1) 어텐션 메커니즘 (Attention Mechanism)
1. 어텐션(Attention)의 아이디어
2. 어텐션 함수(Attention Function)
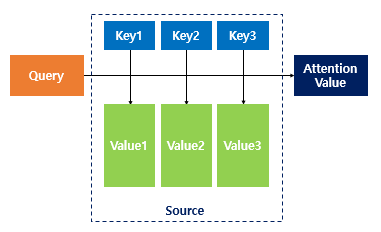
Attention(Q, K, V) = Attention Value
- 어텐션 함수는 주어진 쿼리에 대해서 모든 키와의 유사도를 각각 구한다. 구한 유사도를 키와 맵핑되어있는 각각의 값에 반영해준다. 유사도가 반영된 갓을 모두 더해서 리턴하는데 이 값을 어텐션 값이라고 한다
Q = Query : t 시점의 디코더 셀에서의 은닉 상태
K = Keys : 모든 시점의 인코더 셀의 은닉 상태들
V = Values : 모든 시점의 인코더 셀의 은닉 상태들
3. 닷-프로덕트 어텐션(Dot-Product Attention)
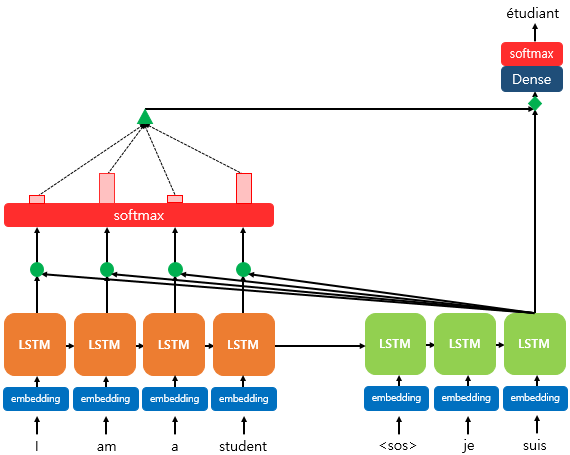
<세번째 LSTM 셀에서 출력 단어 예측할 때의 모습. 이와 같은 과정이 매 셀에 일어난다>
- 인코더의 모든 입력 단어들의 정보를 다시 한번 참고
- 소프트맥스 함수 : I, am, a, student 단어 각각이 출력 단어를 예츨할 때 얼마나 도움이 되는지 정도를 수치화한 값
- 이 값을 하나의 정보로 담아서 디코더로 전송 -> 더 정확히 예측
1) 어텐션 스코어(Attention Score)를 구한다.
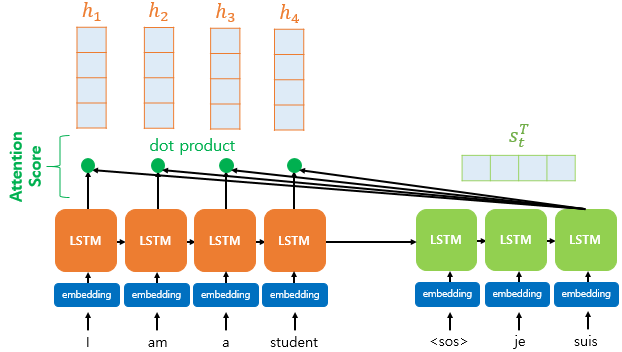
-
h1, h2, ...., hn : 인코더의 시점 1~N에서의 인코더의 은닉 상태
-
St : 디코더의 현재시점 t에서의 디코더의 은닉 상태
-
인코더와 디코더의 은닉상태 차원이 같다고 가정
-
디코더의 현재 시점(t)에서 필요한 값 :
- 이전 시점 (t-1) 의 은닉 상태
- 이전 시점 (t-1) 의 출력 단어
- 어텐션 매커니즘에서 추가 ) 어텐션 값 (at)
-
어텐션 스코어 (at) : 현재 디코더 시점 t에서 단어를 예측하기 위해 인코더의 모든 은닉 상태 각각이 디코더의 현 시점의 은닉 상태 St와 얼마나 유사하지를 판단하는 스코어 값 (스칼라 값)
-
닷 프로덕트 어텐션에서는 어텐션 스코어를 구하기 위해 St를 전치하고 각 은닉 상태와 내적
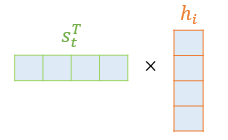
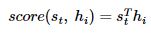

2) 소프트맥스(softmax) 함수를 통해 어텐션 분포(Attention Distribution)를 구한다.
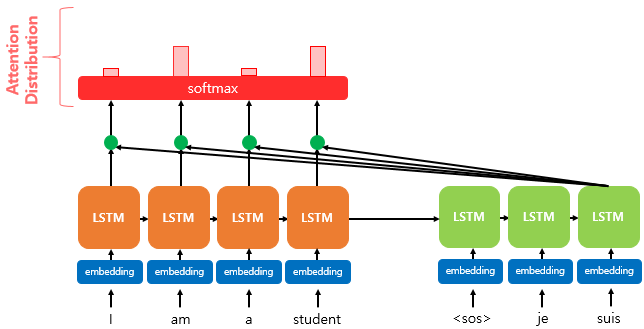

3) 각 인코더의 어텐션 가중치와 은닉 상태를 가중합하여 어텐션 값(Attention Value)을 구한다.
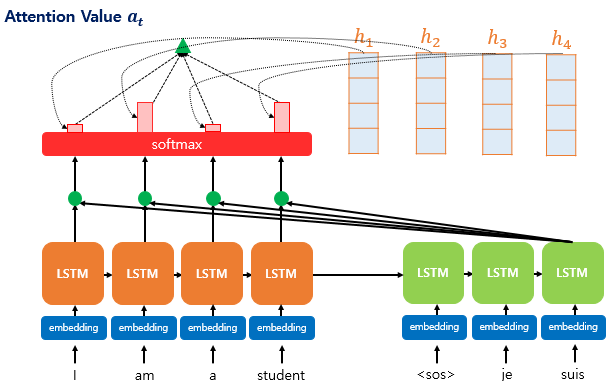
- 모든 정보를 하나로 합치는 단계
- 가중합 (Weighted Sum) : 각 인코더의 은닉 상태와 어텐션 가중치를 곱하여 모두 더함
- 어텐션 값 (Attention Value) = 컨텍스트 벡터 (Context Vector)

4) 어텐션 값과 디코더의 t 시점의 은닉 상태를 연결한다.(Concatenate)
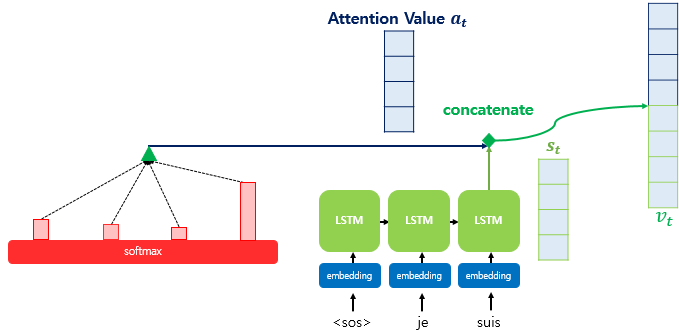
- 벡터를 하나로 만드는 작업 : at와 st를 결합
- 이 값을 y 값 예측의 입력으로 사용한다
5) 출력층 연산의 입력이 되는 St 를 계산합니다.
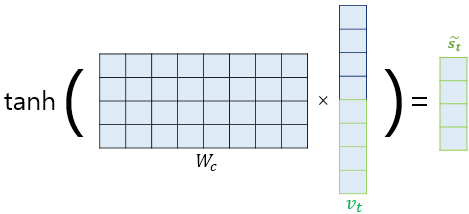
- 가중치 행렬과 곱한 후 하이퍼볼릭탄젠트 함수를 지나도록하여 출력층 연산을 위한 새로운 벡터인 st를 얻는다

6) St를 출력층의 입력으로 사용합니다.
- st를 출력층의 입력으로 사용하여 예측벡터를 얻는다

4. 다양한 종류의 어텐션(Attention)
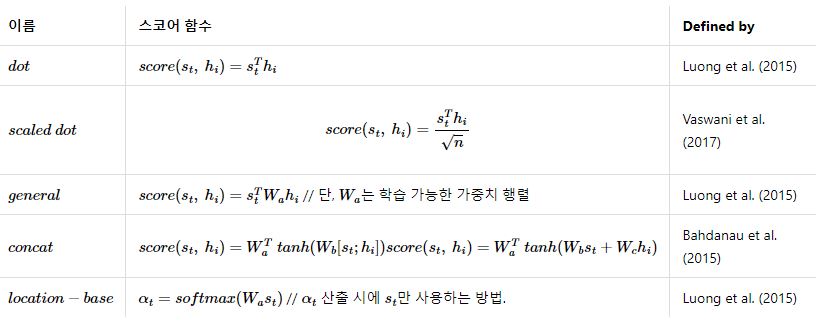
2) 바다나우 어텐션(Bahdanau Attention)
1. 바다나우 어텐션 함수(Bahdanau Attention Function)
Attention(Q, K, V) = Attention Value
t = 어텐션 메커니즘이 수행되는 디코더 셀의 현재 시점을 의미.
Q = Query : t-1 시점의 디코더 셀에서의 은닉 상태
K = Keys : 모든 시점의 인코더 셀의 은닉 상태들
V = Values : 모든 시점의 인코더 셀의 은닉 상태들
2. 바다나우 어텐션(Bahdanau Attention)
1) 어텐션 스코어(Attention Score)를 구한다.
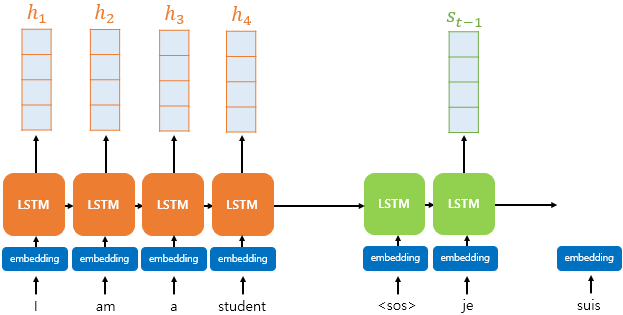

- W : 학습 가능한 가중치 행렬 & H : h1~hn 을 하나의 행렬로 표현

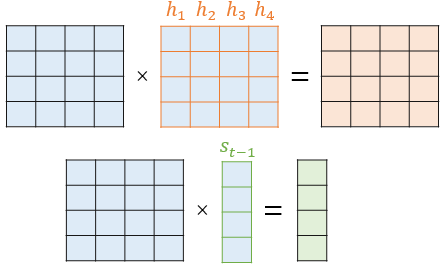
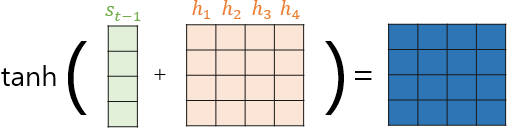

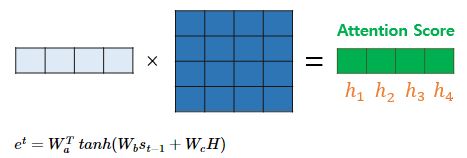
2) 소프트맥스(softmax) 함수를 통해 어텐션 분포(Attention Distribution)를 구한다.
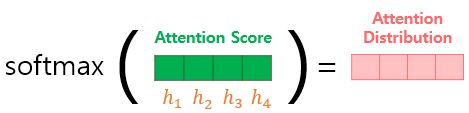
3) 각 인코더의 어텐션 가중치와 은닉 상태를 가중합하여 어텐션 값(Attention Value)을 구한다.
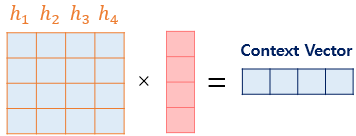
4) 컨텍스트 벡터로부터 St를 구합니다.
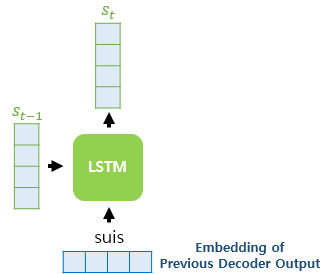
- 기존의 LSTM : 이전 시점의 셀로부터 전달받은 은닉상태 (St-1) + 현재 시점의 입력 (xt)로 연산
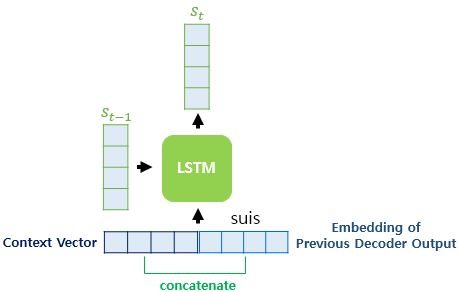
- 바다나우 어텐션 : (컨텍스트벡터& 현재시점 입력 단어의 임베딩 벡터 concat) + 이전 시점의 은닉 상태 (St-1)