[Object Detection] 4-1. YOLO v3 : dataset 준비
YOLO v3
Architecture
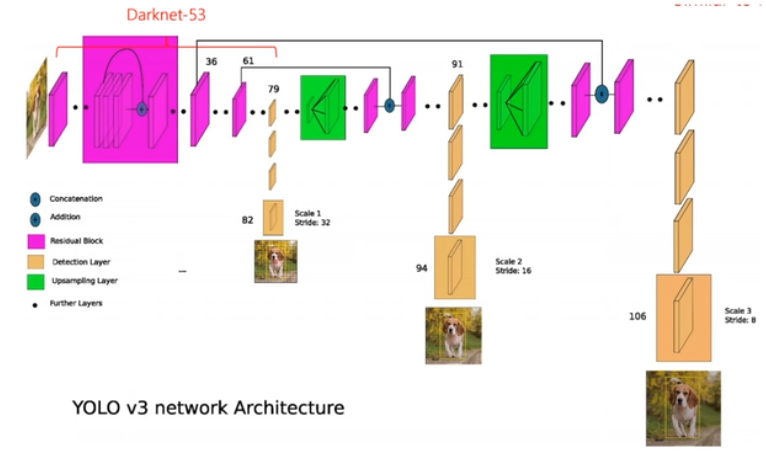
- backbone으로 Darknet-53 사용하여 feature map 생성
- 3개의 feature map을 추출하여 bbox를 예측한다
- 13x13, 26x26, 52x52 3가지의 scale output이 나온다
- 13x13는 큰 object를, 52x52는 작은 object를 찾는데 장점이 있다
- cfg file을 사용하여 model을 만든다
- cfg file에 포한된 내용들
- model architecture
- hyperparameter : lr, step 등등
- data augmentation
- input resolution
prepare data
- 우리의 경우 PASCAL VOC format을 사용하지만 annotaion은 xml이 아니라 txt 파일로 사용한다

- 이미지 파일과 그에 해당하는 annotaion file이 한 폴더에 있다
- train.list : train에 필요한 데이터들의 경로가 들어있다
- 이 경우 train.list 파일을 읽어서 이미지를 가져오고 같은 폴더에 있는 해당 annotaion 파일도 가지고 온다
- YOLO labeling format
- class_index, x_center, y_center, width, height
- x, y, w, h는 normalized되어 0~1 사이의 값이다
- 이미지와 해당 annotaion 정보가 서로 다른 폴더에 있다
- 이렇게 분류해놓는 것이 관리하기 편하다
KITTI dataset 다운
- object의 type
- 9개의 class
- car, ven, truck, pedestrian(보행자), person_sitting, cyclist, tram, misc(애매한 차량들), dontcare(labeling 하지 않아야한 것, 너무 멀거나, 잘렸거나)
- truncated
- 이미지 밖으로 나간 정도
- 0 : non-truncated
- 1 : truncated
- occluded
- object에 의해 가려진 정도
- 0 : fully visibel
- 1 : partly occluded
- 2 : largely occluded
- 3 : unknown
- alpha
- bbox
- 4개의 값
- xmin, y_min, x_max, y_max
- dimensions
- location
- 3개의 값
- x, y, z (camera coordinated)
- rotation_y
- score
- 5개의 data
- class_index, x_center, y_center, width, height
- 학습할 data가 어떻게 annotation 되어 있는지, 각 class릐 정보가 어떤 object를 의미하는지, object가 어떤 특성이 있는지, truncation과 occlusion이 어떻게 되어있는지 확인해야한다
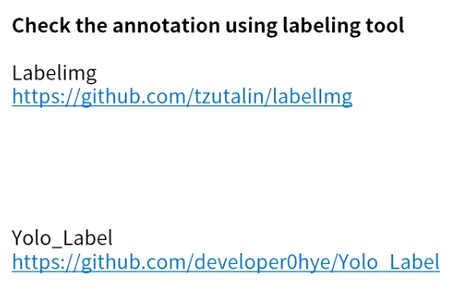