Semantic segmentation
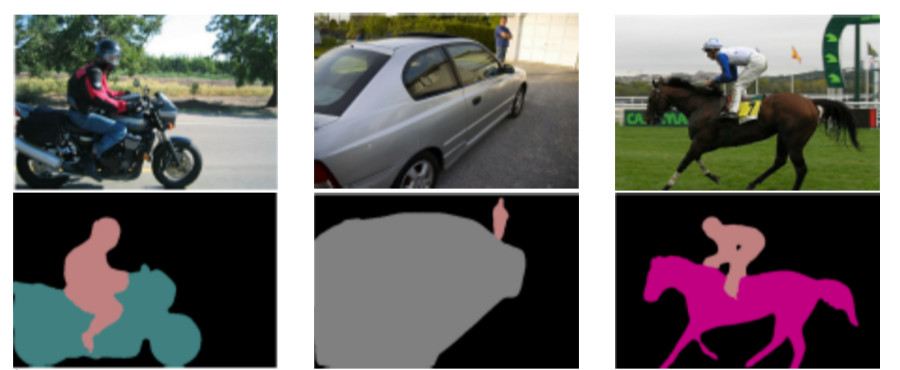
- Image classification을 영상 단위가 아니라 pixel 단위로 시행하고, 같은 class는 모두 같은 물체로 판단
- 이미지에 있는 모든 픽셀에 대한 예측을 하는 것이기 때문에 dense prediction 이라고도 불림
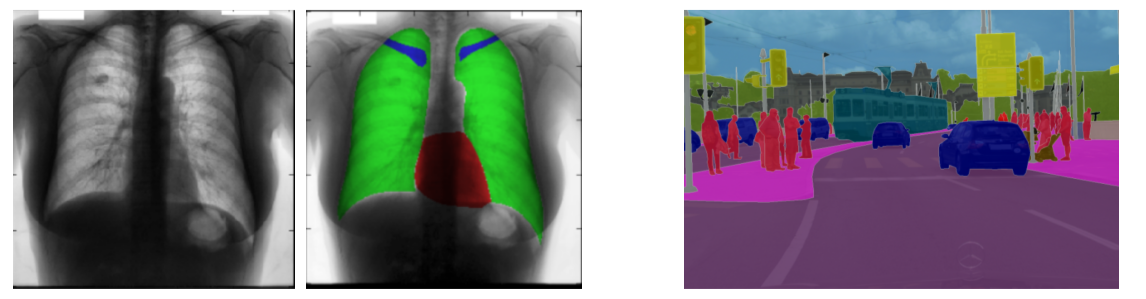
- 영상 컨텐츠에 대한 이해
- 특정 object에 대한 처리(computational photography)
Sematic segmentation architectures
FCN, Fully Convolutional Networks
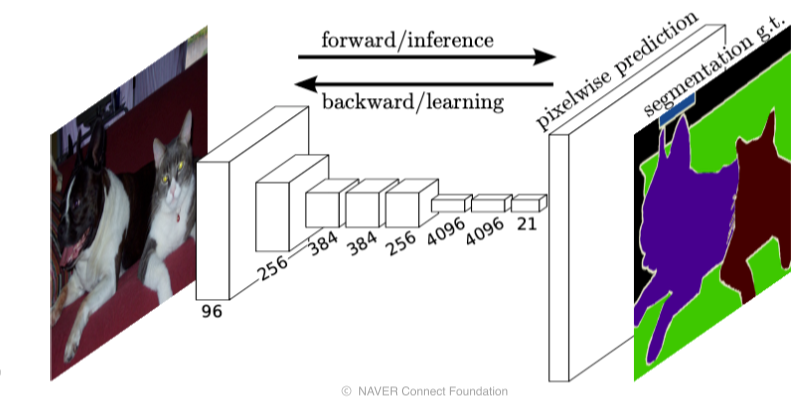
- First end-to-end architecture for semantic segmentation
end-to-end
: 입력부터 출력까지 모두 미분 가능한(학습 가능한) NN의 형태
입력&출력만 있으면 NN을 학습시켜서 target task 수행 가능
- 이전까지는 사람의 손으로 알고리즘을 결합해 semantic segmentation을 수행했었기 때문에 data가 많아도 학습 가능한 부분이 제한적이었음
- FCN은 input의 해상도가 자유롭고, 출력도 input의 해상도에 따라 맞출 수 있고, 그 사이의 NN이 모두 학습 가능한 형태기 때문에 문제를 바로 풀 수 있게 됨
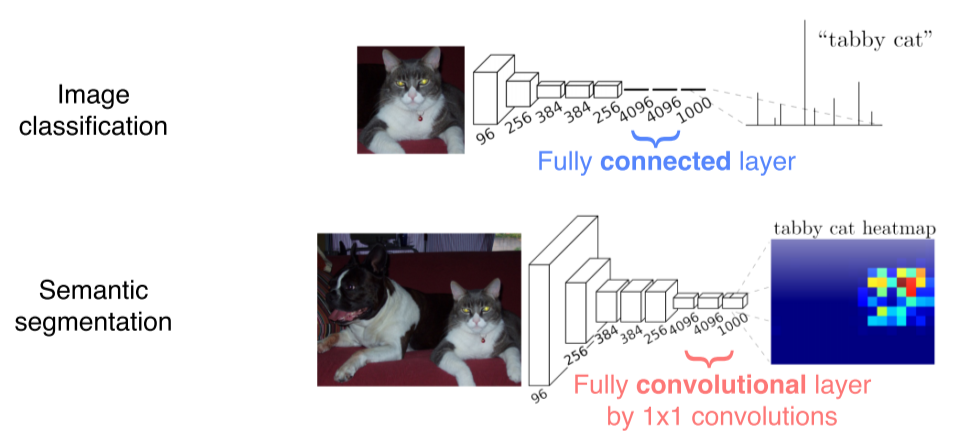
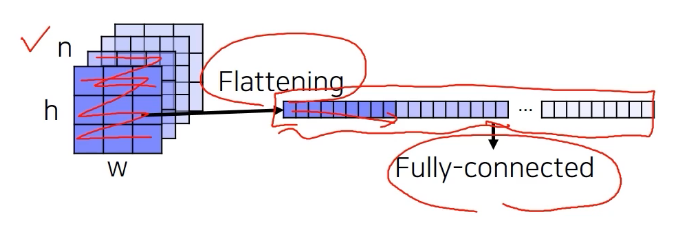
- Fully connected layer: 공간 정보를 고려하지 않고 fixed dimensional vector가 주어지면 또다른 fixed dimensional vector로 출력
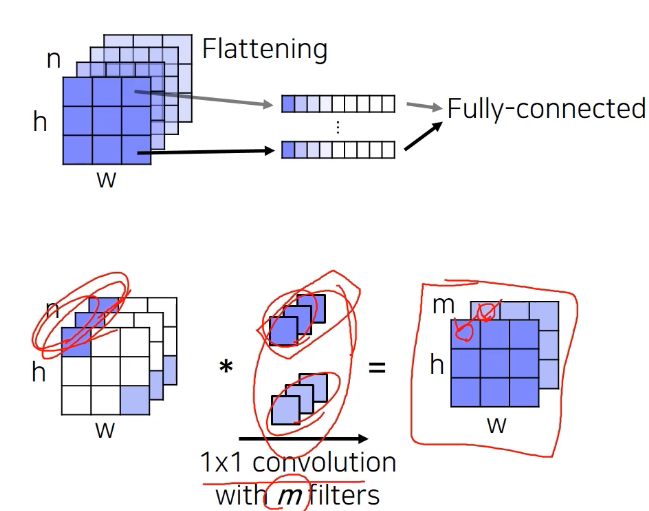
- Fully convolutional layer: 입출력이 모두 activation map 형태라서 spatial coordinate가 유지된 형태로 수행됨, 1x1 conv로 구현
--> 공간에 대한 정보가 남아있다
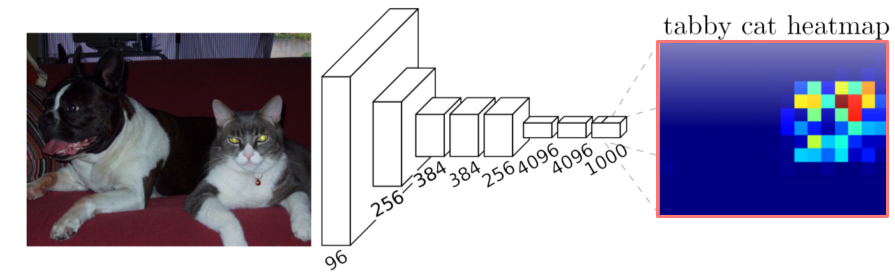
- 더 넓은 context를 판단하고 더 정답에 가까운 결론을 얻고싶어 stride, pooling layer를 이용해 receptive field를 키움
- 하지만 receptive field를 키우면 최종 activation map의 해상도는 매우 low한 trade-off가 발생
- 이를 해결하기 위해 upsampling layer를 이용
Transposed convolution
Convolution 계산을 하면 feature map이 작아진다. 반대로 feature map이 커지게 하려면 어떻게 해야할까? 단순하게 생각해보면 Convolution 계산과정을 역으로 하면 된다.
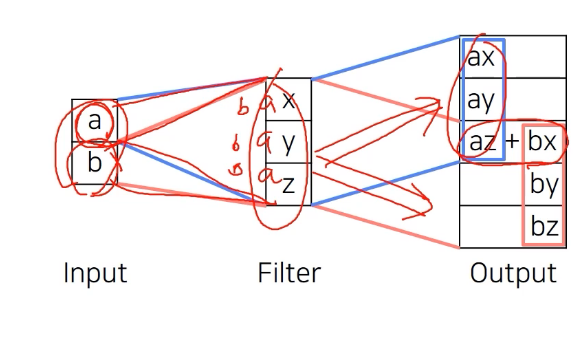
- Input에 filter를 먹여서 겹치게 output을 출력해서 크기를 키움
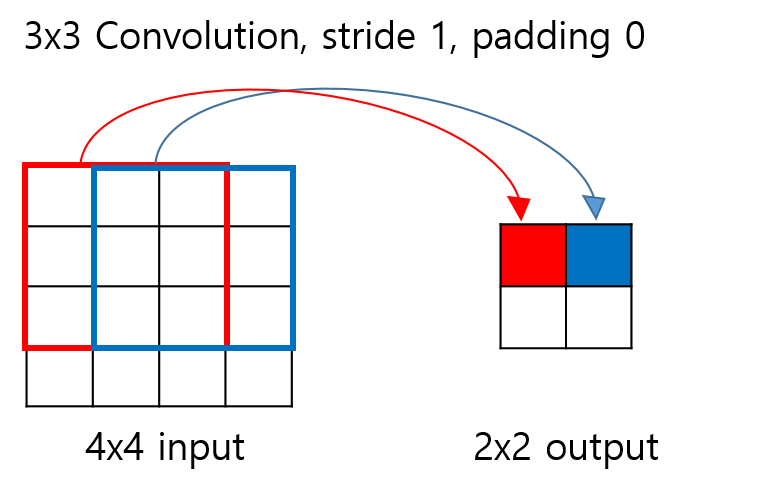
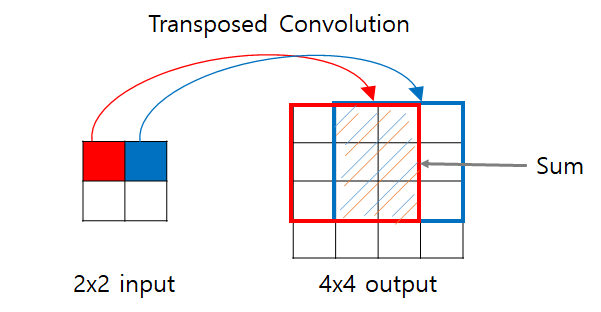
-
Transposed Convolution에서는 input의 빨간색 원소를 3x3 kernel에 곱해서 output의 대응하는 자리에 집어넣는다.
-
같은 방법으로 input의 파란색 원소를 3x3 kernel에 곱해서 output의 대응하는 위치에 집어넣는다.
-
이 때 output에 겹치는 구간(빗금 표시)이 발생하는데, 겹치는 부분의 값은 모두 더해준다.
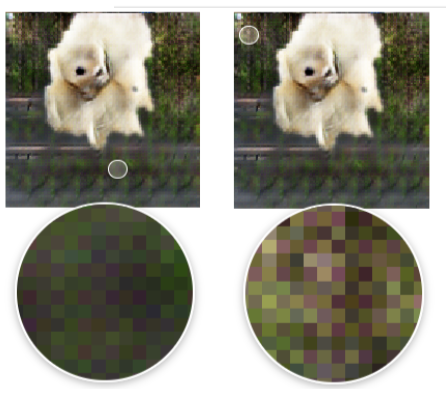
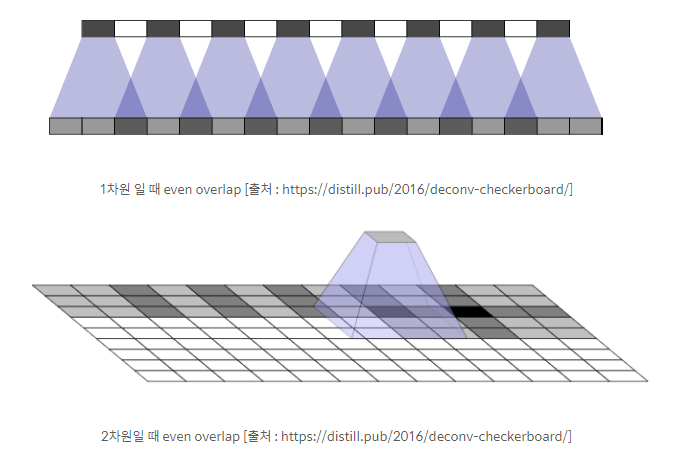
- Transposed convolution를 사용할 땐 위와 같은 checkboard artifact가 생기지 않도록 convolution size와 stride parameter를 잘 tuning해줘야 함
Upsampling
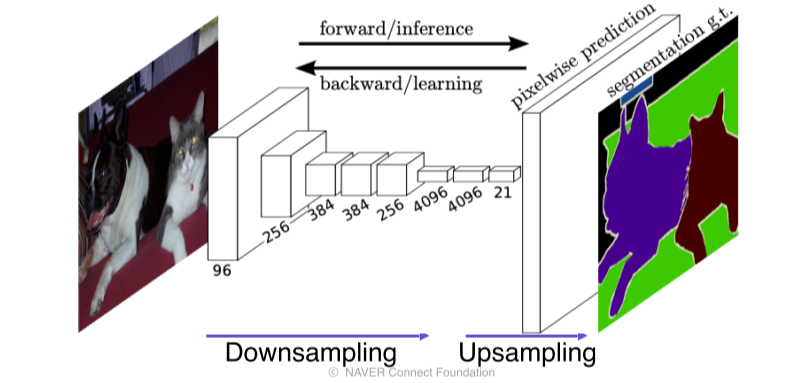
-
Stride, pooling을 제거하면 고해상도의 activation map을 얻게되나 receptive field의 size가 작기 때문에 영상의 전반적인 context를 파악하지 못하는 trade-off
-
그래서 일단은 작게 만들어서 receptive field를 최대한 키워 성능을 높이고 나중에 upsampling으로 강제로 해상도를 맞춰줌
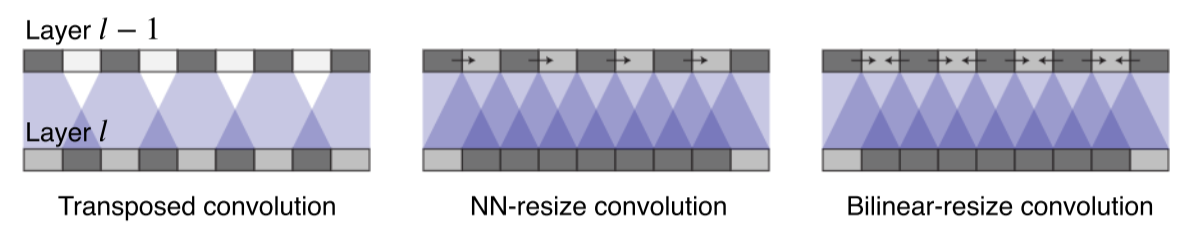
-
Transposed convolution은 애매하게 일부분만 overlap(중첩)되는 문제가 생겼던 반면에, upsampling은 그런 중첩 문제가 없고 골고루 영향을 받게 만듬
-
Upsampling convolution은 upsampling operation을 2개로 분리
- Nearest-neighbor(NN), Bilinear 같은 interpoltaion을 먼저 적용
이땐 학습 가능한(learnable) parameter가 들어있지 않음
- 여기에 학습 가능한 learnable upsampling으로 만들어주기 위해 convolution layer를 적용
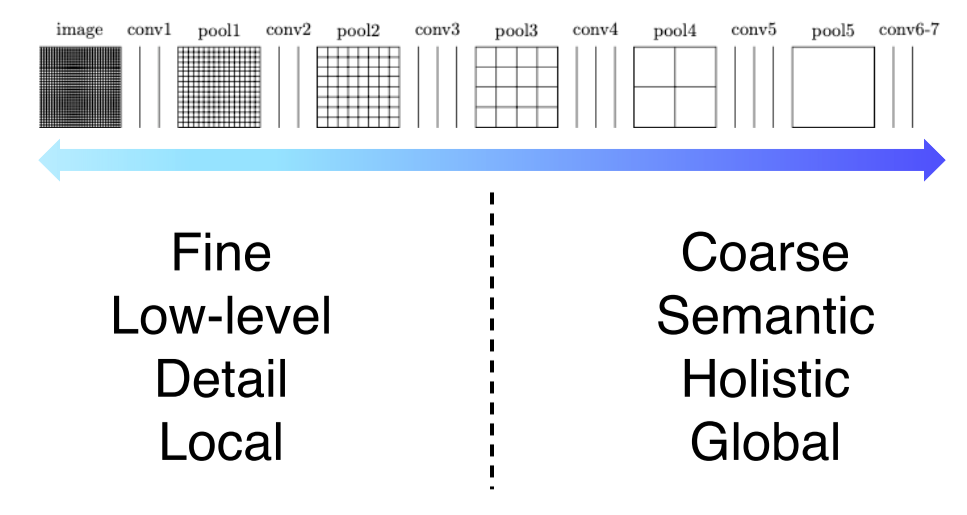
- 위는 각 layer별로 activation map의 해상도와 의미에 대한 정보
- semantic segmentation에 필요한 것은 둘 모두(전체적인 정보+경계에 대한 detail)
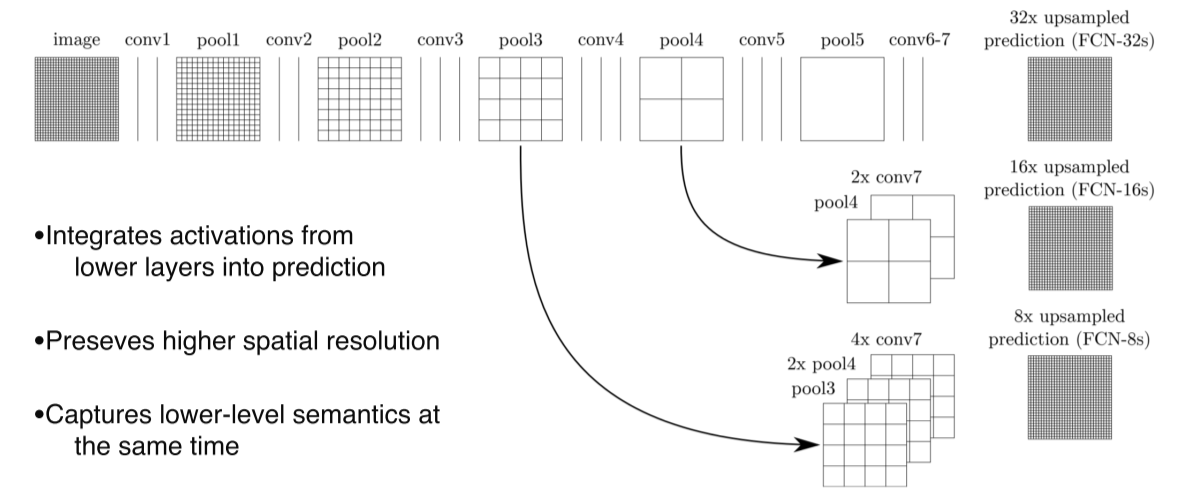
- 각 layer의 activation map을 concat해서 최종 output을 만들어서 각 class마다의 score를 출력
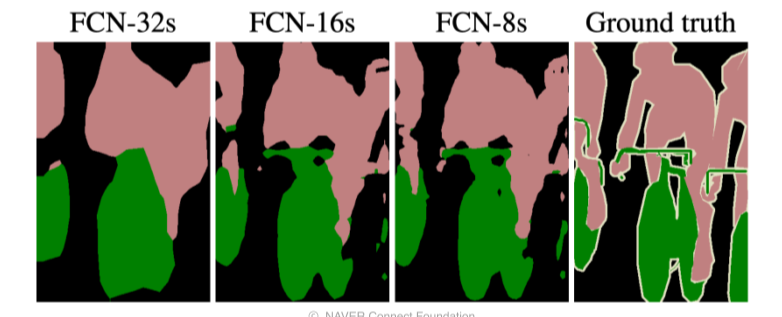
- 중간단계의 특징들을 합쳐서 쓰는 것이 도움이 되는 것을 볼 수 있음
HyperColumns for object segmentation
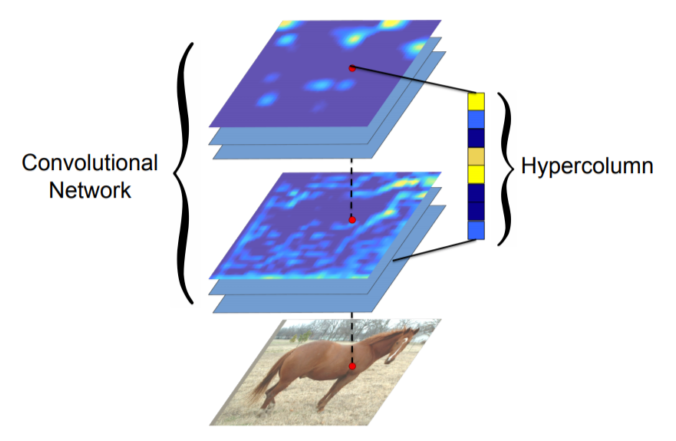
- Hypercolumn at a pixel is a stacked vector of all CNN units on that pixel
- FCN과 마찬가지로 낮은 layer와 높은 layer의 특징을 해상도를 맞춰놓고 합쳐서 사용하는 것을 제시
- Fine localized information is extracted from earlier layers
- Coarse semantic information is extracted from latter layers
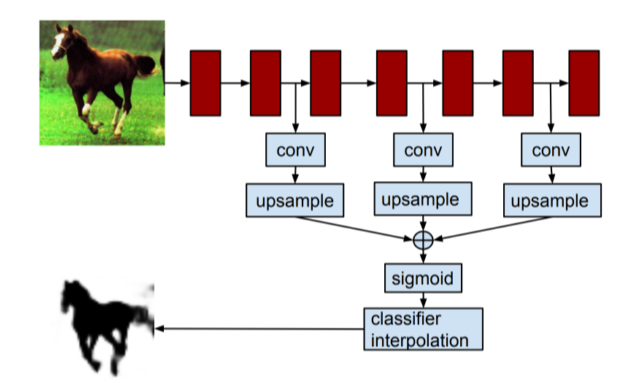
U-Net
-
영상과 비슷한 size의 출력을 가지는 모델이나 object detection, segmentation 등 영상 전체가 아니라 일부분을 좀 더 자세하게 봐야되는 기술들은 U-Net에 기원을 둔게 많음
-
Fully convolutional
-
Skip connection으로 낮은 층의 feature와 높은 층의 feature를 결합하는 방법을 제시해서 더 정교한 segmentation을 구현
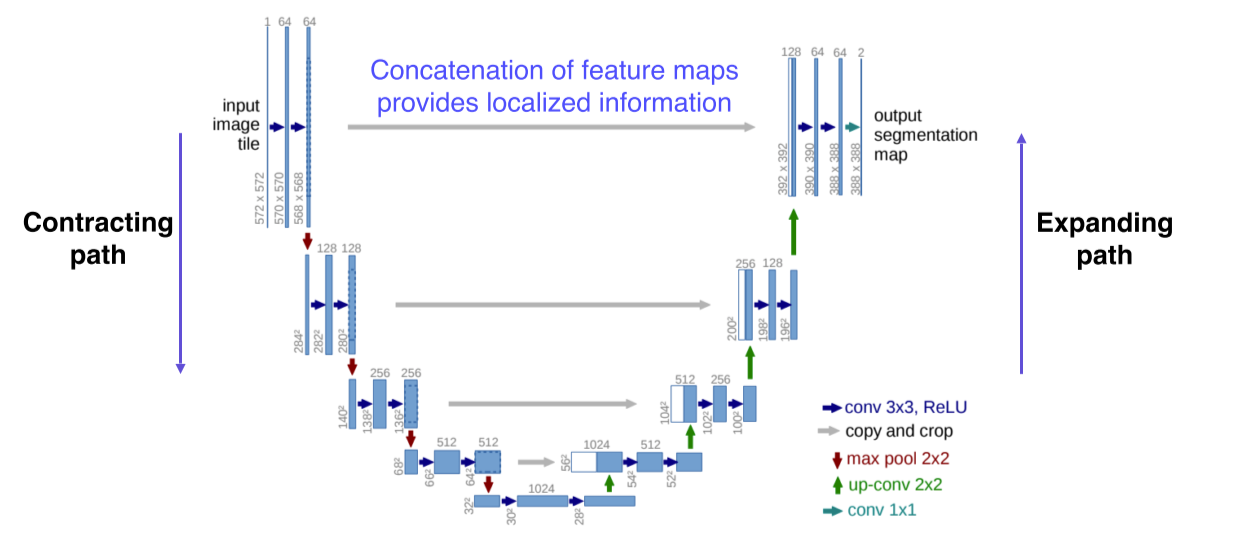
- 낮은 layer에서 전달된 특징이 localized 된 정보를 준다
- 공간적으로 높은 해상도와 입력이 약간 바뀌는 것만으로 민감한 정보를 제공하기 때문에 경계선이나 공간적으로 중요한 정보들은 뒤쪽 layer에 바로 전달하는 중요한 역할을 한다
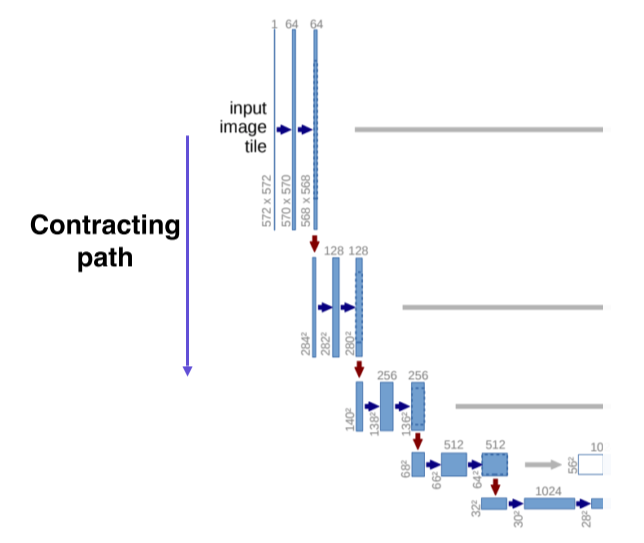
- Contracting path, encoding
- 입력 영상을 3개의 layer에서 convolution시키고 pooling을 통해 (receptive field를 크게 확보하기 위해서) 해상도를 낮추고 channel 수를 2배로 늘림(64->128)
- 이 과정을 몇 번 거쳐서 작은 activation map을 구하고 거기에 영상의 전반적인 정보가 잘 녹아있다고 가정
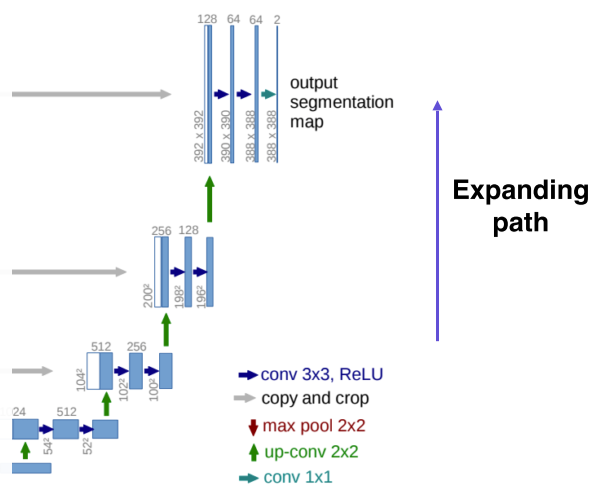
- Upsampling, expanding path, decoding
- Upsampling하는 대신에 단계적으로 activation map의 해상도와 channel size를 늘려줌
- Activation map의 해상도와 channel size는 contracting path에서부터 오는, 대칭되는 layer와 맞춰서 낮은 층에 있는 activation map을 합쳐서 사용할 수 있게 만들어줌
- Channel 수는 절반으로 줄어들면서 해상도는 늘어나는 구조
- 합치는건 concat을 사용
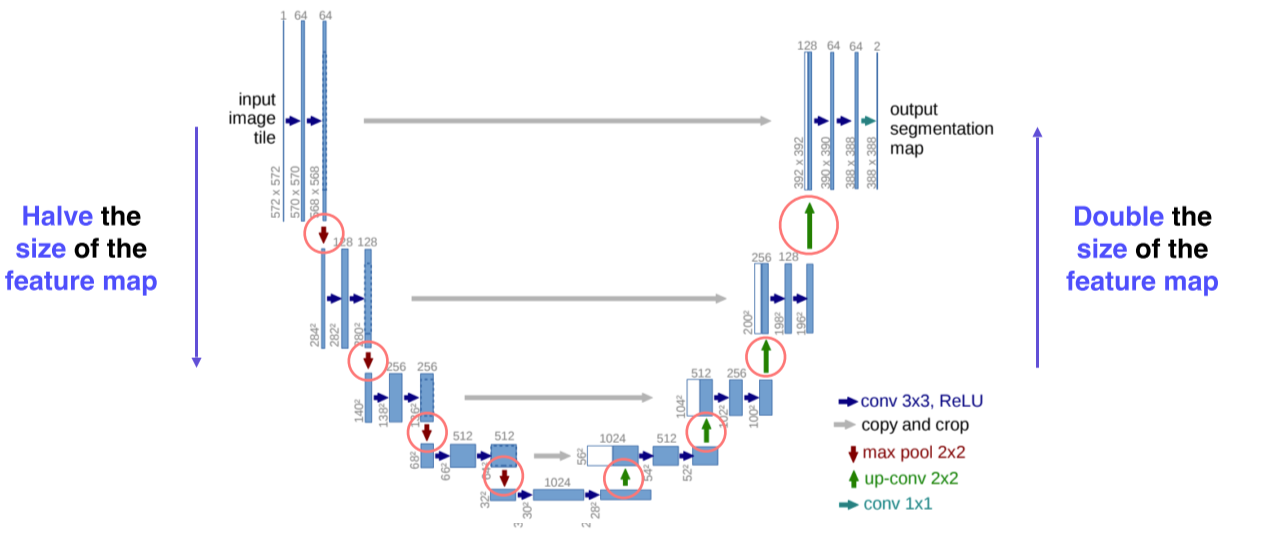
- Activation(feature) map의 해상도는 절반씩 줄고, 2배로 늘어남
- Channel 수는 2배씩 늘고, 절반으로 줄어듬
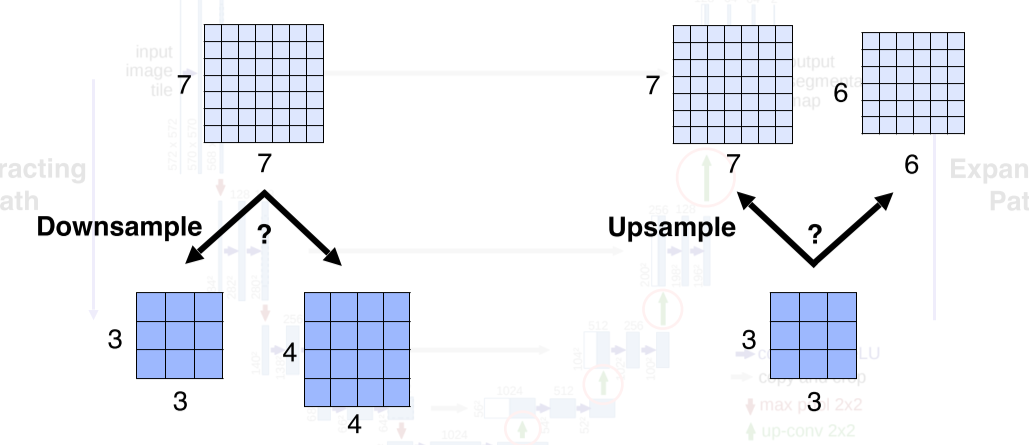
- U-Net에서는 downsampling과 upsampling이 굉장히 빈번하게 일어나기 때문에 feature map의 size는 항상 짝수여야한다는 특징이 존재
DeepLab
Segmentation에서 한 획을 그었던 논문
- CRF(Conditional Random Field)라는 후처리
- Atrous(Dilated) Convolution의 사용
CRF, conditional random field
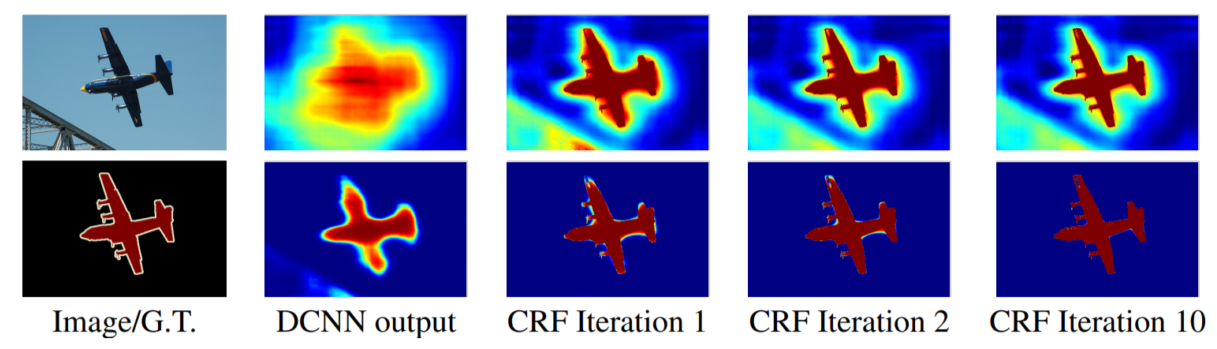
-
Pixel간의 관계를 이어서 regular한 grid(pixel map)을 관찰
-
모든 NN model은 처음 NN을 하면 DCNN output과 같이 굉장히 blur한 output이 나오는 단점이 존재
-> 입력이 feeding 되고 나서 출력이 나오고, 그 출력을 다시 입력과 비교대조하는 feedback이 없는 feed-forward 구조이기 때문
-
이를 해결하기 위해 출력 score map과 이미지의 edge 같은 경계선을 뽑아 score map이 경계선에 잘 맞도록 확산을 시킴
-
반대로 background에 대한 score는 물체 경계까지 확산돼서 fore-background의 경계선을 굉장히 tight하게 만들어주는 알고리즘
Dilated Convolution
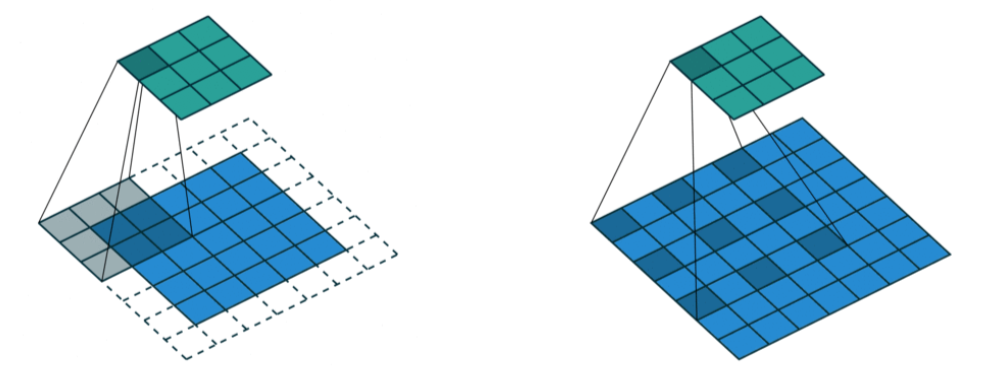
-
convolution 할 때 dilation factor만큼 일정 공간을 넣어줌
위에서는 weight 사이를 1칸씩 띄어서 parameter 수는 늘리지 않으면서 실제 convolution kernel보다 넓은 영역을 고려할 수 있게 만듬
-
Dilated Convolution을 반복 사용해서 receptive field가 exponential하게 증가하는 효과를 얻을 수 있다
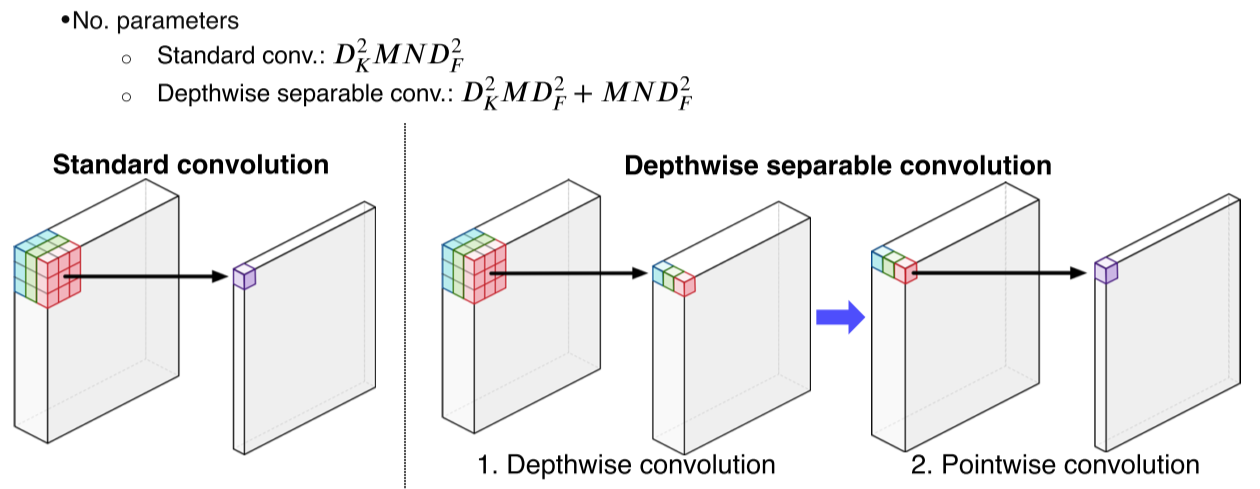
-
DeepLab v3에서는 semantic segmentation의 입력해상도가 워낙 크기 때문에 연산이 오래걸리는 것을 줄이기 위해 dilate convolution을 depth-wise convolution과 결합한 Astous Convolution을 사용
-
기존 convolution은 channel 전체에 걸쳐 내적을 했던 반면, astrous convolution은 depthwise-pointwise로 나눔
DeepLab v3+
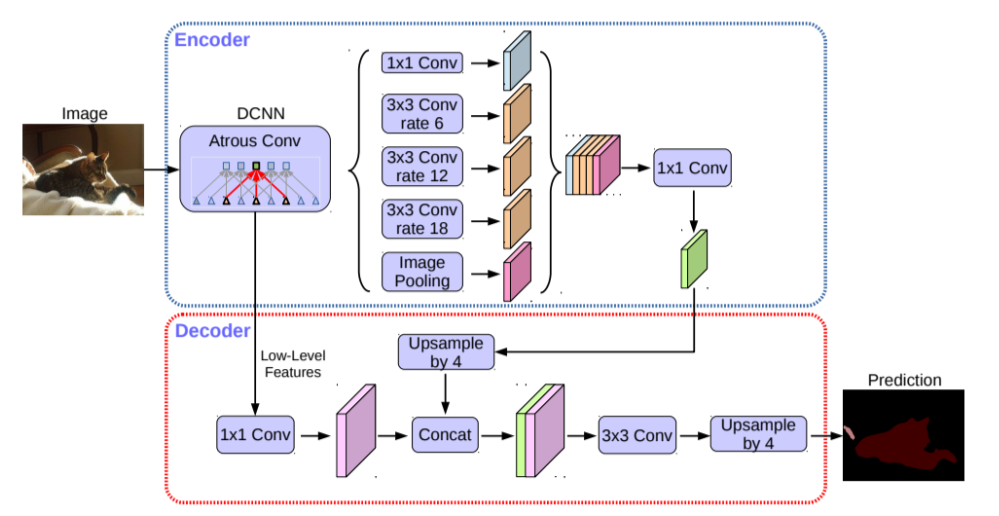
- 가장 최신 모델
- Encoder
- DCNN에서 dilated convolution으로 더 큰 receptive field를 갖는 CNN을 적용해서 feature map을 구함
- 그 후 Astrous spatial pyramid pooling을 통해 다양한 rate의 dilate conv를 통해 multi-scale을 처리
- 이렇게 구해진 다양한 feature map은 concat을 통해 1x1 conv시킴
- Decoder
- Low level feature를 구하고, pyramid pooling을 거친 feature를 upsampling한 결과를 concat으로 결합
- 다시 upsampling으로 최종 segmentation map을 추출
Reference
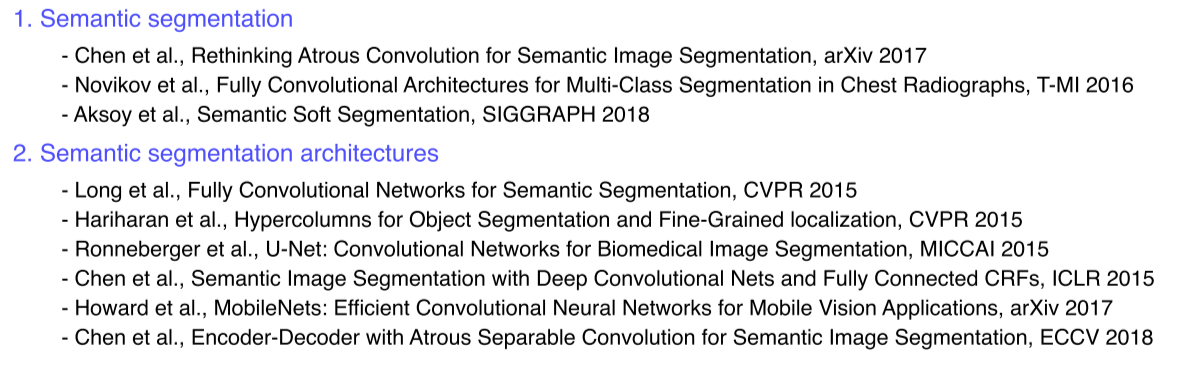