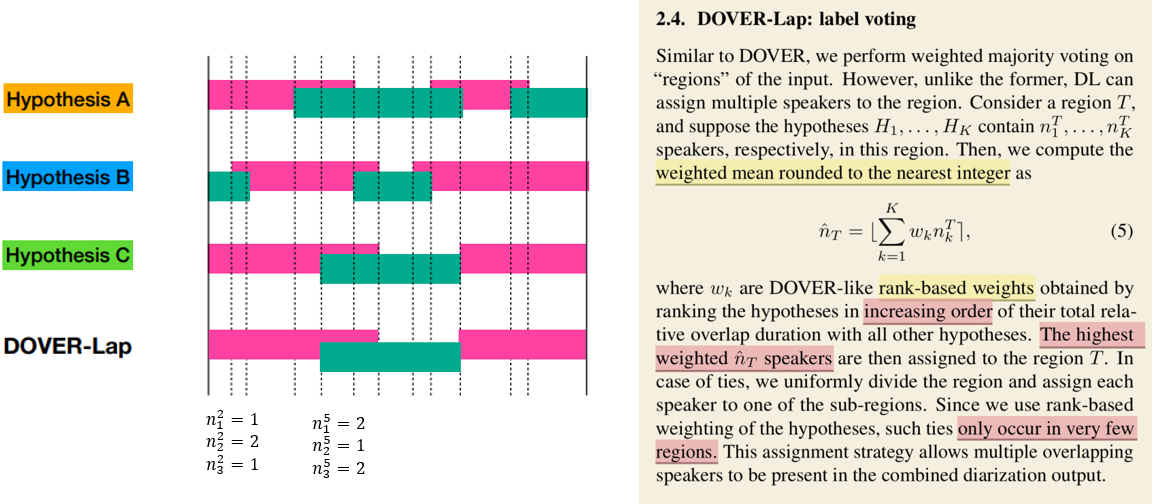
Experiments Result
Results: AMI dataset
- DOVER 관점
- Global mapping 으로 Speaker Confusion Error은 상당히 줄어들었다.
- 여전히 DER은 ensemble 이후에도 최고 성능보다 떨어짐을 볼 수 있다.
- DOVER-lap 관점
- Speaker Confusion Error 는 ensemble 에 참여한 모든 결과보다 좋은 성능을 보였지만, DOVER의 Global mapping 보다는 떨어지는 성능을 보였다.
- 최고 성능이었던 VB-based overlap assignment의 DER보다 더 좋은 DER 값을 가지는 것을 보였다.
Results: Breakdown on LibriCSS eval
- Speaker Confusion: 모든 부분에서 Dover-lap이 더 좋은 성능을 보여준다.
- False Alarm: 기존 VB-based overlap assignment 성능보다는 조금 떨어지지만 나머지 3개보다 좋은 성능을 보였다. 즉 적절하게 ensemble 되었음을 나타낸다.
- Missed speech: ensemble 모델들의 평균 정도의 성능을 보인다.
Conclusion
- DOVER 는 Speaker Confusion 관점에서 성능 향상을 보였지만, 전반적인 DER 과 overlap 측면에서는 아쉬움을 보였다.
- DOVER-lap 은 적절한 global speaker label를 3D matching을 통해서 찾아내었으며, overlap 문제 또한 효과적으로 해결하여 Ensemble를 통한 성능 향상을 보였다.
Still Remain Limitation
- Cannot effectively combine mixed-type hypotheses (e.g. 2 with overlaps and 1 without)
- Greedy search used to get maximal matching from cost tensor -> Can be improved?