Abstract
Input video의 structure에 visual & textual description의 content를 주입하는 'Structure and content-guided video diffusion model' 제안. Monocular depth estimation이 structure & content fidelity를 제어하는데 유용함을 보였으며, temporal consistency에 explicit control을 부여하는 새로운 guidance 기법을 제시함.
1. Introduction
-
Temporal consistency와 spatial detail을 모두 살려야 하는 video editing process.
-
본 논문의 모델은 1) 주어진 이미지나 프롬프트와 일관된 영상을 생성하며, 2) input video의 structure에 대한 의존도를 조절할 수 있는 information obscuring process가 적용되어 있고, 3) classifier-free guidance로 temporal consistency를 유지.
3. Method
-
영상을 구성하는 요소를 다음의 두 가지로 구분한다.
- Structure: 물체의 형태와 위치, 시간적 움직임에 해당하는 geometry와 dynamics
- Content: 물체의 색과 스타일, 장면의 조명 등에 해당하는 appearance와 semantics
-
Input video에서 얻어낸 structure에 대한 condition s와, text prompt/image에서 얻어낸 content에 대한 condition c로부터 video x를 생성하는 모델 p(x∣s,c)를 구성하는 것이 목표.
Latent Diffusion Models
- v-parameterization이 color consistency를 유지하는데 유용함을 발견.
Spatio-Temporal Latent Diffusion
- x∈R3×H×W−(Encoder)→x∈R4×H/8×W/8 의 LDM encoder 사용.
- Temporal Extension: Video input에 활성화되는 temporal layer 추가(이외의 모든 layer는 image와 video model이 서로 공유). Temporal axis에 대한 convolution/attention layer가 포함된 residual/attention block 사용.
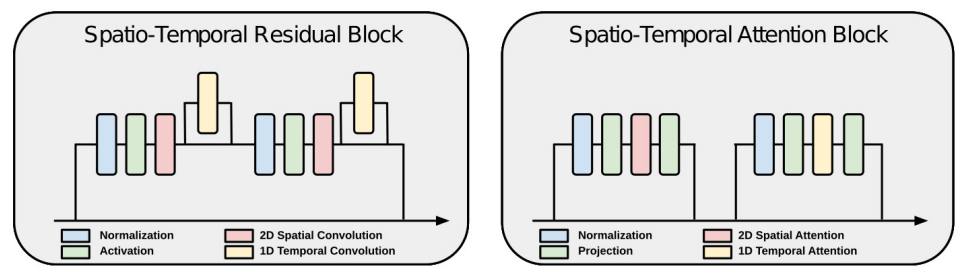
Representing Content and Structure
Conditional Diffusion Models
- Paired video-text dataset 없이 video x에 대해, structure s=s(x)와 content c=c(x)를 추출하여 학습에 사용함.
Per-example loss λt∣∣μt(E(x)t,E(x)0)−μθ(E(x)t,t,s(x),c(x))∣∣2
- Inference 시에는 input video y와 text prompt t로부터 structure s=s(y)와 content c=c(t) 추출.
z∼pθ(z∣s(y),c(t)), x=D(z)
Content Representation
- Training stage에서의 target video x에 대해선 임의로 frame을 한 장 선택해 CLIP image embeddings 사용. Reference stage에서는 prompt의 text embedding에서 image embedding 추출.
Structure Representation
- Content와 structure 간 최대한의 분리를 위해 depth estimates를
4. Results, 5. Conclusion
(생략)